Ambient Temperature and Mortality in South Africa (2018) PDF
Document Details
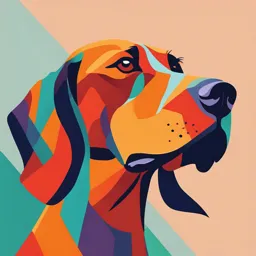
Uploaded by NeatPeridot6462
2018
Noah Scovronick
Tags
Summary
This article analyzes the association between ambient temperature and mortality in South Africa during 1997-2013. Using a national dataset of over 8.8 million deaths, a time-series analysis explored this relationship at the district level. The results reveal an association between daily maximum temperature and mortality, with a stronger impact on the youngest and oldest age groups and from cardiovascular and respiratory issues.
Full Transcript
Environmental Research 161 (2018) 229–235 Contents lists available at ScienceDirect Environmental Research...
Environmental Research 161 (2018) 229–235 Contents lists available at ScienceDirect Environmental Research journal homepage: www.elsevier.com/locate/envres The association between ambient temperature and mortality in South Africa: T A time-series analysis ⁎ Noah Scovronicka, , Francesco Serab, Fiorella Acquaottac, Diego Garzenac, Simona Fratiannic, Caradee Y. Wrightd, Antonio Gasparrinib a Woodrow Wilson School, Princeton University, Princeton, NJ 08544, USA b Department of Social and Environmental Health Research, London School of Hygiene and Tropical Medicine, London WC1H 9SH, UK c Department of Earth Sciences, University of Turin, Turin 10124, Italy d Environment and Health Research Unit, South African Medical Research Council and Department of Geography, Geoinformatics and Meteorology, University of Pretoria, Pretoria 0001, South Africa A R T I C L E I N F O A B S T R A C T Keywords: Background: There is an extensive literature describing temperature-mortality associations in developed regions, Temperature but research from developing countries, and Africa in particular, is limited. Weather Methods: We conducted a time-series analysis using daily temperature data and a national dataset of all 8.8 Climate million recorded deaths in South Africa between 1997 and 2013. Mortality and temperature data were linked at Mortality the district municipality level and relationships were estimated with a distributed lag non-linear model with 21 South Africa Cardiovascular days of lag, and pooled in a multivariate meta-analysis. Respiratory Results: We found an association between daily maximum temperature and mortality. The relative risk for all- age all-cause mortality on very cold and hot days (1st and 99th percentile of the temperature distribution) was 1.14 (1.10,1.17) and 1.06 (1.03,1.09), respectively, when compared to the minimum mortality temperature. This “U” shaped relationship was evident for every age and cause group investigated, except among 25–44 year olds. The strongest associations were in the youngest (< 5) and oldest (> 64) age groups and for cardior- espiratory causes. Heat effects occurred immediately after exposure but diminished quickly whereas cold effects were delayed but persistent. Overall, 3.4% of deaths (~ 290,000) in South Africa were attributable to non- optimum temperatures over the study period. We also present results for the 52 district municipalities in- dividually. Conclusions: An assessment of the largest-ever dataset for analyzing temperature-mortality associations in (South) Africa indicates mortality burdens associated with cold and heat, and identifies the young and elderly as particularly vulnerable. 1. Introduction also differ. Additionally, large segments of the population live in dwellings that do not adequately protect against heat and cold (Makaka There is an extensive literature describing temperature-mortality and Meyer, 2006; Scovronick and Armstrong, 2012; United Nations associations in developed regions, but research from developing coun- Human Settlements Programme, 2011). All of these factors are known tries, and Africa in particular, is limited (Basu, 2009; Benmarhnia et al., or putative modifiers of the effect of temperature on mortality (Basu, 2015; Gasparrini et al., 2015b; Ryti et al., 2016). Furthermore, the few 2009; Benmarhnia et al., 2015; Ryti et al., 2016; Scovronick and studies from Africa report results for only one or a few cities and over Armstrong, 2012). relatively short time periods (Azongo et al., 2012; Diboulo et al., 2012; The urgency to better understand temperature-health relationships Egondi et al., 2012; McMichael et al., 2008; Wichmann, 2017). in developing regions is heightened when considering the near-term There are several reasons that the relationship between ambient opportunities for intervention; rapid rates of economic development temperature and mortality in Africa may differ when compared to and demographic change, combined with explicit government pro- wealthier regions. Populations often have distinct mortality