Decision Analysis 2 - Bocconi University
Document Details
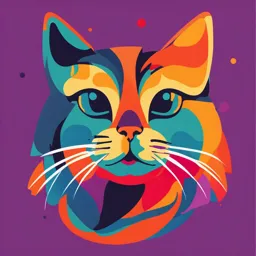
Uploaded by LuxuriantFreedom8108
Bocconi University
Tags
Summary
This document is a set of notes on decision analysis, including examples of how to apply decision analysis to business problems such as evaluating the success or failure of new products or predicting future customer preferences. It appears to be for an undergraduate class in business management.
Full Transcript
DECISION ANALYSIS DECISION ANALYSIS 2 AGENDA 1. WHY WE NEED A SCIENTIFIC APPROACH TO DECISION-MAKING 2. BASICS ON PROBABILITIES AND DISTRIBUTION 3. MEAN AND STANDARD DEVIATION 4. CONDITIONAL PROBABILITY AND JOINT PROBABILITY 5. PREDICTING OF EVE...
DECISION ANALYSIS DECISION ANALYSIS 2 AGENDA 1. WHY WE NEED A SCIENTIFIC APPROACH TO DECISION-MAKING 2. BASICS ON PROBABILITIES AND DISTRIBUTION 3. MEAN AND STANDARD DEVIATION 4. CONDITIONAL PROBABILITY AND JOINT PROBABILITY 5. PREDICTING OF EVENTS 6. MAKING DECISIONS 7. APPLICATION: SOUTHWEST AIRLINES 1.0 WHY WE NEED A SCIENTIFIC APPROACH TO DECISION-MAKING WHY WE NEED A SCIENTIFIC APPROACH TO DECISION-MAKING 4 "In just a few short weeks on the job, I had already realized that because every tough decision came down to a probability, then certainty was an impossibility — which could leave me encumbered by the sense that I could never get it quite right. So rather than let myself get paralyzed in the quest for a perfect solution, or succumb to the temptation to just go with my gut every time, I created a sound decision-making process — one where I really listened to the experts, followed the facts, considered my goals and weighed all of that against my principles. Then, no matter how things turned out, I would at least know I had done my level best with the information in front of me.” BARACK OBAMA WHY WE NEED A SCIENTIFIC APPROACH TO DECISION-MAKING 5 Poll time! How many subscribers did Disney Plus secure in its first year of existence? In November 2019, Disney launched its new Disney+ A) 10 millions streaming service. B) 20 millions When journalists asked Reed Hastings (the boss of C) 40 millions Netflix) how he thought Disney+ was going to perform D) 70 millions in its first year, Hastings said it would secure 20 million subscribers “at best”. At the time, Netflix had about 160 million subscribers. Its streaming service was launched in 2007. WHY WE NEED A SCIENTIFIC APPROACH TO DECISION-MAKING 6 Poll time! How many total ChotuKool units did Godrej sell in the first two years? A) 15,000 In 2008, Indian household appliances manufacturer B) 300,000 Godrej introduced ChotuKool, a low-cost refrigerator C) 2,500,000 targeted at the hundreds of millions of Indians who could not afford a regular refrigerator or did not have D) 12,000,000 stable access to electricity. The COO (chief operating officer) of Godrej said that they were expecting to sell “probably millions” of units in the first three years. WHY WE NEED A SCIENTIFIC APPROACH TO DECISION-MAKING 7 WHY WE NEED A SCIENTIFIC APPROACH TO DECISION-MAKING 8 February 2019 BMW and Daimler announced that they would invest $1 billion in Free Now, their car-sharing joint venture. Free Now included Share Now, the division centered on short-term car rentals, that already had 4m customers in more than 30 cities under the BMW brand DriveNow and Daimler’s Car2go. The plan was to extend Free Now’s services to another 90 cities and grow tenfold in the next three years. WHY WE NEED A SCIENTIFIC APPROACH TO DECISION-MAKING 9 December 2019 BMW and Daimler announced that Free Now would leave the United States and Canada due the “volatile state of the global mobility landscape” and the “rising infrastructure complexities facing North American transportation”. The business also said it would leave Brussels, London and Florence due to “low adoption rates”. DriveNow and Car2Go, which had already pulled out of more than a dozen locations, will continue to operate in 18 European cities, including seven in Germany. WHY WE NEED A SCIENTIFIC APPROACH TO DECISION-MAKING 10 WHY WE NEED A SCIENTIFIC APPROACH TO DECISION-MAKING 11 Uncertainty MON TUE WED THU FRI rules also in our daily lifes Think about weather forecast: MON TUE WED THU FRI which one is more informative? Chance of Chance of rainfall: Chance of Chance of Chance of rainfall: 20% 40% rainfall: 90% rainfall: 70% rainfall: 80% WHY WE NEED A SCIENTIFIC APPROACH TO DECISION-MAKING 12 3 main goals In this class we will learn how to make predictions using probability and updating our beliefs to make better decisions BE LESS CERTAIN LEARN TO MAKE THINK PREDICTIONS PROBABILISTICALLY Overconfidence depends Question how often an Have a theory to on culture, personality, outcome typically envision probability of expertise, … happen events 2.0 BASICS ON PROBABILITIES AND DISTRIBUTIONS BASICS ON PROBABILITIES AND DISTRIBUTIONS 14 Let’s introduce probability https://www.khanacademy.org/math/cc-seventh- grade-math/cc-7th-probability-statistics/cc-7th-basic- prob/v/basic-probability BASICS ON PROBABILITIES AND DISTRIBUTIONS 15 A random variable is a variable that can take on some values (x1, x2,..xn). Each value is assigned a probability (p1, p2,.. pn) Random variable Possible values Random events Probability 0 0.5 X= 1 0.5 BASICS