Introduction to Statistics: Statistics and Behavioral Sciences PDF
Document Details
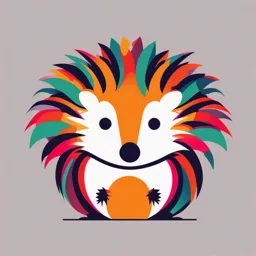
Uploaded by SharpestLimit5950
University of Rizal System
M. MASAYA
Tags
Summary
This document provides an introduction to statistics and its significance in the behavioral sciences. It covers key concepts like population, sample, variables, and the purpose of statistics in research. The document also highlights parameters, statistics, and the use of descriptive and inferential statistics.
Full Transcript
M. MASAYA CHAPTER 1: INTRODUCTION TO To study variables, measurements are taken, and the result for each individual is called a datum. A data set is a STATISTICS...
M. MASAYA CHAPTER 1: INTRODUCTION TO To study variables, measurements are taken, and the result for each individual is called a datum. A data set is a STATISTICS collection of these measurements or scores from individual STATISTICS AND BEHAVIORAL SCIENCES PARAMETERS AND STATISTICS Statistics can refer to specific facts and figures (like averages When describing data, it's important to specify whether the data or totals) or, in a broader sense, to a field of mathematics comes from a population or a sample. focused on analyzing and interpreting data. Parameter - is a characteristic that describes a In this context, statistics refers to the methods used to population. Example: The average score for the summarize and evaluate research results, especially in fields entire population is a parameter. like the behavioral sciences. Statistic - is a characteristic that describes a sample. Example: The average score for a sample PURPOSE OF STATISTICS IN RESEARCH of individuals is a statistic. 1. Organize and summarize data to help the researcher understand the results and communicate them clearly. PARAMETERS AND STATISTICS 2. Help answer research questions and draw valid conclusions Descriptive Statistics - are used to simplify, organize, and from the data summarize data in ways that make it easier to understand and interpret. These techniques focus on presenting the data in a STATISTICS PROVIDE TWO KEY BENEFITS clear and manageable form without making conclusions beyond 1. Present and interpret data in a clear an accurate way the data itself. 2. Offer standardized techniques that are recognized and Example: Summarizing data in tables and graphs understood by researchers worldwide. Inferential Statistics - uses sample data to make generalizations or inferences about a larger population. These In short, statistics help bring order to complex data and make it techniques allow researchers to draw conclusions, test understandable, ensuring that conclusions drawn from research are hypotheses, and make predictions about populations based on reliable and can be interpreted consistently by other researchers. the data from a sample. Example: "We are 95% confident that the true POPULATION AND SAMPLE population mean lies between 45 and 55." Population is the entire group that a researcher wants to study. A population can be large or specific. Populations can consist of This interval gives a sense of how precise the sample estimate is and anything, not just people (e.g., animals, companies, products). allows for uncertainty in generalizing from the sample to the Sample is a smaller, manageable group selected from the population. population for study. Samples are chosen to represent the population. SAMPLING ERROR AND MARGIN OF ERROR Because populations are often too large to study fully, A key concept in inferential statistics is sampling error, the researchers study a sample and then use the sample’s results to natural discrepancy that occurs between the sample statistic make conclusions about the entire population. and the population parameter. Margin of error reflects this uncertainty. For instance, in VARIABLES AND DATA political polling, a margin of error indicates how much the A variable is a characteristic or condition that can change sample estimate could differ from the true population value. A or have different values for different individuals. poll might report that Candidate X has 51 support, with a Examples of variables include weight, gender identity, margin of error of ±3%. This means the true support could personality, motivation, temperature, or time of day range from 48% to 54% M. MASAYA of children in a family or the number of students attending OBSERVATIONS, MEASUREMENT, AND VARIABLES class. If you observe class attendance from day to day, you may count 18 students one day and 19 students the next OBSERVATIONS AND MEASUREMENTS day. However, it is impossible ever to observe a value Science is empirical. This means it is based on observation between. 18 and 19. A discrete variable may also consist of rather than intuition or conjecture. Whenever we make a precise observations that differ qualitatively. For example, people observation we are taking a measurement, either by assigning a can be classified by birth order (first-born or later-born), numerical value to observations or by classifying them into by occupation (nurse, teacher, lawyer, etc.), and college categories. Observation and measurement are part and parcel of students can be classified by academic major (art, biology, the scientific method. In this section, we take a closer look at chemistry, etc.). In each case, the variable is discrete the variables that are being measured and the process of because it consists of separate, indivisible categories. measurement. CONSTRUCTS AND OPERATIONAL DEFINITIONS Constructs-Internal characteristics used to explain behavior (e.g., motivation, anxiety, hunger). These are intangible and cannot be directly observed. Hypothetical Constructs-Constructs like intelligence, self When measuring weight to the nearest whole pound, 149.6 esteem, and hunger, which are not directly observable. and 150.3 are assigned the value of 150 (top). Any value in Operational Definitions-Defines constructs by describing the interval between. 149.5 and 150.5 is given the value of observable behaviors. For example: 150. Self-esteem: Measured by Rosenberg For a continuous variable, there are an infinite number of Self-Esteem Scale. possible values that fall between any two observed values. Hunger: Measured by hours since last eating. A continuous variable is divisible into an infinite number of fractional parts. DISCRETE AND CONTINUOUS VARIABLE Suppose, for example, that a researcher is measuring The variables in a study can be characterized by the type of weights for a group of individuals participating in a diet values that can be assigned to them and, as we will discuss study. Because weight is a continuous variable, it can be in later chapters, the type of values influences the pictured as a continuous line (Figure 1.4). statistical procedures that can be used to summarize or Note that there are an infinite number of possible points on make inferences about those values. A discrete variable the line without any gaps or separations between consists of separate, indivisible categories. For this type of neighboring points. For any two different points on the line, variable. there are no intermediate values between two it is always possible to find a third value that is between adjacent categories. Consider the num- ber of questions the two points. Two other factors apply to continuous that each student answers correctly on a 10-item multiple variables. choice quiz. Between neighboring values-for example, Real limits are the boundaries of intervals for scores that seven correct and eight correct-no other values can ever are represented on a continuous number line. The real limit be observed. separating two adjacent scores is located exactly halfway A discrete variable consists of separate, indivisible between the scores. Each score has two real limits. The categories. No values can exist between two neighboring upper real limit is at the top of the interval, and the lower categories. real limit is at the bottom. Discrete variables are commonly restricted to whole, The concept of real limits applies to any measurement of a countable numbers (Le.. integers) for example, the number continuous variable, even when the score categories are M. MASAYA not whole numbers. For example, if you were measur- ing these scales are crucial because they highlight the time to the nearest tenth of a second, the measurement limitations of certain types of measurements and indicate categories would be 31.0. 