profiles grams aimed at infrastructure upgrading and poverty alleviation may (cause/age of death) and age distributions, while climatic factors may all affect vulnerability to ambient temperature. Examples in South ⁎ Correspondence to: Princeton University, 411a Robertson Hall, Princeton, NJ 08544, USA. E-mail address: [email protected] (N. Scovronick). https://doi.org/10.1016/j.envres.2017.11.001 Received 18 April 2017; Received in revised form 7 September 2017; Accepted 1 November 2017 Available online 20 November 2017 0013-9351/ © 2017 The Authors. Published by Elsevier Inc. This is an open access article under the CC BY license (http://creativecommons.org/licenses/BY/4.0/). N. Scovronick et al. Environmental Research 161 (2018) 229–235 Africa include government programs to help provide ~ 1.5 million We carried out a three-step quality control procedure on all tem- homes to low-income households over the coming years as well as perature series from both sources to exclude values resulting from ei- household energy and water supply projects (Department of Energy ther erroneous transcription or instrument malfunction (Aguilar et al., (Republic of South Africa), 2015; Department of Human Settlements 2003; Alexander and Herold, 2016; Perkins et al., 2012; Zhang et al., (Republic of South Africa), 2016; Department of Water and Sanitation 2011). First, we removed records where the maximum temperature was (Republic of South Africa), 2014). lower than the minimum. Second, we compared every series with The shortage of information on how temperature affects health in corresponding data from two or more nearby weather stations, selected South Africa is of extra concern in the context of climate change. Mean for proximity and data correlation with the reference series (Milewska warming has increased at least 50% faster in South Africa compared to and Vincent, 2016; Venema et al., 2012). If the reference series re- the global average and (like for much of the continent) this trend is corded an outlier (values above/below the 90th/10th percentiles), that expected to continue (Niang et al., 2014; Ziervogel et al., 2014). As a outlier was removed if the difference with the comparison series result, there is strong consensus that adaptation will be key to protect was ± two root root-mean-square errors. The root-mean-square error populations in Africa from future climate impacts and that early action between the reference and comparison series was calculated daily. is needed (Niang et al., 2014; Schaeffer et al., 2013). However, the Additionally, we removed all duplicate sets – defined as periods with at Intergovernmental Panel on Climate Change, along with independent least five consecutive days recording the same temperature. And third, researchers and the South African government have all pointed to a lack for series exhibiting a break in recording or where metadata indicated a of research on climate- and weather-health relationships as a barrier to change or relocation of the instrument, the series was tested for climate-informed decision-making (Government of the Republic of homogeneity and corrected if necessary (Fortin et al., 2016; Wang and South Africa, 2011; Niang et al., 2014; Smith et al., 2014; Wright et al., Feng, 2010; Wang, 2008a, 2008b; Wang et al., 2010, 2007). Only one 2014; Ziervogel et al., 2014). district (Buffalo City) required an adjustment. Accordingly, in this study we analyze the association of temperature After the quality control procedure, we assembled a final composite and mortality in South Africa using a national dataset that includes all dataset consisting of one representative station for each district (Fig. 1), 8.8 million recorded deaths between 1997 and 2013. This is the first selected for the length of the series and fewest missing data points. In study to employ such a large-scale dataset from anywhere in Africa, and the first year of the study period, there was temperature data available to our knowledge no comparable study exists from any country at a for 29 districts, rising to cover all 52 by 2007 (Fig. S1). For each series similar level of economic development. in the final dataset, we reconstructed missing data if the data gap was less than or equal to six days in length, using information from the 2. Methods nearby comparison stations (Acquaotta et al., 2009, 2016; Eischeid et al., 2000). We conducted a time-series regression analysis of the temperature- Overall, seven percent of values were reconstructed for the daily mortality relationship in South Africa using a national mortality dataset maximum and 12% for the daily minimum temperature. Due to the and two independent sources of temperature data. higher number of missing values and because improper instrument management generally has