Instrumental Variables: Lecture on LATE Theorem PDF
Document Details
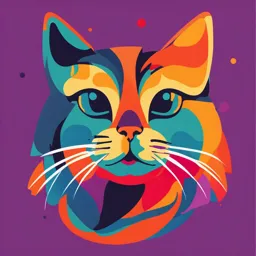
Uploaded by LongLastingGalaxy
Magnus Carlsson
Tags
Summary
These lecture slides introduce instrumental variables (IV) and the Local Average Treatment Effect (LATE) theorem. The material covers the assumptions needed for LATE, and relates this work within the context of economics and econometrics, using examples from the Vietnam draft lottery to illustrate the concepts. The lecture discusses both sides of the usefulness of LATE with pros and cons.
Full Transcript
Instrumental variabels 2 Magnus Carlsson IV with heterogeneous potential outcomes (A & P, 4.4) So far, we have assumed a constant causal effect. Realistic? We will now relax this assumption and focus on the case with a zero-one causal variable, like a binary treatment We will allow for tre...
Instrumental variabels 2 Magnus Carlsson IV with heterogeneous potential outcomes (A & P, 4.4) So far, we have assumed a constant causal effect. Realistic? We will now relax this assumption and focus on the case with a zero-one causal variable, like a binary treatment We will allow for treatment effect heterogeneity, or a distribution of causal effects across individuals Treatment effect heterogeneity relates strongly to the discussion about internal versus external validity IV with heterogeneous potential outcomes (A & P, 4.4) With heterogeneous treatment effects, IV will fail to capture either ATE or ATET. So what is the intuition? Take the draft lottery instrument discussed above Clearly, not everybody was affected by the draft lottery. The ones who were affected, however, are called compliers As we will see, under reasonable assumption, IV will capture the effect of treatment only on the group of compliers This effect is called a local average treatment effect (LATE) A generalized potential outcomes concept To understand the LATE concept, we will next generalize the potential outcomes concept. Let 𝑦𝑖 (d, z) denote the potential outcome of individual 𝑖 when this person has treatment status 𝐷𝑖 = 𝑑 and instrument value 𝑍𝑖 = 𝑧 Now let 𝐷1𝑖 denote 𝑖’s potential treatment status when 𝑍𝑖 = 1, while 𝐷0𝑖 denotes 𝑖’s potential treatment status when 𝑍𝑖 = 0. A generalized potential outcomes concept: example ln the draft lottery example, treatment status 𝐷𝑖 is whether a person was “treated” by going to Vietnam. Call this “veteran status” The instrument 𝑍𝑖 denotes whether or not the person was assigned by the lottery to go to Vietnam 𝐷1𝑖 is then potential veteran status when assigned by the lottery to go 𝐷0𝑖 is then potential veteran status when assigned by the lottery not to go A generalized potential outcomes concept We can then write observed treatment status as: 𝐷𝑖 = 𝐷0𝑖 + (𝐷1𝑖 − 𝐷0𝑖 )𝑍𝑖 (4) 𝐷𝑖 , i.e. treatment status, now takes on the role of outcome of the process of itself being “treated” with the instrument. Only one of the potential treatment assignments is ever observed for a given person A generalized potential outcomes concept We can now index potential outcomes against both treatment status 𝑑 and the instrument 𝑧. ln our example, there are now four potential outcomes for earnings: 𝑦𝑖 1,1 if 𝐷𝑖 = 1, 𝑍 =1 𝑦𝑖 1,0 if 𝐷𝑖 = 1, 𝑍 =0 Potential earnings = (5) 𝑦𝑖 0,1 if 𝐷𝑖 = 0, 𝑍 =1 𝑦𝑖 0,0 if 𝐷𝑖 = 0, 𝑍 =0 The assumptions needed for LATE From the table above, it is clear that treatment