BI MW 1, 2, and 3 PDF
Document Details
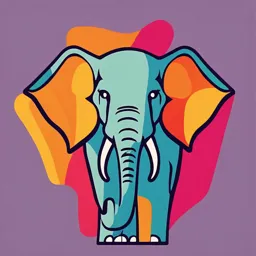
Uploaded by PleasurableCharacterization4936
Marywood University
2024
Rasha Abdelaziz Youssef
Tags
Related
- Practical Applications of Data Analytics Chapter 2 PDF
- Big Data and Business Intelligence PDF
- Business Intelligence PDF
- Technologies Business Intelligence - Big Data Analytics Master 2_S3 - PDF
- Business Intelligence, Analytics, and Data Science: A Managerial Perspective PDF
- Business Intelligence, Analytics, and Data Science: A Managerial Perspective - PDF
Summary
This document is course material for a Business Intelligence course in a Master of Business Administration program. Topics covered include the role of BI in decision-making, data analytics, data warehousing, and ethical/legal issues. The content also describes different types of organizations, networks, and functional/enterprise systems structures.
Full Transcript
Master of Business Administration Graduate School of Business Management Information Systems Major...
Master of Business Administration Graduate School of Business Management Information Systems Major Marywood University – Pennsylvania Program September 2024 Business Intelligence Prof. Dr. Rasha Abdelaziz Youssef Head of Business Information Systems dept. (AASTMT) Principal Investigator [Internationally Funded Projects by the British Council] Rasha Abdelaziz Youssef – AI in Business Organization TREY research 1 Course Instructor Prof. Dr. Rasha Abd El Aziz, PhD, SFHEA Professor and Head of Business Information Systems Department PhD: The University of the West of England, Bristol, England, UK MSc: Master of Business Administration, AASTMT Bachelor: Business Administration, Major MIS (1st with honour) Email: [email protected] https://www.linkedin.com/in/rasha-abd-el-aziz-822b7a33/ www.facebook.com/rasha.ayo TREY research Course Assessments Your Grade will be divided to several assessments: Group Project Report & Presentation: 30 Marks Individual Assignment: 20Marks Written Exam: 40 Marks Attendance/Participation: 10 Marks TREY research Textbook: Business Intelligence, Analytics, Data Science, and AI, 5th ed Published by Pearson (August 30, 2023) © 2024 Ramesh Sharda Oklahoma State University Dursun Delen Oklahoma State University Efraim Turban Oklahoma State University, University of Hawaii 1-4 TREY research Digitalization, Systems, and Models Introduction to Business Intelligence 1 BI role in decision-making, tools and technologies Data Analytics and Decision Making Data Warehousing & ETL Processes 2 Descriptive Analytics Data Visualization and Reporting Data Mining and Predictive Analytics Course Prescriptive Analytics 3 Ethical/Legal Issues in BI Big Data and Business Intelligence Content 4 Group Presentations and Discussions Final Exam 5 Emerging Trends in BI and Artificial Intelligence TREY research Digital Terms and Basics - 1 E-commerce and E-Business Digitization/Digitalization/Digital Transformation E-Commerce: Digitization Converting non-digital content into digital format. Buying, selling, or exchanging products, services, or information electronically Digitalization A process/business model, E-Business: Digitalize the business process A broader definition of EC that includes not just the buying and selling of goods and services, but Digital Transformation also servicing customers, collaborating with Is digitalization but at scale. business partners, and conducting electronic Going across departments, transactions within an organization eliminating inf silos, unifying different software, or a companywide move Add a footer TREY research 6 Digital Terms and Basics - 2 Types of Organizations Types of Networks Brick-and-mortar: Internet: A global network of interconnected devices/computers. It is public and accessible to Organizations that perform their primary business anyone with internet connection to communicate and offline, selling physical products by means of physical collaborate across geographical boundaries. agents Intranet: An internal corporate or government network that uses Internet tools, such as Web Virtual/Pure-play: browsers, and Internet protocols but restricted to Organizations conduct business activities solely online authorized users. Extranet: A network that uses the Internet to link Click-and-mortar: multiple intranets. An extension of an intranet that Organizations conduct some EC activities usually as an allows authorized external users, such as customers, additional marketing channel partners, suppliers, or vendors, to access specific resources and services. Add a footer TREY research 7 Digital Terms and Basics - 3 Add a footer TREY research 8 Digital Terms and Basics - 4 Disintermediation* Reintermediation* Resistance to Change Add a footer TREY research 9 Digital Terms and Basics - 5 Data Information Knowledge Streams of raw facts. Data shaped into Information in action meaningful form. Add a footer TREY research 10 Digital Terms and Basics - 6 System Vendor System Type System Name Add a footer TREY research 11 Digital Terms and Basics - 7 Functional Systems structure Enterprise Systems structure The most common organizational structure. Divided into functions, or departments, each is responsible Comprehensive, integrated platforms that support for a set of closely related activities. core business processes and functions, such as finance, human resources, supply chain management, Typical functions/departments are purchasing, customer relationship management (CRM), and operations, R & D, Finance, HR, and IT. enterprise resource planning (ERP) across the By focusing on their steps, they lose the “big picture”. enterprise. This is referred to as the silo effect. Research & Development Information Technology Sales Warehouse Accounting Finance & Accounting Human Resources Sales Warehouse Sales & Marketing Accounting Receive Prepare Send Send Receive Customer Shipment Shipment Invoice Payment Order Purchasing Warehouse Operations Receive Prepare Send Send Receive Customer Shipment Shipment Invoice Payment Order Enter/Update Enter/Update Enter/Update Enter/Update Enter/Update Data Data Data Data Data Send Paperwork Send Paperwork Enter/Update Enter/Update Enter/Update Enter/Update Enter/Update Data Data Data Data Data Delay! Delay! Enterprise Information System Sales Warehouse Accounting Information Information Information System System System Add a footer TREY research 12 Business Intelligence and Analytics: Systems for Decision Support Business Intelligence, Analytics, and Decision Support Plan of the Course ◼ Part I – Introduction: ◼ Overview of