a greater impact on minimum temperature 2.1. Mortality dataset recordings (Acquaotta et al., 2015; Caussinus and Mestre, 2004; Nigrelli et al., 2015; Rangwala and Miller, 2012; Trewin, 2010; Vincent et al., We obtained a dataset of all recorded deaths (n = 8,814,625) in 2009), all subsequent analyses in this paper use the daily maximum South Africa from 1997 to 2013, inclusive (17 years). The information temperature. is from the country's civil registration system, the only national source of mortality statistics. The dataset was provided by Statistics South 2.3. Statistical approach Africa, which estimates that death registration for adults is ~ 89% complete early in the study period, rising to ~ 94% by the end (com- We applied a two-stage time-series modeling strategy, described in pleteness of child records has not been reported) (Statistics South several recent methodological papers and previously applied in both Africa, 2014a, 2014b). Anonymized individual data reported where multi-city and multi-country contexts, thus allowing for consistency each death occurred at the level of the district municipality (there are and comparability between studies (Gasparrini, 2011; Gasparrini et al., 52 in South Africa and we refer to them hereafter as “districts”). District 2012, 2015a, 2016, 2015b). We briefly describe the modeling stages sizes range from relatively small urban areas to much larger areas lo- below and also refer readers to these prior publications for more details cated in the more unpopulated regions of the country (Fig. 1). In ad- (Gasparrini, 2011; Gasparrini et al., 2012, 2015a, 2016, 2015b). dition to death district, individual data included cause and age of death. In the first stage, we applied standard time-series quasi-Poisson Data on district of residence was not available. regression models separately for each district to derive estimates of After dropping records for stillbirths (n = 226,593), deaths with location-specific temperature-mortality associations. For this step, we incomplete district information (n = 82,154) and with an incorrect modeled the exposure-response association with a natural cubic spline (nonsensical) date of death (n = 49), the final all-cause, all-age dataset with three internal knots at the 10th, 75th and 90th percentiles of lo- consisted of 8,509,130 records. The number of all-age all-cause deaths cation-specific temperature and the lag-response association using a for each district can be found in Table S1 of the Supplementary mate- natural cubic spline with an intercept and three knots equally spaced on rial. the log scale. We controlled for season and trend with a natural cubic spline with eight degrees of freedom per year and also for day of the 2.2. Temperature dataset week. In the second stage, we reduced the association in two dimensions: We obtained daily minimum and maximum temperature data from first to the overall temperature-mortality association, cumulating the two sources: the National Oceanographic and Atmospheric Association risk over a 21-day lag period to account for the delayed effects of cold (NOAA) of the United States and South Africa's Agricultural Research and for potential short-term mortality displacement; then to the lag- Council. The NOAA dataset included 63 daily series covering a subset of response associations corresponding to the 99th and 1st percentiles 30 districts, while the Agricultural Research Council dataset included using the district-specific minimum mortality temperature (MMT) as a 50 series, one for each district except the City of Johannesburg and reference. We pooled the estimated location-specific estimates using a Nelson Mandela Bay. There was no overlap in measurement points multivariate meta-analytical model, controlling for location-specific (stations) between the two sources. average (maximum) temperature and temperature range. The fitted 230 N. Scovronick et al. Environmental Research 161 (2018) 229–235 Fig. 1. Mean maximum temperature for each district, location of the weather monitoring station, and source of the data. ARC = Agricultural Research Council (South Africa), NOAA = National Oceanographic and Atmospheric Administration (USA). meta-analytical model was used to derive the best-linear unbiased response curves by age and cause groupings. The all-age all-cause curve prediction of the overall cumulative exposure-response association in shows elevated mortality on both sides of the temperature distribution, each district. with relative risks of up to ~ 20% at the extreme ends when compared We quantified the total attributable number of all-cause deaths as- to the minimum mortality temperature. Heat and cold effects are evi- sociated with non-optimum temperatures, which is given by the sum of dent for all the age and