Statistical Workshop Series - Workshop 1 - SPSS Introduction, Data & Attributes
Document Details
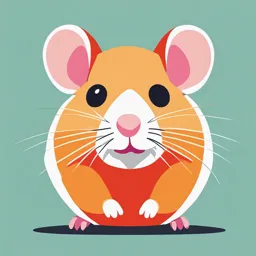
Uploaded by PoignantCynicalRealism
Sulaimani College of Dentistry
Arass J. Noori
Tags
Summary
This presentation outlines a statistical workshop series, specifically Workshop 1 centered around SPSS introduction and data attributes. It covers fundamental concepts like data types, variables, central tendency, and introduces descriptive statistics. It's a useful learning resource for introductory statistics.
Full Transcript
Statistical workshop series Workshop 1: SPSS introduction, data and its attributes. Arass J. Noori The software name originally stood for Statistical Package for the Social Sciences (SPSS), reflecting the original market, then later changed to Statistical Product and Service Solutions....
Statistical workshop series Workshop 1: SPSS introduction, data and its attributes. Arass J. Noori The software name originally stood for Statistical Package for the Social Sciences (SPSS), reflecting the original market, then later changed to Statistical Product and Service Solutions. Goals Data Normality Statistics Variables Distributions Test selection Data : all the information we collect to answer the research question Variables : Outcome, treatment, study population characteristics Terms Subjects : units on which characteristics are measured Observations : data elements Population : all the subjects of interest Sample : a subset of the population for which data are collected Descriptive vs Inferential Statistics SamplefromPopulation Sample And Population Numbers, numbers and numbers everywhere 555-867-5309 9001 9 3.5.05 97.5 502 4,832 834,722 77 999.998 65.87 362 4001.56732 51 1,248,965 2,387 9 21 672 145 999-99-9999 324 409 35.5 Represents a composite measure of a variable. Scales Series of items arranged according to value for the purpose of quantification. Provides a range of values that correspond to different characteristics or amounts of a characteristic exhibited in observing a concept. Scales come in four different levels: Nominal, Ordinal, Interval, and Ratio. A collective recording of observations either numerical or categorical is called data. It is classified into: 1. Qualitative data: when data is collected on the basis of attributes like sex, malocclusion, color, etc. (Binary, Nominal and Ordinal) Data 2. Quantitative data: when the data is collected through measurement using calipers like arch length, arch width, fluoride concentration in water supply. a. Discrete: when the variable under observation take only fixed value like whole numbers as DMF. (1, 4, 5, 77, 102, …etc) b. Continuous: if the variable can take any value in a given range decimal or fractional like arch length, mesiodistal width of the erupted tooth surface. (1.33, 34.6, 11.11 … etc). Variables Interval Ratio Scales Scale of measures Descriptive Statistics Types of descriptive statistics: Organize Data Tables Graphs Summarize Data: MEASURES OF CENTRAL TENDENCY (MEAN, MEDIAN, MODE). MEASURES OF LOCATION (POSITION) (RELATIVE STANDING) (PERCENTILES, DECILES AND QUARTILES). MEASURES OF VARIATION(SUCH AS THE RANGE, VARIANCE AND STANDARD DEVIATION , STANDARD ERROR). Frequency distribution Descriptive Statistics Summarize Data: MEASURES OF CENTRAL TENDENCY (MEAN, MEDIAN, MODE). MEASURES OF LOCATION (POSITION) (RELATIVE STANDING) (PERCENTILES, DECILES AND QUARTILES). MEASURES OF VARIATION (SUCH AS THE RANGE, VARIANCE AND STANDARD DEVIATION). These statistics answer the question: What is a typical score? Central The statistics provide information about the grouping of the numbers in a Tendency distribution by giving a single number that characterizes the entire distribution. Exactly what constitutes a “typical” score depends on the level of measurement and how the data will be used. For every distribution, three characteristic numbers can be identified: Mode Median Mean 1. Means can be badly affected by outliers (data points with extreme values unlike the rest) 2. Outliers can make the mean a bad measure of central tendency or common experience Mean Mean is the Average Income in the U.S. Bill Gates All of Us Mean Outlier The middle value when a variable’s values are ranked in order; the point that divides a distribution into two equal halves. Median When data are listed in order, the median is the point at which 50% of the cases are above and 50% below it. Class A--IQs of 13 Students The 50th percentile. 89 93 97 98 Median = 109 102 (six cases above, six below) 106 109 All of Us 110 115 119 Bill Gates 128 outlier Mean 131 140 Median Interquartile range The most common data point is called the mode. Mode The combined IQ scores for Classes A & B: 80 87 89 93 93 96 97 98 102 103 105 106 109 109 109 110 111 115 119 120 2.0 127 128 131 131 140 162 1.8 1.6 t n u o C 1.4 1.2 BTW, It is possible to have more than one mode! 1.0 82.00 89.00 96.00 98.00 103.00 106.00 109.00 115.00 120.00 128.00 140.00 87.00 93.00 97.00 102.00 105.00 107.00 111.00 119.00 127.00 131.00 162.00 IQ The Normal distribution (The Gaussian distribution) The Bell Curve.01.01 Significant Significant Mean=70 In probability theory, the central limit theorem says that, under certain conditions, the sum of many independent identically-distributed random variables, when scaled appropriately, converges in distribution to a standard normal distribution. Central limit theorem Standard normal distribution (z-distribution) Classification of normality tests: 1. Statistical - Kolmogrov-Smirnov test - Shapiro-wilk test Where to do normality 2. Graphical - Q-Q probability plots tests ? - box plots Before doing parametric tests on continuous data. Many statistical tests -including ANOVA, t- tests and regression- require the normality assumption: variables must be normally distributed in the population. However, the normality assumption is only needed for small sample sizes. 1. Mean/median represents the center of the distribution: If the mean of the data represents the center of the distribution, we can use the parametric test. When to use the if the median of the data represents the center of the distribution, we can use non-parametric test. parametric and non-parametric test 2. The population sample size: If a sample size is reasonably large, the applicable parametric test can be used. if the sample size is too small, the application of nonparametric tests is the only suitable option. 3. The analyzed data is ordinal or nominal: parametric tests can work only with continuous data Non-parametric tests can be applied to other data types such as ordinal or nominal data. Chi-Square Nonparametric Wilcoxon signed rank Tests Mann-Whitney U test Kruskal Wallis or H test Friedman ANOVA Spareman Rank correlation Parametric Non parametric Pearson correlation Spearman correlation Not Chi square Independent means test Mann-Whitney Dependent means- t-test Wilcoxon One way ANOVA Kruskal Wallis One-way repeated measures ANOVA, Friedman analysis of variance Flowchart Q&A