Unit 7: Experiments PDF
Document Details
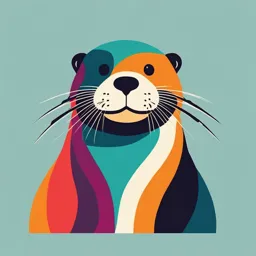
Uploaded by AwesomeCarnelian4810
Copenhagen Business School, Vienna University of Economics and Business
Martin / Zulehner
Tags
Summary
This document describes various scenarios in experiments, including motivation, vaccine effectiveness, and different types of experiments. It also discusses potential outcomes, causal effects, and ideal experiments, as well as threats to the validity of experiments and examples of specific experiments such as Project STAR.
Full Transcript
Unit 7: Experiments Martin / Zulehner: Introductory Econometrics 1 / 37 Motivation Suppose there is a new disease or virus... which triggers a necessity for new vaccines Are these new vaccines effective? For example... Martin / Zulehner: Introductory Econometri...
Unit 7: Experiments Martin / Zulehner: Introductory Econometrics 1 / 37 Motivation Suppose there is a new disease or virus... which triggers a necessity for new vaccines Are these new vaccines effective? For example... Martin / Zulehner: Introductory Econometrics 2 / 37 Motivation Martin / Zulehner: Introductory Econometrics 3 / 37 Vaccine effectiveness What does “effective” even mean? Suppose for each individual i, we obtain a continuous health measure yi (higher values = better, healthier).. and a treatment indicator xi ∈ {0, 1}, e.g., whether they received the vaccine or not Effectiveness: E (y |x = 1) > E (y |x = 0) How can we measure this? Martin / Zulehner: Introductory Econometrics 4 / 37 Scenario 1: Everyone gets the vaccine Can we learn anything from this? No! We get E (y |x = 1) ≈ 1.05, but we don’t get an estimate for E (y |x = 0) Which of the Gauss-Markov assumptions is violated? Martin / Zulehner: Introductory Econometrics 5 / 37 Scenario 1: Everyone gets the vaccine Can we learn anything from this? No! We get E (y |x = 1) ≈ 1.05, but we don’t get an estimate for E (y |x = 0) Which of the Gauss-Markov assumptions is violated? SLR.3 Sample variation (Var (x ) ̸= 0), or more generally MLR.3 No perfect collinearity Martin / Zulehner: Introductory Econometrics 5 / 37 Scenario 2: People choose to take the vaccine Suppose only people who are really sick choose to take the vaccine We certainly have variation in x We now get E (y |x = 1) ≈ −0.85 and E (y |x = 0) ≈ −0.04, so it seems better NOT to take the vaccine Martin / Zulehner: Introductory Econometrics 6 / 37 Scenario 2: People choose to take the vaccine Which of the Gauss-Markov assumptions is violated? Martin / Zulehner: Introductory Econometrics 7 / 37 Scenario 2: People choose to take the vaccine Which of the Gauss-Markov assumptions is violated? SLR.2 / MLR.2 Random sampling What can be done? Randomize assignment in an experiment! Martin / Zulehner: Introductory Econometrics 7 / 37 Scenario 3: Random assignment (experiment) Half of individuals get the vaccine, the other half get a placebo with random assignment We certainly have variation in x We now get E (y |x = 1) ≈ 1.05 and E (y |x = 0) ≈ −0.04, so it is better to take the vaccine Martin / Zulehner: Introductory Econometrics 8 / 37 Scenario 3: Random assignment (experiment) Martin / Zulehner: Introductory Econometrics 9 / 37 Scenario 3: Random assignment (experiment) Martin / Zulehner: Introductory Econometrics 10 / 37 Outline 1 Potential Outcomes, Causal Effects, and Idealized Experiments 2 Threats to Validity of Experiments 3 Application: The Tennessee STAR Experiment Martin / Zulehner: Introductory Econometrics 11 / 37 Why study experiments? Ideal randomized controlled experiments provide a conceptual benchmark for assessing observational studies. Actual experiments are rare (as costly) but influential. Experiments can overcome the threats to internal validity of observational studies, however they have their own threats to internal and external validity. Thinking about experiments helps us to understand quasi-experiments, or “natural experiments,” in “natural” variation induces “as if” random assignment. Martin / Zulehner: Introductory Econometrics 12 / 37 Terminology: experiments and quasi-experiments An experiment is designed and implemented consciously by human researchers. An experiment randomly assigns subjects to treatment and control groups (think of clinical drug