ON PROBABILITIES AND DISTRIBUTIONS 16 Random variables - values CONTINUOUS QUALITATIVE / DICHOTOMOUS / CATEGORICAL BINARY Variables that take on a Variables that do not Variables that can take value in a range come from measuring or on only two values counting BASICS ON PROBABILITIES AND DISTRIBUTIONS 17 We define PROBABILITIES Probabilities indicate the likelihood of the occurrence of a random event Probabilities are a sequence of numbers [0,1] that have to sum up to 1 BASICS ON PROBABILITIES AND DISTRIBUTIONS 18 Cumulative Values Probabilities Probabilities 10 0.20 0.20 20 0.10 0.30 30 0.20 0.50 A random variable X can take on a 40 0.20 0.70 certain value based on the assigned 50 0.10 0.80 probability 60 0.05 0.85 i.e. the probability of getting a value 70 0.10 0.95 of X =10 is 0.2 or 20% 80 0.05 1.00 BASICS ON PROBABILITIES AND DISTRIBUTIONS 19 Cumulative Values Probabilities Probabilities 10 0.125 0.125 20 0.125 0.250 UNIFORM DISTRIBUTION 30 0.125 0.375 Each value has the same probability 40 0.125 0.500 X has an equal likelihood of being 50 0.125 0.625 any of these 8 outcomes. 60 0.125 0.750 70 0.125 0.875 i.e. the variable can take on 8 80 0.125 1.000 different values (10, 20, 30,.., 80) but the probability of each value is always 12.5% BASICS ON PROBABILITIES AND DISTRIBUTIONS 20 Graphical representation: rolling a dice has a uniform distribution probability 0.15 0.10 0.05 0 1 2 3 4 5 6 outcome 21 How informative is the uniform distribution? BASICS ON PROBABILITIES AND DISTRIBUTIONS 22 Cumulative Values Probabilities Probabilities 10 0.02 0.02 20 0.08 0.10 30 0.15 0.25 NORMAL DISTRIBUTION 40 0.25 0.50 In a normal distribution, the 50 0.25 0.75 outcomes tend to distribute around a 60 0.15 0.90 central value 70 0.08 0.98 i.e. in the table: Which values are 80 0.02 1.00 more likely? And which ones are less likely? 23 Can you think of examples where you may observe a normal distribution? BASICS ON PROBABILITIES AND DISTRIBUTIONS 24 Grade distribution BASICS ON PROBABILITIES AND DISTRIBUTIONS 25 Cumulative Values Probabilities Probabilities 10 0.02 0.02 20 0.03 0.05 30 0.05 0.10 NORMAL DISTRIBUTION 40 0.05 0.15 Left skewed: The values of the 50 0.10 0.25 observations cluster more around the 60 0.15 0.40 right side of the distribution 70 0.40 0.80 80 0.20 1.00 BASICS ON PROBABILITIES AND DISTRIBUTIONS 26 BASICS ON PROBABILITIES AND DISTRIBUTIONS 27 Cumulative Values Probabilities Probabilities 10 0.20 0.20 20 0.40 0.60 30 0.15 0.75 NORMAL DISTRIBUTION 40 0.10 0.85 Right skewed: values cluster more 50 0.05 0.90 around the left side of the distribution 60 0.05 0.95 70 0.03 0.98 80 0.02 1.00 BASICS ON PROBABILITIES AND DISTRIBUTIONS 28 BASICS ON PROBABILITIES AND DISTRIBUTIONS 29 These distributions are not very normal… Why? BASICS ON PROBABILITIES AND DISTRIBUTIONS 30 This distribution is normal… Why? 3.0 MEAN AND STANDARD DEVIATION MEAN AND STANDARD DEVIATION 32 Summary Statistics (or moments) PROBABILITY SUMMARY STATISTICS THE TWO MOST DISTRIBUTIONS HAVE ARE USED TO IMPORTANT SUMMARY STATISTICS, DESCRIBE DATA OR STATISTICS ARE OR MOMENTS THE SAMPLE — Mean — Standard deviation (= square root of the variance) 33 Do you think Bocconi’s students are tall or short? MEAN AND STANDARD DEVIATION 34 Mean Probably you have no clue! But if I tell you that the mean of the height of Bocconi students is 175 cm, then what would you answer? Mean =175 cm And if now I tell you that the mean of the height of the students at Politecnico is 180 cm, then what would you answer? Height of 15,000 ONE NUMBER CAN TELL YOU A LOT ABOUT MANY NUMBERS students at Bocconi MEAN AND STANDARD DEVIATION 35 Variance (or standard deviation) The mean tells you where the Means do not tell you whether the center of the distribution is values of the observations are positioned concentrated around it, or dispersed away from it Mean =175 cm Small variance : most of the Bocconi students’ heights are around 175 cm Height of 15,000 High variance: many Bocconi students at Bocconi students are either very short (155 cm) or very tall (195 cm) MEAN AND STANDARD DEVIATION 36 MEAN AND STANDARD DEVIATION 37 MEAN AND STANDARD DEVIATION 38 Cumulative Values Probabilities Probabilities 10 0.20 0.20 Mean formula: μ = σ𝑛𝑖=𝟏 𝑝𝑖 𝑥𝑖 , 20 0.10 0.30 Where xi is the value of an observation, and pi is the probability of whether that value 30 0.20 0.50 occurs. 40 0.20 0.70 50 0.10 0.80 The mean of the distribution in the table is: 60 0.05 0.85 10*0.20 + 20*0.10 + 30*0.20 + 40*0.20 + 50*0.10 + 60*0.05 + 70*0.10 + 80*0.05 = 37 70 0.10 0.95 80 0.05 1.00 MEAN AND STANDARD DEVIATION 39 Cumulative Values Probabilities Probabilities Variance formula: 𝜎 2 = σ𝑛𝑖=𝟏 𝑝𝑖 𝑥𝑖 − 𝜇 2 , 10 0.20 0.20 Where xi is the value of an observation, and pi is 20 0.10 0.30 the probability of whether that value occurs 30 0.20 0.50 The variance of the distribution in the table is: 40 0.20 0.70 (10 – 37)2*0.20 + (20 – 37)2*0.10 + 50 0.10 0.80 (30 – 37)2*0.20 + (40 – 37)2*0.20 + 60 0.05 0.85 (50 – 37)2*0.10 + (60 – 37)2*0.05 + 70 0.10 0.95 (70 – 37)2*0.10 + (80 – 37)2*0.05 = 431 80 0.05 1.00 Standard deviation = 𝑽𝒂𝒓𝒊𝒂𝒏𝒄𝒆 What’s the value of the standard deviation of the distribution? MEAN AND STANDARD DEVIATION 40 Poll time! Year ID Family_firm ROE ROA Turnover Sector What type of variable 2019 1 1 12.10 10.60 58,155.96 Manufacturing is “Family_firm”? 