status and the instrument does not always go hand in hand For instance, some people would still go to Vietnam despite 𝑍 = 0 and the opposite is true as well This means that we have treatment heterogeneity, i.e. not all people react the same way to the instrument We will next examine the assumptions needed in order to still get some meaningful IV-estimate in the heterogeneous treatment effect case Assumption 1. The independence assumption The independence assumption: the instrument 𝑍𝑖 is independent of potential outcomes and potential treatment assignments Example: take two persons, one randomly assigned to go to Vietnam, the other not. The person assigned to serve have the same chance of actually serving as the person not assigned to serve would have if he was assigned to serve. And vice versa. The independence assumption is basically saying that the instrument is as good as randomly assigned (at least conditional on covariates) Assumption 2. The exclusion restriction The exclusion restriction: the instrument operates through a single know causal channel. Formally: 𝑦𝑖 𝑑, 0 = 𝑦𝑖 𝑑, 1 for 𝑑 = 0, 1 (6) This says that given treatment status, there is no way in which the instrument affects potential outcomes 𝑦𝑖. ln our example: the instrument only affects earnings through treatment status (going to war or not) Could this fail even with random assignment? Assumption 2. The exclusion restriction Yes! Even if the instrument is randomly assigned, the exclusion restriction could still be violated The exclusion restriction fails for draft-lottery instruments if men with low draft lottery numbers were affected in some way other than through an increased likelihood of service. For example, Angrist and Krueger (1992) looked for an association between draft lottery numbers and schooling. The exclusion restriction cont. With the exclusion restriction, we can now index potential outcomes solely against treatment status since: 𝑌1𝑖 = 𝑌𝑖 1,1 = 𝑌𝑖 (1, 0) (7) 𝑌0𝑖 = 𝑌𝑖 0,1 = 𝑌𝑖 (0, 0) (8) We can then write the observed outcome 𝑦𝑖 in terms of potential outcomes: 𝑌𝑖 = 𝑌𝑖 0, 𝑍𝑖 + [𝑌𝑖 1, 𝑍𝑖 − 𝑌𝑖 0, 𝑍𝑖 ]𝐷𝑖 (9) = 𝑌0𝑖 + (𝑌1𝑖 − 𝑌0𝑖 )𝐷𝑖 (10) Assumption 3: the monotonicity assumption While the instrument may have no effect on some people, the ones who are affected must be affected in the same way: 𝐷1𝑖 > 𝐷0𝑖 ∀𝑖 or vice versa (11) ln the draft example, this means that although draft eligibility may not affect the probability of doing service for some men, the ones who were affected all had an increased probability of doing service Without monotonicity, we cannot be sure we are getting a weighted average of the individual causal effects. We’ll return to this. Assumption 4: the existence of a first-stage There exists a first-stage such that: 𝐸[𝐷1𝑖 − 𝐷0𝑖 ] ≠ 0 (12) In other words, there s a significant first-stage effect of the instrument on the treatment LATE Given the: independence assumption the exclusion restriction the monotonicity assumption the existence of a first-stage, the lV-estimate can be interpreted as the effect of treatment status (veteran status) on those whose treatment status was changed by the instrument (compliers) This parameter is called the local average treatment effect (LATE) Compliers and other subpopulations The LATE framework portions the population under study into four subgroups, defined by the manner by which they react to the instruments: Compliers: The subpopulation with 𝐷1𝑖 = 1 and 𝐷0𝑖 = 0 Always-takers: The subpopulation with 𝐷1𝑖 = 1 and 𝐷0𝑖 = 1 Never-takers: The subpopulation with 𝐷1𝑖 = 0 and 𝐷0𝑖 = 0 Defiers: The subpopulation with 𝐷1𝑖 = 0 and 𝐷0𝑖 = 1 Defiers are ruled out by the monotonicity assumption The LATE Theorem Under assumptions 1-4, the Wald estimator (the simplest case) can be written as: 𝐸[𝑌𝑖 𝑍𝑖 =1 −𝐸 𝑌𝑖 |𝑍𝑖 =0 = 𝐸[𝑌1𝑖 − 𝑌0𝑖 𝐷1𝑖 > 𝐷0𝑖 (13) 𝐸[𝐷𝑖 𝑍𝑖 =1 −𝐸 𝐷𝑖 |𝑍𝑖 =0 This is the average treatment effect for the group defined by 𝐷1𝑖 > 𝐷0𝑖. What is that group? Since 𝐷𝑖 is zero or one: 𝐷1𝑖 > 𝐷0𝑖 ⇔ {𝐷1𝑖 = 1, 𝐷0𝑖 = 0} This means that 𝐷1𝑖 > 𝐷0𝑖 is the group for whom the instrument changes the treatment status Proof of the LATE theorem (simplified proof compared to book) To give a proof, start with the first bit of the Wald estimator: 𝐸[𝑌𝑖 |𝑍𝑖 = 1] This can be written as weighted average of the effects among compliers, never-takers, always-takers, and defiers: 𝐸[𝑌𝑖 |𝑍𝑖 = 1] = 𝐸[𝑌𝑖 𝑍𝑖 = 1, complier ∙ Pr (complier|𝑍𝑖 = 1) +𝐸[𝑌𝑖 𝑍𝑖 = 1, never taker ∙ Pr (never taker|𝑍𝑖 = 1) +𝐸[𝑌𝑖 𝑍𝑖 = 1, always taker ∙ Pr (always taker|𝑍𝑖 = 1) +𝐸[𝑌𝑖 𝑍𝑖 = 1, defier ∙ Pr (defier|𝑍𝑖 = 1) Proof of the LATE theorem We rule out defiers and re-write: 𝐸[𝑌𝑖 |𝑍𝑖 = 1] = 𝐸[𝑌1𝑖 𝐶 ∙ 𝜋𝑐 + 𝐸[𝑌0𝑖 |𝑁] ∙ 𝜋𝑛 + 𝐸[𝑌1𝑖 |𝐴] ∙ 𝜋𝑎 , where 𝜋𝑐 , 𝜋𝑛 , and 𝜋𝑎 are the fraction of compliers, never-takers, and always-takers, respectively and where 𝐶, 𝑁, and 𝐴 denotes compliers, never-takers, and always-takers respectively. Proof of the LATE theorem Consider now the second term: 𝐸[𝑌𝑖 |𝑍𝑖 = 0] In a similar vain, this can be written as: 𝐸[𝑌𝑖 |𝑍𝑖 = 0] = 𝐸[𝑌0𝑖 𝐶 ∙ 𝜋𝑐 + 𝐸[𝑌0𝑖 |𝑁] ∙ 𝜋𝑛 + 𝐸[𝑌1𝑖 |𝐴] ∙ 𝜋𝑎 , Proof of the LATE theorem The difference: 𝐸[𝑌𝑖 |𝑍𝑖 = 1] - 𝐸[𝑌𝑖 |𝑍𝑖 = 0] can be written as: 𝐸[𝑌1𝑖 𝐶 ∙ 𝜋𝑐 + 𝐸[𝑌0𝑖 𝑁 ∙ 𝜋𝑛 + 𝐸[𝑌1𝑖 𝐴 ∙ 𝜋𝑎 −𝐸[𝑌0𝑖 |𝐶] ∙ 𝜋𝑐 + 𝐸[𝑌0𝑖 |𝑁] ∙ 𝜋𝑛 + 𝐸[𝑌1𝑖 |𝐴] ∙ 𝜋𝑎 = 𝐸[𝑌1𝑖 𝐶 ∙ 𝜋𝑐 − 𝐸[𝑌0𝑖 |𝐶] ∙ 𝜋𝑐 = 𝐸[𝑌1𝑖 −𝑌0𝑖 𝐶 ∙ 𝜋𝑐 Proof of the LATE theorem Next, turn to the denominator, where we can use similar arguments: 𝐸[𝐷𝑖 𝑍𝑖 = 1 = 𝐸[𝐷1𝑖 𝐶 ∙ 𝜋𝑐 + 𝐸[𝐷0𝑖 𝑁 ∙ 𝜋𝑛 + 𝐸[𝐷1𝑖 𝐴 ∙ 𝜋𝑎 , and 𝐸[𝐷𝑖 𝑍𝑖 = 0 = 𝐸[𝐷0𝑖 𝐶 ∙ 𝜋𝑐 + 𝐸[𝐷0𝑖 𝑁 ∙ 𝜋𝑛 + 𝐸[𝐷1𝑖 𝐴 ∙ 𝜋𝑎 , The difference is 𝐸[𝐷𝑖 𝑍𝑖 = 1 − 𝐸[𝐷𝑖 𝑍𝑖 = 0 = 𝐸[𝐷1𝑖 𝐶 ∙ 𝜋𝑐 − 𝐸[𝐷0𝑖 𝐶 ∙ 𝜋𝑐 = 𝐸[𝐷1𝑖 − 𝐷0𝑖 𝐶 ∙ 𝜋𝑐 = 𝜋𝑐 Proof of the LATE theorem We can now write: 𝐸[𝑌𝑖 𝑍𝑖 =1 −𝐸[𝑌𝑖 𝑍𝑖 =0 (14) 𝐸[𝐷𝑖 𝑍𝑖 =1 −𝐸[𝐷𝑖 𝑍𝑖 =0 𝐸[𝑌1𝑖 −𝑌0𝑖 𝐶 ∙𝜋𝑐 = = 𝐸[𝑌1𝑖 − 𝑌0𝑖 𝐶 , (15) 𝜋𝑐 or: 𝐸[𝑌1𝑖 − 𝑌0𝑖 𝐷1𝑖 > 𝐷0𝑖 , This is the treatment effect among compliers What does the theorem say? The theorem says that: - an instrument that is as good as randomly assigned, - affects the outcome through a single known channel, - has a first stage, - affects the causal channel of interest only in one direction can be used to estimate the average causal effect for the group affected by the instrument (LATE), i.e. the compliers What’s the use of this the theorem? Example: How to save an experiment with imperfect compliance” As we learned in the lectures on experiments, the “perfect” experience can be hard to obtain People randomly assigned to treatment may refuse to take the treatment (resisters), and some randomly assigned to not take the treatment may take it anyway This typically happens when countries use a lottery to assign people to military service Those assigned to serve may resist doing so and those not assigned might volunteer Example: IV in the context of “imperfect” experiments ln the LATE context, the volunteers can be said to