trials) A quasi-experiment or natural experiment has a source of randomization that is “as if” randomly assigned, but this variation was not the result of an explicit randomized treatment and control design. Program evaluation is the field of statistics aimed at evaluating the effect of a program or policy, for example, an ad campaign to cut smoking, or a job training program. Martin / Zulehner: Introductory Econometrics 13 / 37 Different Types of Experiments: Three Examples Clinical drug trial: does a proposed drug lower cholesterol? ▶ Y = cholesterol level ▶ X = treatment or control group (or dose of drug) Job training program (Job Training Partnership Act) ▶ Y = has a job, or not (or Y = wage income) ▶ X = went through experimental program, or not Class size effect (Tennessee class size experiment) ▶ Y = test score (Stanford Achievement Test) ▶ X = class size treatment group (regular, regular + aide, small) Martin / Zulehner: Introductory Econometrics 14 / 37 Potential Outcomes, Causal Effects, and Idealized Experiments A treatment has a causal effect for a given individual: give the individual the treatment and something happens, which is (possibly) different than what happens if you don’t get the treatment. A potential outcome is the outcome for an individual under a potential treatment or potential non-treatment. For an individual, the causal effect is the difference in potential outcomes if you do or don’t get the treatment. An individual’s causal effect cannot be observed because you can give the subject the treatment, or not - but not both! Martin / Zulehner: Introductory Econometrics 15 / 37 From potential outcomes to regression Consider subject i drawn at random from a population and let: Xi = 1 if subject i treated, 0 if not (binary treatment) Yi (0) = potential outcome for subject i if untreated Yi (1) = potential outcome for subject i if treated We observe (Yi , Xi ), where Yi is the observed outcome: Yi = Yi (1)Xi + Yi (0) (1 − Xi ) = Yi (0) + [Yi (1) − Yi (0)] Xi = E [Yi (0)] + [Yi (1) − Yi (0)] Xi + [Yi (0) − E (Yi (0))] where the expectation is over the population distribution. Martin / Zulehner: Introductory Econometrics 16 / 37 From potential outcomes to regression Thus Yi = E [Yi (0)] + [Yi (1) − Yi (0)] Xi + [Yi (0) − E (Yi (0))] = β0 + β1i Xi + ui where β0 = E [Yi (0)] β1i = Yi (1) − Yi (0) = individual i’s causal effect ui = Yi (0) − E (Yi (0)) , so Eui = 0. The regression model with heterogeneous treatment effects each person has his or her own treatment effect, is: Yi = β0 + β1i Xi + ui where β1i is individual i’s causal effect (“treatment effect”). Martin / Zulehner: Introductory Econometrics 17 / 37 Average Treatment Effect Heterogeneous treatment effect regression model: Yi = β0 + β1i Xi + ui where β1i is individual i’s causal effect ("treatment effect"). In general, different people have different treatment effects. For people drawn from a population, the average treatment effect is the population mean value of the individual treatment effects: Average Treatment Effect = ATE = E (β1i ) In many applications, the object of interest is the ATE (the average effect in the population of interest). For now we suppose there is no heterogeneity in treatment effects, so that all individuals have the same treatment effect β1. We return to heterogeneous treatment effects later Martin / Zulehner: Introductory Econometrics 18 / 37 Estimating the treatment effect in an ideal randomized controlled experiment An ideal randomized controlled experiment randomly assigns subjects to treatment and control groups. Let X be the treatment variable and Y the outcome variable of interest. If X is randomly assigned (for example by computer) then X is independent of all individual characteristics. Let the (homogeneous) treatment effect be β1 : Yi = β0 + β1 Xi + ui If Xi is randomly assigned, then Xi is independent of ui , so E (ui | Xi ) = 0, and OLS yields an unbiased estimator of β1. The causal effect is the population value of β1 in an ideal randomized controlled experiment Yi = β0 + β1 Xi + ui When the treatment is binary, β̂1 is just the difference in mean outcome (Y ) in the treatment vs. control group Ȳ treated − Ȳ control. This difference in means is sometimes called the differences estimator. Martin / Zulehner: Introductory Econometrics 19 / 37 Additional regressors Let Xi = treatment variable and Wi = control variable(s). Yi = β0 + β1 Xi + β2 Wi + ui Two reasons to include W in a regression analysis of the effect of a randomly assigned treatment: 1 If Xi is randomly assigned then Xi is uncorrelated with Wi so omitting Wi doesn’t result in omitted variable bias. But including Wi reduces the error variance and can result in smaller standard errors. 