AMAT 131 SG 11 Introduction to Experimental Design PDF
Document Details
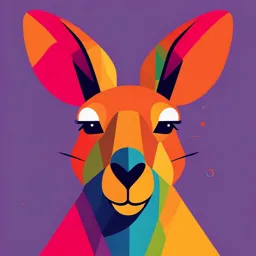
Uploaded by HaleUvite
University of the Philippines Mindanao
2021
Tags
Summary
This document provides an introduction to experimental designs. It covers observational and experimental studies, sources of variation in experimental studies, characteristics of experiments, and constructing models to represent reality. Key concepts like treatment factors, experimental units, response variables, and applications of experimental design are also explored. The document appears to be a study guide for a course on statistical methods and experimental designs at the University of the Philippines Mindanao for the 2nd semester of 2020-2021.
Full Transcript
Introduction to Experimental Designs AMAT 131: Statistical Methods and Experimental Designs Study Guide 11 2nd Semester, AY 2020-2021 Department of Mathematics, Physics, and Computer Sc...
Introduction to Experimental Designs AMAT 131: Statistical Methods and Experimental Designs Study Guide 11 2nd Semester, AY 2020-2021 Department of Mathematics, Physics, and Computer Science University of the Philippines Mindanao 1 Types Of Scientific Study Observational Study - Observes individuals and measures variables of interest but does not attempt to influence the responses - Purpose: describe some group or situation - The application and manipulation of conditions to see effect on outcomes are not possible or not ethical Example: sample survey, observing behavior of animals in the wild, observing interaction between teacher and students Experimental Study - Actively imposes treatment to observe response - Purpose: to study whether the treatment causes a change in the response - To understand cause and effect, experiments are the only source of fully convincing data Example: Experimental study answer the following types of questions: ▪ Does aspirin reduce the pain of a heart attack? ▪ Does life come from nonliving organisms? ▪ Which chemicals, of several under study, show insecticidal activity? ▪ How is yield related to plant spacing, and does this relationship vary between varieties? 2 Sources Of Variation In Experimental Study Source of variation is anything that could cause an observation to have a different numerical value from another observation. Treatment Factor: this is the factor of interest in an experiment; any substance or item whose effect on the data is to be studied; level: specific types or amount of the treatment factor that will actually be used in the experiment; treatment factor and its levels should be selected; quantitative levels of a treatment factor are usually equally spaced; an experiment can have more than one treatment factor Example: Treatment factor: drug; Levels: different amounts (in grams) of drugs to be studied Experimental Units: the material to which the levels of the treatment factor are applied; should be representative of the material of the material and conditions to which the conclusions of the experiment will be applied Example: If medicine is the treatment factor, humans or animals may be the experimental units; the conclusions of an experiment that uses university students as experiments units may not apply to all adults in the country Response Variable: the variable whose variation depends on other variables; it is the subject of change within an experiment, often as a result of differences in the treatment factor; the variable of interest in an experiment Example: In a study about the low-carb Atkins diet is comparison to other diets: the treatment factor is the diet; the experiment units are the people participating in the diets; the response variable is the amount of weight loss and the level of cholesterol. 3 Characteristics Of Experiments Experiments allow us to set up a direct comparison between treatments of interest. We can design experiments to minimize any bias in the comparison. We can design experiments so that the error in the comparison is small. We are in control of experiments, and having that control allows us to make stronger inferences about the nature of differences that we see in the experiment. We may make inferences about causation. 4 Constructing Models To Represent Reality Model = representation of the real world Mathematical Model: uses mathematical objects as representation Statistical Model: considers uncertainty and allows for variability in the process that may arise from: - Sampling variation - Biological variation between individuals - Inaccuracies in measurement - Influential variables being omitted (knowingly or not) from the model General from of Statistical Model: 𝑅𝑒𝑠𝑝𝑜𝑛𝑠𝑒 = 𝑆𝑦𝑠𝑡𝑒𝑚𝑎𝑡𝑖𝑐 𝐶𝑜𝑚𝑝𝑜𝑛𝑒𝑛𝑡 + 𝑅𝑎𝑛𝑑𝑜𝑚 𝐶𝑜𝑚𝑝𝑜𝑛𝑒𝑛𝑡 5 Applications Of Experimental Design Experimental design is a critically important tool in the scientific and engineering world for improving the product realization process. Critical components of these activities are in new manufacturing process design and development, and process management. The application of experimental design techniques early in process development can result in Improved process yields Reduced variability and closer conformance to nominal or target requirements Reduced development time Reduced overall costs. Experimental design methods are also of fundamental importance in engineering design activities, where new products are developed, and existing ones improved. Some applications of experimental design in engineering design include Evaluation and comparison of basic design configurations Evaluation and comparison of basic design configurations Selection of design parameters so that the product will work well under a wide variety of field conditions, that is, so that the product is robust Determination of key product design parameters that impact product performance Formulation of new products. 