cause groupings, with the exception of 25–44 the contributions from all days in the series, and its ratio with the total year olds. The steepest curves are found in the youngest and (in par- number of deaths provides the total attributable fraction. We calculated ticular) oldest age groups. Cardiovascular and respiratory diseases also the components attributable to cold and heat by summing the subsets exhibited higher risks from cold and heat, respectively. corresponding to days with temperatures below or above the MMT The best-linear unbiased predictions of the overall cumulative ex- using the district-specific temperature distributions. To explore the ef- posure response associations for all-age all-cause mortality in each of fect of extreme cold and heat specifically, we also report attributable the 52 districts are presented in Fig. S2. Like for the pooled results, most mortality due to temperatures below and above the 2.5th and 97.5th districts show evidence of a ‘U’ shaped curve, although several do not temperature percentiles, respectively. have clear cold and/or heat effects, the latter including some of the We calculated empirical confidence intervals for the exposure-re- most populous urban districts (e.g. City of Cape Town and City of Jo- sponse curves using Monte Carlo simulations assuming a multivariate hannesburg). There is evidence of moderate heterogeneity across dis- normal distribution of the best linear unbiased predictions of the re- tricts (Cochran Q p-value < 0.001, I2 = 41.9%). duced coefficients. Confidence intervals for the MMTs were determined The proportion of deaths nationally attributable to low and high using an approximate parametric bootstrap estimator (Tobías et al., temperatures combined was 3.0% and 0.4%, respectively, for a total 2016). attributable fraction from non-optimum temperatures of 3.4%. The The modeling strategy described above was selected from several district range was 1–6% (Fig. 3, Table S1). Only a small percentage (~ potential approaches and therefore we conducted sensitivity analyses 13%) of the attributable mortality was due to extreme temperatures on key modeling choices including the lag period, the degrees of (Table S2). freedom used for seasonal control and in the lag-response association, The high proportion of total temperature-attributable deaths related and the type of spline and knot location for the exposure-response as- to cold is a function of the cold slope and the relatively high minimum sociation. mortality temperature (Table 1). High minimum mortality tempera- All analyses were conducted with the R software packages dlnm and tures were also evident in the other age- and cause-groups, with the mvmeta, which have been used extensively and are documented in de- exception of the youngest groups (Table 1). A total mortality burden of tail elsewhere (Gasparrini, 2011; Gasparrini et al., 2012, 2015b). 3.4% is equivalent to ~ 290,000 deaths, or an average of ~ 17,000 per year, over the study period. 3. Results The timing of risk differed for exposure to cold and heat. The cold effect peaked on the second or third day after exposure and persisted at The mean daily maximum temperature for South Africa as a whole a low level for two weeks or more (Fig. 4). In contrast, the strongest during the study period was 25.3 °C. District-level means ranged from heat effects occurred immediately but diminished quickly, followed by 15.8 °C (Buffalo City) to 30.6 °C (Vhembe) (Fig. 1, Table S1), with an an extended period of slightly negative risk that may suggest (marginal) interquartile range between 21.7 °C and 29.2 °C. In total, 89% (n = mortality displacement (Fig. 4). 7,576,674) of all deaths in the database had matching temperature data Changing modeling assumptions generally had a modest impact on and could therefore be included in the temperature-mortality analyses results, with attributable fractions remaining between 2.5% and 5.0% (Table 1). (Table S3). Of the parameters tested, the length of the lag had the Fig. 2 displays pooled results for the overall cumulative exposure- largest influence, with a shorter lag reducing the size of the effect and 231 N. Scovronick et al. Environmental Research 161 (2018) 229–235 Table 1 Deaths analyzed and results from pooled analyses including minimum mortality temperature percentiles (MMP) and relative risks at select temperatures compared to the minimum. Age/cause group Deathsa MMP Relative risk Total Includedb 1st percentile 99th percentile All-cause, all-age 8,509,130 7,576,674 84 (56,89) 1.14 (1.10,1.17) 1.06 (1.03, 1.09) Cardiovascularc 1,299,688 1,154,896 80 (64,89) 1.33 (1.24,1.42) 1.08 (1.02, 1.14) Respiratoryd 1,046,914 931,556 84 (60,90) 1.16 (1.07,1.25) 1.10 (1.03,1.18) Other causes 6,162,528 5,490,222 84 (27,90) 