Historical Volatility Estimation PDF
Document Details
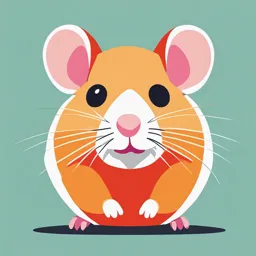
Uploaded by PreEminentPiccolo8712
UGent
Tags
Summary
This document provides an overview of different methods for estimating historical volatility in finance. It highlights the importance of volatility for investment decisions, performance evaluation, and risk management. The document covers issues like sample length, frequency, and measures used for calculating historical volatility. It also touches on the relationship between volatility and financial market characteristics like price jumps and volatility clusters.
Full Transcript
Stuvia - Koop en Verkoop de Beste Samenvattingen Portfolios and investments section 1: historical volatility The problem of estimating volatility Volatility is important for: 1. Investment decisions (->next se...
Stuvia - Koop en Verkoop de Beste Samenvattingen Portfolios and investments section 1: historical volatility The problem of estimating volatility Volatility is important for: 1. Investment decisions (->next section) 2. Performance evaluation (->last section) 3. Risk management (not in this course, sorry…) There are two ways to get a volatility forecast: 1. Implied volatility: the volatility that is consistent with the observed option prices. Look at what other people on average believe about the future. But markets can be wrong, so it isn’t guaranteed that markets in the future will actually look like that. 2. Historical volatility: one can use historical price series to estimate historical volatility and use it as a tool to forecast future volatility. Look at the past and hope that you can draw some conclusions from the past about the future. The problem is that volatility is a latent factor and not observable. You cannot look into parallel universes and see all the different outcomes, you can only draw conclusions from the one sample that you get. Therefore, we have to use estimators. There is not a single “correct” way to estimate the historical volatility. The concrete value depends on a couple of decisions we have to make. Sample length: How far do we look back? Sample frequency: How frequent do we collect/use data? Daily, weekly, monthly, … Measure to use: Which assumptions on the price process? S AMPLE LENGTH The choice of the sample length is a trade-off between two goals: accuracy and currentness. Long samples increase accuracy if the samples are stable. But we know that there are volatility clusters so that they aren’t stable. There may be structural changes as well or the central bank may decide to peck your currency against another currency. Short samples better capture time-variations: it is better to look at the past at a similar length then as you want to look in the future. If you want to forecast for 1 year then it would be good to also look back for 1 year. Looking only a couple of days back would be useless… Gedownload door: hannahducatteeuw | [email protected] Wil jij9€76 per Dit document is auteursrechtelijk beschermd, het verspreiden van dit document is strafbaar. maand verdienen? Stuvia - Koop en Verkoop de Beste Samenvattingen Estimating the volatility over a long sample period would rather measure the long-term average volatility. On the other hand, a sample period that is too short will introduce estimation errors and put too much weight on the very recent past. It is good to use multiples of three months because that is in accordance with the quarterly reporting periods of macro announcements. This is to ensure a constant number of volatility increasing quarterly reporting periods, when the rolling window moves. S AMPLING FREQUENCY Most commonly used are daily and weekly sampling frequencies. DAILY DATA: has to huge advantage of almost five times as many observations, which should increase the accuracy of the estimation. If you use daily data, you have much more data in 1 month. If you work with monthly data you only have 1 observation, with weekly data you have 4 observations per month and with daily data you have 21 observations per month. On the other hand, the use of weekly or monthly data decreases the impact of holidays or vacations, in daily data these would be missing observations. For monthly data you should always be able to make a calculation. Rule of thumb: weekly data is better for longer forecasting horizons and several markets are considered, otherwise you use daily data. T HE CHOICE OF VOLATILITY MEASURE : PRICE RANGES The concrete choice of the volatility measure has a huge impact on the volatility estimate. Therefore, this is a very difficult question! Often the standard deviation of the past returns is referred to as historical volatility: However, there are many more ways to measure volatility. Efficiency is measured as the deviation of the accuracy of a volatility estimator compared to the volatility of a benchmark estimator. The benchmark estimator that is traditionally used is the classical estimator or the close-to-close range- based estimator or squared