Causal Analysis Notes PDF - University of Bern
Document Details
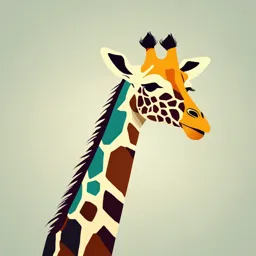
Uploaded by AppreciatedUranium
University of Bern
2024
Michael Gerfin,Blaise Melly
Tags
Summary
This document is a set of lecture notes on Causal Analysis from the University of Bern, Spring 2024. The notes cover topics like the definition of causality, counterfactuals, potential outcomes, and related concepts in economics.
Full Transcript
Causal Analysis The Definition of Causality: Counterfactuals and Potential Outcomes Michael Gerfin Blaise Melly University of Bern Spring 2024 Course information Instructors: Blaise Melly ([email protected]) Michael Gerfin ([email protected]) Lectures with computer examples (no exercise se...
Causal Analysis The Definition of Causality: Counterfactuals and Potential Outcomes Michael Gerfin Blaise Melly University of Bern Spring 2024 Course information Instructors: Blaise Melly ([email protected]) Michael Gerfin ([email protected]) Lectures with computer examples (no exercise sessions) Readings 1 Angrist and Pischke (2009) Mostly Harmless Econometrics: An Empiricist’s Companion, Princeton University Press (MHE) 2 Wooldridge, J.M. (2010) Econometric Analysis of Cross Section and Panel Data, 2nd edition, MIT Press. Angrist and Pischke (2014) Mastering ’Metrics: The Path from Cause to Effect, Princeton University Press (MM) Data/programs: http://economics.mit.edu/faculty/angrist/data1/mhe 3 Data/programs: http://masteringmetrics.com/resources/ Course materials available on ILIAS Software package: Stata (v18, or earlier) Exam: June 3 (first date); September 11 (second date) 2 / 48 Correlation is Not Causation 3 / 48 Outline 1 The Definition of Causality: Counterfactuals and Potential Outcomes 2 Randomized experiments 3 Regression and Causality 4 Matching 5 Instrumental variables 6 Difference in differences 7 Regression discontinuity 8 Quantile Regression 4 / 48 Contents of this chapter 1. Introduction 2. Research FAQs 3. Counterfactuals and Potential Outcomes 4. Directed Acyclic Graphs (DAG) 5 / 48 Introduction Nobel Price in Economics 2021 Awarded to Joshua Angrist (MIT, Cambridge) and Guido Imbens (Stanford University) for their methodological contributions to the analysis of causal relationships and to David Card (Berkeley University) for his empirical contributions to labour economics This year’s Laureates have provided new insights about the labor market and have shown what conclusions about cause and effect can be drawn from natural experiments. Their approach has spread to other fields and revolutionized empirical research. 6 / 48 Introduction Importance of Causal Analysis in Economics Curry et al. (2020), Technology and Big Data Are Changing Economics:Mining Text to Track Methods, AEA Papers & Proceedings 7 / 48 Introduction Importance of Causal Analysis in Economics Curry et al. (2020), Technology and Big Data Are Changing Economics:Mining Text to Track Methods, AEA Papers & Proceedings 8 / 48 Introduction Importance of Causal Analysis in Economics Curry et al. (2020), Technology and Big Data Are Changing Economics:Mining Text to Track Methods, AEA Papers & Proceedings 9 / 48 Introduction Importance of Causal Analysis in Economics Curry et al. (2020), Technology and Big Data Are Changing Economics:Mining Text to Track Methods, AEA Papers & Proceedings 10 / 48 Research FAQs 1. Introduction 2. Research FAQs 3. Counterfactuals and Potential Outcomes 4. Directed Acyclic Graphs (DAG) 11 / 48 Research FAQs Research FAQ (1) FAQ (1) What is the causal relationship of interest? Class size on test scores Training on employment probability Health insurance on health care demand solution in randomized selection overreaching Schooling on wages Number of children on female labour supply Mask wearing on infection rates Needed for predictions about consequences of changing policies, or hypothetical thought experiments “What would happen if...” possible randomized selection in the groups that accomplished sth - selection bias 12 / 48 Research FAQs Research FAQ (2) which randomized trial could help to answer FAQ (2) to the question? Which experiment could ideally be used to capture the causal effect of interest? Ideal experiments are often hypothetical But thinking about them can be very useful If you cannot think of an experiment in a no-restrictions world that answers your question you will probably