1.09 (1.06,1.12) 1.05 (1.01,1.09) < 5 years 800,268 700,088 26 (1,44) 1.08 (0.99,1.18) 1.24 (1.15,1.34) 5–24 years 623,687 553,088 19 (9,99) 1.10 (1.00,1.20) 1.10 (1.00,1.20) 25–44 years 2,665,115 2,382,762 95 (1,99) 1.02 (0.97,1.06) 1.00 (0.97,1.04) 45–64 years 2110,984 1,889,622 81 (47,99) 1.16 (1.09,1.22) 1.04 (0.99,1.08) 65+ years 2,265,979 2,016,041 85 (74,88) 1.34 (1.28,1.41) 1.13 (1.07,1.20) MMP: Minimum mortality temperature percentile. a Excludes stillbirths, deaths without location information and nonsensical dates of death. b Deaths were included for all days with temperature data. c ICD-10: I00-I99. d ICD-10: J00-J99. All−causes Cardiovascular causes Respiratory causes 1.8 1.8 1.8 1.6 1.6 1.6 Relative risk 1.4 1.4 1.4 1.2 1.2 1.2 1.0 1.0 1.0 0.8 0.8 0.8 0 1 10 50 90 99 100 0 1 10 50 90 99 100 0 1 10 50 90 99 100 Other causes 0 to 4 years 5 to 24 years 1.8 1.8 1.8 1.6 1.6 1.6 Relative risk 1.4 1.4 1.4 1.2 1.2 1.2 1.0 1.0 1.0 0.8 0.8 0.8 0 1 10 50 90 99 100 0 1 10 50 90 99 100 0 1 10 50 90 99 100 25 to 44 years 45 to 64 years 65+ years 1.8 1.8 1.8 1.6 1.6 1.6 Relative risk 1.4 1.4 1.4 1.2 1.2 1.2 1.0 1.0 1.0 0.8 0.8 0.8 0 1 10 50 90 99 100 0 1 10 50 90 99 100 0 1 10 50 90 99 100 Temperature percentile Temperature percentile Temperature percentile Fig. 2. Pooled overall relative risks of mortality associated with maximum daily temperature cumulated over a 21-day lag period for different cause and age groups. Dotted vertical line show the minimum mortality temperature percentile. 232 N. Scovronick et al. Environmental Research 161 (2018) 229–235 Fig. 3. Attributable fraction for heat and cold by district. Exact values available in Table S1. vice versa. We found a MMP of 84, which is higher than what is often reported in multi-country studies (Guo et al., 2014). However, we use the daily maximum rather than daily mean temperature, making direct com- 4. Discussion parisons difficult. In terms of lag-response relationships, our finding of a relatively immediate and short-lived heat effect, but a delayed and This is the first long-term, national-level study of temperature- persistent cold effect is commonly reported (Braga et al., 2002; Guo mortality associations in South Africa or anywhere on the African et al., 2014). continent. Our findings provide evidence of an increased risk of death In sub-group analyses, we found evidence of elevated risks from from both cold and heat, and suggest that approximately 3.4% of the cardiovascular and respiratory diseases and in the oldest and youngest total mortality burden in the country was related to non-optimum age groups. Though not universal, these results are consistent with temperatures over the 17-year study period. The majority of the burden studies from around the world, including in parts of Africa (Basu, 2009; (almost 90%) was from cold, defined as days with temperatures below Benmarhnia et al., 2015; Diboulo et al., 2012; Egondi et al., 2012; Ryti the optimum, and most was from moderate rather than extreme cold, et al., 2016; Wichmann, 2017). Higher risks amongst the elderly and for which is in line with previous research (Gasparrini et al., 2015b). cardiorespiratory causes may have important implications for how the A total attributable mortality of 3.4% is at the lower end of the temperature-mortality association in South Africa may evolve over time levels recently reported in a 13-country analysis using related methods as the population continues to age and mortality further transitions (Gasparrini et al., 2015b). In that study, the two countries (Brazil and away from infectious causes and towards chronic disease (Mayosi et al., Thailand) with the most similar overall attributable mortality were also 2009; United Nations, 2015). two of the most similar to South Africa in national-level indicators of The ability to investigate temperature effects in children < 5 years economic development, such as GDP per capita and income inequality is a particular strength of the study, as there are relatively few papers (World Bank, 2017). How these factors relate to temperature vulner- reporting results in such young age groups. We found evidence of a ability may warrant additional study. A) 1st percentile of Tmax B) 99th percentile of Tmax 1.05 1.05 Relative risk 1.00 1.00 0.95 0.95 0 5 10 15 20 0 5 10 15 20 Lag (days) Lag (days) Fig. 4. Pooled relative risk of all-age all-cause mortality over the 21-day lag period at the 1st (panel A) and 99th (panel B) percentile of the distribution of daily maximum temperatures (Tmax) relative to the minimum mortality temperature. 