BI, Analytics and Data Science ◼ Part II - Descriptive Analytics ◼ Nature of Data, Statistical Modeling and Visualization ◼ BI and DW ◼ Part III - Predictive Analytics ◼ Data Mining and Web Analytics ◼ Part IV - Prescriptive Analytics ◼ Optimization and Simulation ◼ Part V – Future Trends ◼ Big Data and Future Directions for Business Analytics ◼ Artificial Intelligence Changing Business Environment & Computerized Decision Support ◼ Companies are moving aggressively to computerized support of their operations Business Intelligence ◼ Business Pressures–Responses–Support Model ◼ Business pressures result of today's competitive business climate ◼ Responses to counter the pressures ◼ Support to better facilitate the process The Business Environment The environment today is complex, creating opportunities, and problems. Business environment factors: markets, consumer demands, technology, and societal… Organizational Responses ◼ Be Reactive, Anticipative, Adaptive, and Proactive ◼ Managers may take actions, such as ◼ Employ strategic planning. ◼ Use new and innovative business models. ◼ Restructure business processes. ◼ Participate in business alliances. ◼ Improve corporate information systems. ◼ … more [in your book] Closing the Strategy Gap Major Objective One of the major objectives of computerized decision support is to facilitate closing the gap between the current performance of an organization and its desired performance, as expressed in its mission, objectives, and goals, and the strategy to achieve them. Add a footer TREY research 18 Managerial Decision Making Defined Management is a process by which organizational goals are achieved by using resources. Inputs: resources Output: attainment of goals Measure of success: outputs / inputs Management Decision Making Decision making: selecting the best solution from two or more alternatives Add a footer TREY research 19 The Nature of Managers’ Interpersonal Work Mintzberg's 10 1. Figurehead Managerial Roles 2. Leader 3. Liaison Decisional 7. Entrepreneur Informational 8. Disturbance handler 4. Monitor 9. Resource allocator 5. Disseminator 10. Negotiator 6. Spokesperson Add a footer TREY research 20 Managers usually make decisions by following a four-step process 1. Define the problem (or opportunity) 2. Construct a model that describes the real-world problem. 3. Identify possible solutions to the modeled problem and evaluate the solutions. 4. Compare, choose, and recommend Decision-MakingProcess a potential solution to the problem. (a.k.a. the scientific approach) Add a footer TREY research 21 Characteristics of Decision Making Decision Support Systems (DSS) Dissecting DSS into its main concepts → Building successful DSS requires a thorough understanding of these concepts TREY research Decision Making Process A process of choosing among two or more alternative courses of action for the purpose of attaining a goal(s) Managerial decision making is synonymous with the entire management process - Simon (1977) Example: Planning What should be done? When? Where? Why? How? By whom? Add a footer TREY research 23 Decision-Making Disciplines Behavioral and Scientific Behavioral: law, political science, psychology Scientific: computer science, decision analysis, economics, engineering, the hard sciences (e.g., biology, chemistry, physics), management science/operations research, mathematics, and statistics Each discipline has its own set of assumptions and each contributes a unique, valid view of how people make decisions Add a footer TREY research 24 Decision-Making Disciplines Better decisions Tradeoff: accuracy versus speed Fast decision may be damaging Many areas suffer from fast decisions Effectiveness versus Efficiency Effectiveness → “goodness” “accuracy” Efficiency → “speed” “less resources” A fine balance is what is needed! TREY research Decision Style The manner by which decision makers think and react to problems perceive a problem cognitive response values and beliefs When making decisions, people… follow different steps/sequence give different emphasis, time allocation, and priority to each step TREY research Decision Style Decision Making Styles Decision-making styles Heuristic versus Analytic Autocratic versus Democratic Consultative (with individuals or groups) A successful computerized system should fit the decision style and the decision situation Should be flexible and adaptable to different users (individuals vs. groups) Add a footer TREY research 27 Decision Makers Small organizations Individuals Conflicting objectives Medium-to-large organizations Groups Different styles, backgrounds, expectations Conflicting objectives Consensus is often difficult to reach Help: Computer support, GSS, … TREY research Phases of Decision-Making Process Four Main Phases Humans consciously or subconsciously follow a systematic decision-making process - Simon (1977) 1) Intelligence 2) Design 3) Choice 4) Implementation 5) (?) Monitoring (a part of intelligence?) Add a footer TREY research 29 Simon’s Decision-Making Process TREY research Decision Making: Intelligence Phase Scan the environment, either intermittently or continuously Identify problem situations or opportunities Monitor the results of the implementation Problem is the difference between what people desire (or expect) and what is actually occurring Symptom versus Problem Timely identification of opportunities is as important as identification of problems TREY research Decision Making: Intelligence Phase Phase 1 Potential issues in data/information collection and estimation Lack of data Cost of data collection Inaccurate and/or imprecise data Data estimation is often subjective Data may be insecure Key data may be qualitative Data change over time (time-dependence) Add a footer TREY research 32 Decision Making: Intelligence Phase Problem Classification Classification of problems according to the degree of structuredness Problem Decomposition Often solving the simpler subproblems may help in solving a complex problem. Information/data can improve the structuredness of a problem situation Problem Ownership Outcome of intelligence phase → A Formal Problem Statement TREY research Decision Making:The Design Phase Phase 2 Finding/developing and analyzing possible courses of actions A model of the decision-making problem is constructed, tested, and validated Modeling: conceptualizing a problem and abstracting it into a quantitative and/or qualitative form (i.e., using symbols/variables) Abstraction: making assumptions for simplification Tradeoff (cost/benefit): more or less abstraction Modeling: both an art and a science Add a footer TREY research 34 Decision Making:The Design Phase Phase 2 Selection of a Principle of Choice It is a criterion that describes the acceptability of a solution approach Reflection of decision-making objective(s) In a model, it is the result variable Choosing and validating against High-risk versus low-risk Optimize versus satisfice Criterion is not a constraint! See Technology Insight 2.1 Add a footer TREY research 35 Decision Making:The Design Phase Phase 2 Normative models (= optimization) the chosen alternative is demonstrably the best of all possible alternatives Assumptions of rational decision makers Humans are economic beings whose objective is to maximize the attainment of goals For a decision-making situation, all alternative courses of action and consequences are known Decision makers have an order or preference that enables them to rank the desirability of all consequences Add a footer TREY research 36 Decision Making: The Design Phase ▸ Heuristic models (= suboptimization) ▹ The chosen alternative is the best of only a subset of possible alternatives ▹ Often, it is not feasible to optimize realistic (size/complexity) problems ▹ Suboptimization may also help relax unrealistic assumptions in models ▹ Help reach a good enough solution faster ▹ The rule of thumb ▹ An experienced trial and error TREY research 37 Decision Making:The Design Phase Phase 2 Descriptive models Describe things as they are or as they are believed to be (mathematically based) They do not provide a solution but information that may lead to a solution Simulation - most common descriptive modeling method (mathematical depiction of systems in a computer environment) Allows experimentation with the descriptive model of a system Add a footer TREY research 38 Decision Making: The Design Phase Good Enough, or Satisficing “something less than the best” A form of suboptimization Seeking to achieve a desired level of performance as opposed to the “best” Benefit: time saving Simon’s idea of bounded rationality TREY research Decision Making:The Design Phase Phase 2 Risk Lack of precise knowledge (uncertainty) Risk can be measured with probability Scenario (what-if case) A statement of assumptions about the operating environment (variables) of a particular system at a given time Possible scenarios: best, worst, most likely, average (and custom intervals) Add a footer TREY research 40 Decision Making:TheChoice Phase Phase 3 The actual decision and the commitment to follow a certain course of action are made here The boundary between the design and choice is often unclear (partially overlapping phases) Generate alternatives while performing evaluations Includes the search, evaluation, and recommendation of an appropriate solution to the model Solving the model versus solving the problem! Add a footer TREY research 41 Search approaches Analytic techniques (solving with a formula) Algorithms (step-by-step procedures) Heuristics (rule of thumb) Blind search (truly random search) Additional activities DecisionMaking:TheChoicePhase Sensitivity analysis What-if analysis Phase 3 Goal seeking Add a footer TREY research 42 Decision Making: The Implementation Phase “Nothing more difficult to carry out, nor more doubtful of success, nor more dangerous to handle, than to initiate a new order of things.” - The Prince, Machiavelli 1500s Solution to a problem Change Change management ?.. Implementation: putting a recommended solution to work Add a footer TREY research 43 How Decisions are Supported TREY research DSS Capabilities Early and New Definitions DSS early definition: it is a system intended to support managerial decisions in semistructured and unstructured decision situations DSS were meant to support decision makers extend their capabilities They are computer based and would operate interactively online, and preferably would have graphical output capabilities Nowadays, simplified via Web browsers and mobile devices Add a footer TREY research 45 DSS Capabilities Add a footer TREY research 46 Components of DSS Four DSS Components 1. Data Management Subsystem Includes the database that contains the data Database management system (DBMS) Can be connected to a data warehouse 2. Model Management Subsystem Model base management system (MBMS) 3. User Interface Subsystem 4. Knowledgebase Management Subsystem Organizational knowledge base Add a footer TREY research 47 DSS Components: Data Management Subsystem DSS database DBMS Data directory Query facility Add a footer TREY research 48 DSS Components: Model Management Subsystem Model base MBMS Modeling language Model directory Model execution, integration, and command processor Add a footer TREY research 49 DSS Components: User Interface Subsystem Interface Application interface DSS User Interface Portal Graphical icons Dashboard Color coding Interfacing with PDAs, cell phones, etc. Add a footer TREY research 50 An Early Decision Support Framework (by Gory and Scott-Morten, 1971) Add a footer TREY research 51 An Early Decision Support Framework Degree of Structuredness (Simon, 1977) Decisions are classified as Highly structured (a.k.a. programmed) Semi-structured Highly unstructured (i.e., nonprogrammed) Types of Control (Anthony, 1965) Strategic planning (top-level, long-range) Management control (tactical planning) Operational control Slide 1-52 52 Definition of BI [Narrow Definition] Descriptive analytics tools and techniques (i.e., reporting tools) [Broad Definition] An umbrella term that combines architectures, tools, databases, analytical tools, applications, and methodologies BI is a content-free expression, so it means different things to different people BI's major objective is to enable easy access to data (and models) to provide business managers with the ability to conduct analysis BI helps transform data, to information (and knowledge), to decisions, and finally to action TREY research BI versus AI Does Business Intelligence Need Artificial Intelligence? BI and AI are distinct but complementary. The “intelligence” in AI refers to computer intelligence, while in BI it refers to the more intelligent business decision-making that data analysis and visualization can yield. Add a footer TREY research 54 Data Analytics vs BI Data Analytics is focused on discovering patterns, trends, and insights in large, complex data sets, while Business Intelligence is typically focused on using data to monitor business performance and make informed decisions Add a footer TREY research 55 A Brief History of BI The Evolution of Business Intelligence The term BI