31.1. 31 2, and so on. Each of which statistical procedures are appropriate. For example, these categories represents an interval on the scale that is categorizing people as tall, medium, or short gives a basic bounded by real limits. For example, a score of X 31.1 classification of height, but it does not provide enough seconds indicates that the actual measurement is in an detail to calculate averages or offer a precise interval bounded by a lower real limit of 31.05 and an measurement. To answer more detailed questions, more upper real limit of 31.15. Remember that the real limits are sophisticated measurements are necessary. always halfway between adjacent categories. THE NOMINAL SCALE: The nominal scale involves Later in this book, real limits are used for constructing classifying individuals into categories that have different graphs and for various calculations with continuous scales. names but are not numerically related. For example, For now, however, you should realize that real limits are a academic majors like art, biology, or business fall under necessity whenever you make measurements of a nominal categories. While the nominal scale helps continuous variable. determine whether individuals are different, it doesn’t Finally, we should warn you that the terms continuous and indicate the direction or size of the difference. For discrete apply to the variables that are being measured and instance, being an art major and a biology major shows not to the scores that are obtained from the measurement. they are different, but it doesn’t imply one is "more than" For example, measuringpeople's heights to the nearest inch the other. Other examples include classifying people by produces scores of 60, 61, 62, and so on. Although the race, gender, or occupation. Though nominal categories are scores may appear to be discrete numbers,the underlying often represented by numbers for coding purposes, these variable is continuous. One key to determining whether a numbers do not reflect any quantitative differences. variable is continuous or discrete is that a continuous THE INTERVAL AND RATIO SCALES: Both interval and variable can be divided into any number of fractional parts. ratio scales consist of ordered categories with equal Height can be measured to the nearest inch, the nearest intervals between them. Interval scales, like temperature in 0.5 inch, or the nearest (0,1 inch. Similarly, a professor Fahrenheit or Celsius, have intervals of the same size but evaluating students' knowledge could use a pass/fail an arbitrary zero point, meaning zero doesn't represent a system that classifies students into two broad categories. complete absence of the variable. In contrast, ratio scales, However, the professor could choose to use a 10-point quiz such as time in seconds or weight in pounds, have a true that divides student knowledge into 11 categories zero point, indicating the complete absence of the variable, corresponding to quiz scores from 0 to 10. Or the professor allowing for comparisons in terms of ratios. For example, a could use a 100-point exam that potentially divides student tank with 10 gallons of fuel has twice the amount of a tank knowledge into101 categories from 0 to 100. Whenever with 5 gallons. Ratio scales are commonly used in both you are free to choose the degree of precision or the physical sciences and behavioral sciences, like reaction number of categories for measuring a variable, the variable time or the number of errors on a test. must be continuous STATISTICS AND SCALE OF MEASUREMENT SCALES OF MEASUREMENT Scales of measurement are crucial because they determine Measurement involves assigning individuals or events to the statistical methods used to analyze data. Numerical categories, which can either be names (e.g., scores from interval or ratio scales allow for arithmetic introvert/extrovert) or numerical values (e.g., height in operations like addition and averaging, while nonnumerical inches or weight in pounds). These categories form a scale scores from nominal or ordinal scales do not. For example, of measurement, and the relationships between them recall scores can be added and averaged, but academic determine the type of scale used. The distinctions among majors, being non-numerical, cannot be added. Most M. MASAYA statistical techniques apply to numerical data, but for structures that also help to classify different research nominal or ordinal scales, alternative methods are required, methods and statistical techniques. such as the median, mode, Spearman correlation, and Data Structure 2. One Group with Two Variables Measured chi-square tests. for Each Individual: The Correlational Method In the correlational method, two different variables are THREE DATA STRUCTURES, RESEARCH METHODS, observed to determine whether there is a relationship AND STATISTICS between them. Descriptive research or the descriptive research strategy One method for examining the relationship between involves measuring one or more separate variables for each variables is to observe the two variables as they exist individual with the intent of simply describing the naturally for a set of individuals. That is, simply measure individual variables. the two variables for each individual. Some research studies are conducted simply to describe For example, research results tend to find a relationship individual variables as they exist naturally. For example, a between Facebook™ use and academic performance, college official may conduct a survey to describe the especially for freshmen (Junco, 2015). The researchers eating, sleeping, and study habits of a group of college then look for consistent patterns in the data to provide students. Although the researcher might measure several evidence for a relationship between variables. different variables, the goal of the study is to describe each For example, as Facebook time changes from one student variable separately. In particular, this type of research is to another, is there also a tendency for academic not concerned with relationships between variables. performance to change? TABLE 1.2: Three separate variables measured for each Figure 1.5 also shows the scores for the eight students in a individual in a group of eight students graph called a scatter plot. In the scatter plot, each individual is represented by a point so that the horizontal position corresponds to the student’s Facebook time and the vertical position corresponds to the student’s academic performance score. The scatter plot shows a clear relationship between Facebook time and academic performance: as Facebook time increases, academic When the results from a descriptive research study consist performance decreases. of numerical scores—such as the number of hours spent studying each day—they are typically described by statistical techniques. RELATIONSHIPS BETWEEN VARIABLES Most research, however, is intended to examine relationships between two or more variables. For example, is there a relationship between the amount of violence in the video games played by children and the amount of STATISTICS FOR THE CORRELATIONAL METHOD aggressive behavior they display? Is there a relationship When the data from a correlational study consist of between vocabulary development in childhood and numerical scores, the relationship between the two academic success in college? To establish the existence of variables is usually measured and described using a a relationship, researchers must make observations—that statistic called a correlation. Occasionally, the is, measurements of the two variables. The resulting measurement process used for a correlational study simply measurements