PSY260 Laboratory Workshop 6: Experimental Designs & ANOVA
Document Details
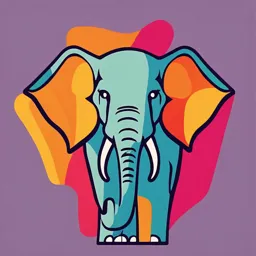
Uploaded by WellWishersConnemara2829
Klein Independent School District
Tags
Summary
This document presents the content for Laboratory Workshop 6 of PSY260, focusing on experimental designs and ANOVA. It covers topics such as experimental design recap, between-subjects and within-subjects designs, ANOVA, and post-hoc tests. The document provides theoretical knowledge and practical guidance for students to conduct and interpret statistical analysis.
Full Transcript
Building on Experimen tal Designs Laboratory Workshop 6 PSY260 24/25 In today’s session: A brief recap of experimental designs from Stage 1 Expanding upon experimental designs Considering independent variable with 3+ levels (for both between- and within- designs) Practicing c...
Building on Experimen tal Designs Laboratory Workshop 6 PSY260 24/25 In today’s session: A brief recap of experimental designs from Stage 1 Expanding upon experimental designs Considering independent variable with 3+ levels (for both between- and within- designs) Practicing conducting, interpreting, and reporting different analyses Course Representation Course representation is very important both for you and for us. We need course representatives on each stage of each UG programme. Please have a look at the SU Website for more information about the role. You have until Friday 1st November to put yourself forward to be a Course Rep. Complete the nomination form and a member of the Voice team will be in touch about attending training. Experimental Designs: A Recap We have a single independent variable with two levels (groups or conditions). Everything should stay the same except for these two levels By having greater control within our study, experiments allow us: To collect the strongest type of evidence To better isolate cause and effect Any significant differences observed in the outcome (i.e. our dependent variable) are then attributed to (i.e. caused by) the differences between the levels in question For example: We might look to compare two different groups (e.g. extroverts vs. introverts) or two different conditions (e.g. cue word vs. cue emoji) in relation to Episodic Future Thinking (EFT) Between-Subjects: score participants on neuroticism (grouping into either high or low) and then complete several trials where they are given the same cue words (randomised order) and asked the same questions (e.g. how far into the future are you thinking? how intense are the emotions you’re experiencing?) Pp are in one of the two levels of your IV Within-Subjects: participants aren’t scored on any individual difference factor, but instead take part in a series of different conditions. For example, they may have several trials where they are given positive cue words and several trials where they are given negative cue words (counterbalanced) Pp are in both levels of your IV Experimental Designs: A Recap Between-Subjects (IG) Within-Subjects (RM) Pp are only in one level of Pp take part in both levels of the IV the IV Less practice/fatigue Much easier to compare effects scores as individual Less dropout (aka attrition) differences are lessened (less noise) Harder for Pp to work out Can be prone to order effects the aim of the study (e.g. practice, boredom, But have to balance two fatigue) groups to control for noise Counterbalancing helps to reduce this Experimental Analysis: A Recap For analysis, we assess whether the means of our two levels are significantly different or not As long as the data was parametric (e.g. continuous, normally distributed) we used t-tests (ideally with Welch’s correction) Either Independent t-test (for between-subjects) Or, Repeated t-test (for within-subjects) If the data was non-parametric (e.g. violated assumptions), we used either: A Mann-Whitney U (for between-subjects) Or, A Wilcoxon Signed-Rank Test (for within-subjects) If the p-value was below the alpha level (.05), we would reject our H0, and conclude that there was a difference between our two levels But what if your IV had more than two levels?! 45 Say you were comparing the effects of stress levels on 40 35 performance. If we had just ‘high’ and ‘low‘ stress, we Performance 30 25 could just do a t-test 20 However, splitting stress levels into high and low is 15 10 5 rather inaccurate, so we could categorise as ‘high’, 0 low high ‘medium’, and ‘low’ stress levels instead Stress N.B. need to think about how we’re splitting our sample But a t-test only compares two levels, so we’d need to do three t-tests: High vs. low High vs. medium Medium vs. low Familywise Error Rate 1 Probability of at least one Type 0.9 0.8 Leve Comparis 0.7 Red ls ons 0.6 Oran 3 3 I error 0.5 ge 4 6 0.4 Yello 5 10 0.3 w 6 15 0.2 Gree 7 21 0.1 n 8 28 0 Blue 0 1 2 3 4 5 6 7 8 9 10 11 12 13 14 15 16 17 18 19 20 21 22 23 24 25 26 27 28 29 30 31 32 33 34 35 36 37 38 39 40 41 42 43 44 45 46 47 48 49 50 Purpl Number of e comparisons ANalysis Of VAriance (ANOVA) can essentially be thought of as multiple t- tests At the basic level, ANOVA Solution? assess whether the means of several different levels are ANOVA! significantly different from one another ANOVA can make these multiple comparisons whilst controlling for the familywise error rate (i.e. no need for manual adjustments) Today, we are considering One-Way ANOVA One IV (with 3+ levels), One DV e.g. IV = stress, levels (3) = high, medium, low Different e.g. IV = experimental drug, levels (4) = placebo, low dose, medium dose, high dose ANOVA e.g. IV = individual difference characteristic, levels (3) = high, medium, low ANOVA is the analysis (design is still either a between- or within-subjects experiment) and can be either: one-way independent measures ANOVA (for between-subjects) Or, a one-way repeated measures ANOVA (for within-subjects) Different Examples: Between-Subjects Within-Subjects Looking to assess whether A related topic within dark tourism is kenopsia – the eerie feeling we get psychopathy is linked to being in empty/abandoned places that engagement with dark tourism should be bustling with life - but are all Score Pp on the PCL-R (0-40) and empty places the same? We take our Pp to Pripyat (in Ukraine), then group into low (0-15), Tyneham (in Dorset), and the medium (16-24), and high (25- University late at night 40) (counterbalanced) Rate how much they’d like to Pp walk around each