233 N. Scovronick et al. Environmental Research 161 (2018) 229–235 heat- and cold-effect, though the former was more definitive. Heat inform early warning systems and heat-health action plans and be used vulnerability in young children has been reported in systematic reviews to project future mortality impacts under climate change. and several individual studies in developing regions (Azongo et al., 2012; Basu, 2009; Diboulo et al., 2012; Geruso and Spears, 2017; Xu Acknowledgments et al., 2012). There is only one previously published time-series study on tem- NS thanks Princeton's Climate Futures Initiative for support. We perature-related mortality in South Africa, which analyzes data from thank Statistics South Africa (StatsSA) for providing the mortality data Cape Town between 1996 and 1999. Similar to our study, they reported and the Agricultural Research Council (ARC) of South Africa for pro- evidence of cold-related mortality but no clear heat effect in Cape Town viding temperature data. We also thank the anonymous reviewers for (McMichael et al., 2008). A similar result was also found in an un- their helpful suggestions. published time-series analysis of Cape Town using newer data (2001–2004) (Kovats, 2010). In contrast, a recent case-crossover study Funding sources based on data from 2006 to 2010 did find an association between all- age all-cause mortality and heat in Cape Town, but using an alternative AG and FS are supported by a research grant from the Medical temperature metric (apparent temperature) and different analytical Research Council UK (Grant ID: MR/M022625/1). CYW is supported by method (Wichmann, 2017). Like our results, that study did not report research funding from the National Research Foundation and the South significant associations in the other two cities investigated (City of African Medical Research Council. Johannesburg and Durban). All four studies relied on the same source of mortality data, albeit for different (but partially overlapping) time Competing financial interests periods. The mortality dataset itself is a potential source of error, as Statistics The authors declare they have no actual or potential competing fi- South Africa estimates that up to 11% of deaths may not have been nancial interests. captured by the national registry early in our study period, declining to around 6% by the end (Statistics South Africa, 2014a, 2014b). External Appendix A. Supporting information mortality estimates suggest the incompleteness may be larger (Institute for Health Metrics and Evaluation, 2015). If missing death records are Supplementary data associated with this article can be found in the random, they would likely have little impact on our relative risk esti- online version at http://dx.doi.org/10.1016/j.envres.2017.11.001. mates, but if exclusions were somehow systematically related to tem- perature, they could be meaningful. References The temperature dataset also posed certain challenges and limita- tions. The most obvious is that we did not have data for all days in all Acquaotta, F., et al., 2009. On the continuity and climatic variability of the meteor- districts, with much of the missing data concentrated in the early per- ological stations in Torino, Asti, Vercelli and Oropa. Meteorol. Atmos. Phys. 103, 279–287. iods. Specifically, 11% of deaths had no corresponding temperature Acquaotta, F., et al., 2015. Temperature changes in the North-Western Italian Alps from data, although this varied across districts. For many districts and time 1961 to 2010. Theor. Appl. Climatol. 122, 619–634. periods however, we were able to obtain data from multiple stations, Acquaotta, F., et al., 2016. Assessment of parallel precipitation measurements networks in Piedmont, Italy. Int. J. Climatol. 36, 3963–3974. helping us conduct a quality control procedure that was based in part Aguilar, E., et al., 2003. Guidelines on Climate Metadata and Homogenization. Vol. World on comparisons with nearby locations. This process allowed us to select Climate Programme Data and Monitoring WCDMP-No. 53, WMO-TD No. 1186. World the best available dataset for each district and to exclude problematic Meteorological Organization, Geneva. Alexander, L., Herold, N., 2016. ClimPACT2 indices and software (R software package). data points (duplicates and false outliers). Ultimately, we selected the World Clim. Program. User Man. 46. maximum daily temperature as our metric of choice, instead of the Azongo, D.K., et al., 2012. A time series analysis of weather variability and all-cause minimum or mean, because the minimums had substantially more mortality in the Kasena-Nankana Districts of Northern Ghana 1995–2010. Glob. Health Action 5, 19073. missing data (12% vs 7%). Previous analyses indicate that, on average, Barnett, A.G., et al., 2010. What measure of temperature is the best predictor of mor- different temperature measures have similar predictive abilities, due tality? Environ. Res. 110, 604–611. largely to their strong