can be classified into two distinct data M. MASAYA classifies individuals into categories that do not correspond to numerical values Limitations of the Correlational Method The results from a correlational study can demonstrate the existence of a relationship between two variables, but they do not provide an explanation for the relationship. In particular, a correlational study cannot demonstrate a When the measurement procedure produces numerical cause-and-effect relationship scores, the statistical evaluation typically involves Data Structure 3. Comparing Two (or More) Groups of computing the average score for each group and then Scores: Experimental and Nonexperimental Methods comparing the averages. The second method for examining the relationship between If the measurement process simply classifies individuals two variables compares two or more groups of scores. In into non-numerical categories, the statistical evaluation this situation, the relationship between variables is usually consists of computing proportions for each group examined by using one of the variables to define the and then comparing proportions. groups, and then measuring the second variable to obtain scores for each group. EXPERIMENTAL AND NONEXPERIMENTAL METHODS Statistics for Comparing Two (or More) Groups of Scores There are two distinct research methods that both produce Most of the statistical procedures presented in this book groups of scores to be compared: the experimental and the are designed for research studies that compare groups of nonexperimental strategies. These two research methods scores like the study in Figure 1.6. use exactly the same statistics and they both demonstrate For example, Polman, de Castro, and van Aken (2008) a relationship between two variables. The distinction randomly divided a sample of 10-year-old boys into two between the two research strategies is how the groups. One group then played a violent video game and relationship is interpreted. The results from an experiment the second played a nonviolent game. After the allow a cause-and-effect explanation gameplaying session, the children went to a free play THE EXPERIMENTAL METHOD period and were monitored for aggressive behaviors One specific research method that involves (hitting, kicking, pushing, frightening, name-calling, comparing groups of scores is known as the fighting, quarreling, or teasing another child). experimental method or the experimental Figure 1.6. The researchers then compared the scores for research strategy. The goal of an experimental the violent-video group with the scores for the nonviolent study is to demonstrate a cause-and-effect video group relationship between two variables. Specifically, For example, Polman, de Castro, and van Aken (2008) an experiment attempts to show that changing randomly divided a sample of 10-year-old boys into two the value of one variable causes changes to occur groups. One group then played a violent video game and in the second variable the second played a nonviolent game. After the game 1. Manipulation-the researcher playing session, the children went to a free play period and manipulates one variable by changing were monitored for aggressive behaviors (hitting, kicking, its value from one level to another. pushing, frightening, name-calling, fighting, quarreling, or 2. Control-the researcher must exercise teasing another child). control over the research situation to Figure 1.6. The researchers then compared the scores for ensure that other, extraneous variables the violent-video group with the scores for the nonviolent do not influence the relationship being video group examined. Control usually involves M. MASAYA matching different groups as closely as Control Conditions in an Experiment-often an experiment possible on those variables that we will include a condition in which the participants do not receive any don’t want to manipulate. experimental treatment. The scores from these individuals are then 3. Environmental Variables-these are compared with scores from participants who do receive the characteristics of the environment treatment. such as lighting, time of day, and Individuals in a control condition do not receive the weather conditions. A researcher must experimental treatment. Instead, they either receive no treatment or ensure that the individuals in they receive a neutral, placebo treatment. The purpose of a control treatment A are tested in the same condition is to provide a baseline for comparison with the environment as the individuals in experimental condition. treatment B. Individuals in the experimental condition do receive the 4. Participant Variables-these are experimental treatment. characteristics such as age, gender, motivation, and personality that vary NONEXPERIMENTAL METHODS: Nonequivalent from one individual to another Groups and Pre-Post Studies In informal conversation, there is a tendency for people to In the experimental method, one variable is manipulated use the term experiment to refer to any kind of research while another variable is observed and measured. To establish a study. You should realize, however, that the term applies cause-and-effect relationship between the two variables, an only to studies that satisfy the specific requirements experiment attempts to control all other variables to prevent them outlined earlier. In particular, a real experiment must from influencing the results. include manipulation of an independent variable and The individuals in a research study differ on a variety of rigorous control of other, extraneous variables. As a result, participant variables such as age, weight, skills, motivation, and there are a number of other research designs that are not personality. The differences from one participant to another are true experiments but still examine the relation known as individual differences. Terminology in Nonexperimental Research-in each Terminology in the Experimental Method-specific names case, one variable is used to create groups, and a second are used for the two variables that are studied by the experimental variable is measured to obtain scores within each group. In method. The variable that is manipulated by the experimenter is an experiment, the groups are created by manipulation of called the independent variable. It can be identified as the treatment the independent variable, and the participants’ scores are conditions to which participants are assigned. the dependent variable. The same terminology is often used The independent variable is the variable that is to identify the two variables in nonexperimental studies. manipulated by the researcher. In behavioral research, the That is, the variable that is used to create groups is the independent variable usually consists of the two (or more) treatment independent variable and the scores are the dependent conditions to which subjects are exposed. The independent variable is variable. manipulated prior to observing the dependent variable In a non experimental study, the “independent variable” The dependent variable is the one that is observed to that is used to create the different groups of scores is assess the effect of the treatment. The dependent variable is the often called the quasi-independent variable. variable that is measured in the experiment and its value changes in a way that depends on the status of the independent variable. An experimental study evaluates the relationship between two variables by manipulating one variable (the independent variable) and measuring one variable (the dependent variable). Note that in an experiment only one variable is actually measured M. MASAYA Proportion measures the fraction of the total group that is associated with each score. In Example 2.2, there were two individuals with X = 4. Thus, 2 out of 10 people had X= 4, so the proportion would be = 0.20. In general, the proportion associated with each score is CHAPTER 2: FREQUENCY DISTRIBUTION TABLE Because proportions describe the frequency (f) in relation to the total number (N), they often are called relative frequencies. Although proportions can be expressed as fractions FREQUENCY DISTRIBUTION TABLE Percentages can be included in a frequency distribution A frequency distribution is an organized tabulation of the table by adding a column headed with %. Example 2.4 demonstrates number of individuals located in each category on the scale of the process of adding proportions and percentages to a frequency measurement, it takes a disorganized set of scores and places them distribution table. 