Instrumental Variables (IV) - Lecture - PDF
Document Details
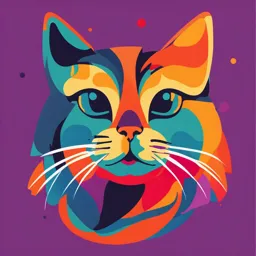
Uploaded by LongLastingGalaxy
Magnus Carlsson
Tags
Related
- Applied Econometrics Endogeneity & Instrumental Variables Lecture 3 PDF
- Causal Analysis Instrumental Variables Part 1 PDF
- Causal Analysis Instrumental Variables PDF
- Instrumental Variables - Part 2 PDF
- Econometrics Lecture 9 - Instrumental Variables (25117) PDF
- Instrumental Variables: Lecture on LATE Theorem PDF
Summary
This lecture, authored by Magnus Carlsson, provides an introduction to Instrumental Variables (IV) in econometrics. It explores how IV methods can address endogeneity and omitted variable bias by using instruments to isolate variation in causal variables. The lecture covers the concept of IV, 2SLS, the Wald estimator and the assumptions needed for their validity.
Full Transcript
Instrumental variabels 1 Magnus Carlsson Introduction to Instrumental Variables (IV) ln the OLS lectures, we have seen that the zero conditional mean assumption is unlikely to be fulfilled in many cases What do we then do when 𝐶𝑜𝑣 𝑥𝑖 , 𝑢𝑖 ≠ 0? 1. Conduct a real experiment. Not possible in...
Instrumental variabels 1 Magnus Carlsson Introduction to Instrumental Variables (IV) ln the OLS lectures, we have seen that the zero conditional mean assumption is unlikely to be fulfilled in many cases What do we then do when 𝐶𝑜𝑣 𝑥𝑖 , 𝑢𝑖 ≠ 0? 1. Conduct a real experiment. Not possible in many cases 2. Look for quasi-experimental methods Instrumental variables is a quasi-experimental method that in some circumstances can be used to mimic an experiment, even when there is no experiment Roadmap of IV Under what circumstances is an instrument useful? IV under the assumption of constant effects Assumptions needed for IV The Wald estimator Two-stage least squares (TSLS) IV tests IV under the assumption of heterogeneous effects Literature Angrist and Pischke, chapter 4 Wooldridge, chapter 15.1-15.5 Articles See Reading list Where an instrument can help The IV-method may provide a solution to the following threats to internal validity: Omitted variables Measurement errors in X (see appendix of IV lectures slides part 2) Simultaneity bias ln each of these three cases, the bias arises because 𝐸 𝑢ȁ𝑋 ≠ 0 Endogeneity Endogeneity is often used as a characterization of these three threats to internal validity that cause 𝐸 𝑢ȁ𝑋 ≠ 0. If a variable X is endogenous, OLS does not measure a causal effect, but only a correlation. Exogeneity refers to the case where the variable X is uncorrelated with u. IV: two cases We will study under IV under two cases; (1) where the effect is assumed to be the same for everybody (constant effect case; this case is repetition from previous courses). (2) where the effect is allowed to differ across different people (heterogeneous effect case) IV: The constant effect case We start with the constant effects case and again consider the example of a causal link between schooling and wages, where potential outcomes can be written: 𝑦𝑠𝑖 = 𝑓𝑖 (𝑠) Here, 𝑦𝑠𝑖 denotes potential outcomes under various levels of schooling (like years of schooling). Moreover, we have that: 𝑓𝑖 𝑠 = 𝜋0 + 𝜋1 𝑠 + 𝜂𝑖 where 𝜂𝑖 , denotes unobserved factors that affect wages. IV: The constant effect case Assume now that 𝜂𝑖 can be written as a function of a vector of variables 𝐴𝑖 , called ability, such that: 𝜂𝑖 = 𝐴´𝑖 𝛾 + 𝜐𝑖 where 𝛾 denotes a vector of population regression coefficients (the coefficients show the “effect” of abilities) and 𝜐𝑖 is a random term Since 𝛾 are “true” population coefficients, 𝜐𝑖 and 𝐴𝑖 are uncorrelated by construction. IV: The constant effect case Our model so far can thus be written: 𝑦𝑖 = 𝛼 + 𝑝𝑠𝑖 + 𝜂𝑖 (1) We now assume that we have an omitted variables problem because the 𝐴𝑖 variables, contained in 𝜂𝑖 , are correlated with 𝑠𝑖. Note, however, that the 𝐴𝑖 variables are the only reason why 𝑠𝑖 and 𝜂𝑖 are correlated so that: 𝐸[𝑠𝑖 𝜐𝑖 ] = 0 (2) IV: The constant effect case Let’s now instead assume that 𝐴𝑖 was observed so that we could include it in the “long” regression: 𝑦𝑖 = 𝛼 + 𝑝𝑠𝑖 + 𝐴´𝑖 𝛾 + 𝜐𝑖 ln this equation, 𝜐𝑖 is the random part of potential outcomes that is left over after controlling for 𝐴𝑖. Since 𝜐𝑖 , is uncorrelated with 𝑠𝑖 by construction, the population regression of 𝑦𝑖 , on 𝑠𝑖 , and 𝐴𝑖 gives the causal coefficients in the equation. IV: The constant effect case But; how do we estimate the long-regression coefficient, 𝜌, when 𝐴𝑖 is unobserved (which it usually is)? Instrumental variables methods can do this when there exists a variable that is: 1. correlated with the causal variable of interest 𝑠𝑖 and 2. uncorrelated with any other determinants of the dependent variable 𝑦𝑖 We call such a variable an instrument and denote it 𝑧𝑖 IV: The constant effect case What does “uncorrelated with any other determinants of the dependent variable” mean? In our example, this phrase is the same as saying that 𝐶𝑜𝑣 (𝜂𝑖 , 𝑧𝑖 ) = 0 or that 𝑧𝑖 is uncorrelated with both 𝐴𝑖 and 𝜐𝑖 This means that 𝑧𝑖 is a variable that is uncorrelated with abilities 𝐴𝑖 and other unobserved determinants of wages BUT; 𝑧𝑖 should at the same time be correlated with schooling 𝑠𝑖. The intuition behind IV Assume now that we have such a variable 𝑧𝑖 and the model: 𝑦𝑠𝑖 = 𝜋0 + 𝜋1 𝑠 + 𝜂𝑖 (3) where 𝐸 𝜂𝑖 𝑠 ≠ 0. What is the intuition behind IV? IV breaks the variation in s into two parts: one part that is correlated with 𝜂𝑖 , and one part that is not correlated with 𝜂𝑖. The part of 𝑠 that is correlated with 𝜂𝑖 , is the “problematic” part The part of 𝑠 that is uncorrelated with 𝜂𝑖 is the “unproblematic” part The intuition behind IV With an instrument 𝑧𝑖 we isolate, or predict, variation in the part of 𝑠 that is uncorrelated with 𝜂𝑖 , allowing us to get a consistent estimate of 𝜋1. An instrument is a variable that helps us to distinguish and separate the two parts (the uncorrelated and the correlated part) in 𝑠. The instrument thus detects movements in s that are uncorrelated with 𝜂𝑖 and uses these to estimate 𝜋1. ln our example, 𝑧𝑖 would be a variable that let us only use the variation in schooling 𝑠 that is unrelated to abilities 𝐴𝑖 Example: Angrist and Kreuger’s quarter of birth instrument Angrist and Kreuger (1991) wanted to study the causal effect of education on earnings. Worried by omitted variables bias from unobserved ability, they used institutional knowledge about school-starting age policies and compulsory schooling laws in the US to generate an instrument for schooling. Their strategy starts with the observation that most states in the US require children to start school in the calendar year in which they turn 6 (and school usually starts in September). Example: Angrist and Kreuger’s (1991) quarter of birth instrument cont. This means that all children born in the same year start school in September that year However, compulsory schooling laws only