correlation, and therefore practical concerns like Basu, R., et al., 2008. Characterizing temperature and mortality in nine California data completeness should be the determining factor (Barnett et al., counties. Epidemiology 19, 138–145. Basu, R., 2009. High ambient temperature and mortality: a review of epidemiologic 2010; Basu et al., 2008). A final limitation with regard to temperature is studies from 2001 to 2008. Environ. Health 8, 1. that for some larger districts, the use of a single station may not ade- Benmarhnia, T., et al., 2015. Review article: vulnerability to heat-related mortality: a quately capture all local weather conditions, though this issue was systematic review, meta-analysis, and meta-regression analysis. Epidemiology 26, 781–793. somewhat tempered by the fact that total mortality was more con- Braga, A.L., et al., 2002. The effect of weather on respiratory and cardiovascular deaths in centrated in the smaller districts; for example, only 14% of deaths oc- 12 US cities. Environ. Health Perspect. 110, 859. curred in the largest 25% of districts. Caussinus, H., Mestre, O., 2004. Detection and correction of artificial shifts in climate series. J. R. Stat. Soc. Ser. C Appl. Stat. 53, 405–425. Some researchers investigating temperature-mortality relationships Department of Energy (Republic of South Africa), 2015. Strategic Plan 2015–2020. include ambient air pollution as a potential confounder a priori, since it Pretoria. can be correlated with temperature, while others argue that such con- Department of Human Settlements (Republic of South Africa), 2016. Annual Report 2015/2016. Pretoria. trol is inappropriate because air pollution is on the causal pathway Department of Water and Sanitation (Republic of South Africa), 2014. Strategic Plan for (McMichael et al., 2008). We did not have air pollution data, but note the Fiscal Years 2015/2016 to 2019/2020. Pretoria. that large-scale studies exploring the issue generally find that it has Diboulo, E., et al., 2012. Weather and mortality: a 10 year retrospective analysis of the Nouna Health and Demographic Surveillance System, Burkina Faso. Glob. Health only a small influence on effect estimates and may even strengthen the Action 5, 19078. temperature association (Basu et al., 2008; Gasparrini et al., 2015b; Egondi, T., et al., 2012. Time-series analysis of weather and mortality patterns in McMichael et al., 2008). Accordingly, a previous study from Cape Town Nairobi's informal settlements. Glob. Health Action 5, 19065. reported that controlling for ozone had a very small impact overall Eischeid, J.K., et al., 2000. Creating a serially complete, national daily time series of temperature and precipitation for the western United States. J. Appl. Meteorol. 39, while controlling for particulate air pollution increased the heat effect 1580–1591. (McMichael et al., 2008). Fortin, G., et al., 2016. The evolution of temperature extremes in the Gaspé Peninsula, In conclusion, our work provides valuable insights into tempera- Quebec, Canada (1974–2013). Theor. Appl. Climatol. 1–10. Gasparrini, A., 2011. Distributed lag linear and non-linear models in R: the package dlnm. ture-mortality relationships in a country and region that is under- J. Stat. Softw. 43, 1. studied and underrepresented in the literature. The results can help 234 N. Scovronick et al. Environmental Research 161 (2018) 229–235 Gasparrini, A., et al., 2012. Multivariate meta‐analysis for non‐linear and other multi‐- Contribution of Working Group III to the Fifth Assessment Report of the parameter associations. Stat. Med. 31, 3821–3839. Intergovernmental Panel on Climate Change. Cambridge University Press, Cambridge Gasparrini, A., et al., 2015a. Temporal variation in heat–mortality associations: a mul- and New York, pp. 811–922. ticountry study. Environ. Health Perspect. 123, 1200–1207. Statistics South Africa, 2014a. Mortality and Causes of Death in South Africa, 2011: Gasparrini, A., et al., 2015b. Mortality risk attributable to high and low ambient tem- Findings from Death Notification. Pretoria. perature: a multicountry observational study. Lancet 386, 369–375. Statistics South Africa, 2014b. Mortality and Causes of Death in South Africa, 2013: Gasparrini, A., et al., 2016. Changes in susceptibility to heat during the summer: a Findings from Death Notification. Pretoria. multicountry analysis. Am. J. Epidemiol. 