was coined by the Gartner Group in the mid-1990s However, the concept is much older 1970s - MIS reporting - static/periodic reports 1980s - Executive Information Systems (EIS) 1990s - OLAP, dynamic, multidimensional, ad-hoc reporting -> coining of the term “BI” 2010s - Inclusion of AI and Data/Text Mining capabilities; Web- based Portals/Dashboards, Big Data, Social Media, Analytics 2020s - yet to be seen Add a footer TREY research 56 The Architecture of BI BI Four Major Components a data warehouse, with its source data business analytics, a collection of tools for manipulating, mining, and analyzing the data in the data warehouse business performance management (BPM) for monitoring and analyzing performance a user interface (e.g., dashboard) Add a footer TREY research 57 A High-Level Architecture of BI Data Warehouse Business Analytics Performance and Environment Environment Strategy Data Technical staff Business users Managers / executives Sources Built the data warehouse Access Data ü Organizing Warehouse BPM strategy ü Summarizing Manipulation ü Standardizing Results User Interface Future component - browser intelligent systems - portal - dashboard TREY research BusinessValueofBIAnalyticalApplications Examples Include Customer segmentation Propensity to buy Customer profitability Fraud detection Customer attrition Channel optimization Add a footer TREY research 59 AMultimediaExerciseinBusinessIntelligence TUN (TeradataUniversityNetwork.com) BSI Videos (Business Scenario Investigations) Analogues to CSI (Crime Scene Investigation) Go To www.youtube.com/watch?v=NXEL5F4_aKA See the www.slideshare.net/teradata/bsi-how-we-did-it-the-case-of-the- misconnecting-passengers.slides Discuss the case presented in the video and in the slides Slide 1-60 TREY research 60 Analytics Overview A Simple Taxonomy of Analytics (proposed by INFORMS) Descriptive Analytics Predictive Analytics Prescriptive Analytics Add a footer TREY research 61 Transaction Processing versus Analytic Processing Operational databases Online ERP, SCM, CRM, … Transaction Processing (OLTP) Goal: data capture Data warehouses Online Analytical Goal: decision support Processing (OLAP) Slide 1-62 62 Analytics Overview Analytics…a relatively new term/buzz-word Analytics…the process of developing actionable decisions or recommendations for actions based on insights generated from historical data According to the Institute for Operations Research and Management Science (INFORMS) Analytics represents the combination of computer technology, management science techniques, and statistics to solve real problems. Slide 1-63 TREY research 63 Business Analytics Slide 1-64 Descriptive Analytics Descriptive or reporting analytics Answering the question of what happened Retrospective analysis of historic data Enablers OLAP / DW Data visualization Dashboards and Scorecards Descriptive statistics Slide 1-65 TREY research 65 Predictive Analytics Aims to determine what is likely to happen in the future (foreseeing the future events) Looking at the past data to predict the future Enablers Data mining Text mining / Web mining Forecasting (i.e., time series) Slide 1-66 TREY research 66 Aims to determine the best possible decision Uses both descriptive and predictive to create the alternatives, and then determines the best one Enablers Optimization Simulation Multi-Criteria Decision Modeling Heuristic Programming PrescriptiveAnalytics Analytics Applied to Many Domains Analytics or Data Science? Slide 1-67 TREY research 67 Analytics Examples in Selected Domains Analytics in Retail Value Chain FIGURE 1.12 Example of Analytics Applications in a Retail Value Chain Slide 1-68 TREY research 68 Analytics Examples in Retail Value Chain For the complete table, refer to your textbook Slide 1-69 TREY research 69 A Brief Introduction to Big Data Analytics What Is Big Data? (Is it just “big”?) Big Data is data that cannot be stored or processed easily using traditional tools/means Big Data typically refers to data that comes in many different forms: large, structured, unstructured, continuous 3Vs – Volume, Variety, Velocity Data (Big Data or otherwise) is worthless if it does not provide business value (and for it to provide business value, it has to be analyzed) More on Big Data Analytics is in Chapter 7 Slide 1-70 TREY research 70 MARKETING AND ADVERTISING IN E-COMMERCE 71 TREY research ONLINE CONSUMER BEHAVIOR Influential factors Consumer Characteristics Merchant and Intermediary-Related Factors Product/Service Factors Motivational Factors (payment, SEO, shopping cart) Hygiene Factors (product quality, packaging, product warranty) Environmental Factors Social Variables Cultural/Community Variables Other Environmental Variables TREY research PERSONALIZATION Personalization in E-Commerce Personalization The matching of services, products, and advertising content with individual consumers and their preferences User profile The requirements, preferences, behaviors, and demographic traits of a particular customer Cookie A data file that is placed on a user’s hard drive by a remote Web server, frequently without disclosure or the user’s consent, which collects information about the user’s activities at a site TREY research Behavioral Marketing Behavioral targeting Targeting that uses information collected about an individual’s Web-browsing behavior, such as the pages they have visited or the searches they have made, to select an advertisement to display to that individual Collaborative filtering A market research and personalization method that uses customer data to predict, based on formulas derived from behavioral sciences, what other products or services a customer may enjoy; predictions can be extended to other customers with similar profiles TREY research 8-74 The Advertising Cycle TREY research Web Advertising BASIC INTERNET ADVERTISING TERMINOLOGY ad views The number of times users call up a page that has a banner on it during a specific period; known as impressions or page views button A small banner that is linked to a website; may contain downloadable software TREY research 8-76 Web Advertising click (ad click): A count made each time a visitor clicks on an advertising banner to access the advertiser’s website CPM (cost per mille, i.e., thousand impressions): The fee an advertiser pays for each 1,000 times a page with a banner ad is shown conversion rate: The percentage of clickers who actually make a purchase click-through rate/ratio (CTR): The percentage of visitors who are exposed to a banner ad and click on it Hit: A request for data from a Web page or file