Notities les 1 zowi PDF
Document Details
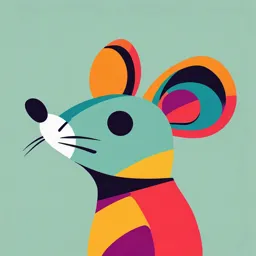
Uploaded by ExceptionalUkulele109
University of Antwerp
null
Prof. dr. Steven Abrams
Tags
Summary
These notes cover medical statistics, including descriptive and inferential statistics. They include examples and questions. The document details statistical concepts relevant for medical research.
Full Transcript
Wetenschappelijke Vorming 2: Medische Statistiek Herhaling - Beschrijvende en inferentiële statistiek Academiejaar 2024–2025 Prof. dr. Steven Abrams Some introductory questions 2/152 type uitkomstvariabele: zo weet je welke methode je moet gebruiken om gegevens te analyseren...
Wetenschappelijke Vorming 2: Medische Statistiek Herhaling - Beschrijvende en inferentiële statistiek Academiejaar 2024–2025 Prof. dr. Steven Abrams Some introductory questions 2/152 type uitkomstvariabele: zo weet je welke methode je moet gebruiken om gegevens te analyseren kwalitatief: kwantitatief: discreet Quiz nominaal: geen ordening continu ordinaal: wel ordening (vb testscore) 1. Which type of data is given in the following examples: I Male/female, kwalitatief nominaal kwantitatief discreet (word niet gemeten op I Number of heart beats per minute, continue schaal, je kan geen anderhalve hartslag hebben) I Blood pressure? kwantitatief continu 2. What is the median value of the observations xi : (110 + 125)/2 80, 90, 110, 125, 130, 135? 3. How does the previous result change when adding an observation 140 to the aforementioned series? dan is mediaan = 125 4. How do you calculate the sample mean and variance of the series? 5. Explain the difference between Xi and xi ? 3/152 4. steekproef gemiddelde en steekproef variantie: gemiddelde is alle waarden optellen en delen door aantal waarden. Steekproef variantie (maat van spreiding, variabiliteit rond gemiddelde) = word altijd uitgedrukt als afwijkijng vh steekproefgemiddelde, afwijking van elke obserbatie tov gemiddelde (kleine x 'streep'). Waarde linkerkant v gemiddelde: bijdrage die neg is en rechts van gemiddelde: bijdrage pos. zie formule 1 5. grote Xi = stochastische veranderlijke dus volgt een verdeling van waarden en kleine xi is een getal (vb 7) grote S: volgt een verdeling - heeft niet één waarde maar als je steekproef trekt zal het bepaalde waarde hebben, als we meerdere steekproeven trekken is zodra we één waarden trekken uitverdeling dan spreken we van kleine s. Bloeddruk Y - N(mu, signa^2) populatie stochastische veranderlijke volgt bepaalde verdeling Dus grote letter: heeft bepaalde verderling kleine letter: is een waarde/getal Quiz - Main dish I I In a clinical trial researchers found that a new drug against nausea is effective in I 90% of the cases for children (0-18 years) I 95% of the cases for adults (19-65 years) I 80% of the cases for elderly (65+) I Research question I: What is the probability to have an effective drug for a random person given that 25% of the affected individuals is younger than 18 years, 45% thereof is adult and 30% of individuals is older than 65 years? I Research question II: What is the probability that a treated person is an adult, given that the drug was effective? 