PSYC21061 Module 1 Handout PDF
Document Details
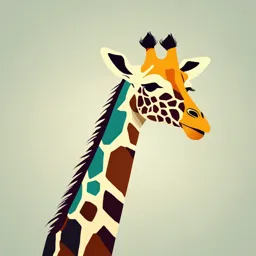
Uploaded by BlissfulOnyx2600
University of Manchester
Tags
Summary
This document is a handout for module 1 of the PSYC21061 course. It covers various topics in statistics, including scales of measurement, different research aims, and experimental designs. The handout is designed to help psychology students understand these fundamental concepts involved in psychological research.
Full Transcript
Module 1a: Which statistic? By the end of this VL, you will – be able to determine the scale of measurement – recognise broad research aims – recognise experimental designs – appreciate the properties of normally distributed data, and variations from it...
Module 1a: Which statistic? By the end of this VL, you will – be able to determine the scale of measurement – recognise broad research aims – recognise experimental designs – appreciate the properties of normally distributed data, and variations from it 1 Which statistic? Choice of statistic, or statistical test, depends on: – Scale of measurement – Research aims Descriptive only Relational (relationships) Experimental (differences) – Experimental design – Subjects design: between or within – Number of independent variables (IVs) – Number of IV levels – Properties of dependent/outcome variable Normally distributed: Parametric 2 Not normally distributed: Non-Parametric Scales of Measurement Nominal Categorical numbers or names serve as labels but no numerical relationship between values e.g. gender, political party, religion Ordinal data is organised by rank values represent true numerical relationships but intervals between values may not be equal e.g. race position, likert scale ratings Continuous Discrete or Interval true numerical relationships and intervals between values are equal but scale has not true zero point e.g. temperature (ºF), shoe size Ratio true numerical relationships, equal intervals and true zero point e.g. height, distance Research Aim: Describe Descriptive statistics: summarise a set of sample values Typically use just two statistics – central tendency – spread Measure of Mean Median Mode central tendency Measure of Standard deviation Range spread Discrete or Discrete or continuous data continuous data When? which is not Categorical data which is normally normally distributed distributed 4 Research Aim: Infer relationships Relational research explore relationship between observed behaviours or phenomena – Nothing is actively manipulated We can describe those relationships and make predictions – But we can’t infer causality exam results hours revision 5 Research Aim: Infer differences Experimental research examines the influence of one or more variables (IVs) on other variables (DVs) Can make claims about causality IF we have controlled for confounding variables – using random allocation, counterbalancing etc. – NB not always possible (e.g. quasi-experimental designs) 100 120 Is there a 80 100 difference? 60 80 Score 40 60 20 40 0 20 0 1 6 2 3 4 5 6 7 8 9 10 Experimental Designs: Variables Independent variable(s): hypothesised to influence the DV – a.k.a. factor(s) – e.g. drug treatment group, age, etc. – always measured on categorical scale Dependent variable: hypothesised to be ‘dependent’ on the IV – a.k.a. the outcome – e.g. test score, reaction time, etc. – ideally measured on discrete or continuous scale We measure the DV under different levels of the IV – In order to ascertain the effect of the IV 7 Independent Variables: Levels Independent variables have at least 2 levels – True-experimental IVs IVs are actively manipulated random allocation is possible (can make claims about causality) e.g. sport context (2 levels: solo, competitive) e.g. treatment group (3 levels: placebo, drugs, counselling) – Quasi-experimental IVs IV reflects fixed characteristics random allocation is not possible (must be cautious about implying causality) e.g. handedness (2 levels: right, left) 8 e.g. age (3 levels: 18-20yrs, 20-22yrs, 22-24yrs) Independent Variables: Subjects Design Subjects design: distribution of participants across IV levels Between-subjects (Independent Groups): – participants