be “always-taker”, since they take the treatment irrespective of the lottery outcome The ones who refuse military service can be characterized as “never- takers” Those who obey the lottery outcome are the “compliers”. We can probably rule out “defiers”. Example: IV in the context of “imperfect” experiments We can still exploit the lottery outcome as an instrument for actually doing military service Estimates using the draft lottery instrument capture the effect of military service on men who served because they were draft eligible but would otherwise not have served (compliers). This excludes the effect on volunteers, who would have gone no matter what the lottery said Why do we need the monotonicity assumption? A failure of monotonicity means the instrument pushes some people into treatment while pushing others out. Without monotonicity, the ”reduced” form part of the LATE theorem can be written as: E 𝑌𝑖 |𝑍𝑖 = 1 − E 𝑌𝑖 |𝑍𝑖 = 0 = E 𝑌1𝑖 − 𝑌0𝑖 |𝐶 ∙ 𝜋𝑐 + E 𝑌1𝑖 − 𝑌0𝑖 |𝐷𝐸 ∙ 𝜋𝑑𝑒 where 𝐷𝐸 denotes defiers (see proof of LATE). If monotonicity fails, the sign of the reduced from effect for defiers may be the opposite to compliers. Here, even if the treatment effect is positive for everyone, the reduced form may be zero if the effect of compliers is cancelled out by the effect of defiers Notes about LATE Note that different instruments may very well produce different LATEs depending on the complier group This makes it difficult to conduct a straightforward comparison of the results obtained by using different instruments, since the results may simply reflect that there is heterogeneity in the treatment effect across different groups of compliers. Even comparing results using the same instrument in different settings may be difficult since the number of compliers may differ Thus, instrumental variables estimates may often have low external validity but high internal validity How useful is LATE? Big debate about the usefulness of LATE! Angrist, Imbens, Rubin, and others: The LATE cup is half full: Although we don’t get the average effect on a stable population (like the average treatment effect on the treated), we get something that still makes some sense By getting different LATEs in different situations, we can build an evidence base on a topic How useful is LATE? Heckman, Deaton, and others: The LATE cup is half empty: We don’t get what we want, the average treatment effect on the treated, so IV is somewhat useless We need to augment it with something else to produce economically meaningful parameters (e.g. more structural econometric models). How useful is LATE? Since differences in compliant sub-populations might explain variability in treatment effects from one instrument to another, we would therefore like to learn as much as we can about the compliers for different instruments. Moreover, if the compliant subpopulation is similar to other populations of interest, the case for extrapolating estimated causal effects to these other populations is stronger. Acemoglu and Angrist (2000) argue, for instance, that quarter-of-birth instruments and state compulsory attendance laws affect essentially the same group of people and for the same reasons. We therefore expect IV estimates of the returns to schooling from these two sets of instruments to be similar. How useful is LATE? If the compliant subpopulations associated with two or more instruments