TREY research 8-77 WEB ADVERTISING Why Internet Advertising? Advertising Online and Its Advantages Cost Media richness Easy updating Personalization Location-based Linking to shopping Traditional Versus Online Advertising TREY 1- research 78 ONLINE ADVERTISING BANNERS Banners* Random/keyword banners* Pop-up/Pop-under banners* Benefits and Limitations of Banner Ads Benefit of banner ads is that, by clicking on a banner, users are transferred to an advertiser’s site, frequently directly to the shopping page of that site Disadvantage of banners is their cost TREY 1- research 79 E-MAIL ADS The major advantages of e-mail advertising are: It is a low-cost and effective method Advertisers can reach a large number of consenting subscribers Most Internet users check or send e-mail on a daily basis E-mail is an interactive medium that can combine advertising and customer service E-mail ads can include a direct link to any website, so they act like banners A consumer may be more likely to respond to relevant e-mail messages Limitations of e-mail advertising are: A major limitation of e-mail ads is that these messages are often treated as spam and are blocked by the user’s spam control software TREY 1- research 80 Search Engine Optimization Search Engine Optimization (SEO)* The craft of increasing site rank on search engines; the optimizer uses the ranking algorithm of the search engine (which may be different for different search engines) and best search phrases, and tailors the ad accordingly TREY 1- research 81 Google Online ADVERTISING King Google’s Major Advertising Methods: AdWords and AdSense Google AdWords - an advertising program for sponsored ads AdWords enables businesses & marketers to advertise on Google’s network (search, display, etc). Google AdSense - an affiliate program AdSense enables publishers to reserve space for AdWords placements on their website (text, video, images). Both AdWords and AdSense work together to complete Google’s greater advertising network: website owners put up space for Google’s ads (AdSense) and businesses set budgets and ads to display on Google’s advertising network (AdWords). TREY 1- research 82 MOBILE MARKETING AND ADVERTISING Mobile Marketing and Advertising Campaigns Building brand awareness Changing brand image Promoting sales Enhancing brand loyalty Building customer databases Stimulating mobile word of mouth TREY 1- research 83 ADVERTISING STRATEGIES AND PROMOTIONS Permission Advertising Advertising Strategies Affiliate Marketing and Advertising Ads as a Commodity: Paying People to Watch Ads Personalized Ads Advertising as a Revenue Model Pay per click (PPC)* Live Web Events for Advertising TREY 1- research 84 ADVERTISING STRATEGIES AND PROMOTIONS Localization in Advertising Advertising on Facebook TREY 1- research 85 Cyber Security and Fraud Issues and Protections TREY research INFORMATION SECURITY PROBLEM What Is Cyber Security? ○ Computer security refers to the protection of data, networks, computer programs, computer power, and other elements of computerized information systems TREY 1- research 87 CYBER SECURITY TERMINOLOGY Business continuity plan: A plan that keeps the business running after a disaster occurs. Each function in the business should have a valid recovery capability plan. Cybercrime: Intentional crimes carried out on the Internet. Fraud: Any business activity that uses deceitful practices/devices to deprive another of property or other rights. Malware (malicious software): A generic term for malicious software. Exposure Spam Vulnerability TREY 1- research 88 CYBER SECURITY ISSUES AND LANDSCAPE The Threats, Attacks, and Attackers Unintentional Threats Human Error Environmental Hazards Malfunctions in the Computer System Intentional Attacks and Crimes Hacker* White Hat Hacker Grey Hat Hacker Black Hat Hacker Cracker* TREY 1- research 89 authentication Process to verify (assure) the real identity of an individual, computer, computer program, or EC Web site. authorization Process of determining what the authenticated entity is allowed to access and what operations it is allowed to perform. Auditing Availability nonrepudiation Assurance that online customers or trading partners cannot falsely deny (repudiate) their purchase or transaction. CIA security triad (Confidentiality, Integrity, and Availability) Confidentiality* Integrity* Availability* TREY research deterring measures Actions that will make criminals abandon their idea of attacking a specific system (e.g., the possibility of losing a job for insiders). prevention measures Ways to help stop unauthorized users (also known as “intruders”) from accessing any part of the EC system. detection measures Ways to determine whether intruders attempted to break into the EC system, whether they were successful, and what they may have done. TREY research Software and systems knowledge are used to perpetrate (commit) technical attacks (computer virus) Nontechnical attacks are those in which a perpetrator uses some form of deception or persuasion to trick people into revealing information or performing actions that can compromise the security of a network TREY research How a Computer Virus Can Spread A piece of software code that inserts itself into a host, including the operating systems, in order to propagate (spread); it requires that its host program be run to activate it. TREY research From Phishing to Financial Fraud and Crime Fraud that involves stealing an identity of a person and then the use of that identity by someone pretending to be someone else in order to steal money or get other benefits. TREY research Figure 10.6 How Phishing Is Accomplished TREY research DEFENDING INFORMATION SYSTEMS AND E-COMMERCE Access Control* Authorization Authentication Biometric Systems Biometric authentication* Biometric systems* Thumbprint or fingerprint Retinal scan TREY 1- research 96 ENCRYPTION encryption The process of scrambling (encrypting) a message in such a way that it is difficult, expensive, or time- consuming for an unauthorized person to unscramble (decrypt) it. plaintext An unencrypted message in human-readable form. ciphertext A plaintext message after it has been encrypted into a machine-readable form. key (key value) The secret code used to encrypt and decrypt a message. Symmetric (Private) Key Encryption* uses the same key to encrypt and decrypt TREY 1- research 97 Master of Business Administration Graduate School of Business Management Information Systems Major Marywood University – Pennsylvania Program September 2024 Business Intelligence Prof. Dr. Rasha Abdelaziz Youssef Head of Business Information Systems dept. (AASTMT) Principal Investigator [Internationally Funded Projects by the British Council] Rasha Abdelaziz Youssef – AI in Business Organization TREY research 1 Back to Decision Making Styles Personality Tests Add a footer TREY research 2 Decision Style Personality temperament tests are often used to determine decision styles There are many such tests Myers/Briggs, True Colors (Birkman), Keirsey Temperament Theory, … Various tests measure somewhat different aspects of personality They cannot be equated! TREY research Exploring Your Colors Below are 11 incomplete sentences that describe people. Each sentence has four possible endings. Give four points to the phrase that is “most like you,” three points to the phrase that is “next most like you,” two points to the next phrase, and one point to the phrase that is “least like you.” Use the sentences below to describe your personality. 