2.4 The frequency distribution table from Example in order from highest to lowest, grouping together individuals who all 2.2 is repeated here. This time we have added columns showing the have the same score. proportion (p) and the percentage (%) associated with each score. A frequency distribution can be structured either as a table or a graph, but in both cases, the distribution presents the same two elements: 1. The set of categories that make up the original measurement scale. 2. A record of the frequency, or number of individuals in each category. The simplest frequency distribution table presents the measurement scale by listing the different measurement categories (X values) in a column from highest to lowest. EXAMPLE: PERCENTILE AND PERCENTILE RANKS The percentile rank of a particular score is defined as the The following set of N=20 scores was obtained from a 10-point percentage of individuals in the distribution with scores at statistics quiz. We will organize these scores by constructing a or particular value. frequency distribution table When a score is identified by its percentile rank, the score is called a percentile. Scores: 8 9 8 7 10 9 6 4 9 8 CUMULATIVE FREQUENCY AND CUMULATIVE PERCENTAGE 7 8 10 9 8 6 9 7 8 8 The cumulative frequencies show the number of individuals located at or below each score. To find PROPORTIONS AND PERCENTAGES percentiles, we must convert these frequencies into M. MASAYA percentages. The result-in values are called cumulative REAL LIMITS AND FREQUENCY DISTRIBUTION percentages because they show the percentage of The concept of real limits also applies to the class intervals individuals who are accumulated as you move up the scale. of a grouped frequency distribution table. For example, a class interval of 40 49 contains scores from X = 40 to X = GUIDELINES IN CONSTRUCTING A FREQUENCY 49. These values are called the apparent limits of the DISTRIBUTION TABLE interval because it appears that they form the upper and There are several guidelines that help guide you in the lower boundaries for the class interval. If you are construction of a grouped frequency distribution table. Note that measuring a continuous variable, however, a score of X = these are simply guidelines, rather than absolute requirements, but 40 is actually an interval from 39.5 to 40.5. Similarly, X = they do help produce a simple, well-organized, and easily understood 49 is an interval from 48.5 to 49.5. Therefore, the real table. limits of the interval are 39.5 (the lower real limit) and GUIDELINE 1: The grouped frequency distribution table should have 49.5 (the upper real limit) about 10 class intervals. If a table has many more than 10 intervals, it becomes cumbersome and defeats the purpose of a frequency GRAPHS FOR INTERVAL OR RATIO DATA distribution table. On the other hand, if you have too few intervals, When the data consist of numerical scores that have been you begin to lose information about the distribution of the scores. At measured on an interval or ratio scale, there are two the extreme, with only one interval, the table would not tell you options for constructing a frequency distribution graph. anything about how the scores are distributed. Remember that the The two types of graphs are called histograms and purpose of a frequency distribution is to help a researcher see the polygons. data. With too few or too many intervals, the table will not provide a Histograms to construct a histogram, you first list the clear picture. You should note that 10 intervals is a general guide. If numerical scores or class intervals (the categories of you are constructing a table on a blackboard, for example, you measurement) along the X-axis. Then you draw a bar above probably want only 5 or 6 intervals. If the table is to be printed in a each X value so that scientific report, you may want 12 or 15 intervals. In each case, your a. the height of the bar corresponds to the goal is to present a table that is relatively easy to see and frequency for that category. understand. b. For continuous variables, the width of the bar extends to the real limits of the category. For GUIDELINE 2: The width of each interval should be a relatively discrete variables, each bar extends exactly half simple number. For example, 2, 5, 10, or 20 would be a good choice the distance to the adjacent category on each for the interval width. Notice that it is easy to count by 5s or 10s. side. These numbers are easy to understand because one can readily see EXAMPLE OF HISTOGRAM: how you have divided the range of scores. GUIDELINE 3: The bottom score in each class interval should be a multiple of the width. If you are using a width of 10 points, for example, the intervals should start with 10, 20, 30, 40, and so on. Again, this makes it easier for someone to understand how the table has been constructed. Polygons - The second option for graphing a distribution GUIDELINE 4: All intervals should be the same width. They should of numerical scores from an interval or ratio scale of cover the range of scores completely with no gaps and no overlaps, measurement is called a polygon. To construct a polygon, so that any particular score belongs in exactly one interval. you begin by listing the numerical scores (the categories of measurement) along the X - axis. Then: M. MASAYA A. A dot is centered above each score so that the In a symmetrical distribution, it is possible to draw a vertical position of the dot corresponds to the vertical line through the middle so that one side of the frequency for the category. distribution is a mirror image of the other (see Figure B. A continuous line is drawn from dot to dot to 2.12). connect the series of dots. In a skewed distribution, the scores tend to pile up toward one end of the scale and taper off gradually at the EXAMPLES OF POLYGONS: other end (see Figure 2.12). The section where the scores taper off toward one end of a distribution is called the tail of the distribution. A skewed distribution with the tail on the right-hand side is positively skewed because the tail points toward the positive (above-zero) end of the X-axis. If the tail points to the left, the distribution is negatively skewed (see Figure GRAPHS FOR NOMINAL OR ORDINAL DATA 2.12). When the scores are measured on a nominal or ordinal scale (usually non-numerical values), the frequency distribution can be displayed in a bar graph. Bar Graphs is essentially the same as a histogram, except that spaces are left between adjacent bars. For a nominal scale, the space between bars emphasizes that the scale consists of separate, distinct categories. EXAMPLE OF BAR GRAPH: STEM AND LEAF DISPLAYS A stem-and-leaf display is a method of organizing quantitative data to visualize its distribution while preserving individual data points. Introduced by John W. Tukeyin 1977, this technique offers an alternative to grouped frequency distributions. Constructing a Stem-and-Leaf Display Separate Each Score: GRAPHS FOR POPULATION DISTRIBUTIONS Divide each data point into a ‘stem’ (all but Stem And Leaf Relative Frequencies Sometimes samples are so large Displays the final digit) and a ‘leaf’ (the last digit). that reporting absolute frequencies does not sufficiently Example: For the number 85, the stem is 8, and the leaf is simplify the data. A common alternative is using relative 5. frequencies. List Stems: Write all possible stems in a vertical column, Smooth Curves When a population consists of numerical covering the range of the data set. Attach Leaves: For each scores from an interval or a ratio scale, it