exposed to only one IV level – e.g. intervention vs. control group – e.g. teachers vs. accountants vs. nurses Within-subjects (Repeated Measures): – participants exposed to all IV levels – e.g. sober vs. drunk – e.g. at the end of Year 1, Year 2 and Year 3 of Uni Mixed designs: – at least one IV is between subjects AND at least one IV is within subjects 9 ID the Experimental Design IV; IV levels; DV; design; true/quasi? An investigator is interested in whether right and left-handed people differ in their performance on different types of computer-games (his theory is that left-handed people will have an advantage on games requiring better visuo-spatial skills). He measures the scores of 30 right handers and 30 left handers who each play Daley Thompson’s Decathlon, Horace Goes Skiing & Tetris IV: IV: Levels: Levels: Subjects design: True/Quasi: Subjects design: DV: Experimental design: 10 Experimental Designs: Summary Experimental: Tests of Differences 2 IVs 1 IV (Factorial Designs) Between Ps: 2 levels >2 levels 2-way Independent ANOVA Between Ps: Between Ps: Within Ps: Independent t-test 1-way Independent 2-way Repeated ANOVA Measures ANOVA Within Ps: Mixed Design: Within Ps: 1-way Repeated 2-way Mixed 11 Paired t-test Measures ANOVA ANOVA Properties of Data: Normally Distributed Properties of normal distribution Symmetrical about the mean Bell shaped 12 Kurtosis Mesokurtic Leptokurtic: small s.d. Platykurtic: large s.d. (+ve kurtosis value) (-ve kurtosis value) 13 Skew No skew Positive skew Negative skew 14 Properties of Data: Other Non-Normal Distributions Uniform Bimodal 15 Such data can not be considered normally distributed i.e. parametric statistics are not appropriate Module 1b: Drawing Inference z-scores By the end of this VL, you will – understand that we use statistics to draw inference about populations – recognise the properties of normally distributed data – be able to convert raw scores to z-scores – be able to determine the proportion of scores falling above or below a given score drawn from a normally distributed population 16 Using statistics to draw inference Goal: To say something about a population The truth: population parameters µ = 55.86; σ = 5.04 The estimate: sample statistics 𝑥ҧ = 56.41; s = 8.19 Use sample statistics to infer population parameters Sampling Error: degree to which sample statistics differ from underlying population parameters Minimising error – sample must be: – Representative (randomly selected) – Sufficient in size 17 The Normal Distribution 18 Z-scores Scores from a normally distributed population can be converted to z-scores 𝑥−𝜇 𝑧= 𝜎 Examples – Score of 118 is converted to z- score of 1.8 =0 – Score of 104 is converted to z- =1 score of 0.4 – Score of 96 is converted to z- frequency score of -0.4 118−100 𝑧 𝑧== 96−100 ==.04 104−100 1.8 -.04 10 10 19 z Standard Normal Distribution Percentage of scores within single standard deviation boundaries 2.14 13.59 34.13 34.13 13.59 2.14 -3 σ -2 σ -1 σ 0 +1σ +2 σ +3 σ 20 70 80 90 100 110 120 130 Standard Normal Distribution 95% of values lie within ±1.96 standard deviations of the mean 1.96 below 1.96 above 100 − 1.96 ∗ 10 = 80.4 100 + 1.96 ∗ 10 = 119.4 𝜇 − (1.96𝜎) 𝜇 + (1.96𝜎) 80.4 119.6 -3 σ -2 σ -1 σ 0 +1σ +2 σ +3 σ 21 70 80 90 100 110 120 130 Table of z-scores A small selection of values from the table of z-scores provided in the Dancey & Reidy textbook (Appendix 1) z-score Proportion Proportion below score above score 0.00 0.5000 0.5000 1.00 0.8413 0.1587 1.65 0.9505 0.0495 1.96 0.9750 0.0250 2.00 0.9772 0.0228 3 0.9987 0.0013 22 Module 1c: Sampling Distributions By the end of this VL, you will – be familiar with the concept of sampling distributions – appreciate how the sampling distribution of the mean relates to the underlying population distribution – be able to calculate the standard error (and the estimated standard error) – appreciate how the standard error can be used to quantify sampling error 23 Sampling Distributions Sample scores Sample scores Sample scores ഥ = 𝟓. 𝟖𝟏 𝒙 ഥ = 𝟔. 𝟎𝟐 𝒙 ഥ = 𝟔. 𝟏𝟔 𝒙 If the mean of each sample is plotted, over Sampling Distribution: infinite samples, they distribution of a statistic form a normal across an infinite distribution. number of samples (e.g. The mean of the sampling distribution of sampling distribution of the mean) the mean is equivalent 𝝁 = 𝟓. 