requires students to stay in school until their 16th birthday. This means that students born in different quarters will be in different grades, or through a given grade to a different degree, when they reach the legal dropout age. This creates a “natural experiment” where individuals with different birthdays are required to stay in school for different amounts of time Example: Angrist and Kreuger (1991) quarter of birth instrument cont. Example: a person born in January can drop out of school 3 months earlier than a person born in April and 7 months earlier than a person born in August and so on. This may cause lesser schooling being obtained among those born earlier in the year. Evidence also suggests so, see graph: Average education and quarter of birth in Angrist and Kreuger´s study Average earnings and quarter of birth in Angrist and Kreuger´s study Angrist and Kreuger cont. Clearly, their instrument, 𝑧𝑖 , quarter of birth, seems to affect years of schooling. The quarter-of-birth instrument could be used to distinguish between “problematic” and “unproblematic” variation in schooling 𝑠𝑖 It seems plausible that the part of variation in schooling that is only caused by variation in quarter of birth should be unrelated to an individual’s ability If quarter of birth would also affect ability, or other unobserved determinants of earnings, it would be a bad instrument. The assumptions needed for a valid IV in the constant effects case Let us now formalize the assumptions needed for a valid instrument 𝑧𝑖 1. The instrument 𝑧𝑖 must have a clear effect on the causal variable of interest (schooling in our example). We say that there must be a strong first-stage. If the instrument has no effect, it will not provide any information about our causal variable of interest This assumption is easy to check in the data For example, in the example above, quarter of birth (the instrument) must have significant impact on years of schooling (the causal variable of interest). The assumptions needed for a valid IV in the constant effects case 2. The second assumption is that the only reason for a relationship between our outcome variable, 𝑦𝑖 , and the instrument, 𝑧𝑖 , is the first stage. This means that 𝑧𝑖 should only affect 𝑦𝑖 via its effect on 𝑠𝑖 ln the example above, it means that quarter of birth should only affect earnings through its effect on schooling. This is called an exclusion restriction and can be written 𝐶𝑜𝑣(𝑧𝑖 , 𝜂𝑖 ) = 0 Note; the exclusion restriction cannot be tested! It is an identifying assumption. Don’t believe any tests (more on this later)! The assumptions needed for a valid IV in the constant effects case Why is it called an exclusion restriction? The reason is that since the instrument 𝑧𝑖 is assumed to be uncorrelated with the unobserved determinants of the outcome variable 𝑦𝑖 , it can be excluded from the main outcome equation ln other words, excluding it will not cause omitted variables bias. ln the example above, quarter of birth can be excluded from the earnings equation, since it is not correlated with ability or any other observed variables other than schooling The exclusion restriction Note the similarity between the exclusion restriction of the instrument and randomization! A proper instrument, where the exclusion restriction holds, provides variation in our causal variable of interest (like schooling) that is as good as random The exclusion restriction thus says that the