is customary to data point, place its leaf next to the corresponding stem. draw the distribution with a smooth curve instead of the jagged, step-wise shapes that occur with histograms and EXAMPLE: polygons. Stems Leaves THE SHAPE OF FREQUENCY DISTRIBUTION 3 3 M. MASAYA 4 2 6 5 2 7 6 3 8 7 1 4 6 8 1 3 5 9 3 7 INTERPRETING THE DISPLAY Distribution Visualization: The display shows the shape of the data distribution, similar to a histogram. Frequency Indication: The number of leaves next to each stem indicates the frequency of data points within that range. Data Retention: Unlike histograms, stem-and-leaf displays retain the original data values, allowing for precise CHAPTER 2: CENTRAL TENDENCY identification of individual scores. ADVANTAGES OVER GROUPED FREQUENCY DYSLEXIA DISTRIBUTIONS Dyslexia is a learning disability that affects language skills, Detail Preservation: Stem-and-leaf displays maintain especially reading and writing. Children with dyslexia might have individual data points, facilitating exact calculations and difficulty learning to read and problems with word and letter data recovery. interpretation. Today much reading is done on a screen, such as a Efficient Data Analysis: They provide a clear view of the computer monitor, tablet, or smartphone, rather than on a printed data’s distribution and frequency without losing specific paper page. One advantage of material presented on a computer information, which is beneficial for detailed statistical monitor, for example, is the ability to modify the background color analysis. and the color of the letters. Rello and Bigham (2017) conducted a study to examine By understanding and utilizing stem and leaf displays, one can reading speed in adults with and without dyslexia, varying the effectively organize and interpret data distributions, combining the background color on the screen. Participants read short passages clarity of graphical representation with the precision of raw data. with different pale-colored backgrounds, and their reading time and comprehension were measured. The results showed that participants with dyslexia were slower readers compared to those without dyslexia. However, both groups read faster with warm-colored backgrounds (peach, orange, yellow) and slower with cool-colored backgrounds (blue, blue-gray, green). These findings suggest that using warm-colored backgrounds on screens could make reading material more accessible for people with dyslexia. Warm background color: 11, 13, 15, 11, 12, 10, 14, 12, 10, 12 Cool background color: 17, 16, 18, 16, 15, 20, 17, 17, 20, 14 M. MASAYA the scale around X = 8, but tapering off to the left all the way down to X = 1. Where is the “center” in this case?Some people might select X 5 8 as the center because more individuals had this score than any other single value. However, X = 8 is clearly not in the middle of the distribution. In fact, the majority of the scores (10 out of 16) have values less than 8, so it seems reasonable that the “center” should be defined by a value that is less than 8. 3. 3. Now consider the third distribution [Figure 3.2(c)]. Again, the distribution is symmetrical, but now there are two distinct piles of scores. Because the distribution is CENTRAL TENDENCY symmetrical with X = 5 as the midpoint, you may choose X Central tendency is a statistical measure to determine a = 5 as the “center.” However, none of the scores is located single score that defines the center of a distribution. The goal of at X = 5 (or even close), so this value is not particularly central tendency is to find the single score that is most typical or good as a representative score. On the other hand, because most representative of the entire group there are two separate piles of scores with one group Central tendency aims to identify the “average” or “typical” centered at X = 2 and the other centered at X = 8, it is individual within a population or sample, providing a simple way to tempting to say that this distribution has two centers. But describe data. It is also useful for comparing groups or datasets. For can one distribution have two centers? example, weather data for Seattle and Phoenix highlights how central tendency can summarize large amounts of information with one "Center" of a distribution can be challenging, and while representative number. However, there’s no single, universal method some distributions have a clear center, others may lead to differing to determine central tendency, as different situations may require opinions. To address this, statisticians have created three methods to different measures. The example with three distributions shows how measure central tendency: the mean, median, and mode. Each is finding the most representative score is not always straightforward. calculated differently and works best in different situations. The goal is to identify the most representative score, and the choice of measure depends on the specific distribution being analyzed. Further discussion will explore which measure is best for each case. The mean, also known as the arithmetic average, is computed by adding all the scores in the distribution and dividing by the number of scores. The mean for a population is identified by the Greek letter mu, μ (pronounced “mew”), and the mean for a sample is identified by M or x̄ (read “x-bar”). The mean for a distribution is the sum of the scores 1. The first distribution [Figure 3.2(a)] is symmetrical, with divided by the number of scores. the scores forming a distinct pile centered around X = 5. For this type of distribution, it is easy to identify the The formula for the population mean is μ = ∑X/N “center,” and most people would agree that the value X = 5 is an appropriate measure of central tendency. First, add all the scores in the population, and then divide 2. In the second distribution [Figure 3.2(b)], however, by N. For a sample, the computation is exactly the same, but the problems begin to appear. Now the scores form a negatively skewed distribution, piling up at the high end of M. MASAYA formula for the sample mean uses symbols that signify sample values: sample mean = M = ∑X/N We use Greek letters to identify characteristics of a population (parameters) and letters of our own alphabet to stand for sample values (statistics). If a mean is identified with the symbol M, you should realize that we are dealing with a sample. Also note that the equation for the sample mean uses a lowercase n as the symbol for the number of scores in the sample. The mean is always located between the highest and lowest scores in a distribution, so it cannot be outside this range. For For the following population of N 5 4 scores, example, if the lowest score is 8 and the highest is 15, the mean 3, 7, 4, 6 must be between 8 and 15. If the calculated mean is outside this range, an error has occurred. The concept of the mean as a balance the mean is point, like a seesaw, helps in understanding how adding or removing μ = ∑X/N scores affects the mean. For instance, adding a score of 10 would = 20/4 change the mean, as explained further in Box 3.1. =5 Often it is necessary to combine two sets of scores and then find the overall mean for the combined group. Suppose, for Dividing the Total Equally The first alternative is to example, that we begin with two separate samples. The first sample think of the mean as the amount each individual receives when the has total (∑X) is divided equally among all the individuals (N) in the n = 12 scores and a mean of distribution. Consider the following example. M = 6. The second sample has A group of n = 6 children buys a box of baseball cards at a n = 8 and M = 7. If the two samples are combined, what is the mean garage sale and discovers that the box contains a total of 180 cards. for the total group? If the children divide the cards equally among themselves, how many cards will each child get? You should recognize that this problem represents the standard procedure for computing the mean. Specifically, the total ((∑X)) is divided by the number (n) to produce the mean, 180/6 = 30 cards for each child. The Mean as a Balance Point The second alternative definition of the mean describes the mean as a balance point for the distribution. Consider a population consisting of N = 5 scores (1, 2, 6, 6, 10). For this population, ∑X = 25 and m = 25/5 = 5. Figure 3.3 To calculate the overall mean, we need two values: shows this population drawn as a histogram, with each score 1. The overall sum of the scores for the combined group (∑X) represented as a box that is sitting on a seesaw. If the seesaw is 2. The total number of scores in the combined group (n) positioned so that it pivots at a point equal to the mean, then it will be balanced and will rest level. The total number of scores in the combined group can be found easily by adding the number of scores in the first sample (n1) and the number in the second sample (n2). M. MASAYA Remember that you also can determine the number of scores by adding the frequencies, n = ∑f. For the data Table 3.1 n = ∑f = 1 + 2 + 4 + 0 + 1 = 8 One you have found ∑X and n, you compute the mean as Steps to calculate the overall mean: usual. For these data 1. Add the sum of scores (∑X) for both groups. 