Repeated Measures ANOVA - PDF
Document Details
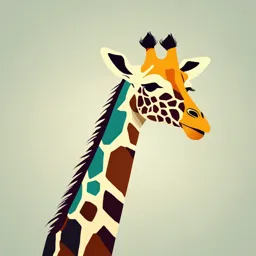
Uploaded by FondMonkey75
King Khalid University, Abha
Dr Noha Saleh
Tags
Related
- Statistiques L3 Sem1-Chapitre 3 Anova à Mesures Répétées-Partie 2 PDF
- Repeated Measures ANOVA Lecture PDF
- Psyc.242 Lecture 7.1 Repeated Measures Design PDF
- Psyc 242 Lecture 7.2 Repeated Measures Design PDF
- Repeated Measures Design PDF
- Lecture 7 - One Way, Factorial Repeated Measures and Mixed Factorial ANOVA PDF
Summary
This document presents lecture notes on Repeated Measures ANOVA. It covers the definition of repeated measures, examples of use cases, data organization, and important assumptions such as normality and sphericity, and how to run analysis in SPSS. It concludes with possible questions and data organization approaches.
Full Transcript
Repeated Measures ANOVA Dr Noha Saleh Associate professor of preventive medicine Taif program Objectives of lecture Understand what is meant by repeated measures Be able to set out data in required format Carry out simple analyses in SPSS Interpret the output Other Names Repeated measures ANOVA...
Repeated Measures ANOVA Dr Noha Saleh Associate professor of preventive medicine Taif program Objectives of lecture Understand what is meant by repeated measures Be able to set out data in required format Carry out simple analyses in SPSS Interpret the output Other Names Repeated measures ANOVA is also referred to as a • “Dependent groups” or • “ANOVA for correlated samples” What is a repeated measure? • A repeated measure is a variable measured two or more times, usually before, during and/or after an intervention or treatment. Measure is repeated over time (dependent, correlated or paired). • Repeated measures ANOVA gives the statistic tools to determine whether or not change has occurred over time. Examples of Repeated Measures Measuring cholesterol in a randomised controlled trial of a new statin at base line 3, 6 and 12 months Implementing weight loss intervention and measuring weight at different time points (baseline, after 1 w then after 2 w) Organisation of data (1) Generally each unit in one row and repeated measures in separate columns Unit 1 2 3 Score 1 2.8 5.6 4.3 Score2 3.1 5.7 4.1 Score3 4.1 5.1 5.4 Assumptions Variable It should be measured at the interval or ratio level (continuous), such as • Intelligence score • Exam test results • Weight Assumptions Cont... Normally distributed Dependent variable • The dependent variable between the two or more related groups should approximately normally distributed be Assumptions Cont... No significant outliers Data values that are "far away" from the main group of data •Distorting the differences between the related groups •Reduces the accuracy of results Assumptions Cont... Sphericity • Refers to differences between variances in levels of the repeatedmeasures factor • Violation of the assumption of sphericity, leads to an increase in the Type I error. • Mauchly's Test of Sphericity can help to test for this assumption (should be not significant p> 0.05) Violation of Assumptions — Normality assumption violation: • Regardless of scale of measurement • Nonparametric Version -> Friedman Test (Not covered) Hypothesis for RM ANOVA • The repeated measures ANOVA tests for whether there are any differences between related population means • H0: p1 = p2 = p3 = ... = pk • H0: There are no differences between population means. HA: At least one observation mean is significantly different from other. • Possible questions... • Overall, are there significant differences between time points? • Overall, are there significant changes from baseline? • The difference appear at which time points? Data are in the form of one row per subject: • If there is no control group, use a 1-way repeatedmeasures ANOVA • With a control group, use a 2-way repeated-measures ANOVA. Denominator of F ratio Repeated measure ANOVA table SS df Between within subjects error Total df between = a -1 ^I^/jSJI --& df within = N - a o/UJil j£ J'^' df subjects = S -1 ^^l —& df error = df within - df subjects df total = N -1 MS Examplel • example of a 2 weeks measurement of anxiety score after treatment where seven subjects had their scores level measured on three occasions: pre-, 1 week, and 2 weeks. Their data is shown below: Anxiety score before 9 Week 1 7 Week 2 4 . g Multivariate Tests r. [| Mauchl/sTestof: :. Tests DfWithin-Sc . 