return. All range-based estimators assume that the price P t follows the geometric Brownian motion: μ = the drift term σ = the constant volatility Bt = standard Brownian motion If only closing prices are available and if the mean return is 0, this is the average of the close-to-close return or classical estimator. The classical estimator for σ is: Gedownload door: hannahducatteeuw | [email protected] Wil10 jij €76 per Dit document is auteursrechtelijk beschermd, het verspreiden van dit document is strafbaar. maand verdienen? Stuvia - Koop en Verkoop de Beste Samenvattingen The first formula gives the normal standard deviation. If you would take the average of all the daily standard deviations (last formula) then you would get approximately the same as the top formula. 1 Except you don’t get the mean, so you get *∑(rt)². N The classical estimator is unbiased if and only if μ* = 0. The estimator is quite noisy, its error is comparatively large. It can be used as a benchmark, but also as an input for constructing new measures. The average of all the squared returns of the day would give you a measure of the volatility. Is that a good measure? Well if one thing, it is straightforward. If the security is not continuously traded, so if the exchange is closed during certain hours per day, we can also incorporate the opening prices and get the following measure: The market closes and when it opens again, there is a sudden price jump. This means that the value of a stock may change, even if the stock isn’t traded at the moment. So if the price represents the value, then the price should move as well even when the market is closed. We would have price jumps overnight, we will never be able to observe that, but we need to remember that these prices are constantly moving. Ot = opening time Ct = closing time F = a fraction of the day, e.g. if the market is open from 8 to 5, the fraction is 9/24 So if we only consider opening and closing prices, we will tend to underestimate the volatility, because the distance between opening and closing prices is always smaller (or equal) to the real range. For this reason Parkinson suggest a range-based estimator based on high (Ht) and low (Lt) prices. High and low prices are the highest and lowest prices that appear during the trading day. Gedownload door: hannahducatteeuw | [email protected] Wil11 jij €76 per Dit document is auteursrechtelijk beschermd, het verspreiden van dit document is strafbaar. maand verdienen? Stuvia - Koop en Verkoop de Beste Samenvattingen But a logical next question is: if we know that there are price jumps overnight and that the price changes when the market is closed, then why don’t we simply include that? If we have opening and closing value and the highest and lowest value then why don’t we simply use everything that we know? Garman and Klass then included opening and closing prices as well in the formula: (You don’t need to know the formulas by hard, what you should need to know is which points you can use to estimate the volatility) Lastly, Garman and Klass suggested to combine the classical estimator and the estimator by Parkinson: And this is the best we can do, if there is NO PRICE DRIFT i.e. μ*=0 !! What is left is to find a solution for non-zero drifts. A suitable estimator has been suggested by Rogers and Satchell. This if for continuous trading: And Yang and Zhang come with an alternative for non-continuous trade: OVERVIEW: If we look at the efficiency and take the classical estimator as a benchmark then we see that Parkinson for example gets an efficiency of 5,2. This means that, to get the same accuracy as you would get with the Parkinson estimator, you would need 5,2 times more data / information for the classical estimator. The more data you use (C, O, H, L), the less data you need and the less you are exposed to structural changes. Which these estimator you can calculate the values for one day, if you want to go from 1 day to a longer period you need to calculate the average. However, there is one thing that the estimators cannot deliver and that are volatility clusters, because they just measure volatility over an interval and there is no time dimension in that. Gedownload door: hannahducatteeuw | [email protected] Wil12 jij €76 per Dit document is auteursrechtelijk beschermd, het verspreiden van dit document is strafbaar. maand verdienen? Stuvia - Koop en Verkoop de Beste Samenvattingen The main advantage of the range-based measures is that they do not require lots of data. In the worst case the only data you need are open, close, high and low prices together with information about the times when the market closed. GARCH models We already mentioned that volatility is heteroscedastic and clusters. Heteroscedastic With non-constant volatility Homoscedastic With constant volatility Now, let us assume a very simple time series model: Rt = a + εt Rt = return, a = average and ε = error term εt N(0, σ²) the return of an asset is linked to the market’s return plus an error term. In