are very different, yet the IV estimates they generate are similar, we might be prepared to adopt homogeneous effects as a working hypothesis. This reasoning is illustrated by the study of the effects of family size on children’s education by Angrist, Lavy, and Schlosser (2006). As it turns out, IV estimates of the effect of family size using a number of different instruments, each with very different compliant subpopulations, all generate results showing no effect of family size. Angrist, Lavy, and Schlosser (2006) argue that their results point to a common treatment of zero for just about everybody in the Israeli population they study. Summary of IV Instruments may provide a solution to problems of omitted variables, measurement errors in X, and simultaneity bias ln each of the three cases the bias arises because 𝐸[𝑢|𝑋] ≠ 0 and we say that we have an endogeneity problem ln the constant effects case, we need (1) a strong first-stage and (2) a believable exclusion restriction We can use 2SLS to estimate the effects Summary of IV ln the heterogenous effects case, we need additional assumptions We need the (1) independence assumption, (2) exclusion restriction, (3) monotonicity assumption and (4) the existence of a first-stage Under these assumptions, our IV estimate will give us a Local Average Treatment Effect This parameter gives us the causal effect for those who were actually affected by the instrument, i.e. the group of compliers Appendix: IV as a solution to measurement errors To see how IV may help solve measurement error problems, let’s assume classical measurement errors, such that: 𝑋 = 𝑋෨ + 𝜈, Where 𝑋෨ is the true variable, 𝑋 is the measured, and 𝜈 is a measurement error term that is independent of the true value 𝑋,෨ i.e. a random or “classical” measurement error. Consider the true model: 𝑦 = 𝛼 + 𝛽𝑋෨ + 𝑢, which conforms to the standard OLS assumptions. The estimated model uses 𝑋 as a proxy for 𝑋෨ : 𝑦 = 𝛼 + 𝛽(𝑋 − 𝜈) + 𝑢, IV and the problem of measurement errors This can be written in the “omitted variables form”: 𝑦 = 𝛼 + 𝛽𝑋 − 𝛽𝜈 + 𝑢 (16) 𝑦 = 𝛼 + 𝛽𝑋 + 𝜔, where 𝜔 = 𝑢 − 𝛽𝜈. Problem is now that 𝐸[𝜔|𝑋] = 0, just like in the omitted variables problem, since 𝐶𝑜𝑣 𝑋, 𝜔 = 𝐶𝑜𝑣 𝑋෨ + 𝑣, 𝑢 − 𝛽𝑣 = −𝛽𝜎 2, 𝑣 (17) assuming independence between 𝑣 and 𝑢. IV and the problem of measurement errors We can next derive the bias. Our OLS estimator of the equation above is: 𝐶𝑜𝑣 𝑦, 𝑋 መ 𝛽𝑂𝐿𝑆 = 𝑉𝑎𝑟 𝑋 𝐶𝑜𝑣 𝛼 + 𝛽𝑋෨ + 𝑢, 𝑋෨ + 𝑣 = 𝑉𝑎𝑟 𝑋෨ + 𝑣 𝑉𝑎𝑟 𝑋෨ ෨ 𝑣 + 𝐶𝑜𝑣 𝑢, 𝑋෨ + 𝑣 𝐶𝑜𝑣 𝛽𝑋, = 𝛽+ 𝑉𝑎𝑟 𝑋෨ + 𝑣 𝑉𝑎𝑟 𝑋෨ + 𝑣 𝑉𝑎𝑟 𝑋෨ = 𝛽 ෨ 𝑉𝑎𝑟 𝑋 + 𝑉𝑎𝑟 𝑣 IV and the problem of measurement errors cont. መ 𝑉𝑎𝑟 𝑋෨ So, 𝛽 converges in probability to a fraction < 1 of the 𝑉𝑎𝑟 𝑋෨ +𝑉𝑎𝑟 𝑣 true 𝛽. This bias is called attenuation bias since 𝛽መ will always be biased towards zero with classical measurement errors. If we could find an instrument variable that is correlated with 𝑋, but that is uncorrelated with 𝜔, that would help us to deal with the 𝐸[𝜔|𝑋] ≠ 0 problem. IV and the problem of measurement errors Note: the results above is for models with one explanatory variable, where the measurement error is classical If a variable is measured with error, and this variable is correlated with other explanatory variables in the model, the result cannot be generalized. The same goes if the measurement error is non-classical. BUT: the key role of IV is to solve endogeneity problems due to omitted variables!