2. Add the total number of scores (n) from both groups. 3. Use the formula to compute M. CHARACTERISTICS OF THE MEAN The following table summarizes the conclusion Every score in a distribution contributes to the total of (∑X) and the number of scores (n), both of which determine the mean. Changing a Score - Changing the value of any scores will change the mean.. EXAMPLE: a sample of quiz scores for a psychology lab section COMPUTING THE MEAN FROM A FREQUENCY consists of 9, 8, 7, 5 and 1. (note that the sample consist of n = 5 DISTRIBUTION TABLE scores with ∑X = 30). The mean for this sample is: Now suppose that the score of X = 1 is changed to X = 8. When a set of scores has been organized in a frequency (note that we have added 7 points to this individual’s score, which distribution table, the calculation of the mean is usually easier if you will also add 7 points to the total (∑X). After changing the score, the remove the individual scores from the table. (Table 3.1 shows a new distribution consist of distribution of scores organized in a frequency distribution table.) 9, 8, 7, 5, 8 The values in the table show that the distribution consist of one 10s, two 9s, four 8s, and one 6, for the total of n=8 scores There are still n = 5 scores, but now the total is ∑X = 37. Thus, the new mean is To find the sum of the scores, you must add all eight scores: ∑X= 10 + 9 + 9 + 8 + 8 + 8 + 8 + 6 = 66 Note that you can also find the sum of the scores by computing ∑fX as we demonstrated in Chap 2 pp. 47. For Introducing a New Score or Removing a Score - Adding or the data in Table 3.1 removing a score usually changes the mean unless the score is equal ∑X = ∑fX = 10+ 18 + 32 + 0 + 0 + 6 = 66 to the current mean. M. MASAYA The mean acts as a balance point, similar to a seesaw, and For Example: you multiply by 60; to change from inches to feet, you shifts when new scores are added or removed unless they divide by 12. match the mean. For Example: with n = 5, ∑X = 35, and M = 7, adding a score of 13 increases n to 6 and ∑X to 48, resulting in a new mean of M = 8. How a sample of n = 5 scores measured in inches would be transformed into a set of scores measured in centimeters. The first column shows the original scores that total ∑X = 50 with M = 10 inches. Second column, each of the original scores has been multiplied by 2.54 and the resulting values total ∑X = 127 with M = 25. 4 has also caused the mean to be multiplied by 2.54. EXAMPLE: We begin with sample of n = 5 scores with ∑X = 35 and THE MEDIAN M = 7. If one score with a value of X = 11 is removed from the sample, The median is the midpoint of a distribution, dividing what is the mean for the remaining scores? (you should obtain a scores into two equal halves. mean of M = 6) It is the point below which 50% of scores lie. The median has no special symbols and is identified as Adding or Subtracting a Constant from Each Score - When a "median." constant is added to or subtracted from every score in a dataset, the same constant will be added to or subtracted from the mean. Finding the Median: 1. Arrange scores in ascending order. For Example: n = 4 participants with a warm-color background, the 2. Identify the midpoint: total reading time ∑X is 48, resulting in a mean of M = 48/4 = 12. a. For odd numbers of scores, the median is the When using a cool-color background adds 3 seconds to each reading middle value. time, increasing total to ∑X = 60, so the mean is M = 60/4 = 15. b. For even numbers of scores, it is the point between the two middle values. Multiplying or Dividing Each Scores by a Constant - If every score in a distribution is multiplied by (or divided by) a constant FINDING THE MEDIAN FOR EVEN NUMBERS AND value, the mean will change in the same way. CONTINUOUS VARIABLES Multiplying or dividing each score by constant value is a common Median for Even Numbers method for changing the unit of measurement. Arrange scores in ascending order and identify the middle two scores. M. MASAYA Calculate the median as the average of the middle two values. Example: Scores :1,1,4,5,7,8 Middle scores: 4 and 5 In this example, one block is needed from an interval with four blocks, so the fraction of each block is 1/4. The median is The median is the first point beyond which 50% of scores halfway through the interval from 3.5 to 4.5. With the interval width lie. being 1 point, a fraction of 0.25 points is used. Adding 0.25 to 3.5 If there is a gap between the middle two scores (e.g., and), gives the median value of 3.75. This median divides the distribution the median is the midpoint. into two equal halves, with 50% of the data below and 50% above it. Median for Continuous Variable Interpolation is a method used to estimate a value Continuous variables can be divided into infinite fractional between two known values. For example, if a puppy weighs 10 pounds parts (e.g,, time in milliseconds). at 20 weeks and 20 pounds at 30 weeks, its weight at 25 weeks can When scores are continuous, the median is a precise point be estimated as 15 pounds, since 25 weeks is halfway between 20 that splits the distribution into two equal halves. and 30 weeks. Interpolation is useful when the median falls within a Example: Scores 1,2,3,4,4,4,4,6 range of tied scores. The process involves five steps to estimate an A discrete median is 4, but continuous scores cover intermediate value, helping to find values like the median in intervals (e.g., 4.00 is actually 3.5ân14.5) continuous data. The precise median is calculated using the distribution's histogram and lies within the interval that includes the Step 1: Determine how many scores should fall above and below the middle values. median by taking one-half of N, or 0.5N. Step 2: Count the number of scores (or blocks in the graph) below the lower real limit of the tied values. The notation for this frequency is fBELOW LRL. STEP 3: Find the number of additional scores (blocks) needed to make exactly one-half of the total distribution, 0.5N 2 fBELOW LRL Step 4: To determine what fraction of the tied scores fall below the median, divide Step 3 by the number of tied scores, fTIED The distribution has several scores that are grouped around the median, which is placed in such a way that the scores are evenly divided. The median splits the distribution so that half of the scores are on one side and half are on the other. This results in an equal number of scores on both sides of the median, representing 50% of STEP 5: Add the fraction (Step 4) to the lower real limit, XLRL, of the data. the interval containing the tied scores. The goal is to reach 50% of the data, represented by four blocks. By 3.5 on the X-axis, three blocks are accumulated. To complete the four blocks, one more is needed, but the next interval has four blocks. The solution is to take a fraction from each block, like a quarter, so that the fractions combine to form a full