this simple model the variance of the error term is not-time varying, the volatility σ² is constant (homoscedastic). The question arises: how can we incorporate the observed volatility clusters into the this model? A first important step was taken by Engle who let the error term’s volatility depend on the squared previous period’s disturbance. Use yesterday’s volatility to estimate today’s volatility! If yesterday’s price movements already have a little bit of an indication of today’s price movement then that is actually a good indicator. If yesterday’s price change was large, then today’s price change will probably also be large. We don’t know which sign it will have, but we know it will be large. However, we cannot observe volatility. So what can we observe? We can observe yesterday’s innovation, thus εt-1. So why not use εt-1²? Rt = a + εt εt N(0, σ²) σt² = ω + β.εt-1² This model provides us with a simple parameterization of the observed volatility clusters: the larger yesterday’s disturbance was, the more likely it is to observe a large disturbance today, of either sign. Volatility σ² is now conditional and autoregressive, thus time-dependent and depending on its own history! Engle called this model the ARCH model = AutoRegressive Conditional Heteroscedasticity σt² = ω + β.εt-1² is an arch-model of order 1 because the volatility is determined by the disturbance with lag 1. So we only look 1 day into the past and we know that because the formula uses t-1. Gedownload door: hannahducatteeuw | [email protected] Wil13 jij €76 per Dit document is auteursrechtelijk beschermd, het verspreiden van dit document is strafbaar. maand verdienen? Stuvia - Koop en Verkoop de Beste Samenvattingen p 2 This model can also be generalized to any order: σt² = ω + ∑ β i. ε t −i i=1 ω p And the unconditional variance is: σt² = 1−∑ β i i=1 In practical applications, it turns out that for modelling financial time series, high orders of the ARCH process are necessary. Bollerlev asked himself how to avoid the huge order of ARCH models. For this reason he developed the generalized ARCH model of GARCH. p q 2 2 σt² = ω + ∑ ai. ε t−i + ∑ b j. σ t − j i+ 1 j+1 In contrast to Engle’s original ARCH model, the conditional variance now does not only depend on yesterday’s disturbance term, but also on yesterday’s conditional variance. In practise it turns out that for almost every financial time series a GARCH(1,1) model is sufficient to capture all ARCH effects: σ 2t =ω+ a. ε 2t−1 +b. σ 2t −1 σ 2t−1 this captures additional disturbances. You only need to look at yesterday’s price change and yesterday’s volatility, so only look back one day into the past. This means the lowest order model is actually almost always sufficient because in fact σ 2t−1 already includes a whole history about the volatility. Therefore you only need to include omega, a and b. 2 2 The current volatility σ t is therefore a function of the whole history of price evolution. σ t is the CONDITIONAL variance of the garch process. ω σ 2= p q The UNCONDITIONAL variance is: 1−∑ ai −∑ b j i=1 j =1 a + b should NEVER exceed 1.The closer the sum is too one, the longer the volatility shocks last, so the longer it takes for them to die out. If a + b is close to 1 it will take a very long time before the volatility goes back to the normal level after a volatility shock. a + b < 1 : you expect that it will go back to the normal level very fast. a + b = 1 : volatility shocks would never die out, therefore it is not allowed for a + b to equal 1. a = b = 0 : we have a constant volatility model, yesterday’s volatility will not have any impact on today’s volatility. In practical applications we see that the sum is always very close to one, which implies that financial markets are characterized by a high degree of volatility persistence. Gedownload door: hannahducatteeuw | [email protected] Wil14 jij €76 per Dit document is auteursrechtelijk beschermd, het verspreiden van dit document is strafbaar. maand verdienen? Stuvia - Koop en Verkoop de Beste Samenvattingen The unconditional variances captures very well volatility clusters and also the size of the kurtosis. The unconditional kurtosis of a GARCH(1,1) process is: 6 a2 K = 3+ 1−b 2−2 ab−3 a2 The kurtosis always exceeds the normal distribution’s kurtosis of 3, if a > 0. The unconditional distribution of GARCH(1,1) is therefore leptokurtic (while the conditional one is normal). The GARCH model is able to model the two most important stylized facts regarding series of financial returns: volatility clusters leptokurtic unconditional distributions GARCH-in-mean The GARCH-in-mean captures the leverage effect, the observation that declining share prices often go along with higher volatility. Rt = α + β.RM + θ.σt² + εt σt² = ω + a.εt-1² + b.σt-1² GJR GARCH This model makes the volatility process asymmetric by adding an additional term to the variance equation that only occurs if the shock εt-1 in the previous period was negative. Rt = α + β.RM + εt σt² = ω + a.εt-1² + d.I