2 If the probability of assignment depends on Wi , so that Xi is randomly assigned given Wi , then omitting Wi can lead to to OV bias, but including it eliminates that OV bias. This situation is called “Randomization based on covariates”. Martin / Zulehner: Introductory Econometrics 20 / 37 Randomization based on covariates Example: men (Wi = 0) and women (Wi = 1) are randomly assigned to a course on table manners (Xi ), but women are assigned with a higher probability than men. Suppose women have better table manners than men prior to the course. Then even if the course has no effect, the treatment group will have better post-course table manners than the control group because the treatment group has a higher fraction of women than the control group. That is, the OLS estimator of β1 in the regression, Yi = β0 + β1 Xi + ui has omitted variable bias, which is eliminated by the regression, Yi = β0 + β1 Xi + β2 Wi + ui Martin / Zulehner: Introductory Econometrics 21 / 37 Randomization based on covariates In this example, Xi is randomly assigned, given Wi , so E (ui | Xi , Wi ) = E (ui | Wi ) In words, among women, treatment is randomly assigned, so among women, the error term is independent of Xi so, among women, its mean doesn’t depend on Xi. Same is true among men. Thus if randomization is based on covariates, conditional mean independence holds, so that once Wi. is included in the regression the OLS estimator is unbiased. Martin / Zulehner: Introductory Econometrics 22 / 37 Estimating causal effects that depend on observables The causal effect in the previous example might depend on observables, perhaps β1, men > β1, women (men could benefit more from the table manners course than women). We already know how to estimate different coefficients for different groups use interactions. In the table manners example, we would simply estimate the interactions model, Yi = β0 + β1 Xi + β2 Xi × Wi + β3 Wi + ui when Wi is 1 for women and 0 for men, β1 gives β1, men and β1 + β2 gives β1, women We return to differences in β1i ’s (unobserved heterogeneity - in contrast to heterogeneity that depends on observable variables like sex) in unit 18. Martin / Zulehner: Introductory Econometrics 23 / 37 Threats to Validity of Experiments Threats to Internal Validity 1 Failure to randomize (or imperfect randomization) ▶ for example, openings in job treatment program are filled on first-come, first-serve basis; latecomers are controls ▶ result is correlation between X and u 2 Failure to follow treatment protocol (or “partial compliance”) ▶ some controls get the treatment ▶ some of those who should be treated aren’t ▶ If you observe whether the subject actually receives treatment (X ), if you know whether the individual was initially assigned to a treatment group (Z ), and if initial assignment was random, then you can estimate the causal effect using initial assignment as an instrument for actual treatment. Martin / Zulehner: Introductory Econometrics 24 / 37 Threats to Validity of Experiments 3 Attrition (some subjects drop out) ▶ Suppose the controls who get jobs move out of town; then corr(X , u) ̸= 0 ▶ this may lead to a sample selection bias: the sample is selected in a way related to the outcome variable (see unit 16) 4 Experimantal effects ▶ experimenter bias (conscious or subconscious): treatment X is associated with “extra effort” or “extra care,” so corr(X , u) ̸= 0 ▶ subject behavior might be affected by being in an experiment, so corr(X , u) ̸= 0 (Hawthorne effect) Just as in regression analysis with observational data, threats to the internal validity of regression with experimental data implies that corr(X , u) ̸= 0 so OLS (the differences estimator) is biased. In an experiment, this can sometimes be mitigated by using a “double blind” protocol in which neither the