instrument is independent of potential outcomes but not of the causal variables of interest (schooling in our case). ln the example above, it is assumed that a person’s quarter of birth, conditional on some covariates, is as good as random. Angrist and Kreuger formally Let us now formalize how we obtain the IV-estimate. Take the quarter of birth story again, which can be written as: 𝑠𝑖 = 𝑋´𝑖 𝜋10 + 𝜋11 𝑧𝑖 + 𝜁1𝑖 (4) 𝑦𝑖 = 𝑋´𝑖 𝜋20 + 𝜋21 𝑧𝑖 + 𝜁2𝑖 (5) The first equation is called the first-stage equation and regresses schooling 𝑠𝑖 on the instrument 𝑧𝑖 and other controls 𝑋´𝑖. The second equation is called the reduced form equation and estimates the direct effect of the instrument on earnings Angrist and Kreuger formally If the exclusion restriction holds, it means that reduced form equation shows the direct effect of the instrument on earnings, that is assumed to run only through schooling The first-stage shows the effect of the instrument on schooling By combining these two effects we could construct the IV-estimate of schooling, which shows the causal effect of increasing schooling by one year on earnings IV: The constant effect case Given the exclusion restriction, the IV-estimate can be obtained as (which we will later prove!): 𝐶𝑜𝑣(𝑦𝑖 , 𝑧𝑖 ) 𝐶𝑜𝑣(𝑦𝑖 , 𝑧𝑖 )Τ𝑉(𝑧𝑖 ) 𝜌= = 𝐶𝑜𝑣(𝑠𝑖 , 𝑧𝑖 ) 𝐶𝑜𝑣(𝑠𝑖 , 𝑧𝑖 )Τ𝑉(𝑧𝑖 ) The second equality in the equation above is nice because it expresses 𝜌 in terms of the ratio of the regression coefficients of the first stage and reduced form equations. ln this expression, 𝜌 is the ratio of the population regression of 𝑦𝑖 on 𝑧𝑖 (the reduced form) to the population regression of 𝑠𝑖 on 𝑧𝑖 (the first stage) The Wald estimator The simplest IV estimator uses a single binary (0-1) instrument to estimate a model with one endogenous regressor and no covariates. Given the simplification that 𝑧𝑖 , is a dummy variable that equals 1 with probability p, it can be shown that: 𝐶𝑜𝑣(𝑦𝑖 , 𝑧𝑖 )={E [𝑦𝑖 𝑧𝑖 = 1 − E [𝑦𝑖 ȁ𝑧𝑖 = 0]} 𝑝(1 − 𝑝) (6)* with an analogous formula for 𝐶𝑜𝑣(𝑠𝑖 , 𝑧𝑖 ). ln this setup, the IV estimator has a simple form: E [𝑦𝑖 𝑧𝑖 =1 −E [𝑦𝑖 ȁ𝑧𝑖 =0] 𝑟𝑒𝑑𝑢𝑐𝑒𝑑 𝑓𝑜𝑟𝑚 𝜌= = (7) E [𝑠𝑖 𝑧𝑖 =1 −E [𝑠𝑖 ȁ𝑧𝑖=0] 𝑓𝑖𝑟𝑠𝑡−𝑠𝑡𝑎𝑔𝑒 * See separate document on MyMoodle The Wald estimator: example I We can see the Wald estimator in action in the Angrist and Krueger (1991) study using quarter of birth as instrument The reduced form: in the paper, the difference in log earnings between men born in the first and second halves of the year is -.01349 (s.e.=.00337) The first stage: the difference in years of schooling between men born in the first and second halves of the year is -.1514. The ratio of these two differences,.0891 (s.e.=.021), is a Wald estimate of the economic value of schooling in per-year terms. The Wald estimator: example II The Angrist (1990) study of the effects of Vietnam-era military service on the earnings of veterans ln the 1960s and early 1970s, young men were at risk of being drafted for military service, and a draft lottery was used to determine priority for conscription. A promising instrumental variable for Vietnam veteran status is therefore draft-eligibility, since this was determined by a lottery. In practice, many draft-eligible men were still exempted from service for health or other reasons, while many men who were draft-exempt nevertheless volunteered for service. The Wald estimator: example II Veteran status was thus not completely determined by randomized draft-eligibility, but