3 Tests ofWita-Si :. . Li Tests of Between- 0. it| Estimated Margin; . | Title fr^time . t Title i. L| Estimate 4 General Linear Model [DataSetl] C:' ,'lU3ers\pc\Desktop\repEated ANOYAkepeated ANOYA.sav Within-Subjects Factors Measure: MEASURE.! ;. . i|PaiMse ;. . [|Mullivaric Dependent time Variable 1 before 2 INI W2 Descriptive Statistics Mean Std. Deviation N before 8.14 .690 7 W1 6.71 .756 7 W2 3.00 .016 7 Multivariate Tests9 F Comment on mean for each time interval mean±SD Mauches Test of Sphericity Measure: MEASURE 1 Epsilonb Approx. Chi Square GreenhouseGeisser Huynh-Feldt Lower-bound df Within Subjects Effect Mauchly'sW / Sig \ time 2 \ W .750 1.438 .800 1.000 .500 Tests the null hypothesis that the error covariance matrix of the oithonormW transformed dependent variables is proportional to an identity matrix. :i Dr-i in: Intd^pt .'.itliiir ^il'jecri De-iiin:time P value not significant So sphericity assumed b. May be used to adjust the degrees of freedom for the averaged tests of significance. Corrected tests are displayed in the Tests ofWithin-Subjects Effectstable. a. Design: imercepi Within Subjects Design: time b. May be used to adjust the degrees of freedom forthe averaged tests of significance. Corrected tests are displayed in the T ofWithin-Subjects Effects table. Tests ofWithin-Subjects Effects Measure: MEASURE 1 Type III Sum of Squares Source time Errorftime) Mean Square df J— -Sjo^ Sphericity Assumed 93.667 2 49.333 Q22.000 Greenhouse-Geisser 98.667 1.600 61.667 222JJUU ------ *000 Huynh-Feldt 98.667 2.000 49.333 222.000 .000 Lower-bound 98.667 1.000 98.667 222.000 .000 Sphericity Assumed 2.667 12 .222 Greenhouse-Geisser 2.667 9.600 .278 Huynh-Feldt 2.667 12.000 .222 Lower-bound 2.667 6.000 .444 If sphericity assumed report F and p of first line (p value of Mauchly test not significant) If sphericity not assumed report F and p of second line (Greenhouse-Geisser) - ‘Output! [Document!]- IBM SPSS Statistics Viewer File Edit View Data Transform Insert Format Analyze ig meral Linear Model Title 1 Notes | Active Dataset | Within-Subjects Factors I Descriptive Statistics I Multivariate Tests g Mauchly's Test of Sphericity j Tests ofWithin-Subjects Effects | Tests ofWithin-Subjects Contras | Tests of Between-Subjects Effect | Estimated Marginal Means i. t Title i-1 time ]. . t Title I. . j Estimates + □ Pairwise Comparisons L. . j Multivariate Tests Direct Marketing Graphs Utilities Add-ons Window Help 1 6.714 .286 6.015 7.413 3 3.000 .309 2.245 3.755 Pairwise Comparisons Measure: MEASURE.! (I)lime (J) lime 1 2 3 2 3 Mean Difference (IStd. Error J) 1.429' .297 5.143' .261 95% Confidence Interval for Difference1 Sig? Lower Bound Upper Bound .009 .451 2.406 .000 4.285 6.000 1 -1.429' .297 .009 -2.406 -.451 3 3.714' .184 .000 3.108 4.321 1 ■5.143' .261 .000 ■6.000 ■4.285 2 ■3.714' .184 .000 ■4.321 ■3.108 Based on estimated marginal means ‘ The mean difference is significant at the .05 level, b. Adjustment for multiple comparisons: Bonferroni. Muttr/oiiate Tests Value F Hypothesis df Error df Sig. Comment on post hoc (pairwise comparison) □ X Effect size * Multivariate Tests3 Value F Hypothesis df Error df Effect factorl Pillai's Trace .991 290.000b 2.000 5.000 Wilks'Lambda .009 290.000b 2.000 5.000 Hotelling's Trace 116.000 290.000b 2.000 5.000 Roy's Largest Root 116.000 290.000b 2.000 5.000 3. Design. Intercept Within Subjects Design, factorl b. tat statistic Sig. .000 .000 .000 .000 Partial Eta Squared .991 .991 .991 Partial eta squared -denoted as n2- is the effect size n2 = 0.01 indicates a small effect; n2 = 0-06 indicates a medium effect; n2 = 0.14 indicates a large effect. Thanks