2019 2 0 9.80 6.74 316,792.54 Fashion 2019 3 0 5.42 3.15 22,631.89 Transport and logistics 2019 4 0 2.44 1.85 136,295.32 Metal products A) Qualitative 2019 5 0 6.37 2.66 51,651.85 Transport and logistics B) Continuous 2019 6 1 16.59 5.86 48,288.88 Wholesale 2019 7 1 30.41 7.26 152,355,00 Manufacturing C) Dichotomous 2019 8 1 9.94 5.22 36,519.24 Fashion 2019 9 0 9.40 4.71 194,447.17 Wholesale 2019 10 0 -12.81 -4.35 338,855.24 Metal products MEAN AND STANDARD DEVIATION 41 Poll time! Year ID Family_firm ROE ROA Turnover Sector What type of variable 2019 1 1 12.10 10.60 58,155.96 Manufacturing is “Turnover”? 2019 2 0 9.80 6.74 316,792.54 Fashion 2019 3 0 5.42 3.15 22,631.89 Transport and logistics 2019 4 0 2.44 1.85 136,295.32 Metal products A) Qualitative 2019 5 0 6.37 2.66 51,651.85 Transport and logistics B) Continuous 2019 6 1 16.59 5.86 48,288.88 Wholesale 2019 7 1 30.41 7.26 152,355,00 Manufacturing C) Dichotomous 2019 8 1 9.94 5.22 36,519.24 Fashion 2019 9 0 9.40 4.71 194,447.17 Wholesale 2019 10 0 -12.81 -4.35 338,855.24 Metal products MEAN AND STANDARD DEVIATION 42 Poll time! Year ID Family_firm ROE ROA Turnover Sector What type of variable 2019 1 1 12.10 10.60 58,155.96 Manufacturing is “Sector”? 2019 2 0 9.80 6.74 316,792.54 Fashion 2019 3 0 5.42 3.15 22,631.89 Transport and logistics 2019 4 0 2.44 1.85 136,295.32 Metal products A) Qualitative 2019 5 0 6.37 2.66 51,651.85 Transport and logistics B) Continuous 2019 6 1 16.59 5.86 48,288.88 Wholesale 2019 7 1 30.41 7.26 152,355,00 Manufacturing C) Binary 2019 8 1 9.94 5.22 36,519.24 Fashion 2019 9 0 9.40 4.71 194,447.17 Wholesale 2019 10 0 -12.81 -4.35 338,855.24 Metal products MEAN AND STANDARD DEVIATION 43 Poll time! Year ID Family_firm ROE ROA Turnover Sector What type of variable 2019 1 1 12.10 10.60 58,155.96 Manufacturing is “ROE”? 2019 2 0 9.80 6.74 316,792.54 Fashion 2019 3 0 5.42 3.15 22,631.89 Transport and logistics 2019 4 0 2.44 1.85 136,295.32 Metal products A) Qualitative 2019 5 0 6.37 2.66 51,651.85 Transport and logistics B) Continuous 2019 6 1 16.59 5.86 48,288.88 Wholesale 2019 7 1 30.41 7.26 152,355,00 Manufacturing C) Dichotomous 2019 8 1 9.94 5.22 36,519.24 Fashion 2019 9 0 9.40 4.71 194,447.17 Wholesale 2019 10 0 -12.81 -4.35 338,855.24 Metal products MEAN AND STANDARD DEVIATION 44 Poll time! Year ID Family_firm ROE ROA Turnover Sector How do you expect 2019 1 1 12.10 10.60 58,155.96 Manufacturing turnover to be 2019 2 0 9.80 6.74 316,792.54 Fashion distributed? 2019 3 0 5.42 3.15 22,631.89 Transport and logistics 2019 4 0 2.44 1.85 136,295.32 Metal products 2019 5 0 6.37 2.66 51,651.85 Transport and logistics A) Normally 2019 6 1 16.59 5.86 48,288.88 Wholesale distributed 2019 7 1 30.41 7.26 152,355,00 Manufacturing 2019 8 1 9.94 5.22 36,519.24 Fashion B) Left skewed 2019 9 0 9.40 4.71 194,447.17 Wholesale C) Right skewed 2019 10 0 -12.81 -4.35 338,855.24 Metal products 4.0 CONDITIONAL PROBABILITY AND JOINT PROBABILITY CONDITIONAL PROBABILITY AND JOINT PROBABILITY 46 What is conditional probability? The probability of an event (situation, characteristic, condition, …) occurring given that another event has already taken place CONDITIONAL PROBABILITY AND JOINT PROBABILITY 47 Conditional probability is everywhere If you want to know the probability that your friend has Covid, it is useful to know whether she is vaccinated! If you want to know the probability that Messi goes back to Barcelona FC the next season, it is useful to know whether the team can afford to pay him If you want to know the probability that customers will buy your new product, it is useful to know what a sample of them think of the test version. CONDITIONAL PROBABILITY AND JOINT PROBABILITY 48 Should Share Now be in Barcelona? Madrid is currently the only city in Spain in which Share Now operates. You are an intern at Share Now and your boss asks you to assess the business case of entering Barcelona. That is, they want to know how probable it is that Share Now could turn a profit in that city. Your boss told you that they want the average subscriber to drive at least 20 hours per year. What variables do you think the probability of turning a profit could depend on? CONDITIONAL PROBABILITY AND JOINT PROBABILITY 49 Should Share Now being in Barcelona? Consider per-capita income. Is it make more likely that Share Now will turn a profit in the city? Maybe yes (high-income people do not like to waste time in the traffic and are ready to pay for rental of small cars) Maybe not (high-income people like to own their car) Maybe it has not impact (not a variable or different effects that balance each other) You usually need to check data to know whether an event (like profit) depend on something else (like per-capita income). CONDITIONAL PROBABILITY AND JOINT PROBABILITY 50 Area of origin Italy Asia Others MARGINAL 2 0 2 0 2 The frequency table to the left reports the 3 1 5 0 6 number of letters of the last name of 93 4 3 1 0 4 students based on whether originate from 5 9 2 2 13 Italy, Asia or somewhere else 6 8 2 4 14 # letters in last 7 21 0 5 26 name The number of letters in the last name is 8 9 0 2 11 (X) our random variable X 9 7 0 2 9 10 4 0 0 4 11 1 0 0 1 This type of table is called a 14 0 0 2 2 “contingency table”: it shows the values 23 0 0 1 1 of X contingent on another variable. MARGINAL 63 12 18 93 The totals of rows and columns are marginal frequencies (that is, the “last” row/column). CONDITIONAL PROBABILITY AND JOINT PROBABILITY 51 Area of origin Italy Asia Others MARGINAL 2 0 2 0 2 Conditional probability: P(X|Y) 3 1 5 0 6 4 3 1 0 4 What is the probability of a student with 5 5 9 2 2 13 letters in his or her last name? 6 8 2 4 14 # letters E.g. 13/93 = 13.9% in last 7 21 0 5 26 name 8 9 0 2 11 What is the probability of a student with 5 (X) 9 7 0 2 9 letters in his or her last name GIVEN that 10 4 0 0 4 the student is Italian? 