1. When I make decisions: a. I do it quickly and go with the first impressions. b. I think about it, consider the options and then decide. c. I listen to my feelings and consider how my decisions will affect others. d. I take it seriously and always try to make the right decision. 2. The best way for others to show me they care about me is to: a. Do fun things with me. b. Give me space to be myself. c. Spend time with me doing whatever. d. Do what I want to do; not let me down or go back on their word. 3. When I’m with my friends, I like to provide: a. The excitement; the fun; the jokes. b. Questions; answers; a logical way of looking at things. c. Concern for others; a lot of caring. d. The planning; a sense of security; a good standard. Add a footer TREY research 4 4. I like to: a. Act on a moment’s notice; do risky things. b. Provide answers or give thought to people’s questions. c. Help maintain a sense of harmony and togetherness. d. Be responsible, dependable, and helpful to others. 5. One thing I am really good at is: a. Acting courageously. b. Thinking. c. Being sensitive. d. Organizing. 6. Friends who know me best would say that I am: a. Competitive. b. Reserved, thoughtful. c. Emotional, friendly. d. Neat, prepared. 7. My basic approach to life is: a. To take one day at a time and have fun. b. To figure out what life is all about. c. To help others and be happy and succeed. d. To plan for the future and make it as good as possible. Add a footer TREY research 5 8. When I am feeling discouraged or “down in the dumps”: a. I often become rude, mad, or sometimes even mean. b. I withdraw, don’t talk very much, and try to think my way out of the problem. c. I feel emotional, am sad, and usually like to talk it over with someone close to me. d. I try to figure out what’s causing the problem and fix it. 9. I feel good about myself when: a. I can do things that are difficult. b. I can solve problems or figure things out. c. I can help other people. d. I am appreciated or rewarded for things I do. 10. Teachers at school who saw me when I wasn’t on my best behavior might describe me as: a. Rowdy or a little wild. b. Arrogant. c. Talkative. d. Someone who wants things my way; dominant; worrying. 11. Teachers at school (who like me and in whose class I do pretty well) would probably describe me as: a. Charming, a natural leader, clever, someone who is fun to have around. b. Thoughtful, someone who has good answers, someone who likes to figure out problems. c. Nice, friendly, someone who gets along with other students and is helpful to the teacher and others. d. Neat, organized, prepared, someone who does assignments and is a good student. Rank each number as 4, 3, 2, 1; where 4 is the one most like you and 1 is the least like you. Add a footer TREY research 6 CalculateYour Answers Number A B C D Total your columns and place your results in the blanks below. 1 Orange 2 Green 3 Blue 4 5 Gold 6 7 What is your first color? 8 What is your second color? 9 10 11 TOTAL Add a footer TREY research 7 Plan of the Book FIGURE 1.15 Plan of the Book Slide 1-8 TREY research 8 Changing Business Environments and Evolving Needs for Decision Support and Analytics ◼Increased hardware, software, and network capabilities ◼Group communication and collaboration ◼Improved data management ◼Managing giant data warehouses and Big Data ◼Analytical support ◼Overcoming cognitive limits in processing and storing information ◼Knowledge management ◼Anywhere, anytime support Slide 1-9 Evolution of Computerized Decision Support to Analytics/Data Science ◼FIGURE 1.8 Evolution of Decision Support, Business Intelligence, and Analytics Slide 1-10 A Framework for Business Intelligence ◼DSS → EIS → BI ◼Definition of Business Intelligence ◼[Broad Definition] An umbrella term that combines architectures, tools, databases, analytical tools, applications, and methodologies ◼[Narrow Definition] Descriptive analytics tools and techniques (i.e., reporting tools) ◼A Brief History of BI – 1970s → 1980s → 1990s … ◼The Origins and Drivers of BI (See Figure 1.9) ◼The Architecture of BI (See Figure 1.10) Slide 1-11 A Framework for Business Intelligence ◼FIGURE 1.9 Evolution of Business Intelligence (BI) → Slide 1-12 A Framework for Business Intelligence ◼ The Architecture of BI ◼ FIGURE 1.10 A High-Level Architecture of BI Slide 1-13 Transaction Processing versus Analytic Processing ◼Online Transaction Processing (OLTP) ◼Operational databases ◼ERP, SCM, CRM, … ◼Goal: data capture ◼Online Analytical Processing (OLAP) ◼Data warehouses ◼Goal: decision support Slide 1-14 Appropriate Planning and Alignment with the Business Strategy ◼Planning and Execution → Business, Organization, Functionality, and Infrastructure ◼Functions served by BI Competency Center ◼How BI is linked to strategy and execution of strategy ◼Encourage interaction between the potential business user communities and the IS organization ◼Serve as a repository and disseminator of best BI practices between and among the different lines of business. ◼Standards of excellence in BI practices can be advocated and encouraged throughout the company ◼… Slide 1-15 Real-Time, On-Demand BI Is Attainable ◼Emergence of real-time BI applications ◼Leveraging the enablers ◼RFID ◼Web services ◼Intelligent agents Slide 1-16 Critical BI System Considerations ◼Developing or Acquiring BI Systems ◼Make versus buy ◼Justification and Cost–Benefit Analysis ◼Security and Protection of Privacy ◼Integration to Other Systems and Applications Slide 1-17 Analytics Overview ◼Analytics…a relatively new term/buzz-word ◼Analytics…the process of developing actionable decisions or recommendations for actions