draft-eligibility provides a binary instrument highly correlated with Vietnam-era veteran status. Where do you find good instruments? Often instruments come from detailed institutional knowledge in combination with knowledge how the causal variable of interest is generated The latter can be obtained from knowledge about the costs and benefits that determine various decisions. Schooling example: the decision to go to college is determined by the costs and benefits of doing so. Possible instruments are therefore policies that for instance shifts the costs of going to college, such as differences in rules for student loans or some subsidies. Under certain circumstances, changes in such policies could occur in a manner that is independent of potential outcomes. Terminology The dependent variables in the two equations are called endogenous variables (determined within the system) The variables on the right-hand side are called exogenous variables (determined outside the system) The instrument(s) 𝑧𝑖 is a subset of the exogenous variables The exogenous variables that are not instruments are called exogenous covariates Two-stage least squares (2SLS) In practice, it is more convenient to use 2SLS than IV when there are also other exogenous variables in the equation The 2SLS estimator is similar except that additional exogenous variables are used in the first and second stage 2SLS can also be used when the model is overidentified, i.e. when we have several instruments instead of just one With several instruments we can also conduct some (weak) tests of the validity of the exclusion restriction Two-stage least squares (2SLS) Consider first the causal model (“the structural model”) with covariates: 𝑦𝑖 = 𝑋´𝑖 𝛼 + 𝑝𝑠𝑖 + 𝜂𝑖 , where 𝜂𝑖 = 𝐴´𝑖 𝛾 + 𝜐𝑖. This is the equation of interest, but we fear that 𝐶𝑜𝑣 𝑠𝑖 , 𝜂𝑖 ≠ 0. Assume we have an instrument 𝑧𝑖 so that the first-stage equation can be written as: 𝑠𝑖 = 𝑋´𝑖 𝜋10 + 𝜋11 𝑧𝑖 + 𝜁1𝑖 (8) Two-stage least squares (2SLS) We can now substitute this first-stage equation into this causal model of interest: 𝑦𝑖 = 𝑋´𝑖 𝛼 + 𝜌[𝑋´𝑖 𝜋10 + 𝜋11 𝑧𝑖 + 𝜁1𝑖 ] + 𝜂𝑖 (9) = 𝑋´𝑖 𝛼 + 𝜌𝜋10 + 𝜌𝜋11 𝑧𝑖 + [𝜌𝜁1𝑖 + 𝜂𝑖 ] (10) = 𝑋´𝑖 𝜋20 + 𝜋21 𝑧𝑖 + 𝜁2𝑖 (11) where 𝜋20 = 𝛼 + 𝜌𝜋10 , 𝜋21 = 𝜌𝜋11 and 𝜁2𝑖 = 𝜌𝜁1𝑖 + 𝜂𝑖. Two-stage least squares (2SLS) We can also re-arrange (9) such that: 𝑦𝑖 = 𝑋´𝑖 𝛼 + 𝜌[𝑋´𝑖 𝜋10 + 𝜋11 𝑧𝑖 ] + 𝜁2𝑖 (12) where 𝑋´𝑖 𝜋10 + 𝜋11 𝑧𝑖 is the population fitted (predicted) value from the first-stage regression of 𝑠𝑖 on 𝑋𝑖 and 𝑧𝑖. Now note that this fitted value, denoted 𝑠ෝ𝑖 is uncorrelated with the composite error term 𝜁2𝑖. This is because the exclusion restriction assumes that Cov 𝜂𝑖 , 𝑧𝑖 = 0 and since Cov 𝜁1𝑖 , 𝑧𝑖 = 0 Two-stage least squares (2SLS) ln a sample, the first stage fitted values can be consistently estimated with: 𝑠ෝ𝑖 = [𝑋´𝑖 𝜋ො 10 𝑖 + 𝜋ො 11 𝑧𝑖 ] (13) We can now replace 𝑠𝑖 with 𝑠ෝ𝑖 in our causal effect of interest such that: 𝑦𝑖 = 𝑋´𝑖 𝛼 + 𝜌𝑠ෝ𝑖 + [𝜂𝑖 + 𝜌 𝑠𝑖 − 𝑠ෝ𝑖 ], (14) where 𝑠𝑖 − 𝑠ෝ𝑖 = 𝜁1𝑖. This is consistent estimator of 𝜌 since 𝑠ෝ𝑖 is uncorrelated with both 𝜂𝑖 and 𝑠𝑖 − 𝑠ෝ𝑖. Two-stage least squares (2SLS) We would thus estimate: 𝑦𝑖 = 𝑋´𝑖 𝛼 + 𝜌𝑠ෝ𝑖 + 𝑣𝑖 , (15) where 𝑣𝑖 = [𝜂𝑖 + 𝜌 𝑠𝑖 − 𝑠ෝ𝑖 ], i.e. the error term ln practice, we usually do not conduct estimation in two step, despite the name Computer programs like Stata and SAS conduct the estimation and gets the standard errors right Deriving the formula of the 2SLS estimator ln the case where we have a single