𝟗𝟖 to the population mean. Sampling Distribution of the Mean Plotting all possible sample means gives us the sampling distribution of the mean (SDM) SDM is normally distributed The SDM’s mean is equivalent to the population mean The SDM’s standard deviation is given a special name – the standard error Standard Error The standard error (SE): the standard deviation of the sampling distribution The standard error is related to , but is also a function of sample size 𝜎 𝑆𝐸 = 𝑛 The standard error decreases as sample size increases – Reflecting fact that sampling error decreases as sample size increases Estimated Standard Error Sampling distributions are theoretical distributions – We never know the real standard error, instead we estimate it Estimated standard error (ESE): an estimate of the standard error, based on our sample s ESE = n Sampling Distribution of the Mean Sampling Distribution of the Mean Mean equivalent to the population mean Standard deviation is the ‘standard error’ Always normally distributed – So can apply the logic of the SND – e.g. 95% of all sampled means will fall within ±1.96 standard errors of the population mean 95% The Sampling Distribution of the mean 95% of all sampled means will fall within the 95% bounds of the population mean (i.e. μ ± 1.96 SE) Sampled means Module 1d: Confidence Intervals By the end of this VL, you will be able to define a confidence interval appreciate the value of stating confidence intervals around sample statistics be familiar with the calculation of the 95% confidence interval around a sample mean 31 Confidence Intervals We use statistics to estimate population parameters – 𝑥ҧ is a single point estimate of – Estimates are subject to sampling error Confidence Intervals (CIs) are interval estimates of population parameters – e.g. “we have 95% confidence that the population mean lies between __ and __” Note, a CI doesn’t express the probability of the population parameter falling within a given interval – Only our level of confidence that the population parameter falls within that interval 32 The Sampling Distribution of the mean 95% of all sampled means will fall within the 95% bounds of the population mean (i.e. μ ± 1.96 SE) Sampled means 33 The Sampling Distribution of the mean 95% of all sampled means will fall within the 95% bounds of the population mean (i.e. μ ± 1.96 SE) Sampled means with 95% CIs By the same logic, the population mean will be captured by 95% of the 95% CIs of sampled means (and missed by 5% of the 95% CIs of sampled means) 34 Calculating Confidence Intervals 95% of all sample means (𝑥)ҧ fall within 95% bounds of the population mean () 95% bounds of the population mean: 𝜇 ± 1.96𝑆𝐸 How do we calculate the 95% CIs around a sample mean? PAUSE 35 Calculating Confidence Intervals 95% of all sample means (𝑥)ҧ fall within 95% bounds of the population mean () 95% bounds of the population mean: 𝜇 ± 1.96𝑆𝐸 How do we calculate the 95% CIs around a sample mean? Following SDM logic, reasonable (but wrong) answer would be: 95% CIs around the sample mean: 𝑥ҧ ±1.96𝐸𝑆𝐸 – ESE because we don’t know 36 Calculating Confidence Intervals The (reasonable but) wrong answer: 95% CIs around the sample mean are calculated as: 𝑥ҧ ±1.96𝐸𝑆𝐸 – (ESE because we don’t know ) Why this is wrong – Figure of 1.96 based on z-distribution (which relates to populations) – Without knowledge of the population distribution, we use the t-distribution (which relates to samples) 37 Calculating Confidence Intervals – t-distribution Normally distributed Spread of scores varies according to sample size – With larger samples, spread is smaller – To calculate the 95% CIs around a sample mean? Look for critical value of t where 2.5% of scores are higher/lower (t0.975) – 95% CIs around 𝑥ҧ are calculated as: 𝑥ҧ ± t0.975 * ESE – N.B. where n>1000, t0.975 = 1.96 – (just like the z-distribution) 38 Module 1e: Hypothesis Testing By the end of this VL, you will – be able to articulate a null hypothesis – be able to interpret a p-value – recognise the errors that we can encounter using p to evaluate a null hypothesis Hypothesis Testing Null hypothesis (H0): there is no difference between the population means Always start by assuming the null hypothesis is true If we find a difference between the sample means, we ask: – What is the chance of measuring a difference of that magnitude if the null hypothesis is true? p-values What is the chance of measuring a difference of that magnitude if the null hypothesis is true? p-value: the probability of measuring a difference of that magnitude if the null hypothesis is true (alpha): threshold level of probability where we will be willing to reject the null hypothesis – In Psychology we typically set α =.05 If p ≤ reject the hull hypothesis – i.e. reject null hypothesis if p ≤.05 p-values What is the chance of measuring a difference of that magnitude if the null hypothesis is true? impossible certain α 0.05 1 If the probability (p) is less than our threshold level (), we reject the null hypothesis If the probability (p) is greater than our threshold level (), we fail to reject the null hypothesis – NB we don’t ‘accept’ the null hypothesis (it still might not be true), but we don’t have strong enough evidence to reject it Error the truth Null Hypothesis Null Hypothesis True False Reject Type I Error Correct α decision we make Null Hypothesis Fail to Reject Type II Error Correct Null Hypothesis Module 1f: Introduction to SPSS By the end of this VL, you will – be familiar with the SPSS interface – be able to input data to SPSS, according to the subjects design – be able to generate descriptive statistics and a histogram (a.k.a. a frequency distribution) 45 Data View Window Variables Participants Tab Every participant gets his/her own row, and all the 46 data related to that participant goes in their row!! Variable View Window Variables Define the attributes of your variables Tab Attributes include: Name (something short but meaningful) Label (expansion of name) Values (numbers assigned to categorical data) Measure: Scale (interval/ratio), Ordinal or Nominal (categorical) Ignore the final column (Role) 47 Example 1 A researcher is interested to explore the impact of background music on audiences’ enjoyment of a film. They ask 20 participants to rate the film on two occasions; with background music and without background music – IV? presence of music – Levels? 2: music / no music – Subjects Design? Within-subjects (repeated measures) – Dependent Variable? Enjoyment rating 48 Setting Up Variables: Repeated Measures In Variable View Enter names for your variables (no gaps) Give them labels Ensure you’ve indicated that they represent ‘scale’ data 49 Inputting Data: Repeated Measures 35.00 29.00 39.00 31.00 Tab to Data View You’ll see your variable columns have been labelled Enter data for each participant (under each condition) 50 Example 2 A researcher is interested to explore the impact of background music on audiences’ enjoyment of a film. They ask 10 participants to rate the film with background music and 10 participants to rate the film without background music – IV? presence of music – Levels? 2: music / no music – Subjects Design? Between-subjects (independent grp) – Dependent Variable? Enjoyment rating N.B. Data entry differs according to the subjects 51 design Why is this not correct for independent groups? Every participant gets his/her own row, and all the data related to that participant goes in their row!! 52 Setting Up Variables: Independent Groups 53 Inputting Data: Independent Groups 1.00 22.00 1.00 35.00 Tab to Data View You’ll see your variable columns have been labelled 2.00 26.00 Identify the group each participant was in Enter their rating Check value labels 54 Data layout Within-Subjects (Repeated Measures) Each level of the IV Between-Subjects (Independent Groups) DV The IV (grouping variable) 55 Obtaining Descriptive Statistics Analyse Descriptive Statistics Explore… 56 Selecting Variables 57 Choose your plots 58 The Output Window 59 Plots of Data 60 Normal Curve 61 Saving Data Always remember to save your SPSS data and output Get organised – Create a file on your P drive (My Documents) e.g. ‘Stats’ – Give your files distinct names e.g. ‘Week 1 Task A’ 62