11 1 0 0 1 E.g. P(X=5|Italy) = 9/63 = 14.3% 14 0 0 2 2 23 0 0 1 1 The conditioning event is often called a MARGINAL 63 12 18 93 condition, clue, data point or SIGNAL (being Italian) CONDITIONAL PROBABILITY AND JOINT PROBABILITY 52 Are conditional probabilities a bit like Akinator? Yes, they are. You want to guess the animal and you usually start with a uniform probability distribution in your mind in which every option has the same likelihood ( = you have initially no clue). Then you ask questions to collect clues, that is, you move from the initial uniform marginal distribution to more precise conditional distributions until you think that: P(Panda bear|all the conditions) = 1 CONDITIONAL PROBABILITY AND JOINT PROBABILITY 53 What is Conditional Expectation? The expected value of a random variable, given that another event has already taken place CONDITIONAL PROBABILITY AND JOINT PROBABILITY 54 Area of origin Italy Asia Others MARGINAL Conditional expectation: 2 0 2 0 2 3 1 5 0 6 E(X|Y) = σ𝑛𝑖=𝟏 𝑝(𝑥𝑖 |Y) 𝑥𝑖 4 3 1 0 4 That is, you multiply all the values by their 5 9 2 2 13 respective conditional probabilities, 6 8 2 4 14 # letters contingent on Y, and then you add them up. in last 7 21 0 5 26 name 8 9 0 2 11 (X) E(X | Italy) = 2* 0/63 + 3* 1/63 + 4* 3/63 + 5* 9 7 0 2 9 10 4 0 0 4 9/63 + 6* 8/63 + 7* 21/63 + 8* 9/63 + 9* 7/63 11 1 0 0 1 + 10* 4/63 + 11* 1/63 + 14* 0/63 + 23* 0/63 = 14 0 0 2 2 7 23 0 0 1 1 MARGINAL 63 12 18 93 E( X | Italy) = 7 = # of letters the last name will have on average given the student is Italian. CONDITIONAL PROBABILITY AND JOINT PROBABILITY 55 What is Joint probability? The probability that two events take place together at the same time CONDITIONAL PROBABILITY AND JOINT PROBABILITY 56 Area of origin Italy Asia Others MARGINAL 2 0 2 0 2 Joint probability: 3 1 5 0 6 P(X,Y) = P(X|Y)*P(Y) 4 3 1 0 4 5 9 2 2 13 That is, the probability that Y happens # letters 6 8 2 4 14 times the probability that X happens once in last 7 21 0 5 26 we know that Y happened name 8 9 0 2 11 (X) 9 7 0 2 9 10 4 0 0 4 E.g. 11 1 0 0 1 P(X=5, Italy) = P(X=5 | Italy) * P (Italy) = 14 0 0 2 2 23 0 0 1 1 = 9/63*63/93 = 14.3% * 67.7% = 9.7% = MARGINAL 63 12 18 93 probability that the student is Italian AND the last name has 5 words CONDITIONAL PROBABILITY AND JOINT PROBABILITY 57 Notice the difference between conditional and joint probabilities CONDITIONAL PROBABILITY JOINT PROBABILITY P(X|Y) P(X,Y)= P(X|Y)*P(Y) P(X=5|Italy) = 9/63 = 14.3% P(X=5, Italy) = 9/63*63/93 = 9.7% CONDITIONAL PROBABILITY AND JOINT PROBABILITY 58 Let’s practice A TLC company is investigating its retention of customers. It selected a sample of 2,185 customers who signed the contract five years ago. Some of them remained (they still have the contract), others left to other providers. The company believes that the age group of customers could have an impact on the retention rate. Millennials (25- Boomers and Gen Z (up to 24) Gen X (41-56) TOTAL 40) older (57+) Remained 200 115 180 210 705 Left 120 160 530 670 1,480 TOTAL 320 275 710 880 2,185 CONDITIONAL PROBABILITY AND JOINT PROBABILITY 59 Let’s practice In this sample: what is the probability of retaining a customer? what is the probability of retaining a Gen Z customer? what is the probability of being a Gen Z customer who remains? Millennials (25- Boomers and Gen Z (up to 24) Gen X (41-56) TOTAL 40) older (57+) Remained 200 115 180 210 705 Left 120 160 530 670 1,480 TOTAL 320 275 710 880 2,185 CONDITIONAL PROBABILITY AND JOINT PROBABILITY 60 Independence Do you remember per-capita income and Share Now in Barcelona? It is always possible that a variable is not relevant. If the conditioning event, or signal, is independent from the event we are interested in, then it does not help predict it In this case, the signal, is not informative CONDITIONAL PROBABILITY AND JOINT PROBABILITY 61 What is the joint probability of rolling two 6’s? CONDITIONAL PROBABILITY AND JOINT PROBABILITY 62 Joint probability with independent events: rolling two dices EVENT 1 EVENT 2 JOINT PROBABILITY OF EVENT 1 AND 2 probability of rolling 6 in probability of rolling 6 in probability of (EVENT 1) the first roll = 1/6 the second roll = 1/6 X probability of (EVENT 2) = 1/6 X 1/6 Joint probability: P(X,Y) = P(X|Y)*P(Y) But, if X and Y are independent, P(X|Y) = P(X) So, in case of independence: Joint probability = P(X|Y)*P(Y) = P(X)*P(Y) In this case, X = probability of rolling 6 in the first round Y = probability of rolling 6 in the second round CONDITIONAL PROBABILITY AND JOINT PROBABILITY 63 Area of origin Italy Asia Others MARGINAL Independence is a matter of 2 0 2 0 2 degree! 