based on insights generated from historical data ◼According to the Institute for Operations Research and Management Science (INFORMS) ◼Analytics represents the combination of computer technology, management science techniques, and statistics to solve real problems. Slide 1-18 Business Analytics ◼FIGURE 1.11 Three Types of Analytics Slide 1-19 Descriptive Analytics ◼Descriptive or reporting analytics ◼Answering the question of what happened ◼Retrospective analysis of historic data ◼Enablers ◼OLAP / DW ◼Data visualization ◼ Dashboards and Scorecards ◼Descriptive statistics Slide 1-20 Predictive Analytics ◼Aims to determine what is likely to happen in the future (foreseeing the future events) ◼Looking at the past data to predict the future ◼Enablers ◼Data mining ◼Text mining / Web mining ◼Forecasting (i.e., time series) Slide 1-21 Prescriptive Analytics ◼Aims to determine the best possible decision ◼Uses both descriptive and predictive to create the alternatives, and then determines the best one ◼Enablers ◼Optimization ◼Simulation ◼Multi-Criteria Decision Modeling ◼Heuristic Programming ◼Analytics Applied to Many Domains Slide 1-22 Analytics Examples in Selected Domains ◼Analytics Application in HealthCare ◼Example 1: Preventing Falls in a Senior Population—An Analytic Approach ◼Example 2 : Predictive Models to Identify the Highest Risk Membership in a Health Insurer Slide 1-23 Analytics Examples in Selected Domains ◼Analytics in Retail Value Chain ◼Example of Analytics Applications in a Retail Value Chain Slide 1-24 Analytics Examples in Retail Value Chain ◼For the complete table, refer to your textbook Slide 1-25 An Overview of the Analytics Ecosystem ◼Data Generation Infrastructure Providers ◼Data Management Infrastructure Providers ◼Data Warehouse Providers ◼Middleware Providers ◼Data Service Providers ◼Analytics Focused Software Developers ◼ Descriptive, Predictive, Prescriptive ◼Application Developers: Industry Specific or General ◼Analytics Industry Analysts and Influencers Slide 1-26 The Nature of Data ◼ Data: a collection of facts ◼ usually obtained as the result of experiences, observations, or experiments ◼ Data may consist of numbers, words, images, … ◼ Data is the lowest level of abstraction (from which information and knowledge are derived) ◼ Data is the source for information and knowledge ◼ Data quality and data integrity → critical to analytics Slide 2-27 The Nature of Data Slide 2-28 Metrics for Analytics Ready Data ◼ Data source reliability ◼ Data content accuracy ◼ Data accessibility ◼ Data security and data privacy ◼ Data richness ◼ Data consistency ◼ Data currency/data timeliness ◼ Data granularity ◼ Data validity and data relevancy Slide 2-29 A Simple Taxonomy of Data ◼ Data (datum—singular form of data): facts ◼ Structured data ◼ Targeted for computers to process ◼ Numeric versus nominal ◼ Unstructured/textual data ◼ Targeted for humans to process/digest ◼ Semi-structured data? ◼ XML, HTML, Log files, etc. ◼ HTML is the primary building block of web development and is used to display content of web pages. XML or JSON can transport data exchange between servers and are often used alongside HTML or other applications. ◼ A log file is a computer-generated data file that contains information about usage patterns, activities and operations within an operating system, application, server or another device. Log files show whether resources are performing properly and optimally. ◼ HTML is semi structured. It contains tags and elements with definitive properties and hierarchies. However, the order and number of those tags varies from document to document. ◼ Slide 2-30 A Simple Taxonomy of Data Slide 2-31 The Art and Science of Data Preprocessing ◼ The real-world data is dirty, misaligned, overly complex, and inaccurate ◼ Not ready for analytics! ◼ Readying the data for analytics is needed ◼ Data preprocessing ◼ Data consolidation ◼ Data cleaning ◼ Data transformation ◼ Data reduction ◼ Art – it develops and improves with experience Slide 2-32 The Art and Science of Data Preprocessing ◼ Data reduction 1. Variables ◼ Dimensional reduction ◼ Variable selection 2. Cases/samples ◼ Sampling ◼ Balancing / stratification Slide 2-33 Data Preprocessing Tasks and Methods Slide 2-34 Improving Student Retention with Data-Driven Analytics ◼ Student retention ◼ Freshmen class ◼ Why it is important? ◼ What are the common techniques to deal with student attrition? ◼ Analytics versus theoretical approaches to student retention problem Slide 2-35 Statistical Modeling for Business Analytics Slide 2-36 Statistical Modeling for Business Analytics ◼Statistics ◼ A collection of mathematical techniques to characterize and interpret data ◼Descriptive Statistics ◼ Describing the data (as it is) ◼Inferential statistics ◼ Drawing inferences about the population based on sample data ◼Descriptive statistics for descriptive analytics Slide 2-37 Descriptive Statistics Measures of Centrality Tendency ◼ Arithmetic mean ◼ Median ◼ The number in the middle ◼ Mode ◼ The most frequent observation Slide 2-38 Descriptive Statistics Measures of Dispersion ◼ Dispersion ◼ Degree of variation in a given variable ◼ Range ◼ Max - Min ◼ Variance Standard Deviation Slide 2-39 Descriptive Statistics Measures of Dispersion ◼ Quartiles ◼ Box-and-Whiskers Plot ◼ a.k.a. box-plot ◼ Versatile / informative Slide 2-40 Descriptive Statistics Shape of a Distribution ◼ Histogram – frequency chart ◼ Skewness ◼ Measure of asymmetry ◼ Kurtosis ◼ Peak/tall/skinny nature of the distribution Slide 2-41 Technology Insights – Descriptive Statistics in Excel Slide 2-42 Technology Insights – Descriptive Statistics in Excel - Creating box-plot in Microsoft Excel Slide 2-43 Regression Modeling for Inferential Statistics ◼ Regression ◼ A part of inferential statistics ◼ The most widely known and used analytics technique in statistics ◼ Used to characterize relationship between explanatory (input) and response (output) variable ◼ It can be used for ◼ Hypothesis testing (explanation) ◼ Forecasting (prediction) ◼ Correlation versus Regression Slide 2-44 Regression Modeling Slide 2-45 Regression Modeling ◼ x: input, y: output ◼ Simple Linear Regression ◼ Multiple Linear Regression ◼ The meaning of Beta () coefficients ◼ Sign (+ or -) and magnitude Slide 2-46 Process of Developing a Regression Model How do we know if the model is good enough? 