instrument 𝑧𝑖 and a single endogenous right-hand side variable 𝑥𝑖 , there is a simple formula for the 2SLS estimator. Let 𝑠𝑧𝑦 be the sample covariance between 𝑧𝑖 and 𝑦 and let 𝑠𝑧𝑥 be the sample covariance between 𝑧𝑖 and 𝑥, The 2SLS estimator with a single instrument can then be written as: 2𝑆𝐿𝑆 𝑠𝑧𝑦 መ 𝛽1 = (16) 𝑠𝑧𝑥 We are now going to show this. The 2SLS formula Remember that in 2SLS, the endogenous variable 𝑥𝑖 is replaced by the predicted value 𝑥ො𝑖 from the first-stage regression on the instrument 𝑧𝑖. ln the second-stage, the dependent variable 𝑦𝑖 is regressed on the predicted value 𝑥ො𝑖 by 𝑂𝐿𝑆 The formula for 2𝑆𝐿𝑆 can thus be re-written as the formula for 𝑂𝐿𝑆, where 𝑥𝑖 is replaced by 𝑥ො𝑖 : 𝛽መ 12𝑆𝐿𝑆 𝑠𝑥𝑦 ො /𝑠 2 𝑥ො , (17) 2 where 𝑠𝑥𝑦 ො is the sample covariance between 𝑥 ො𝑖 and 𝑦𝑖 and 𝑠 𝑥ො is the variance of 𝑥ො𝑖 The 2SLS formula cont. Let 𝑥ො𝑖 be the predicted value of 𝑥𝑖 from the first-stage regression, 𝑥ො𝑖 = 𝜋ො 0 + 𝜋ො 1 𝑧𝑖 The definitions of sample variances and covariance's imply that 2 2 𝑠𝑥𝑦 ො = 𝜋 ො 𝑠 1 𝑧𝑦 and 𝑠 𝑥ො = 𝜋 ො 𝑠 1 𝑧. 2 Why? Start by looking at the expressions for 𝑠𝑥𝑦 ො and 𝑠 𝑥ො. These expressions (remember the expressions for covariance and variance) both involve the mean of 𝑥ො𝑖 , which is: 𝑥ොҧ = 𝜋ො 0 + 𝜋ො 1 𝑧ҧ (18) The 2SLS formula cont. Now use the expression for the mean of 𝑥ොҧ and 𝑥ො𝑖 and plug those into the covariance and variance formulas: 𝑛 𝑠𝑥𝑦 ො = ( 𝑥 ොҧ 𝑖 − 𝑦) ො − 𝑥)(𝑦 ത (19) 𝑖=1 𝑛 = (( 𝜋ො 0 + 𝜋ො 1 𝑧𝑖 ) − (𝜋ො 0 + 𝜋ො 1 𝑧))(𝑦 ҧ 𝑖 − 𝑦) ത (20) 𝑖=1 𝑛 = 𝜋ො 1 ( 𝑧𝑖 − 𝑧)(𝑦 ҧ 𝑖 − 𝑦) ത = 𝜋ො 1 𝑠𝑧𝑦 𝑖=1 (21) Similarly : 𝑛 𝑛 ොҧ 2 = 𝜋ො 12 ( 𝑧𝑖 − 𝑧)ҧ 2 = 𝜋ො 12 𝑠𝑧2 𝑠𝑥2ො = ( 𝑥ො𝑖 − 𝑥) 𝑖=1 𝑖=1 (22) The 2SLS formula cont. Now use the fact that the OLS estimator of 𝜋ො 1 can be written as: 𝑠𝑧𝑥 𝜋ො 1 = (23) 𝑠2𝑧 We can then write the 2SLS estimator as: 2𝑆𝐿𝑆 𝑠𝑥ෝ𝑦 ෝ 1 𝑠𝑧𝑦 𝜋 𝑠𝑧𝑦 𝑠𝑧𝑦 𝑠𝑧𝑦 መ 𝛽1 = 2 = 22 = = 𝑠𝑧𝑥 = (24) 𝑠𝑥ෝ ෝ 1𝑠 𝑧 𝜋 ෝ 1 𝑠2𝑧 𝜋 𝑠 2 𝑧 2 𝑠𝑧𝑥 𝑠𝑧 Some examples of instruments Large literature interested in the effects of family size on (1) female labor supply and (2) child outcomes Common wisdom says that women with more children work less. But is it a causal relationship? More children also means less resources to each child (quality-quantity tradeoff). But does family size causally affect children’s outcomes? Since family size is an endogenous choice variable, a proper instrument is needed Some examples of instruments Two common instruments for family size are: The occurrence of a twin birth: A twin birth typically has a positive effect on completed family size. A twin birth is as good as random (conditional on a woman’s age) Same-sex gender composition: parents whose two first children are of the same sex are more likely to have a third child. Gender composition of two first children is as good as random For an application of both these instruments in the quantity-quality context, see Angrist et al. (2010) Repeating the two-stage least squares intuition Two-stage least squares decomposes the causal variable of interest into one problematic part and one unproblematic part. Only the unproblematic part of the variable is then used to estimate the causal effect ln the quarter-of-birth example, the schooling variable is decomposed into a part that only depends on quarter of birth Since quarter of birth is independent of ability, preferences, etc. this part is unproblematic and uncorrelated with the error term ln sum, 2SLS only retain the variation in 𝑠𝑖 that is generated by the instrument 𝑧𝑖.