never be able to answer it The description of an ideal experiment will help you to formulate the causal question precisely design to really run an experiment Research questions that cannot be identified by an experiment are fundamentally unidentified questions 13 / 48 Research FAQs Research FAQ (3) compliance is an issue in experiments FAQ (3) ensure that the number you get represents a causal effect and NOT a correlation What is your identification strategy? Identification strategy: the manner in which a researcher uses observational data (i.e., data not generated by a randomized experiment) to approximate a real experiment First part of a more technical, econometric toolbox regression is continuity 14 / 48 Research FAQs Research FAQ (4) FAQ (4) What is your mode of statistical inference? The answer to this question depends on The population to be studied The sample to be used The assumptions imposed to compute standard errors Second part of the more technical, econometric toolbox 15 / 48 Counterfactuals and Potential Outcomes 1. Introduction 2. Research FAQs 3. Counterfactuals and Potential Outcomes 4. Directed Acyclic Graphs (DAG) 16 / 48 Counterfactuals and Potential Outcomes Only one road can be taken causal effect is essentially the comparison between turning left and turning right Y0 direction you did not choose, counterfactual Y1 direction you went, factual learn something about the outcome you do not observe from 17 / 48 what you observe, interpelate what you do not observe Counterfactuals and Potential Outcomes Selection problem Example: Do hospitals make people healthier? Group Hospital No hospital Sample Size Mean Health Std. Error 7’774 90’049 3.21 3.93 0.014 0.003 variable that has an impact n both going to the hospital and staying healthy Mean health is computed using answer to question “Would you say your health in general is excellent (5), very good (4), good (3), fair (2) or poor (1)?” then we average the answers into two groups then we conclude by looking at the hospital 18 / 48 Counterfactuals and Potential Outcomes Potential outcomes framework Set the problem in the potential outcomes framework Denote hospital treatment by Di = {0, 1} The outcome of interest (health status in this case) is denoted by Yi Causal question: how is Yi affected by hospital care? Assume we can imagine what might have happened to someone who went to hospital if he/she had not gone to hospital, and vice versa (the counterfactual) For each individual there are two potential health outcomes: Y1i if Di = 1, and Y0i if Di = 0 D_1: Y=Y_1, Y_0? we estimate y_0 and D_0: Y=Y_0, Y_1? y_1 respectively, n using the data we o have not observed t Individual causal effect: Y1i − Y0i if only for one person, i could do everything obekse s constant by definition =) causal effect e 19 / 48 Counterfactuals and Potential Outcomes Hypothetical example Assume that it is possible to observe all potential outcomes Two persons, Khuzdar and Maria, decide on whether to go the hospital Khuzdar has only average health without hospital treatment Maria’s health is robust, so she has very good health even without hospital treatment Khuzdar Potential outcome without hospital Y0i 3 Potential outcome with hospital Y1iH improves 4 seen in data Treatment Di : hospital treatment 1 seen in data Actual outcome Y 4 i Causal effect Y1i − Y0i 1 not seen not seen Maria perfectly 5 healthy 5 hospital cannot 0 make her 5 healthy 0 20 / 48 Counterfactuals and Potential Outcomes Hypothetical example naive suggestion The comparison of the actual (observable) outcomes is 4 − 5 = −1 suggesting a negative effect of hospital treatment Using this decomposition illustrates the mistake YK − YM = Y1K − Y0M = Y1K − Y0K + (Y0K − Y0M ) | {z } | {z } 1 −2 The first term is the causal effect, the second term is the selection effect because Khuzdar has lower health than Maria he selects into treatment because he expects to profit from it put differently, the selection effect of -2 reflects that Khuzdar is less healthy without treatment than Maria 21 / 48 Counterfactuals and Potential Outcomes Average treatment effects Y_i=Y_0+(Y_1i-Y_0i) D_i + U_i regressing Y and D could give me the term T The observed outcome can be written as what I see baseline Yi = Y1i · Di + Y0i · (1 − Di ) = Y0i + (Y1i − Y0i ) · Di (1) Cannot observe anybody in both potential outcomes and hence cannot identify the individual