2 ◼ R (R-Square) ◼ p Values ◼ Error measures (for prediction problems) Slide 2-47 Business Reporting Definitions and Concepts ◼ Report = Information → Decision ◼ Report? ◼ Any communication artifact prepared to convey specific information ◼ A report can fulfill many functions ◼ To ensure proper departmental functioning ◼ To provide information ◼ To provide the results of an analysis ◼ To persuade others to act ◼ To create an organizational memory… Slide # of total 49 What is a Business Report? ◼ A written document that contains information regarding business matters. ◼ Purpose: to improve managerial decisions ◼ Source: data from inside and outside the organization (via the use of ETL) ◼ Format: text + tables + graphs/charts ◼ Distribution: in-print, email, portal/intranet Data acquisition → Information generation → Decision making → Process management Slide # of total 50 Business Reporting Business Functions UOB 1.0 X UOB 2.1 X UOB 3.0 Data UOB 2.2 Transactional Records Exception Event Symbol Count Description Action Machine 1 Failure (decision) DEPLOYMENT CHART PHASE 1 PHASE 2 PHASE 3 PHASE 4 PHASE 5 DEPT 1 DEPT 2 DEPT 3 Data DEPT 4 4 5 2 3 1 Repositories Decision Information Maker (reporting) Slide # of total 51 Data Visualization “The use of visual representations to explore, make sense of, and communicate data.” ◼ Data visualization vs. Information visualization ◼ Information = aggregation, summarization, and contextualization of data ◼ Related to information graphics, scientific visualization, and statistical graphics ◼ Often includes charts, graphs, illustrations, … Slide # of total Which Chart or Graph Should You Use? 52 Slide # of total 53 An Example Gapminder Chart Wealth and Health of Nations See gapminder.org for Interesting animated examples Slide # of total 54 The Emergence of Data Visualization and Visual Analytics ◼ Magic Quadrant for Business Intelligence and Analytics Platforms (Source: Gartner.com) ◼ Many data visualization companies are in the 4th quadrant ◼ There is a move towards visualization Slide # of total 55 The Emergence of Data Visualization and Visual Analytics ◼ Emergence of new companies ◼ Tableau, Spotfire, QlikView, … ◼ Increased focus by the big players ◼ MicroStrategy improved Visual Insight ◼ SAP launched Visual Intelligence ◼ SAS launched Visual Analytics ◼ Microsoft bolstered PowerPivot with Power View ◼ IBM launched Cognos Insight ◼ Oracle acquired Endeca Slide # of total Performance Dashboards Slide # of total Performance Dashboards ◼ Dashboard design ◼ The fundamental challenge of dashboard design is to display all the required information on a single screen, clearly and without distraction, in a manner that can be assimilated quickly ◼ Three layer of information ◼ Monitoring ◼ Analysis ◼ Management Slide # of total Business Intelligence, Analytics, and Data Science: A Managerial Perspective Fourth Edition Chapter 3 Descriptive Analytics II: Business Intelligence and Data Warehousing Copyright © 2018 Pearson Education Ltd. Business Intelligence and Data Warehousing ◼ BI used to be Business Analytics everything related to use of data for managerial Descriptive Predictive Prescriptive decision support Now, it is a part of Questions ◼ What happened? What will happen? What should I do? What is happening? Why will it happen? Why should I do it? Business Analytics BI = Descriptive ü Business reporting ü Data mining ü Optimization Enablers ◼ ü Dashboards ü Text mining ü Simulation Analytics ü ü Scorecards Data warehousing ü ü Web/media mining Forecasting ü ü Decision modeling Expert systems Outcomes Well defined Accurate projections Best possible business problems of future events and business decisions and opportunities outcomes and actions Business Intelligence Advanced Analytics Slide 3-59 What is a Data Warehouse? ◼ A physical repository where relational data are specially organized to provide enterprise-wide, cleansed data in a standardized format ◼ “The data warehouse is a collection of integrated, subject-oriented databases designed to support DSS functions, where each unit of data is non-volatile and relevant to some moment in time” Slide # of total A Historical Perspective to Data Warehousing ü Mainframe computers ü Centralized data storage ü Big Data analytics ü Simple data entry ü Data warehousing was born ü Social media analytics ü Routine reporting ü Inmon, Building the Data Warehouse ü Text and Web Analytics ü Primitive database structures ü Kimball, The Data Warehouse Toolkit ü Hadoop, MapReduce, NoSQL ü Teradata incorporated ü EDW architecture design ü In-memory, in-database 1970s 1980s 1990s 2000s 2010s ü Mini/personal computers (PCs) ü Exponentially growing data Web data ü Business applications for PCs ü Consolidation of DW/BI industry ü Distributer DBMS ü Data warehouse appliances emerged ü Relational DBMS ü Business intelligence popularized ü Teradata ships commercial DBs ü Data mining and predictive modeling ü Business Data Warehouse coined ü Open source software ü SaaS, PaaS, Cloud Computing Slide # of total Characteristics of DWs ◼ Subject oriented ◼ Integrated ◼ Time-variant (time series) ◼ Nonvolatile ◼ Summarized ◼ Not normalized ◼ Metadata ◼ Web based, relational/multi-dimensional ◼ Client/server, real-time/right- time/active... Slide # of total Data Mart A departmental small-scale “DW” that stores only limited/relevant data ◼ Dependent data mart A subset that is created directly from a data warehouse ◼ Independent data mart A small data warehouse designed for a strategic business unit or a department Slide # of total Other DW Components ◼ Enterprise data warehouse (EDW) ◼ A data warehouse for the enterprise ◼ Metadata – “data about data” ◼ In DW metadata describe the contents of a data warehouse and its acquisition and use Slide # of total A Generic DW Framework Data Applications Sources No data marts option (Visualization) Data Marts Routine ERP Business ETL Reporting Process Data mart Select (Marketing) / Middleware Legacy Metadata Data/text Extract mining Data mart Transform Enterprise (Operations) POS Data warehouse OLAP, Integrate API Data mart Dashboard, (Finance) Web Other Load OLTP/Web Replication Data mart (...) Custom built External applications Data Slide 2-65 DW Architecture ◼ Three-tier architecture 1. Data acquisition software (back-end) 2. The data warehouse that contains the data & software 3. Client (front-end) software that allows users to access and analyze data from the warehouse ◼ Two-tier architecture ◼ First two tiers in three-tier architecture are combined into one … sometimes there is only one tier? Slide # of total DW Architectures Tier 1: Tier 2: Tier 3: Client workstation Application server Database server Tier 1: Tier 2: Client workstation Application & database server Slide # of total A Web-based DW Architecture