block. This approach allows reaching 50%, with the fraction determined by the required and available blocks in the interval. M. MASAYA balancing the total distance above and below it. While both the mean and the median measure central tendency, they use different definitions of what it means to be "in the middle." CENTRAL TENDENCY Bureau of Labor Statistics (2017). Bar graph used to present means and medians when the groups or treatment shown on the horizontal axis are measured on a nominal or an ordinal scale. To Remember, finding the precise midpoint by dividing scores construct a bar graph, you simply draw a bar directly above each into fractional parts is sensible for a continuous variable; however, it group or treatment so that the height of the bar corresponds to the is not appropriate for a discrete variable. For example, a median time mean (or median) for that group or treatment. For a bar graph, a of 3.75 seconds is reasonable, but a median family size of 3.75 space is left between adjacent bars to indicate that the scale of children is not. measurement is nominal or ordinal. In figure 3.12, the type of teaching position is a nominal scale of measurement consisting of a THE MEDIAN, THE MEAN, AND THE MIDDLE distinct category. The mean is defined as the "balance point" of a distribution, meaning the total distance above the mean equals the total distance When constructing graphs of any type, you should recall the basic we below it. As a result, the mean is always located between the introduced in Chapter 2, page 55: smallest and largest scores. However, the mean may not always be at the exact center of the scores, especially in distributions where most 1. The height of a graph should be approximately two-thirds of the scores are on one side. For example, in a distribution with 6 to three-quarters of its length. scores, if 5 out of 6 scores are below the mean, the mean still 2. Normally, you start numbering both the X-axis and Y-axis balances the distances, but it is not at the center of the scores. This with zero at the point where the two axes intersect. highlights that the mean focuses on distances rather than the actual However when a value of zero is part of the data, it is position of scores. common to move the zero point away from the intersection so that the graph does not overlap the axes (figure 3.11). Following these rules will help produce a graph that provides an accurate presentation of the information in a set of data. Although it is possible to construct graphs to distort the results of a study (see In a population with 6 scores and a mean of 4, the mean box 2.1), researchers have an ethical responsibility to present an does not always divide the scores into two equal groups. In this case, honest and accurate report of their research report. 5 out of the 6 scores are less than the mean. This shows that while the mean represents the balance point of the distribution, it doesn’t necessarily split the scores into two even halves. The key point is that the mean is based on distances, not the exact distribution of individual scores. The median defines the middle of a distribution in terms of the actual scores, ensuring that half of the scores are above it and THE MODE half are below it. For example, in the distribution shown in Figure 3.6, The final measure of central tendency that we will consider the median is at X=2.5, with 3 scores above and 3 scores below. This is called the mode. In its common usage, the word mode means “the makes the median a true "middle" when defined by the number of customary fashion” or “a popular style.” The statistical definition is scores. In contrast, the mean defines the middle based on distances, M. MASAYA similar in that the mode is the most common observation among a simply identify the score located directly beneath the highest point group of scores. in the distribution. In a frequency distribution, the mode is the score or Although a distribution will have only one mean and only category that has the greatest frequency. one median, it is possible to have more than one mode. Specifically, it As with the median, there are no symbols or special is possible to have two or more scores that have the same highest notation used to identify the mode or to differentiate between a frequency. In a frequency distribution graph, the different modes will sample mode and a population mode. In addition, the definition of the correspond to distinct, equally high peaks. A distribution with two mode is the same for a population and for a sample distribution. modes is said to be bimodal, and a distribution with more than two The mode is a useful measure of central tendency because modes is called multimodal. Occasionally, a distribution with several it can be used to determine the typical or most frequent value for equally high points is said to have no mode. any scale of measurement, including a nominal scale (see Chapter 1). Incidentally, a bimodal distribution is often an indication Consider, for example, the data shown in Table 3.4. These data were that two separate and distinct groups of individuals exist within the obtained by asking a sample of 100 students to name their favorite same population (or sample). For example, if you measured height for restaurants in town. The result is a sample of n 5 100 scores with each person in a set of 100 college students, the resulting each score corresponding to the restaurant that the student named. distribution would probably have two modes, one corresponding For these data, the mode is Luigi’s, the restaurant (score) primarily to the males in the group and one corresponding primarily that was named most frequently as a favorite place. Although we can to the females. identify a modal response for these data, you should notice that it Technically, the mode is the score with the absolute would be impossible to compute a mean or a median. Specifically, highest frequency. However, the term mode is often used more you cannot add restaurants to obtain SX and you cannot list the casually to refer to scores with relatively high frequencies—that is, scores (named restaurants) in order. scores that correspond to peaks in a distribution even though the The mode also can be useful because it is the only measure peaks are not the absolute highest points. For example, Sibbald of central tendency that must correspond to an actual score in the (2014) looked at frequency distribution graphs of student data; by definition, the mode is the most frequently occurring score. achievement scores for individual classrooms in Ontario, Canada. The The mean and the median, on the other hand, are both calculated goal of the study was to identify bimodal distributions, which would values and often produce an answer that does not equal any score in suggest two different levels of student achievement within a single the distribution. For example, in Figure 3.6 (page 89) we presented a class. distribution with a mean of 4 and a median of 2.5. Note that none of For this study, bimodal was defined as a distribution having the scores is equal to 4 and none of the scores is equal to 2.5. two or more significant local maximums. Figure 3.7 shows a However, the mode for this distribution is X 5 2 and there are three distribution of scores that is similar to a graph presented in the individuals who actually have scores of X 5 2. study. There are two distinct peaks in the distribution, one located at X 5 17 and the other located at X 5 22. Each of these values is a mode in the distribution. Note, however, that the two modes do not have identical frequencies. Seven students had scores of X 5 22 and only six had scores of X 5 17. Nonetheless, both of these points are called modes. When two modes have unequal frequencies, researchers occasionally differentiate the two values by calling the taller peak the major mode, and the shorter one the minor mode. By the way, the author interpreted a bimodal distribution as a suggestion for the teacher to In a frequency distribution graph, the greatest frequency consider using