3 1 5 0 6 4 3 1 0 4 P(X=5 | Italy) = 9/63 = 14.3% 5 9 2 2 13 P(X=5) = 13/93 = 14.0% 6 8 2 4 14 # letters in last 7 21 0 5 26 name Although the two events (five letters and being 8 9 0 2 11 (X) Italian) are not independent, the conditional 9 7 0 2 9 10 4 0 0 4 probability (five letters when you are Italian) is very 11 1 0 0 1 close to the marginal probability of having five 5 14 0 0 2 2 letters… 23 0 0 1 1 MARGINAL 63 12 18 93 So, being Italian is not a very informative signal. CONDITIONAL PROBABILITY AND JOINT PROBABILITY 64 Area of origin Italy Asia Others MARGINAL What about whether being Asian 2 0 2 0 2 or Italian predicts whether your 3 1 5 0 6 4 3 1 0 4 last name has 3 letters? 5 9 2 2 13 6 8 2 4 14 # letters in last 7 21 0 5 26 P(X=3 | Asia)= 5/12 = 41.7% name 8 9 0 2 11 P(X=3) = 6/93 = 6.4% (X) 9 7 0 2 9 10 4 0 0 4 P(X=3 | Italy) = 1/63 = 1.6% 11 1 0 0 1 P(X=3) = 6/93 = 6.4% 14 0 0 2 2 23 0 0 1 1 Which one is more informative? MARGINAL 63 12 18 93 Remember: prediction can go either ways 5.0 PREDICTION OF EVENTS PREDICTION OF EVENTS 66 Why is probability relevant to management? PREDICT EVENTS DECIDE ACTIONS PREDICTION OF EVENTS 67 Why is the new robot failing? Your company has recently bought a new robot for the factory, which has shown signs of malfunction. The robot starts regularly but after a few hours, on certain days, it comes to a halt all of a sudden. After thinking about why this may be the case, you hypothesize that the robot fails when the temperature in the factory is low. So, you ask your engineers to have a look at the factory records and collect the temperatures of the days the robot failed. PREDICTION OF EVENTS 68 Poll time! The engineers came back with this graph, which tells you the number of accidents at different Are the failures of the recorded temperatures. robot due to low temperature? A) Yes B) No 50 degrees F = 10 degrees C 75 degrees F = 23.9 degrees C PREDICTION OF EVENTS 69 Think again Your theory is an if-then statement. If the temperature in the factory is low, then the robot fails. How do you test this theory? By looking at all the days in which the temperature is low, and then checking whether the robot fails on those days. It’s like the theory: “If mum is happy, she gives me chocolate”. You want her to give you chocolate every time she is happy. PREDICTION OF EVENTS 70 You need more data The engineers gave you only the temperatures of the days when the robot failed. What about the days without incidents? Maybe the temperature was low on those days too and nothing happened… You tell the engineers. They come up with a new graph with the temperatures of all the days in which the robot operated. PREDICTION OF EVENTS 71 The complete graph What’s your conclusion now? PREDICTION OF EVENTS 72 Table version Days The first graph showed you only Days with without Total the first column (days with accidents accidents incidents). You could calculate: Low 6 0 6 temp P(Low temperature | accident) = (< 65° F) = 6/10 = 60% High 4 17 21 temp (≥ 65° F) But, to test your theory, what you Total 10 17 27 need is the full table to test this probability: P(accident | Low temperature) = = 6/6 = 100% PREDICTION OF EVENTS 73 Definition of theory A series of logical steps linking antecedents to consequences If P then Q, if Q then R, if R then S,..., if V then Z... You eventually show that if P then Z PREDICTION OF EVENTS 74 Why are theories important? Theories tell you what data / factors to examine The factors that affect your outcome improve your ability to make an accurate prediction The data that you find provide signals for your conditional probabilities, i.e., for improving your predictions In practice: 1. You theorize “if A, then B” 2. You collect all the cases with A, and then you measure B in them 3. You calculate P(B|A) 4. If needed, you repeat with a theory about A, and so on PREDICTION OF EVENTS 75 It wasn’t actually a robot PREDICTION OF EVENTS 76 How to predict events You have a target event You want to predict whether it will occur Example: are there at least 10 supporters of Inter Milan in this class? PREDICTION OF EVENTS 77 Step 1 We need a theory! PREDICTION OF EVENTS 78 Step 1 What theory do you have to evaluate whether or not there are at least 10 supporters of Inter Milan in the class? What factors would you use to build What’s your theory? Maybe you look around and check how your many male students there are in the theory? class. Or, how many are from Milan. PREDICTION OF EVENTS 79 Step 1 Based on your theory, you envision a certain probability (e.g. 60%) that there can be at least 10 supporters of Inter Milan in the class. What’s This is called prior probability. your Prior probability is the predicted theory? probability of an event before data is collected. It is the best assessment of the probability of an outcome based on the current knowledge Write down your prior probability! PREDICTION OF EVENTS 80 Step 2 Now that you have a prior probability you decide whether to continue with this probability or to look for more information/signals. Revise your Signals are costly and you usually cannot theory collect too many of them. At least two ways to collect signals: 1. Ask others (experts) 2. Collect data (data analysis) How do you apply them to your research on Inter Milan supporters? PREDICTION OF EVENTS 81 Step 