causal effect Y1i − Y0i Sometimes we can identify moments (mostly the expectation) of the random variable Y1i − Y0i but if someone has the treatment effect, it Average Treatment Effect: AT E = E[Y1i ] − E[Y0i ] still never sees Y_0 Conditional on D = 1 we get the Average Treatment Effect on the Treated: AT T = E[Y1i |Di = 1] − E[Y0i |Di = 1]) term vries with i due to index with i observational problem very easily...counterfactual 22 / 48 Counterfactuals and Potential Outcomes Hypothetical example The comparison of the actual (observable) outcomes is 4 − 5 = −1 suggesting a negative effect of hospital treatment Using this decomposition illustrates the mistake translate what we see into potential outcomes we start with what we see in the data comparing two different people Y0K + (Y0K − Y0M ) in two different states YK − YM = Y1K − Y0M = Y1K − | {z } | {z } 1 −2underlying differences...that is the observational data allows comparisons of people with problem The first term is the causal effect, the second term is the selection effect Y_1 Kustak - Y_0 Kustak, causal effect because Khuzdar has lower health than Maria he selects into treatment because he expects to profit from it put differently, the selection effect of -2 reflects that Khuzdar is less healthy without treatment than Maria 23 / 48 Counterfactuals and Potential Outcomes What we want and what we get Consider the “naive” comparison E[Yi |Di = 1] − E[Yi |Di = 0] = E[Y1i |Di = 1] − E[Y0i |Di = 1] {z } | {z } | AT T observed difference translate into potential outcomes E[Y|D=1] - E[Y|D=0] if D is 1, I see Y1 + E[Y0i |Di = 1] − E[Y0i |Di = 0] | {z } If D=0, I see Y0 selection bias we need to make sure that it goes away This is not what we want, unless the selection bias is zero Random assignment makes the selection bias zero then we have a well defined average potential impact 24 / 48 Counterfactuals and Potential Outcomes Hypothetical example The comparison of the actual (observable) outcomes is 4 − 5 = −1 suggesting a negative effect of hospital treatment Using this decomposition illustrates the mistake YK − YM = Y1K − Y0M = Y1K − Y0K + (Y0K − Y0M ) | {z } | {z } 1 −2 The first term is the causal effect, the second term is the selection effect because Khuzdar has lower health than Maria he selects into treatment because he expects to profit from it put differently, the selection effect of -2 reflects that Khuzdar is less healthy without treatment than Maria 25 / 48 Directed Acyclic Graphs (DAG) 1. Introduction 2. Research FAQs 3. Counterfactuals and Potential Outcomes 4. Directed Acyclic Graphs (DAG) 26 / 48 Directed Acyclic Graphs (DAG) Directed Acyclic Graphs (DAG) Causal graphs have been introduced to causal analysis by Judea Pearl A DAG is a tool to illustrate and analyze causal models It is especially useful for demonstrating identification problems and identification strategies Main elements nodes: variables (outcome, treatment, observed and unobserved factors) arrows: possible direct causal effects the arrows order the variables in time acyclic: no simultaneity, the future does not cause the past missing arrow: sharp assumption about absent direct causal effect D-> Y: show a causal effect. If we start treatment D, we cANNOT come back to Y via D, No arrow, I assume that there is no correlation going on. 27 / 48 Directed Acyclic Graphs (DAG) Directed Acyclic Graphs (DAG) Path: an acyclic sequence of adjacent nodes causal paths: all arrows pointing away from cause D and into outcome Y D → Y (direct causal path) and D → X → Y (indirect causal path) non-causal paths: path from D to Y contains at least one arrow against causal order (←) Important: information can flow along the non-causal path (non-causal correlations) As long as there are open non-causal paths the causal effect is not identified Question: how do we close open non-causal paths? 28 / 48 Directed Acyclic Graphs (DAG) controlling means I am holding it fixed controlling with NO computer but SOME data: compare the outcomes for people with the same age 1. Example age hospital confounders controlling for age can help to close the backdoor paths and turn a correlation into a causal effect health measure strength only causal path Outcome Y , treatment D, confounders X1 , X2 Goal: identify causal effect of D on Y : D → Y (in green) But there are other non-causal paths confounding the causal path: D ← X1 → Y and D ← X2 → Y These paths are also called back-door paths The association between D and Y is non-causal as long as there are open back door paths 29 / 48 Directed Acyclic Graphs (DAG) Connection of DAG with statistical models A DAG represent an underlying Structural Causal Model (SCM) In example 1 the SCM is X1 = f1 (u1 ) X2 = f2 (u2 ) function of an error term, nothing causing x1 but u1 (the error term) D = f3 (X1 , X2 , u3 ) treatment if a function of x1, x2 and u3 Y = f4 (D, X1 , X2 , u4 ) where fi (·) are non-parametric functions, and ui denotes unobserved independent random factors influencing the variables (error terms) The error terms are typically not shown in a DAG 30 / 48 Directed Acyclic Graphs (DAG) Directed Acyclic Graphs (DAG) Path: an acyclic sequence of adjacent nodes causal paths: all arrows pointing away from cause D and into outcome Y D → Y (direct causal path) and D → X → Y (indirect causal path) non-causal paths: path from D to Y contains at least one arrow against causal order (←) Important: information can flow along the non-causal path (non-causal correlations) As long as there are open non-causal paths the causal effect is not identified Question: how do we close open non-causal paths? 31 / 48 Directed Acyclic Graphs (DAG) 2. Example - direct - indrect now we have 2 causal paths Outcome Y , treatment D, observable factors X1 and X2 Goal: identify causal effect of D on Y (all causal paths from D to Y ): D → Y (direct) and D → X1 → Y (indirect) X1 is a mediator, a factor that is influenced by the treatment and has a causal effect on Y Back-door path: D ← X2 → Y The association between D and Y is non-causal as long as the back-door path is open 32 / 48 Directed Acyclic Graphs (DAG) More complex example example of a more complex path D → Y is the causal path Non-causal paths: D ← X3 → Y , D ← X3 ← X4 → X5 → Y , D ← X2 ← X1 → X3 → Y , D ← X2 ← X1 → X3 ← X4 → X5 → Y The task is to check whether these paths are open, and if yes how they can be closed 33 / 48 Directed Acyclic Graphs (DAG) Path structures Complex paths can be decomposed into 3 simple structures outcome 1 Chain: open A → B → C: A has indirect causal effect on C via B, i.e.. A paths and C have causal association change in the value of mediator changes the outcome 2 Fork: A ← B → C: A and C have non-causal association, because they are both influenced by B (common cause confounding ) 3 Inverted fork: A → B ← C: A and C are not associated because the flow of information going out information collides in B (common effect collider ) blocked path of A and C In (1) and (2) the path from A to C is open, in (3) the path from Acollides with B to C is closed Without doing something only paths containing an inverted fork are closed Above this is only true for D ← X2 ← X1 → X3 ← X4 → X5 → Y 34 / 48 Directed Acyclic Graphs (DAG) How can we close open back-door paths? There are essentially two ways to close back-door paths 1 Randomize treatment randomization (if successful) makes potential outcomes independent of treatment in a DAG it removes all arrows pointing to D 2 With observational data we need further statistical tools conditioning on variables in open non-causal paths may solve the problem (regression, matching, subclassification) instrumental variables use of data prior to treatment (difference-in differences, panel data methods) 35 / 48 Directed Acyclic Graphs (DAG) What does conditioning do? 1 2 3 Chain A→B→C A ̸⊥ ⊥C and A ⊥ ⊥ C|B Fork A←B→C A ̸⊥ ⊥C and A ⊥ ⊥ C|B Inverted fork A→B←C A⊥ ⊥C and A ̸⊥ ⊥ C|B where ⊥ ⊥ denotes statistical independence and ̸⊥ ⊥ statistical dependence 36 / 48 Directed Acyclic Graphs (DAG) Interpretation / Intuition Conditioning on B means to set B to one of its possible values but a given value of B does not create variation in the variables coming after B This explains chains and forks: there is no path between A and C conditional on B The reasoning for 3 (inverted fork) is not so intuitive example: B: Hollywood fame; A: acting talent; C: beauty 2 causes for fame: A and C (independent in population) by conditioning on B (only looking at famous actors) talent and beauty become dependent a famous actor has either above average talent and below average beauty or vice versa → A and C are negatively correlated among famous actors Important: conditioning on a common outcome (collider) introduces spurious association between the two causes 37 / 48 Directed Acyclic Graphs (DAG) Conditioning on a collider set obs 5000 g Beauty=rnormal() g Talent=rnormal() g Fame=(Beauty+Talent)>1.76 Correlation of beauty and talent | Fame=1: -0.69 38 / 48 Directed Acyclic Graphs (DAG) Back-door criterion In order to identify a causal effect we need to close all back-door paths The back-door criterion states the conditions under which back-door paths are blocked A path P is blocked by a conditioning set of variables C iff 1 P contains a chain I → M → J or a fork I ← M → J such that the middle node M is in C 2 P contains a collider I → M ← J such that the middle node M is NOT in C With these two rules all DAGs can be examined with respect to identification of causal effects 39 / 48 Directed Acyclic Graphs (DAG) Reconsider Example 1 Two back-door paths: D ← X1 → Y and D ← X2 → Y These paths can be closed by conditioning on X1 and X2 , denoted by the box around X1 and X2 Holding X1 and X2 fixed means we can ignore all arrows going out these variables (right-hand DAG) in practice we do not explicitly remove the arrows, because that would make the DAG incomplete In other words, conditional on X1 and X2 treatment is as good as randomly assigned 40 / 48 Directed Acyclic Graphs (DAG) Variation of Example 1 Here, X2 is not observed (denoted by the shaded node) In this case we cannot condition on X2 Back-door path D ← X2 → Y cannot be closed This is the classic Omitted Variable Bias 41 / 48 Directed Acyclic Graphs (DAG) 2. Variation of Example 1 In this DAG there are two back-door paths: D ← X1 → Y and D ← X1 ← X2 → Y Both are closed by conditioning on X1 , so the causal effect of D on Y is identified (even if X1 is not independent of the unobserved X2 , which will be part of the error term in a regression, U ) Difference to requirement E[U |D, X1 ] = 0 for unbiased estimates in standard regression analysis (we will come back to this) 42 / 48 Directed Acyclic Graphs (DAG) Reconsider Example 2 Only back-door path: D ← X2 → Y Conditioning on X2 closes this path, and we identify the sum of the direct effect ,τ and the indirect effect, δβ1 in the right DAG the size of the effects are denoted by τ, δ, β1 in linear models the effect in the chain D → X1 → Y is the product of the individual effects What happens when we condition on X1 and X2 ? causal path D → X1 → Y is now blocked in this case we are able to identify the direct effect of D on Y, τ 43 / 48 Directed Acyclic Graphs (DAG) Variation of Example 2 Only back-door path: D → X1 ← X2 → Y Path is closed because X1 is collider on this path Regression of Y on D identifies sum of direct and indirect effect What happens when we condition on X1 ? the non-causal path D → X1 ← X2 → Y is opened regression of Y on D and X1 does not identify direct effect X1 is a bad control 44 / 48 Directed Acyclic Graphs (DAG) What is the connection to potential outcomes? Potential outcomes represent well-defined causal states These causal states are not incorporated in the causal graphs Need to introduce how causal states are represented in in Pearl’s variant of causal analysis Notion of an ideal experimental intervention and an operator called the the do(·) operator Two regimes: pre-intervention (observed reality) and under-intervention (hypothetical) In the under-intervention regime, the value that D takes on is set by an intervention applied to all units in the population This intervention is denoted by do(D = 1) or do(D = 0) 45 / 48 Directed Acyclic Graphs (DAG) What is the connection to potential outcomes? All causal quantities are defined by under-intervention distributions, not pre-intervention distributions The two probability distributions that define causal effects are P [Y |do(D = 1)] and P [Y |do(D = 0)] Here, AT E = E[Y |do(D = 1)] − E[Y |do(D = 0)] This corresponds to AT E = E[Y1 ] − E[Y0 ] So there is a clear connection between the potential outcomes and causal graphs We will not go into details of the math of do-operators 46 / 48 Directed Acyclic Graphs (DAG) Summary This was a brief introduction into DAGs The main assumption is that DAGs are complete: all causal paths are there, and everything that is missing is missing correctly Causal graphs offer a disciplined framework for expressing causal assumptions for entire systems of causal relationships. We will use them later to address issues such as bad control and instrumental variables 47 / 48