6 Guidelines For Designing Experiments 1. Recognition of and statement of the problem: list the essential questions or problems that are to be addressed by the experiment; outline the conclusions expected from the analysis of data 2. Selection of the response variable: in selecting the response variable, the experimenter should be certain that this variable really provides useful information about the process under study 3. Choice of factors, levels, and range: determine the potential design factors 4. Choice of experimental design: involves consideration of sample size, selection of suitable run order for the experimental trials, and determination of whether or not blocking or other randomization restrictions are involved 5. Performing the experiment: when running the experiment, it is vital to monitor the process carefully to ensure that everything is being done according to plan; errors in experiments procedure at this stage will usually destroy experimental validity 6. Statistical analysis of the data: statistical methods should be used to analyze the data so that results and conclusions are objective rather than judgmental in nature; if the experiment has been designed correctly and performed according to the design, the statistical methods required are not elaborate 7. Conclusions and recommendations: once the data have been analyzed, the experimenter must draw practical conclusions about the results and recommend a course of action; graphical methods are often useful in this stage, particularly in presenting the results to others; follow-up runs and confirmation testing should also be performed to validate the conclusions from the experiment 7 Common Experimental Designs Completely Randomized Design (CRD) - Simplest form of design - When to use: homogeneous experimental units (no need for blocking) - Each treatment is equally likely to be allocated to each unit - Advantages: easy to set up; simple form of analysis Example: Calcium Pot Trial An experiment was devised to investigate the effect of differences in soil calcium on the root growth of plants. The experimental material consisted of 20 pots, each containing one plant, arranged in a grid with four rows and five columns, under uniform controlled conditions. The treatments comprised four relative concentrations of calcium (A = 1, B = 5, C = 10, D = 20). Each treatment was applied to five pots selected at random to give a CRD. Factor of Interest: Soil calcium concentrations Response Variable: Root growth of plants Blocking: None Randomized Complete Block Design (RCBD) - Simplest design that includes blocking - The number of experimental units per block is the same as the number of treatments - Treatments are randomly assigned to experimental units within a block (all treatments occur within a block) - Weakness: block size must be equal to number of treatments Example: Potato Yields An experiment was devised to investigate the effects of four different types of fungicides (labelled F1, F2, F3, F4) on the yield of potatoes in field plots. An untreated control treatment (labelled Control) was also included to give a baseline comparison. In the field designated for the trial, heterogeneity was thought to be present at large scales, but suitable blocks of five field plots could be identified and so an RCBD with four blocks each of five plots could be used. Factor of Interest: Types of fungicides Response Variable: Yield of potatoes Blocking: Blocks of field plots Latin Square Design (LSD) - Includes two blocking factors - Number of treatments must be equal to the number of columns and rows - Each treatment appears once in each row and in each column - Disadvantage: treatments = columns = rows Example: Lupin Trial An experiment was devised to investigate the effects of soil type on the growth of lupins. The experiment was to be done with pots on a bench in a glasshouse, with a systematic trend running along the bench (left-right) as a result of a temperature gradient, and across the bench (up-down) because of differing light levels. The rows and columns within the array of pots were therefore considered as blocking factors, and an LS design is appropriate. Four treatments, labelled CL, CH, SL and SH, representing different combinations of soil type (clay or sand) and the amount of water supplied (low or high) were used. Each treatment can be found once in each row and once in each column, and each row or column contains all four treatments. Factor of Interest: Soil type and amount of water supplied Response Variable: Growth of lupins Blocking: Temperature gradient, light Levels Balanced Incomplete Block Design (BIBD) - One blocking factor but the number of units per block is smaller than the number of treatments - Each block can contain only a subset of the treatments - Treatments have equal replication - Each pair of treatments occurs together within a block exactly the same number of times Example: Grain Protein Content An experiment was devised to evaluate the grain protein content for six different varieties of pea (labelled A, B, C, D, E, and F). Five independent samples of grain were available for each variety. Only five samples could be assessed within a session, with possible heterogeneity between sessions, so a BIBD with six blocks (corresponding to sessions), each containing five units (corresponding to samples) was used. For this design each variety is replicated five times, as each appears in five of the six sessions, and any pair of varieties is present together in four sessions, for example, varieties E and F are both present in sessions 1, 2, 3, and 6. Factor of Interest: Varieties of pea Response Variable: Grain protein content Blocking: Session Split-Plot Design - At least two factors are present - Treatment/levels of one factor (whole plot) having to be applied to large experimental units - Levels of the other factor (subplot) can be applied to smaller units - Block > replicate Example: Weed Competition Experiment A field trial was set up to study the competitive effects of three different weed species in winter wheat under different levels of water stress. Variation in water stress was provided by the presence or absence of irrigation, which could be applied only to large areas of land whereas the weed species could be applied to small plots. An SP design was therefore deemed suitable, with the two irrigation treatments applied to whole plots. Each whole plot was split into four subplots, and a pre-determined population of each weed species (black-grass, cleavers, and chickweed) was sown in one of these four subplots. The remaining subplot within each whole plot had no weed seeds added, and it was used as a control. This structure was repeated another three times, giving four blocks, with a randomization in each block. Factor of Interest: Weed species in winter wheat, water stress Response Variable: Competitive Effects of Weed Species in Winter Wheat Blocking: None 8 References Design and Analysis of Experiments, 8th Ed by Montgomery - Strategy of Experimentation (Ch 1.1, p 1) - Some Typical Applications of Experimental Design (Ch 1.2, p 8) - Guidelines for Designing Experiments (Ch 1.4, p 14)