183, 1027–1036. Tobías, A., et al., 2016. Investigating uncertainty in the minimum mortality temperature: Geruso, M., Spears, D., 2017. Heat, humidity and infant mortality in the developing methods and applications to 52 Spanish cities. Epidemiology 28, 72–76. world. Economics Working Paper. University of Texas-Austin. Trewin, B., 2010. Exposure, instrumentation, and observing practice effects on land Government of the Republic of South Africa, 2011. National Climate Change Response temperature measurements. Wiley Interdiscip. Rev.: Clim. Change 1, 490–506. White Paper. Pretoria. United Nations, 2015. World Population Aging. Highlights, New York. Guo, Y., et al., 2014. Global variation in the effects of ambient temperature on mortality: United Nations Human Settlements Programme, 2011. Global Report on Human a systematic evaluation. Epidemiology 25, 781. Settlements 2011: Cities and Climate Change. Earthscan, London and Institute for Health Metrics and Evaluation, 2015. Global Burden of Disease Study 2015. Washington DC. Vol. 2016, Seattle. Venema, V.K., et al., 2012. Benchmarking homogenization algorithms for monthly data. Kovats, S., 2010. Temperature-Related Mortality in Delhi and Cape Town. Department of Clim. Past. 8, 89–115. Public Health and Policy. London School of Hygiene and Tropical Medicine London. Vincent, L.A., et al., 2009. Bias in minimum temperature introduced by a redefinition of Makaka, G., Meyer, E., 2006. Temperature stability of traditional and low-cost modern the climatological day at the Canadian synoptic stations. J. Appl. Meteorol. Climatol. housing in the Eastern Cape, South Africa. J. Build. Phys. 30, 71–86. 48, 2160–2168. Mayosi, B.M., et al., 2009. The burden of non-communicable diseases in South Africa. Wang, X.L., et al., 2007. Penalized maximal t-test for detecting undocumented mean Lancet 374, 934–947. change in climate data series. J. Appl. Meteorol. Climatol. 46, 916–931. McMichael, A.J., et al., 2008. International study of temperature, heat and urban mor- Wang, X.L., 2008a. Accounting for autocorrelation in detecting mean shifts in climate tality: the ‘ISOTHURM’ project. Int. J. Epidemiol. 37, 1121–1131. data series using the penalized maximal t or F test. J. Appl. Meteorol. Climatol. 47, Milewska, E.J., Vincent, L.A., 2016. Preserving continuity of long-term daily maxmium 2423–2444. and minimum temperature observations with automation of reference climate sta- Wang, X.L., 2008b. Penalized maximal F test for detecting undocumented mean shift tions using overlapping data and meteorological conditions. Atmosphere-Ocean 54, without trend change. J. Atmos. Ocean. Technol. 25, 368–384. 32–47. Wang, X.L., et al., 2010. New techniques for the detection and adjustment of shifts in Niang, I., et al., 2014. Africa. In: Barros, V. (Ed.), Climate Change 2014: Impacts, daily precipitation data series. J. Appl. Meteorol. Climatol. 49, 2416–2436. Adaptation, and Vulnerability. Part B: Regional Aspects. Contribution of Working Wang, X., Feng, Y., 2010. Rhtests V3. User Manual. Climate Research Division. Group II to the Fifth Assessment Report of the Intergovernmental Panel on Climate Atmospheric Science and Technology Directorate. Science and Technology Branch. Change. Cambridge University Press, Cambridge and New York, pp. 1199–1265. Environment Canada, Ontario. Nigrelli, G., et al., 2015. Climate variability and Alpine glaciers evolution in Northwestern Wichmann, J., 2017. Heat effects of ambient apparent temperature on all-cause mortality Italy from the Little Ice Age to the 2010s. Theor. Appl. Climatol. 122, 595–608. in Cape Town, Durban and Johannesburg, South Africa: 2006–2010. Sci. Total Perkins, S., et al., 2012. Increasing frequency, intensity and duration of observed global Environ. http://dx.doi.org/10.1016/j.scitotenv.2017.02.135. heatwaves and warm spells. Geophys. Res. Lett. 39, 20. World Bank, 2017. Indicators. Vol. 2017. Rangwala, I., Miller, J.R., 2012. Climate change in mountains: a review of elevation- Wright, C., et al., 2014. Human health impacts in a changing South African climate. South dependent warming and its possible causes. Clim. Change 114, 527–547. Afr. Med. J. 104, 568–573. Ryti, N., et al., 2016. Global association of cold spells and adverse health effects: a sys- Xu, Z., et al., 2012. Impact of ambient temperature on children's health: a systematic tematic review and meta-analysis. Environ. Health Perspect. 124, 12–22. review. Environ. Res. 117, 120–131. Schaeffer, M., et al., 2013. Africa’s Adaptation Gap: Technical Report. UNEP, New York. Zhang, X., et al., 2011. Indices for monitoring changes in extremes based on daily tem- Scovronick, N., Armstrong, B., 2012. The impact of housing type on temperature-related perature and precipitation data. Wiley Interdiscip. Rev.: Clim. Change 2, 851–870. mortality in South Africa, 1996–2015. Environ. Res. 113, 46–51. Ziervogel, G., et al., 2014. Climate change impacts and adaptation in South Africa. Wiley Smith, P., et al., 2014. Agriculture, forestry and other land use (AFOLU) (Chapter 11) In: Interdiscip. Rev.: Clim. Change 5, 605–620. Edenhofer, O. (Ed.), Climate Change 2014: Mitigation of Climate Change. 235