place for 1hr, rating how eerie they feel (1-10) every visit dark sites (generally) on a 20mins (taking an average of the three scale of 1-10 readings) Data is normally distributed Shapiro-Wilk test Variance should be similar across each level For independent measures: ANOVA homogeneity of variance (assessed using Levene’s test) For repeated measures: sphericity Assumptio (assessed using Mauchly’s test) ANOVA is robust, but if non-parametric ns we would use the Kruskal-Wallis test This is usually reserved for studies with very uneven levels Remember, assumption checks are just that! These should not constitute a large focus of a report Shapiro-Wilk Test Used to check assumption of normality Can also check histograms and measures of central tendency Plots a normal distribution over our distribution, and assesses the percentage of overlap A significant result indicates our distribution is significantly different from a normal distribution (i.e. not normal) Levene’s Test Homogeneity of variance refers to the idea that the distribution of each level is roughly similar and therefore comparable Used for 2+ levels If we violate this assumption, we can come to incorrect comparisons Need to adjust our df if violated Mauchly’s Test A similar concept to homogeneity of variance; but used for within-subjects, and only when there are 3+ levels Assesses whether the variance between two of the three levels, for example, is similar to the variance between two other levels (i.e. the variance between group A and group B should be similar to the variance between group A and group C, and group B and group C ANOVA: Behind the Curtain Whilst we can conceptualise an ANOVA as multiple t-tests, just assessing whether the means of different levels are statistically different… An ANOVA is actually assessing how much variance (i.e. change) in the DV can be attributed to the IV (versus how much can be attributed to error/noise) A significant result (p <.05) indicates that your IV has an effect on the DV A non-significant result (p >.05) indicates that your IV does not have an effect on the DV The Maths – One-way independent groups Between Groups (due to IV) Total Variance Within groups (random error) We want to ratio these to get an F-statistic – both must first be standardised (divide by their own df This gives you a mean square for each, which we then ratio to get our F-statistic If the null hypothesis is true, F will be around 1 If the null hypothesis is false, F will be greater than 1 Independent ANOVA The Maths – One-way repeated measures Total Sums of You have the same participants in each Squares group so it is not as meaningful to examine the variance within each group compared to the variance between each group Systematic Unsystematic variance i.e. variance i.e. What we must examine instead is how model sums of residual sums much participants’ scores vary from one squares of squares condition to another, i.e. model sums of squares If there is an effect of the IV, we should see a relatively larger change across our levels Divide each by associated df = mean We take into consideration error, boredom, square fatigue etc., i.e. residual sums of squares Model Mean Square Ultimately, this analysis is the same as the Residual Mean Square previous one; just with different labels Repeated ANOVA You can actually do ANOVAs by hand, but we won’t make you! Today is all treats, no tricks! JASP or SPSS (you’ll have guides for both Importa on Canvas) will do the analysis for you Your main focus should be conducting, reporting, and interpreting the nt to appropriate ANOVA correctly– an example APA write-up is included in the guides Note But remember, if an ANOVA is significant, that just means there’s an effect of your IV on the DV – we don’t know where any differences actually are (ANOVA are omnibus test) We would need to run another test to find this out! A Post-Hoc test (Latin for And that other test ‘after this’ or ‘after the event’) is conducted after is… we’ve found a significant result (and we have three or more levels) If the test is non- significant, we don’t need to ‘cos there’s nothing to report! If the test is significant, but we only have two levels, we can just compare the means of these two levels We could just look at the graph and make assumptions about where the significant difference is I don’t need to tell you that that’s bad practice! Post-Hoc Tests Compares each level of the IV against each other, whilst controlling for the familywise error rate i.e. we can make multiple comparisons without increasing our chances of making a Type I error There are many different kinds of post-hoc tests (there is a guide on this week’s Canvas page), but typically: Tukey: between-subjects Bonferroni Pairwise Comparisons: within-subjects We cannot assume that just because the ANOVA was significant, the differences between each level will also be significant – need to investigate all comparisons! Let’s go back to our examples! Post Hoc Tests from an Independent ANOVA Post Hoc Tests from a Repeated ANOVA Reporting an Independent ANOVA Each results section would start by restating the aim, detailing the data wrangling process, justifying use of your specific analysis, and then reporting the inferential analysis There was a significant effect of psychopathy on dark tourism preference, F (2, 27) = 13.40, p <.001, ω2 =.45. Tukey post-hoc tests indicated that people rated dark tourist sites as increasingly preferable as their levels of psychopathy decreased (p ≤.05 in each case). Reporting a Repeated ANOVA There was a significant effect of empty site on sense of eeriness, F (2, 38) = 241.80, p <.001, ω2 =.85. Bonferroni post-hoc tests indicated that people rated both Pripyat (p <.001) and Tyneham (p <.001) as being eerier than the empty University. However, the two did not differ from each other (p >.05). In the rest of the session: Use the guides on Canvas to practice conducting, reporting, and interpreting both an independent and repeated ANOVA There are practice datasets for each of these tests If you get finished, there is a workbook with additional questions/data for your practice Remember, the more you practice with these, the more comfortable you’ll become Next Week: Expand further into experimental designs as we look at adding a second IV Again, we’ll practice conducting, reporting, and interpreting this type of analysis This is what we’ll need for our report in the months ahead!