4/152 Quiz - Main dish II I Define the following events: I A: drug is effective, I Bi : sick individual in age group i = 1, 2, 3 I Law of total probability: 3 X P(A) = P(A|Bi )P(Bi ) i=1 = 0.90 ⇥ 0.25 + 0.95 ⇥ 0.45 + 0.80 ⇥ 0.30 = 0.8925 I Bayes’ theorem: P(A|B2 )P(B2 ) 0.95 ⇥ 0.45 P(B2 |A) = = = 0.479 P(A) 0.8925 I The drug is effective in 89% of the cases, and 48% of the patients for which the drug is effective is adult 5/152 Quiz - Dessert I zie notities op papier I Which statistical test can be used to solve the following problems: 1. In an experiment researchers study the effectiveness of a new antibiotic for the treatment of leprosy in rats. The survival time (in weeks) was measured for 18 different rats that were randomised in a treatment and control group. Control 4.055 4.306 4.393 4.572 4.618 5.207 4.759 4.855 5.053 Treatment 4.953 5.155 5.529 5.585 6.234 6.360 6.360 6.424 6.686 6/152 Quiz - Dessert II 2. In order to evaluate the effect of a new flu vaccine, n = 1000 inhabitants of a village are offered a free vaccine. More specifically, two doses of the vaccine are administered in two weeks, prior to the start of the flu season. Some individuals refuse the invitation, some participated once, and others were vaccinated twice. Number of doses Influenza No dose 1 dose 2 doses Total Yes 24 9 13 46 No 289 100 565 954 Total 313 109 578 1000 7/152 Quiz - Dessert III I Hypothesis testing: H0 : µc = µt H1 : µc < µt I If the survival times in both groups are normally distributed ) Two sample t-test I If n1 and n2 are small AND the survival times are non-normally distributed ) Wilcoxon rank-sum test with normal approximation if min(n1 , n2 ) 10 or based on tables if min(n1 , n2 ) < 10 I Hypothesis testing: H0 : Vaccination scheme and flu are independent H1 : Vaccination scheme and flu are dependent I Chi-square test of independence 8/152 Basic statistical concepts 9/152 Basic concepts – Sample versus population populatie en steekproef Population I A population refers to a well-defined group of subjects in which the researcher is interested from a scientific point of view I Often, a population is too large (or even infinite) to examine all subjects (too expensive, too time-consuming,...) Sample: I A sample is a finite collection of study subjects for which observed characteristics and response values are recorded I Sample needs to be representative in order to provide valid inference at the population level 10/152 Basic concepts – Statistical significant versus clinical relevant gaan er vanuit dat steekproef altijd representatief is vr de populatie Statistical significant: I Statistical significance is based on measurements, observations, numbers,... verschil statischische en klinische relevantie: stel effect van bepaalde behandeling, als ik genoeg observaties I Statistical expertise is required heb ga ik verschil vinden = statisctische significatnie. Is dat verschil betekenisvol? Hoe groot is dat verschil/ Clinical relevant: effect? Is het effect klinisch relevant (heeft niks te maken met statistiek) = klinische relevantie I Which research questions are relevant to answer? I Clinical relevance is determined using domain-specific expertise I Medical doctor, clinical investigator, lab-researcher,... ) Statistical significant 6= clinical relevant 11/152 Basic concepts – Methods of research randomisatie: willekeurige indeling v personen/subjecten in behandelingsgroep/controlegroep - we willen bias vermijden die geinduceerd kan worden waarbij balans verstoord is op vlak van bepaalde karakteristieken. Daarom RCT belangrijkste studie vr aantonen oorzaak en gevolg Two large groups of research/studies: I Experimental studies vb randomized controled trial: behandelingsgroep vergelijken met controlegroep I Studying the effect of a treatment I Example: Clinical trials I Randomisation single blinding: pt geblindeerd en weet niet of behandeling of placebo krijgt double blinding: onderzoeker zelf weet dat ook niet I Blinding triple blinding: persoon die analyse doet weet het ook niet I Placebo ➔ Zo vertekening voorkomen I Aim: Does a causal relationship exist? I Observational studies kijken nr associatie: is er verband tussen blootstelling en resultaat I No active intervention I Example: Has smoking an effect on