experimenter or the subject knows who is in the treatment or control groups. Martin / Zulehner: Introductory Econometrics 25 / 37 Threats to Validity of Experiments Threats to External Validity 1 Nonrepresentative sample 2 Nonrepresentative “treatment” (that is, program or policy) 3 General equilibrium effects (effect of a program can depend on its scale; admissions counseling) Martin / Zulehner: Introductory Econometrics 26 / 37 Project STAR (Student-Teacher Achievement Ratio) 4-year study, $12 million Upon entering the school system, a student was randomly assigned to one of three groups: ▶ regular class (22 − 25 students ) ▶ regular class + aide ▶ small class (13-17 students) regular class students re-randomized after first year to regular or regular + aide Y = Stanford Achievement Test scores Martin / Zulehner: Introductory Econometrics 27 / 37 Deviations from experimental design Partial compliance: ▶ 10% of students switched treatment groups because of “incompatibility” and “behavior problems” - how much of this was because of parental pressure? ▶ Newcomers: incomplete receipt of treatment for those who move into district after grade 1 Attrition ▶ students move out of district ▶ students leave for private/religious schools ▶ This is only a problem if their departure is related to Yi ; for example if high-achieving kids leave because they are assigned to a large class, then large classes will spuriously appear to do relatively worse (corr (ui , Xi ) > 0) Martin / Zulehner: Introductory Econometrics 28 / 37 Regression analysis The “differences” regression model: Yi = β0 + β1 SmallClassi + β2 RegAidei + ui where ▶ SmallClassi if in a small class ▶ RegAidei = 1 if in regular class with aide Additional regressors (W’s) ▶ teacher experience ▶ free lunch eligibility ▶ gender, race Martin / Zulehner: Introductory Econometrics 29 / 37 Regression results Project STAR: Differences Estimates of Effect on Standardized Test Scores of Class Size Treatment Group Grade Regressor K 1 2 3 Small class 13.90 29.78 19.39 15.59 (4.23) (4.79) (5.12) (4.21) [5.48, 22.32] [20.24, 39.32] [9.18, 29.61] [7.21, 23.97] Regular-sized class with aide 0.31 11.96 3.48 -0.29 (3.77) (4.87) (4.91) (4.04) [−7.19, 7.82] [2.27, 21.65] [−6.31, 13.27] [−8.35, 7.77] Intercept 918.04 1039.39 1157.81 1228.51 (4.82) (5.82) (5.29) (4.66) Number of observations 5786 6379 6049 5967 The regressions were estimated using the Project STAR public access data set described in Appendix 13.1 of Stock and Watson. The dependent variable is the student’s combined score on the math and reading portions of the Stanford Achievement Test. Standard errors, clustered at the school level, appear in parentheses, and 95% confidence intervals appear in brackets. Martin / Zulehner: Introductory Econometrics 30 / 37 Regression results Differences Estimates with Additional Regressors for Kindergarten Regressor (1) (2) (3) (4) Small class 13.90 14.00 15.93 15.93 (4.23) (4.25) (4.08) (3.95) [5.48,22.32] [5.55,22.46] [7.81,24.06] [8.03,23.74] Regular-sized class with aide 0.31 -0.60 1.22 1.79 (3.77) (3.84) (3.64) (3.60) [-7.19,7.82] [-8.25,7.05] [-6.04,8.47] [-5.38,8.95] Teacher’s years of experience 1.47 0.74 0.66 (0.44) (0.35) (0.36) [0.60,2.34] [0.04,1.45] [-0.05,1.37] Additional controls? no no no yes School indicator variables? no no yes yes R̄ 2 0.01 0.02 0.22 0.28 Number of observations 5786 5766 5766 5748 The regressions were estimated using the Project STAR public access data set described in Appendix 13.1. The dependent variable is the student’s combined test score on the math and reading portions of the Stanford Achievement Test. All regressions include an intercept (not reported). The number of observations differs in the different regressions because of some missing data. Standard errors, clustered at the school level, appear in parentheses, and 95% confidence intervals appear in brackets. Martin / Zulehner: Introductory Econometrics 31 / 37 How big are these estimated effects? Estimated Class Size Effects in Units of Standard Deviations of the Test Score Across Students Put on same basis by dividing by std. dev. of Y Units are now standard deviations of test scores Grade Treatment Group K 1 2 3 Small class 0.19 0.33 0.23 0.21 (0.06) (0.05) (0.06) (0.06) Regular-sized class with aide 0.00 0.13 0.04 0.00 (0.05) (0.05) (0.06) (0.06) Sample standard deviation 73.75 91.25 84.08 73.27 of test scores (sY ) The estimates and standard errors in the first two rows are the estimated effects in Table 13.1, divided by the sample standard deviation of the Stanford Achievement Test for that grade (the final row in this table), computed using data on the students in the experiment. Standard errors, clustered at the school level appear in parentheses. Martin / Zulehner: Introductory Econometrics 32 / 37 How do these estimates compare to those from the California & Mass. observational studies? Estimated Effects of Reducing the Student-Teacher Ratio by 7.5 Based on the STAR Data and the California and Massachusetts Observational Data Change in Std. Dev. Est. 95% Conf.I. Study B̂ 1 Student - Teacher Test Scores Effect Ratio -13.90 Small class vs. 0.19 STAR (grade K) 73.8 (0.13, 0.25) (2.45) regular class (0.03) -0.73 0.14 California -7.5 38.0 (0.04, 0.24) (0.26) (0.05) -0.64 0.12 Massachusetts -7.5 39.0 (0.02, 0.22) (0.27) (0.05) Note: The estimate coefficient β̂1 or the STAR study is taken from column (1) of Table 13.2. The estimated coefficients for the California and Massachusetts studies are taken from the first column of Table 9.3. The estimated effect is the effect of being in a small class versus a regular class (for STAR) or the effect of reducing the student-teacher ratio by 7.5 (for the California and Massachusetts studies). The 95% confidence interval for the reduction in the student- teacher ratio is this estimated effect ± 1.96 standard errors. Standard errors are given in parentheses under estimated effects. The estimated effects are statistically significantly different from zero at the ∗ 5% significance level or ∗∗ 1% significance level using two-sided test. Martin / Zulehner: Introductory Econometrics 33 / 37 More on the design of Project STAR More on the design of Project STAR Teachers were randomly assigned to small/regular/reg+aide classrooms within their normal school - teachers didn’t change schools as part of the experiment. Because teacher experience differed systematically across schools (more experienced teachers in more affluent school districts), a regression of test scores on teacher experience would have omitted variable bias and the estimated effect on test scores of teacher experience would be biased up (overstated). → school fixed effects Martin / Zulehner: Introductory Econometrics 34 / 37 Effect of teacher experience Without school fixed effects (2), the estimated effect of an additional year of experience is 1.47 (SE=.17) “Controlling for the school” in (3), the estimated effect of an additional year of experience is.74 (SE =.17) The OLS estimator of the coefficient on years of experience is biased up without school effects; with school effects, OLS is an unbiased estimator of the causal effect of X. Martin / Zulehner: Introductory Econometrics 35 / 37 Summary: The Tennessee Class Size Experiment Remaining threats to internal validity partial compliance/incomplete treatment can use TSLS with Z = initial assignment Turns out, TSLS and OLS estimates are similar (Krueger (1999)), so this bias seems not to be large Main findings: The effects are small quantitatively (same size as gender difference) Effect is sustained but not cumulative or increasing (biggest effect at the youngest grades) Martin / Zulehner: Introductory Econometrics 36 / 37 Summary: Experiments Ideal experiments and potential outcomes The average treatment effect is the population mean of the individual treatment effect, which is the difference in potential outcomes when treated and not treated. The treatment effect estimated in an ideal randomized controlled experiment is unbiased for the average treatment effect. Actual experiments Actual experiments have threats to internal validity Depending on the threat, these threats to internal validity can be addressed by: ▶ panel data regression (differences-in-differences) ▶ multiple regression (including control variables), and ▶ IV (using initial assignment as an instrument, possibly with control variables) External validity also can be an important threat to the validity of experiments Martin / Zulehner: Introductory Econometrics 37 / 37