Remote Sensing Summary Notes PDF
Document Details
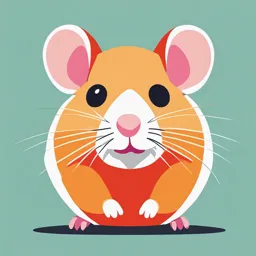
Uploaded by FlashyGenius1432
Universität für Bodenkultur Wien
Tags
Summary
This document provides a summary of remote sensing, including definitions, satellite history, and geographic information systems (GIS). It also covers the technical characteristics of electromagnetic waves.
Full Transcript
Summary Notes Class SIG Revised? Status Not started 1. Remote Sensing Definition: Remote sensing is the science of acquiring data about the Earth’s surface from a distance using reflected or emit...
Summary Notes Class SIG Revised? Status Not started 1. Remote Sensing Definition: Remote sensing is the science of acquiring data about the Earth’s surface from a distance using reflected or emitted electromagnetic radiation. Data is analysed to study surface properties, such as height, temperature, and vegetation health. How it works: Satellites and sensors measure electromagnetic radiation in various wavelengths, with atmospheric corrections needed to adjust for interference. Materials have unique spectral signatures, allowing identification and analysis of features like vegetation (using NDVI), water, and other surface characteristics. Satellite History: 1957: Sputnik launched. 1972: Landsat-1, the first Earth observation satellite, initiated systematic multispectral imaging. 2008: Landsat archive became open access. 2012: Google Earth Engine launched. Recent milestones include ESA’s Sentinel missions (e.g., Sentinel-2 in 2015) and private planetary computing platforms. Types of Satellites: Orbits: Polar or geostationary. Energy Source: Passive (rely on external energy) or active (use onboard energy, e.g., radar). Resolution types: Spatial, temporal, spectral, and radiometric. Summary Notes 1 Advantages: Offers consistent global observations, continuous monitoring, integration with Earth system models, and free web-based data analysis tools. Disadvantages: High data volume, processing complexity, regional limitations, and challenges in achieving high resolution across all parameters. Geographic Information Systems (GIS) Definition: GIS is a collection of tools for managing, analysing, and visualizing spatial data, often in vector or raster formats, with integrated databases for real-world representation. History: 1970s: Distribution of GIS systems like MOSS, GRASSGIS, and commercial platforms like CARIS and ERDAS. 1983: First commercial software (Odyssey). Late 20th century: Internet-based GIS data and open-source software like QGIS emerged. 21st century: Integration with cloud computing, relational databases, and software-as-a- service platforms. Advantages: Supports diverse data formats and hardware/software integrations. Facilitates record-keeping of geographical changes. Disadvantages: Requires prior knowledge in related fields. Expensive to implement and prone to errors due to extensive data needs. Differences Between Remote Sensing and GIS Focus: Remote sensing collects surface data, while GIS analyses and manages various data types. Summary Notes 2 Data Type: Remote sensing uses raster data; GIS can handle both raster and vector data. Output: Remote sensing produces satellite images and derived data, while GIS creates spatial models, maps, or 3D visualizations. Temporal Coverage: Remote sensing often provides periodic or near-real-time data, while GIS data is static or dynamic. 2. Electromagnetic Radiation and Remote Sensing Electromagnetic Waves and Radiation Electromagnetic waves are sinusoidal waves consisting of oscillating electric and magnetic fields that propagate through space, carrying momentum and radiant energy. Momentum is the product of an object’s mass and velocity, while radiant energy is energy emitted by a source. Key properties of electromagnetic waves include: Amplitude (A): Maximum displacement from equilibrium. Wavelength (λ): Distance between equivalent points on successive waves (e.g., peaks). Frequency (ν): Number of oscillations per second (inversely proportional to wavelength). Period (T): Time for successive peaks to pass a point (inverse of frequency). Propagation Speed (v): Distance traveled by the wave in a unit of time (speed of light in vacuum = c). Wave phenomena include: Refraction: Bending of light as it transitions between media. Diffraction: Bending around obstacles or through apertures. Scattering: Redirection of waves due to particle interactions. Polarization: Orientation of oscillations in the wave. Electromagnetic waves exhibit wave-particle duality (wave as oscillations, particle as photons). Higher wavelength means lower photon energy, and vice versa. Electromagnetic Spectrum Applications The spectrum spans various wavelengths, each with specific uses: Summary Notes 3 Radio waves (RADAR, LIDAR): Used for measuring distances and soil moisture. Microwaves: Applied in polarimetric radar systems for moisture sensing. Infrared and visible light: Used in spectrometers and lasers; highly reflective for vegetation and soils. Ultraviolet radiation: Limited to planetary studies. X-rays and gamma rays: Rarely used due to atmospheric opacity. Materials interact with electromagnetic radiation by absorbing, reflecting, or transmitting waves. Specular reflection: Light reflects uniformly on smooth surfaces. Diffuse reflection: Light scatters irregularly on rough surfaces. Transmission: Radiation passes unchanged through materials. Absorption: Radiation converts to other energy forms. Behaviour of Radiation in Specific Materials Snow/Ice/Clouds: Similar in visible light but distinguishable in other wavelengths. Snow reflects highly in visible and NIR spectra. Soils: High reflectivity in visible/NIR, with no transmission. Reflectivity depends on texture and organic content. Vegetation: High reflectivity/transmissivity in NIR, with visible light largely absorbed due to chlorophyll. Leaf structure, thickness, and water content influence reflectivity, providing insights into vegetation health. Summary Notes 4 3. Data Acquisition & Image Processing Remote Sensing Techniques 1. Passive Remote Sensing: Captures reflected or emitted radiation (e.g., LANDSAT, MODIS, Sentinel-2). Works in visible, infrared, thermal, and microwave ranges. Examples include spectrometers, radiometers, and cameras. 2. Active Remote Sensing: Emits signals and records interactions (e.g., LIDAR, RADAR, Sentinel-1). Operates mainly in the microwave spectrum. Image Processing Field of View (FOV): Defines the area (swath) covered by an image. Instantaneous Field of View (IFOV): Determines spatial resolution (area covered by one pixel). Image Composition: Images are matrices of digital numbers (DN) with one DN per pixel per filter (number of filters = number of bands). DNs are transformed into physical reflectance values. Sensor Characteristics: High spatial resolution implies low spectral resolution. Only discrete wavelength values are captured, not continuous ones. Summary Notes 5 Satellite Imaging Viewing Angles: Nadir: Zero-angle position. Hot Spot: Maximum reflectance angle, which may cause anomalies (e.g., water resembling ice). Radiometric Values: Sensor measurements are initially DNs, converted to radiance and reflectance values using calibration. Image Processing steps 1. Restoration: Corrects lost data (e.g., noise, pixel lines) using filters like median filters. 2. Pre-processing: Geometric Corrections: Align images with the Earth using control points, georeferencing, and resampling (e.g., nearest neighbor, bilinear, or bicubic methods). Radiometric Corrections: Converts DNs to radiance using calibration (gain and bias) to account for sensor degradation. Different calibration methods (pre-flight, on-board, field campaigns) ensure accuracy. Reflectivity at the top of the atmosphere Summary Notes 6 Atmospheric Corrections: Removes distortions caused by the atmosphere to retrieve surface reflectance. Algorithms like ATCOR, 6S, and MODTRAN simulate atmospheric conditions for corrections. 3. Enhancement: Improves image quality through color and contrast adjustments. 4. Transformation: Reduces dimensions using techniques like Principal Components Analysis (PCA). 5. Derivative Products: Produces outputs like landcover classifications or segmentations. Image Processing Levels Level 0-1: Raw data (DN values), time-referenced but difficult to use. Level 2: Georeferenced and calibrated data, converted to physical values (useful for landcover mapping). Level 3: Processed data mapped to a uniform grid and averaged/composited for specific time/geographic areas. Level 4: Combines Level 3 data with models or other datasets, easiest to use. Image Enhancement Contrast Adjustment: Improves image visualization by adapting radiometric resolution to display capabilities. Histograms help adjust max/min values to enhance contrast. Color Composites: Multispectral data is visualized via RGB layers (0–255 intensity). RGB composites combine different spectral bands, while grayscale uses the same band for all three layers. Transformations like RGB-to-HSI represent color using Hue (color), Saturation (purity), and Intensity (brightness). Transformations Summary Notes 7 NDVI (Normalized Difference Vegetation Index): Uses red and NIR bands to assess vegetation health. Values range from -1 (water) to 1 (dense vegetation). Tracks seasonal changes and phenology. PCA (Principal Components Analysis): Reduces dimensionality of multispectral data by retaining key information (e.g., first 3 components contain 95% of data). Image Classification Classifies pixels into land cover categories using their spectral characteristics. Can be supervised (trained with reference samples) or unsupervised (based on spectral properties alone). Methods include: Parametric: Uses mathematical functions (e.g., Maximum Likelihood, Minimum Distance). Non-Parametric: No mathematical model (e.g., K-means clustering, decision trees). Accuracy measured via “user accuracy” (row-based) or “producer accuracy” (column- based). Errors (e.g., omission) occur when pixels are misclassified. Land Cover vs. Land Use Land cover: Physical features (e.g., forest, crops). Land use: Functional purposes (e.g., industry). 