the development of lung cancer? I In general no conclusions about causal relationships, only evidence of potential associations 12/152 Basic concepts – Types of data Qualitative data: gegevens over kenmerk met bepaalde uitkomstvariabele die niet numeriek van aard zijn niet geordend I Nominal data: categorical data used to classify an object of characteristic, e.g., gender, group membership, diagnosis geordend I Ordinal data: categorical data with specific ordering, e.g., opinion polls asking whether we strongly disagree, disagree, agree or strongly agree Quantitative (numeric) data: I Discrete data: measurement or count (ordered) data for which values cannot lie arbitrarily close to each other, e.g., meestal gaat het over gehele getallen, vb the number of pregnancies of a woman aantal bpm, aantal zwangerschappen vrouw,... I Continuous data: measurement data which could take all values within a range, e.g., individual’s weight, or length kunnen elke numerieke waarde aannemen 13/152 Basic concepts – Types of data Examples: 1. body length kwantitatief continu 2. color of the eyes kwalitatief nominaal 3. daily number of car accidents in Flanders kwantitatief discreet 4. body mass index kwantitatief continu 5. reading level (S1 – S7) kwalitatief ordinaal 14/152 Basic concepts – Summarising data I Measures of location: als we kijken nr verdeling v bepaalde variabele, waar bevind die zich I (arithmetic) mean I median I (quartiles) I Measures of variation: I variance and standard deviation I range I interquartile range (IQR) mediaan = tweede kwartiel dus 50% vd observaties is kleiner dan de mediaan er is dan ook eerste en derde kwartiel ➔ afstand tussen eerste en derde kwartiel = interkwartielafstand 15/152 Basic concepts – Measures of location zie cursustekst vr extra info Mean The (arithmetic) mean x of numeric observations x1 ,... , xn is given by n 1 X x= xi n i=1 Median The median of a series of n numeric observations is, after ordering of the values in this series, I the middle value, if n is an odd number, I the arithmetic mean of the two middle numbers, if n is even 16/152 Basic concepts – Measures of location I In case of observations in the following xi fi fi⇤ frequency table (n observations; p x1 f1 f1⇤ different values of x) then the arithmetic x2 f2 f2⇤ mean is given by: x3 f3 f3⇤ p......... 1X xi fi xp fp fp⇤ n i=1 17/152 Basic concepts – Quartiles Definition I the first quartile Q1 is the number with rank n+1 4 I the second quartile Q2 is the number with rank n+1 2 3(n+1) I the third quartile Q3 is the number with rank 4 Non-rationale ranks: x(i+p) = x(i) + p ⇥ x(i+1) x(i) 18/152 Basic concepts – Measures of Variation Variance The variance s2 of numeric observations x1 ,... , xn is the (non-negative) number given by n n ! 1 X 1 X s2 = (xi x)2 = xi2 nx 2 n 1 n 1 i=1 i=1 Standard deviation The standard deviation s of numeric observations x1 ,... , xn is the (non-negative) number given by the positive square root of the variance: v v ! u n u n u 1 X u 1 X s=t (xi x)2 = t xi2 nx 2 n 1 n 1 i=1 i=1 19/152 Basic concepts – Measures of Variation Range The range of numeric observations x1 ,... , xn is defined as range = max xi min xi 1in 1in = x(n) x(1) Interquartile range The interquartile range of numeric observations x1 ,... , xn is defined as interquartile range = (third quartile) (first quartile) = Q3 Q1 20/152 Graphical Representation: Boxplot lengte box = interkwartiel afstand kleinste geordende getal dat geobserbeerd is ! ! &'"( &')( = maximale waarde !" $% !# of tweede kwartiel symmetrisch of niet? ➔ niet exact symmetrisch want mediaan ligt oa niet exact tussen Q1 en Q1 en box ligt niet in midden van lijn 21/152 Hypothesis testing 22/152 Hypothesis testing for a population parameter toets van hypothese = gelinked aan onderzoeksvraag ➔ onderzoeksvraag vertalen we nr statistisch toetsingsprobleem met twee hypotheses Hypothesis testing is statistically checking the correctness of a hypothesis. More specifically, hypothesis testing is constructing a decision rule to decide, after sampling, whether the observed data leads to evidence to reject or not reject the null hypothesis denoted as H0 op basis v steekproef die we verzameld hebben gaan we zien of we bewijs hebben tegen de nul hypothese, we willen de nulhypothese verwerpen, bewijzen dat er geen effect/verschil is. We willen beslissing nemen ten toets van hypothese gunsten van de alternatieve hyppthese (wat we trachten te bewijzen) = altijd op bepaald Two kinds of errors we can make by taking a decision: significantie niveau. I Reject H0 when its correct: type I error I Do not reject H0 when its wrong: type II error H0 waar H0 niet waar H0 verwerpen type I fout juist H0 niet verwerpen juist type II fout 23/152 we willen type I en type II fout zo klein mogelijk. Type I Als type I fout nr beneden word geduwd gaat type II fout nr boven dus gaan type I fout controleren, kijken nr bepaald signifantie niveau, we laten toe dat we nulhypothese verwerpen in 5% vd gevallen. Type II: hoe meer datapunten - hoe kleiner type II fout. We gaat dus bepalen hoeveel observaties we nodigen hebben vr voldoende power van de test meer observaties: type II fout nr beneden en kracht van test nr boven. Type II fout bepaald dus hoeveel observaties nodig vr bepaalde power vd test we gebruiken altijd SN 5%, maar als je zekerder wil zijn, kun je die veranderen, alvorens je de analyse doet Hypothesis testing for a population parameter Two kinds of errors: truth H0 is correct H0 is wrong decision reject H0 wrong decision correct decision type I error not reject H0 correct decision wrong decision type II error I ↵ = P(type I error) = significance level (s.l.) I We reject the null hypothesis H0 at s.l. ↵ (or: do not reject at s.l. ↵) I = P(type II error) I 1 = power (of a test) 24/152 Hypothesis testing for a population parameter Possible errors H0 : Not pregnant vs. H1 : Pregnant 25/152 General procedure formuleer het toetsingsprobleem: wat is H0 en wat is H1 1. Formulate the testing problem using the null hypothesis H0 en alternative hypothesis H1 2. Calculate the appropriate test statistic for the testing problem gepaste toetsingsgrootheid berekeken vr toetsingsprobleem vb T-test (gepaard of ongepaard....) etc.. 3. Construct decision rule to reject or not reject H0 based on the sample values: beslissen wnr waarde extreem is, ja of nee. Op basis van kritisch punt in bepaalde verdeling of obv p-waarde 3a. Calculate a critical value (c.v.) of the distribution of the test statistic under H0 and compare the value of the test statistic with the c.v. 3b. Or: calculate the p-value and compare with the s.l. ↵ 4. Formulate a conclusion for the problem Als p-waarde kleiner is dan SN - dan verwerpen we H0 Als p-waarde groter is dan SN - H0 niet verwerpen p-waarden die rond treshold zit (SN: 0,5), er net onder of net boven, dan is het niet significant 26/152 Hypothesis testing for a population mean Testing problems (for a population mean) are: H0 : µ = µH0 versus H1 : µ < µH0 (one-sided) één gemiddelde schatten obv interventie H0 : µ = µH0 versus H1 : µ > µH0 (one-sided) H0 : µ = µH0 versus H1 : µ 6= µH0 (two-sided) with µH0 the value of the population mean if H0 is true 27/152 Step 1: One-sided or two-sided hypotheses Testing problems (for a population mean) are: H0 : µ = µH0 versus H1 : µ < µH0 (one-sided) H0 : µ = µH0 versus H1 : µ > µH0 (one-sided) H0 : µ = µH0 versus H1 : µ 6= µH0 (two-sided) with µH0 the value of the population mean if H0 is true 28/152 Step 2: Select an appropriate test statistic For n large: n is groot >30 , hebben nl heel veel data gemiddelde schatten, doen we adh steekproefgemiddelde (grote X-streep) X µH0 H0 p ⇠ N(0; 1) if 2 known 2 /n als nulhypothese waar is, is waarde normaal verdeeld populatievariantie kunnen we vaak niet dus als waarde in sta sigma kwadraat vervangen door S kwadraat X µH0 H0 2 p ⇠ N(0; 1) if unknown S 2 /n = Z-test For n small and a normally distributed population: vb geboortegewicht: volgt normale verdeling X µH0 H0 Al 2 p ⇠ N(0; 1) if known 2 /n deze waarden kunnen we weten door steekproef te trekken uit verdeling X µH0 H0 2 T-test: zwaardere staart p ⇠ t(n 1) if unknown dan T-test dus kans groter dat je daar terecht komt S 2 /n = één steekproeven T-test 29/152 Step 3a.: Construct a decision rule – critical value Property If Z ⇠ N(0; 1), then P( 1.96 < Z < 1.96) = 0.95 P(Z < 1.645) = 0.05 P(Z > 1.645) = 0.05 !#!&' !#!&' ("#$% ! "#$% 30/152 Step 3b.: Construct a decision rule – p-value Definition A p-value is equal to the probability, when the null hypothesis is true, to have a value for the test statistic that is the same or more extreme (or larger in absolute value) than the observed value for the test statistic Decision rule: I if p-value < 0.05, then H0 is rejected and the results are significant at significance level ↵ I if p-value 0.05, then H0 is not rejected and the results are not significant at significance level ↵ 31/152 Hypothesis testing for a population proportion Testing problems: (a) H0 : ⇡ = ⇡H0 versus H1 : ⇡ < ⇡H0 (b) H0 : ⇡ = ⇡H0 versus H1 : ⇡ > ⇡H0 (c) H0 : ⇡ = ⇡H0 versus H1 : ⇡ 6= ⇡H0 with ⇡H0 the value of the population proportion if H0 is true For large samples, if H0 is true, P ⇡H0 H0 q ⇠ N(0; 1) ⇡H0 (1 ⇡H0 ) n (Rule of thumb: n⇡H0 5 and n(1 ⇡H0 ) 5) 32/152 Overview of statistical tests to compare two or more means/proportions 33/152 testen die we niet bespreken: niet kennen parametrisch = Overview of statistical tests for location Samples Data Type Method one sample continuous parametric one-sample t-test één steekproeven probleem dichotomous parametric one-sample z-test dichotomous non-parametric Binomial test = binair nadeel niet-parametrische nominal non-parametric Chi-square goodness-of-fit test test: verliest kracht ordinal non-parametric one-sample Wilcoxon signed-rank test two paired continuous parametric paired t-test samples dichotomous non-parametric McNemar test twee steekproeven: ordinal non-parametric sign test onderscheid in gekoppeld en niet gekoppeld ordinal non-parametric Wilcoxon signed-rank test two independent continuous parametric unpaired t-test samples dichtomous parametric two-sample z-test nominal non-parametric Chi-square test of independence nominal non-parametric Fisher’s exact test ordinal non-parametric Wilcoxon rank-sum test three or more continuous parametric repeated measures ANOVA paired samples dichotomous non-parametric Cochran’s Q test ordinal non-parametric Friedman test three or more continuous parametric one-way ANOVA independent nominal non-parametric Chi-square test of independence samples ordinal non-parametric Kruskal-Wallis H test ordinal non-parametric Jonckheere-Terpstra test 34/152 Introduction Parametric methods: I Validity depends on knowing population distribution function I One- and two-sample (unpaired) t-tests and paired t-test are robust against violation of the normality assumption when sample size is large I Small sample sizes: unreliable t-tests when normality assumption is untenable als steekproef klein word: krijgen we onbetrouwbare t-teslt resultaten Overview of parametric location tests discussed here: I One-sample t-test – