An Overview of Regression Analysis PDF
Document Details
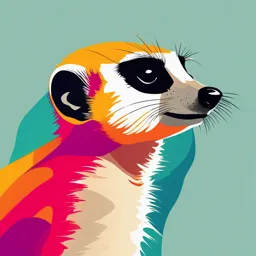
Uploaded by InsightfulAzalea
Tags
Summary
This document provides an overview of regression analysis and econometrics. It covers the uses of econometrics, including describing economic reality, testing hypotheses, and forecasting future economic activity. The document explains regression analysis, variables, and single-equation linear models.
Full Transcript
Here is the transcription of the document in markdown format: # Chapter 1 An Overview of Regression Analysis ## 1.1 What Is Econometrics? - JAIN BOOK SHOP 991136-5555 284, Satya Niketan, New Delhi No Exchange/No Return *"Econometrics is too mathematical; it's the reason my best friend isn't ma...
Here is the transcription of the document in markdown format: # Chapter 1 An Overview of Regression Analysis ## 1.1 What Is Econometrics? - JAIN BOOK SHOP 991136-5555 284, Satya Niketan, New Delhi No Exchange/No Return *"Econometrics is too mathematical; it's the reason my best friend isn't majoring in economics."* *"There are two things you are better off not watching in the making: sausages and econometric estimates."* *"Econometrics may be defined as the quantitative analysis of actual phenomena."* *"It's my experience that 'economy-tricks' is usually nothing more than a justification of what the author believed before the research was begun."* Obviously, econometrics means different things to different people. To beginning students, it may seem as if econometrics is an overly complex obstacle to an otherwise useful education. To skeptical observers, econometric results should be trusted only when the steps that produced those results are completely known. To professionals in the field, econometrics is a fascinating set of techniques that allows the measurement and analysis of economic phenomena and the prediction of future economic trends. You're probably thinking that such diverse points of view sound like the statements of blind people trying to describe an elephant based on which part they happen to be touching, and you're partially right. Econometrics has both a formal definition and a larger context. Although you can easily memorize the formal definition you'll get the complete picture only by understanding the many uses of and alternative approaches to econometrics. That said, we need a formal definition. Econometrics literally, economic measurement is the quantitative measurement and analysis of actual economic and business phenomena. It attempts to quantify economic reality and bridge the gap between the abstract world of economic theory and the real world of human activity. To many students, these worlds may seemfar apart. On the one hand, economists theorize equilibrium prices based on carefully conceived marginal costs and marginal revenues; on the other, many firms seem to operate as though they have never heard of such concepts. Econometrics allows us to examine data and to quantify the actions of firms, consumers, and governments. Such measurements have a number of different uses, and an examination of these uses is the first step to understanding econometrics. ### Uses of Econometrics *** Econometrics have three major uses:. 1. Describing economic reality 2. Testing hypotheses about economic theory and policy 3. Forecasting future economic activity The simplest use of econometrics is description. We can use econometrics to quantify economic activity and measure marginal effects because econometrics allows us to estimate numbers and put them in equations that previously contained only abstract symbols. For example, consumer demand for a particular product often can be thought of as a relationship between the quantity demanded (Q) and the product's price (P), the price of a substitute ($P_s$), and disposable income ($Y_d$). For most goods, the relationship between consumption and disposable income is expected to be positive, because an increase in disposable income will be associated with an increase in the consumption of the product. Econometrics actually allows us to estimate that relationship based upon past consumption, income, and prices. In other words, a general and purely theoretical functional relationship like $Q = B_0 + B_1P + B_2P_s +B_3Y_d $ can become explicit: $Q = 27.7 - 0.11P + 0.03P_s + 0.23Y_d $ This technique gives a much more specific and descriptive picture of the function. Let's compare Equations 1.1 and 1.2. Instead of expecting consumption merely to "increase" if there is an increase in disposable income, Equation 1.2 allows us to expect an increase of a specific amount (0.23 units for each unit of increased disposable income). The number 0.23 is called an estimated regression coefficient, and it is the ability to estimate these coefficients that makes econometrics valuable. The second use of econometrics is hypothesis test. To example, you could test the hypothesis that the product in Equation 1.1 is what economists call a normal good (one for which the quantity demanded increases when disposable income increases). You could do this by applying various statistical tests to the estimated coefficient (0.23) of disposable income ($Y_d$) in Equation 1.2. At first glance; the evidence would seemto support this hypothesis, because the coefficient's sign is positive, but the statistical significance of that estimate would have to be investigated before such a conclusion could be justified. Even though the estimated coefficient is positive, as expected, it may not be sufficiently different from zero to convince the author of that the true coefficient is indeed positive. The third and most difficult use of econometrics is to forest or predict what is likely to happen next quarter, next year, or farther into the future, based on what has happened in the. For example, economists use econometric models to make forecasts of variables like sales and Gross Domestic Product (GDP) and the inflation rate. The accuracy of such forecasts depends in large, business leaders, and politicians tend to be especially insterested in the use of econometrics because they need to make future decisions. *** There are many different approaches to quantitative work. For example, the fields of biology, psychology, and physics all face quantitative questions similar to those faced in economics and business. However, these fields tend to use somewhat different techniques for analysis because the problems they face aren't the same. For example, economic typically is an observational discipline rather than an experimental one. "We need a special field called econometrics, and textbooks about it because is generally accepted #### Steps used in nonexperimental quantitative research: 1. Specifying the model or relationships to be studie 3. The specifications used in step 1 *** This book will focus primarily on one particular econometric approach: single-equation linear regression analysis. The majority of this book will thus concentrate on regression analysis, but it is important for every econometrician to remember that Regression is only one of many approaches to econometric quantification. The importance of critical evaluation cannot be stressed enough. The possibility of missing or inaccurate data implies that the results from repression analysis always should be viewed with some caution. *** ### 1.2) What Is Regression Analysis? Econometrics use regression analysis to make quantitative estimates of economic relationships that previously have been completely theoretical in nature. After all, anybody can claim that the quantity of iPhones demanded will increase the price of the phones which decrease but not many could actually estimate by the number of the iPhones demand increases per dollar price decrease. Regression anaylis is the most used methode to estimated equations in Econometrics. #### Variables Regression analysis is a statistical technique to explain movements. For Example: $Q = β_0 + β_1P + B_2P_s + B_3Y_d$ $Q$ is the dependent variable and $P, Ps$ and $Y_d$ are the independent variables. Regression analysis is a natural tool for economists because most(though not all) economic propositions can be stated in such equations. Much of economics and business is concerned with cause-and-effect propositions. If the price of a good increases by one, then the quantity demanded goes to zero and it is dependent on the number of specify independent variables. Don't be deceived, however. Judgements as to causality must also include a dose of both economic theory and common sense. For example, if a bell on the door of a Flower shop rings before they buy flowers, it does not mean that the bell causes the flower to be purchased. Single-Equation Linear Models The simplest equation model is: $Y = B_0 + B_1X$ Figure 1.1 is a graph of a Graphical Representation of the Coefficients of the Regression Line. The graph of the equation $Y=B_0 + B_1X$ is linear with a constant slope equal to $B_1ΔY/ΔX$ Equation 1.3 states that Y, the dependent variable, is a single equation linear function of X, the independent variable, The model is a single-equation because it's the only equation specified. This means that if you were to plot Equation $1.3$, its would make a straight rather than a curved line . The regression model the response in the predicted value y and this is the slope of the model . $ (Y_2 - Y_1) / (X_2 - X_1) = ΔΥ/ΔΧ = β_1$ #### The Stochastic Error Term Y = β + β₁Χ. (1.3) There is almost always variation that comes from additional sources as well. A stochastic error term will be set to eliminate variations that come from explanatory variables. The addition of a stochastic error term (e) to Equation $1.3$ results in a typical regression equations: $Y = β_0 + β_1x + \epsilon$ (1.4) Equation 1.4 can be thought of as having two components, the deterministic component and the stochastic, or, or random component. The The expression $β_0 + β_1x$ is called the deterministic components of the regression equation. The deterministic part of the equation may written. which states that expected value of X, denoted as $E(YIX)$, is a Linear function of the independent variable (or variables if there are more than one.) Unfortunately, the Value and observed in the real world is unlikely to be exactly equal to the deterministic expression you. There at least there are four sources of variation in Y other than the variation included. -1) More miners are emitted. -2) It is impossible to measurement the valuable. -3)The underlying theoretical equation might have a different from. -4) All Attempts to generalization must contain one unpredictable value #### Figure 1.2 This graph represents an Errors Caused By Using A Linear Functional Form that models a Nonlinear Realationship with the Line showing linear functional form and a curve showing the true realationship *** Figure: Equation used $ \begin{aligned} &Y = β_0 + β_1X; + ϵ_i \\ &Y= \text{weight in pounds} \\ &X_i = \text{height in inches above 5feet} \\ &ϵ= \text{error term} \end{aligned} $ # Table 1.1 Data for and Results of the Weight-Guessing Equation | Observation |Height Above 5’ X | Weight Y| Predicted Weight| Residual e| $ Gain or Loss | |:-------------:|:-----------------:|:---------:|:-----------------:|:----------:| :--------:| | 1 | 5.0 | 140.0 | 135.3 | 4.7 | +2.00 | | 2 | 9.0 | 157.0 | 160.8 | -3.8 | +2.00 | | 3 | 13.0 | 205.0 | 186.3 | 18.7 | -3.00 | | 4 | 12.0 | 198.0 | 179.9 | 18.1 | -3.00 | | 5 | 10.0 | 162.0 | 167.2 | -5.2 | +2.00 | | 6 | 11.0 | 174.0 | 173.6 | 0.4 | +2.00 | | 7 | 8.0 | 150.0 | 154.4 | -4.4 | +2.00 | | 8 | 9.0 | 165.0 | 160.8 | 4.2 | +2.00 | | 9 | 10.0 | 170.0 | 167.2 | 2.8 | +2.00 | | 10 | 12.0 | 180.0 | 179.9 | 0.1 | +2.00 | | 11 | 4.0 | 170.0 | 173.6 | -3.6 | +2.00 | | 12 | 9.0 | 162.0 | 160.8 | 1.2 | +2.00 | | 13 | 10.0 | 165.0 | 167.2 | -2.2 | +2.00 | | 14 | 12.0 | 180.0 | 179.9 | 0.1 | +2.00 | | 15 | 8.0 | 160.0 | 154.4 | 5.6 | +2.00 | | 16 | 9.0 | 155.9 | 160.8 | -5.8 | +2.00 | | 17 | 10.0 | 165.0 | 167.2 | -2.2 | +2.00 | | 18 | 15.0 | 190.0 | 199.1 | -9.1 | +2.00 | | 19 | 13.0 | 185.0 | 186.3 | -1.3 | +2.00 | | 20 | 11.0 | 155.0 | 173.6 | -18.6 | -3.00 | | | | | | | TOTAL= |