4. Platforms for Remote Sensing Types of Platforms: Ground-Based: Drones, backpacks (e.g., for calibration). Airborne: Planes for regional analysis. Space-Based: Satellites orbit Earth with varying resolutions and purposes. Satellite Orbits: LEO (Low Earth Orbit): High spatial resolution (e.g., Landsat). GEO (Geostationary): Fixed position, synchronized with Earth's rotation. Polar Orbits: Cover poles, provide full global coverage. Summary Notes 8 Highly Elliptical Orbits: Manage satellite traffic. Platforms for Sensor Operation: Sensors acquire radiance from the sun and can be mounted on various platforms: Ground-based: Includes backpacks or drones, often used for sensor calibration and ground data collection (e.g., chlorophyll measurements). Air-based: Planes. Space-based: Satellites in various orbits. Satellite Orbits: Low Earth Orbit (LEO): Low height, high spatial resolution (e.g., Landsat). Medium Earth Orbit: Higher altitude, covers more area. Geosynchronous Orbits (GSO): Fixed position, moves with Earth. Polar Orbits: Pass by Earth’s poles. Highly Elliptical Orbits: Reduce satellite traffic; only part of the orbit is close to Earth. Key Satellites and Sensors: MODIS (ESA): 1 km spatial resolution (thermal infrared), high temporal resolution. SMOS: Measures humidity using microwaves. Sentinel Program (Copernicus, ESA): Sentinel 1: Radar, works through clouds, ideal for flood analysis. Sentinel 2: Optical, better spatial resolution but limited by clouds. Sentinel 3: Multiple sensors, lower spatial resolution, broad data collection. Sentinel 4: Focuses on atmospheric monitoring. Sentinel 5: Analyzes atmospheric chemistry. Sentinel 6: Tracks global surface height. Landsat (NASA): Oldest program (since 1970s), offering long-term temporal data of Earth’s surface. Landsat 8: Two thermal bands but 100m spatial resolution. Summary Notes 9 Landsat Next: Planned with better vegetation differentiation (10-20m spatial resolution). Free images available only after 2008. Other Satellite Programs: Many private companies provide better spatial resolution but charge for data. Microwave satellites provide coarse spatial resolution but specific applications. Resolution Types: Spatial: Pixel clarity (pure vs. mixed pixels). Radiometric: Number of bits. Spectral: Number of bands. Temporal: Frequency of sensor passes. 5. Geographic Information Systems (GIS): GIS integrates maps and databases to solve problems, locate information, and analyse spatially referenced data. Spatial data (locations) and attribute data (descriptions) are linked through unique IDs. Vector Data: Represents data as points, lines, or polygons, but only one geometry type per file. If opened in a programming language (not specialized software like ArcGIS), coordinate plotting is required to preserve location information, unlike raster data. Raster Data: Represented as grids or images, where each cell (pixel) has a fixed size. Raster files are inherently matrix-based, making them less prone to location loss. Projections and Coordinate Systems: Earth’s Shape: The Earth is irregularly shaped (geoid), so mathematical models like ellipsoids are used. A datum serves as a reference point for these models. Projections: Methods to map the Earth’s surface. Summary Notes 10 Geographic Coordinate System (GCS): Uses latitude and longitude in degrees, suitable for global-scale mapping but less accurate for smaller areas. Projected Coordinate System (PCS): Optimized for smaller areas, adjusting Earth’s shape for specific regions. Uses units like meters but loses accuracy for larger areas. UTM (Universal Transverse Mercator): A cylindrical projection system ideal for mapping regions. 6. Temporal Series: Definition: A sequence of data points measured over time, at regular or irregular intervals. In remote sensing, these include high temporal frequency observations for every pixel. Types of Temporal Series: Multi-annual: Spanning multiple years. Intra-annual: Within a single year. Key Techniques: High-temporal frequency observations (days to weeks). Smoothing methods (median smoothing, moving average). Trend analysis for long-term patterns. Pre-processing in Temporal Series: Gap Filling: Imputing missing data using ancillary or neighboring data. Smoothing: Applying statistical filters (mean, median, Savitzky-Golay) to reduce noise while retaining key data patterns. Smart Filtering: Advanced filters like Savitzky-Golay maintain features like maxima and minima. Phenology and Remote Sensing: Phenology: Studies life cycles of plants and animals relative to seasons, with metrics like Start of Season (SOS) and End of Season (EOS). Summary Notes 11 Remote Sensing Applications: Tracks seasonal changes (Land Surface Phenology) and uses NDVI (Normalized Difference Vegetation Index) for ecosystem monitoring. Anomalies in Temporal Series: Definition: Deviations from normal conditions (e.g., rainfall, NDVI). Calculation Methods: Absolute difference (physical units). Relative difference (percentages). Standardized anomalies (z-scores). Vegetation Condition Index (VCI): Measures NDVI changes to assess drought conditions. Change Detection: Identifies shifts in data patterns using image differencing techniques, such as: Urbanization: Changes visible in Las Vegas between 1973 and 2008. Deforestation: Monitored in areas like Rondônia, Brazil. Glacier Loss: Observed in the Alps over decades. Applications of Temporal Series Analysis: 1. Biosphere/Agriculture: Crop yield forecasting, water stress detection. 2. Biosphere/Forest: Tree species monitoring, forest health assessment. 3. Nature: Biodiversity mapping, carbon stock estimation. 4. Pedosphere: Soil erosion and contamination tracking. 5. Atmosphere: Air quality, climate modeling. 6. Hydrosphere: Monitoring sea temperature, water salinity. 7. Cryosphere: Glacier monitoring, snow cover assessments. 8. Other Areas: Archaeology, fire detection, epidemiology. Summary Notes 12