two different teaching strategies; one for the high will appear as the tallest part of the figure. To find the mode, you achievers and one designed specifically to help low-achieving students. M. MASAYA same frequency. Still, the mean and the median are in the center of the distribution. CENTRAL TENDENCY AND THE SHAPE OF THE b. Skewed distribution DISTRIBUTION - In skewed distributions, especially a. Symmetrical Distribution distributions for continuous variables, there is a - For a symmetrical distribution, the right-hand strong tendency for the mean, median, and mode side of the graph is a mirror image of the to be located in predictably different positions. left-hand side. If a distribution is perfectly Figure 3.9(a), for example, shows a positively symmetrical, the median is exactly at the center skewed distribution with the peak (highest because exactly half of the area in the graph will frequency) on the left-hand side. This is the be on either side of the center. The mean also is position of the mode. However, it should be clear exactly at the center of a perfectly symmetrical that the vertical line drawn at the mode does not distribution because each score on the left side divide the distribution into two equal parts. To of the distribution is balanced by a have exactly 50% of the distribution on each corresponding score (the mirror image) on the side, the median must be located to the right of right side. As a result, the mean (the balance the mode. Finally, the mean is typically located to point) is located at the center of the distribution. the right of the median because it is influenced Thus, for a perfectly symmetrical distribution, most by the extreme scores in the tail and is the mean and the median are the same [Figure displaced farthest to the right toward the tail of 3.8(a)]. If a distribution is roughly symmetrical, the distribution. Therefore, in a positively skewed but not perfect, the mean and median will be distribution, the most likely order of the three close together in the center of the distribution. measures of central tendency from smallest to - If a symmetrical distribution has only one mode, largest (left to right) is the mode, the median, it will also be in the center of the distribution. and the mean. Thus, for a perfectly symmetrical distribution - Negatively skewed distributions are lopsided with one mode, all three measures of central in the opposite direction, with the scores piling tendency—the mean, the median, and the up on the right-hand side and the tail tapering off mode—have the same value. For a roughly to the left. The grades on an easy exam, for symmetrical distribution, the three measures are example, tend to form a negatively skewed clustered together in the center of the distribution [see Figure 3.9(b)]. For a distribution distribution. On the other hand, a bimodal with negative skew, the mode is on the distribution that is symmetrical [see Figure right-hand side (with the peak), while the mean 3.8(b)] will have the mean and median together is displaced toward the left by the extreme in the center with the modes on each side. A scores in the tail. As before, the median is usually rectangular distribution [see Figure 3.8(c)] has located between the mean and the mode. no mode because all X values occur with the Therefore, in a negatively skewed distribution, the most probable order for the three measures M. MASAYA of central tendency from smallest value to largest value (left to right), is the mean, the median, and the mode. Open-ended distributions A distribution is said to be open-ended when there is no upper limit (or lower limit) for one of the SELECTING A MEASURE OF CENTRAL TENDENCY categories. The table in the margin of the next page provides an example of an open-ended distribution, WHEN TO USE THE MEDIAN showing the number of pizzas eaten during a one-month The median is best used when the data has extreme values period for a sample of n = 20 high school students. or is skewed, as it is not affected by outliers. Unlike the mean, the median provides a more accurate representation of the central tendency in such situations, making it ideal for reporting typical values in datasets like income or other skewed distributions. Extreme Scores or Skewed Distributions The median is a better measure of central tendency when data has extreme values or is skewed. Unlike the mean, the median is not influenced by extremely high or low values. Example: The distribution of n = 10 scores in Figure 3.10. For this sample, the mean is Undetermined Values In cases where some values are unknown or undetermined, calculating the mean becomes impossible. The median is still valid because it is determined by the order of the data, not the actual numerical values. Ordinal Scales Example: Measuring the time preschool children The median is best for ordinal data as it reflects take to complete a puzzle when some children fail to finish. order without needing exact distances, unlike the mean, The median can provide a central value, whereas the mean which relies on measurable differences. Ordinal scales cannot be computed with missing data. show direction but not magnitude, making the median more appropriate. M. MASAYA Psychological Association (APA), as outlined in the WHEN TO USE THE MODE Publication Manual of the American Psychological Outlines cases where the mode is most suitable for Association. For the mean, the APA has recommended that describing central tendency, particularly for nominal data, discrete researchers use the letter M as the symbol for the sample variables, or understanding the distribution's shape. mean. Example: The treatment group showed fewer NOMINAL SCALES errors (M = 2.56) on the task than the control The mode is advantageous for nominal data, group (M = 11.76). which consists of categories distinguished only by name (e.g., occupations or majors). Median: The median can be reported using the Nominal scales cannot be used to calculate the abbreviation Mdn, as in "Mdn = 8.5 errors", or it can simply mean or median because they do not measure quantities. be reported in narrative text. Therefore, the mode is the only option for Example: The median number of errors for the describing central tendency in nominal data, although it is treatment group was 8.5, compared to a median not preferred for interval or ratio scales. of 13 for the control group. Mode: There is no special symbol or convention for DISCRETE VARIABLES reporting the mode. If mentioned at all, the mode is usually Discrete variables exist in whole, indivisible just reported in narrative text. categories (e.g., the number of children in a family or rooms in a house). Using the mean with such data may produce unrealistic fractional values (e.g., 2.4 children). The mode provides sensible and realistic central tendency values, such as "2 children" or "5 rooms," making it more appropriate in many cases. PRESENTING MEANS AND MEDIANS IN GRAPH Graphs are used to compare measures of central tendency, DESCRIBING SHAPE typically showing sample means and occasionally medians. They The mode is often included as a supplementary measure allow multiple values to be displayed for easy comparison across alongside the mean or median. groups or conditions. Independent variables are listed on the It identifies the most frequent value(s) in a dataset and horizontal axis, dependent variables on the vertical axis, and data is highlights peaks in a frequency distribution. represented using line graphs, histograms, or bar graphs, depending This is useful for understanding the distribution's shape and central on the measurement scale. patterns. REPORTING MEASURE OF CENTRAL TENDENCY Measures of central tendency are commonly used in the behavioral sciences to summarize and describe the results of a research study. For example, a researcher may report the sample means from two different treatments or the median score for a large sample. These values may be reported in text, included in tables, or presented in graphs. Five drug dose levels are represented as points, with