2 Try to improve your prior probability by asking the classmates sitting close to you what they think the probability of having at least 10 students Revise your supporting Inter Milan is. theory: Ask others PREDICTION OF EVENTS 82 – They say 80%... In this case you may Step 2 update your probability to a higher level than your prior of 60% (e.g. to 70%) – They say 40%... in which case you update Revise your probability to a lower level than your prior of 60% (e.g. to 50% ) your The new probability is a posterior probability. theory: Posterior probability is the revised Ask others probability of an event occurring after taking new information into account. Write down your posterior probability! PREDICTION OF EVENTS 83 Step 2 Let’s collect data now. Take a sample of 20 students in the class and check how many of them are supporters of Inter Milan. Revise – If they are at least 2 (10%), update your your posterior probability upward theory: – If not, update your posterior probability downward Ask others Write down your NEW posterior probability! PREDICTION OF EVENTS 84 Predict events STEP 1 – THEORY STEP 2 – LOOK FOR STEP 3 – DEFINE YOUR MORE INFORMATION EXPECTATIONS You look at the class Then you ask friends You decide when to (100 students) with a (experts and collect stop collecting theory in mind. data). data/signals and to You set a prior You update you prior finalize your probability. probability (multiple probability, that is, a times), generating posterior probability posterior probabilities that you cannot improve further PREDICTION OF EVENTS 85 Technically P(Y) = 60% P(Y|S) = PROBABILITY OF PRIOR PROBABILITY EVENT Y GIVEN THE OBSERVED SIGNAL S Y = at least 10 S: what the data or your friends students in the class told you support Inter Milan — If S = 80% → P(Y|S=80%) = 70% — If S = 40% → P(Y|S=40%) = 50% PREDICTION OF EVENTS 86 Technically There could be a series of priors, signals, posteriors, in which each posterior becomes the prior before the next signal s1 s2 s3 P(Y) P(Y | s1) P(Y | s1,s2) P(Y | s1,s2,s3) For example: P(Y) =60%, New data collection tells you S 2= 45% Data tell you S1=40%, You update P(Y | S1=40%, S2=45%) = 48% You update P(Y | S1=40%) = 50% And so on…. PREDICTION OF EVENTS 87 So… how many supporters of Inter Milan in this class? Raise your hand if you are a supporter of Inter Milan. 6.0 MAKING DECISIONS MAKING DECISIONS 89 From WHY DO WE NEED TO PREDICT EVENTS? predicting events to An event is You want to making associated with the outcome of an decide whether or not to take that decisions action action and estimating payoffs MAKING DECISIONS 90 From WHY DO WE NEED TO PREDICT EVENTS? predicting Example events to Pay 1,000 € upfront, and you receive 100 € per student who support Inter Milan in this class making (100 students). Therefore: decisions if at least 10 students support Inter you break-even (total cost = 1,000€; total and income = 100€ X 10 students = 1,000€ ) estimating if there are less than 10 supporters, you lose money payoffs if there are more than 10 supporters, you gain money 91 Should you pay 1,000€ upfront? How to approach the decision? MAKING DECISIONS 92 You are facing this situation Scenarios At least 10 Inter Less than 10 Inter Actions supporters supporters Pay 1,000 upfront PROFIT LOSS Not pay 1,000 upfront 0 0 Actions = actions you are going to take Scenarios = states of the world you are going to face MAKING DECISIONS 93 You are facing this situation Scenarios At least 10 Inter Less than 10 Inter Actions supporters supporters Pay 1,000 upfront PROFIT LOSS Not pay 1,000 upfront 0 0 Actions = actions you are going to take Scenarios = states of the world you are going to face This is the set of options that is available to you. You decide on this. MAKING DECISIONS 94 You are facing this situation Scenarios At least 10 Inter Less than 10 Inter Actions supporters supporters Pay 1,000 upfront PROFIT LOSS Not pay 1,000 upfront 0 0 Actions = actions you are going to take Scenarios = states of the world you are going to face This is what could happen. You do not control it. This is what you PREDICT. MAKING DECISIONS 95 You are facing this situation Scenarios At least 10 Inter Less than 10 Inter Actions supporters supporters Pay 1,000 upfront PROFIT LOSS Not pay 1,000 upfront 0 0 Actions = actions you are going to take Scenarios = states of the world you are going to face These are the outcomes of each action- scenario. This is what you are assessing MAKING DECISIONS 96 You are facing this situation Scenarios At least 10 Inter Less than 10 Inter Actions supporters supporters Pay 1,000 upfront PROFIT LOSS Not pay 1,000 upfront Neither profit or loss Neither profit or loss How to proceed? Intuitively, the HIGHER the probability that at least 10 students support Inter Milan, the more likely it is that you get a PROFIT. PREDICT = estimate the probability of the different outcomes MAKING DECISIONS 97 How do you decide? Assume your probability is 52%. Do you pay 1,000 upfront? And if it is 55%? You have already worked on probability estimation. You have a final posterior probability. And if it is 60%? But assume you do not know the real value yet (whether there are at least 10 supporters) You need a criterion MAKING DECISIONS 98 Establish a criterion APPROACH 1 APPROACH 2 Think of a Assign values threshold (profits and losses) probability MAKING DECISIONS 99 1. Threshold You think of a threshold P* such that: if your expected payoff P(Y|S) ≥ P*, then you go ahead with the action if your expected payoff P(Y|S) < P*, then you don’t IMPORTANT Decision-makers should select the threshold before they estimate their expected payoff or see the data. Doing it after often generates confirmatory biases (you choose the threshold you want) leading to bad decisions. MAKING DECISIONS 100 I recently went clothes shopping in Brooklyn with my friend Emma. Showing off a pretty dress, she tugs at the price tag on the back. “Hey, what does this say?” she asks me. “If it’s less than 80 bucks, I’ll buy it.” Now that’s some decision intelligence! Instead of first seeing the price and then talking herself into a decision she’s already made, she uses the data to drive it. With a well-practiced reflex, she weighs how much she likes the dress and her budget, then sets the decision boundary … https://hackernoon.com/data-inspired-5c78db3999b2 Cassie Kazyrkov | Chied Data Scientist at Google MAKING DECISIONS 101 2. Given the probability that you have estimated, you assign values to profits and losses in the Assign values action-scenario matrix. Two approaches. - Value model (you calculate monetary values of profits and losses that you could make in the different scenarios) - Utility model (you attribute «utilities» that represent how much you enjoy a positive outcome or how much you suffer a loss) MAKING DECISIONS 102 Assign values: Value model Scenarios At least 10 Inter Less than 10 Inter Actions supporters supporters Pay 1,000 upfront +500€ -500€ Not pay 1,000 upfront 0 0 You estimate that: if there are at least 10 students supporting Inter, on average there are 15 students supporting Inter → revenue – cost = 15x100€ – 1000€ = 500€ if there are fewer than 10 students supporting Inter, on average there are 5 students supporting Inter → revenue – cost = 5x100€ – 1000€ = -500€ 103 Compute the expected value. Is it greater than 0? MAKING DECISIONS 104 Assign values: Value model Scenarios At least 10 Inter Less than 10 Inter Actions supporters supporters Pay 1,000 upfront +500€ -500€ Not pay 1,000 upfront 0 0 Expected value (Pay 1,000) = 500 * P(Y|S) – 500 * (1 – P(Y|S) Expected value (Not pay 1,000) = 0 * P(Y|S) + 0 * (1 – P(Y|S) = 0 + 0 = 0 Example: P(Y|S) = 55% Expected value (Pay 1,000) = (500 * 0.55) – (500 * (1 – 0.55)) = = (500 * 0.55) – (500 * 0.45) = = 275 – 225 = 50 CRITERION: You choose the action with the greater expected value. MAKING DECISIONS 105 Assign values: Value model The value model derives a threshold probability based on the expected outcomes. Threshold in the value model: the minimum probability required to make the expected value ≥ 0. The Expected value is 500 * P(Y|S) – 500 * (1 – P(Y|S) We want: Expected value ≥ 0. It is then easy to see that this inequality is true if P(Y|S) ≥ ½ , which will then be your threshold (P* = 50%) MAKING DECISIONS 106 Assign values: Utility model Utilities: how much you enjoy a positive outcome or how much you suffer from experiencing a loss This depends on: - Monetary values (money is useful…) - Other aspects of the decision E.g. you may be very worried about losing money (risk aversion) and the loss of 500 € has a disutility of 700 Or you are very excited if you run a successful project, and turn the profit of 500 € has an utility of 1,000 Then you calculate the Expected utility of the actions (same as expected value, but with utilities in place of monetary values). MAKING DECISIONS 107 Assign values: Utility model Scenarios At least 10 Inter Less than 10 Inter Actions supporters supporters Pay 1,000 upfront 800 -400 Not pay 1,000 upfront 0 0 For instance, you set utility equal to 800 if the project succeeds; -400 if it does not. The expected utility is 800*P(Y|S) – 400*(1 – P(Y|S)). Threshold: Expected utility ≥ 0 when P(Y|S) > 1/3 or P* = 33% MAKING DECISIONS 108 Value model vs Utility model 1. The utility model or the value model are formally equivalent. You can see the value model as a special case of the utility model in which your utility depends only on monetary value. 2. The difference is that you base utility on what is important to you MAKING DECISIONS 109 Value model vs Utility model Broad and rigorous Utility approach to introduce model sense-making in managerial decisions Traditional financial Value model planning + probability MAKING DECISIONS 110 Wrap up: A framework for managerial decision-making DEFINE DEFINE A MAP OF ESTIMATE THE MAKE A DECISION ACTIONS ACTION- PROBABILITY AND SCENARIO PAIRS SCENARIOS What are the What are the Have a theory, Based either on a possible courses of possible estimate your predefined action? What can combinations? priors, and decide threshold or on you control? What Assign the payoffs whether to revise monetary you cannot? derived from pair. them values/utility MAKING DECISIONS 111 The framework can accommodate complex decisions Your scenario-action pairs can also have more actions and scenarios, such as the following: Scenarios High Medium Low Very low Actions demand demand demand demand Produce green hats profit profit profit loss Produce red hats profit profit loss loss Produce blue hats profit profit loss loss Do not produce hats 0 0 0 0 In this case you still estimate the probability of the different scenarios, find the highest expected value given these probabilities, and take the action associated with the highest expected value (which could be the default action) 7.0 APPLICATION: SOUTHWEST AIRLINES SOUTHWEST AIRLINES 113 Should Southwest introduce fees? We are in 2016. — Analysts are pressuring Southwest to introduce fees to increase revenue — Southwest’s CEO is worried that fees could undermine customer trust How would you apply our framework to this decision? SOUTHWEST AIRLINES 114 1. Actions General recommendation: let’s keep the model as simple as possible, e.g. minimum number of actions, scenarios, numbers that are easy to calculate, basic theories, etc. There is always time to complicate it later if necessary! WHAT ACTIONS ARE AVAILABLE TO SOUTHWEST AIRLINES? SOUTHWEST AIRLINES 115 1. Actions Which scenarios are relevant to the actions we are considering? 2. Scenarios - They must be important for our decision - They should be out of our control (once we take the action) - We have to be able to estimate the probability that they happen - They should produce payoffs (values/utilities) (depending on our actions) WHICH SCENARIOS SHOULD YOU CONSIDER? SOUTHWEST AIRLINES 116 1. Actions Let’s choose a decision-making framework. Three options: 2. Scenarios 3. Decision model - Threshold - Value Model - Utility Model It’s important that you choose a model you can actually use, given the information that you have. WHICH DECISION MAKING FRAMEWORK DO YOU WANT TO USE? SOUTHWEST AIRLINES 117 Scenarios Customer Customer 1. Actions trust not trust Actions 2. Scenarios impacted damaged 3. Decision model Introduce fees ? ? 4. Utilities Not introduce ? ? fees The utility derived should be linked to the strategy that Southwest Airlines is pursuing. WHAT UTILITIES DO YOU WANT TO INPUT? SOUTHWEST AIRLINES 118 Scenarios Customer Customer 1. Actions trust not trust Actions 2. Scenarios impacted damaged 3. Decision model Introduce fees 100 -300 4. Utilities Not introduce 0 0 fees We can assign utilities to reflect the serious damage on Southwest that results from implementing a strategy that damages customer trust SOUTHWEST AIRLINES 119 Scenarios Customer Customer 1. Actions trust not trust Actions 2. Scenarios impacted damaged 3. Decision model Introduce fees 100 -300 4. Utilities Not introduce 0 0 5. Threshold fees We can now calculate how probable we want the event ”customer trust not impacted” to be, in order to introduce fees. WHAT IS THE THRESHOLD? SOUTHWEST AIRLINES 120 1. Actions The threshold is 75%. We can now ask ourselves: 2. Scenarios 3. Decision model WHAT IS THE PROBABILITY THAT 4. Utilities CUSTOMER TRUST IS NOT IMPACTED BY 5. Threshold THE INCREASE IN FEES? 6. Estimate probability SOUTHWEST AIRLINES 121 1. Actions Have a theory: What are the antecedents of customer trust, for an airline company? 2. Scenarios 3. Decision model Theory: If an airline company is …, or does 4. Utilities …, then customers trust it. 5. Threshold 6. Estimate For example, research shows that trust probability depends a lot on keeping promises. Is this - Have a theory relevant in this case? WHAT IS YOUR PRIOR THAT CUSTOMER TRUST IS NOT IMPACTED BY THE INCREASE IN FEES? SOUTHWEST AIRLINES 122 1. Actions Ask experts: Who could you speak to, or what data can you look for, to improve your prior? 2. Scenarios 3. Decision model Consultants 4. Utilities Equity analysts 5. Threshold Experienced Southwest’s managers 6. Estimate What the other airline companies have probability already done (WestJet, JetBlue) - Have a theory Loyal customers (surveys, focus groups) - Ask experts …. DO YOU WANT TO UPDATE YOUR PRIOR? SOUTHWEST AIRLINES 123 1. Actions Collect data: You could run an experiment on a route or few routes, as suggested by 2. Scenarios the J.P. Morgan’s analyst. 3. Decision model Remember: signals can be costly. 4. Utilities Which is the cost of the signal, in this case? 5. Threshold 6. Estimate probability AFTER THE EXPERIMENT (IF YOU RUN - Have a theory IT), YOU ARRIVE AT YOUR FINAL - Ask experts ESTIMATE - Collect data You make your decision based on whether the final estimate is larger or smaller than the threshold SOUTHWEST AIRLINES 124 What did Southwest do? It has never changed its fee policy. It has never ran an experiment either. SOUTHWEST AIRLINES 125 How did Southwest do? US domestic flights % market share (2010-2019) Operating margin (2010-2019) 100.0 25.0% 90.0 20.8% 20.0% 36.5 36.6 37.0 37.5 19.2% 80.0 39.8 18.4% 46.7 45.2 17.5% 51.5 51.7 49.3 16.6% 70.0 15.0% 15.1% 14.8% 14.6% 13.6% 13.1% 13.9% 13.2% 12.0% 11.9% 12.4% 60.0 10.0% 10.1% 9.6% 9.9% 20.6 20.7 20.4 19.5 9.3% 50.0 20.4 8.2% 8.6% 8.0% 7.2% 7.2% 7.2% 6.7% 17.9 19.1 6.5% 6.4% 6.1% 6.1% 15.2 5.8% 40.0 16.9 17.4 5.0% 4.8% 4.2% 3.6% 16.5 16.3 16.2 16.8 3.3% 30.0 16.5 14.9 15.2 16.0 0.7% 0.2% 0.0% 0.1% 14.3 14.5 20.0 2010 2011 2012 2013 2014 2015 2016 2017 2018 2019 16.2 15.7 15.3 15.5 10.1 10.1 13.4 10.0 10.4 10.2 -5.0% -4.9% 10.0 10.5 10.1 9.7 9.9 10.2 10.8 11.0 10.8 6.9 6.2 0.0 2010 2011 2012 2013 2014 2015 2016 2017 2018 2019 -10.0% United Airlines American Airlines Delta Air Lines American Airlines Delta Air Lines Southwest Others United Airlines Southwest THANK YOU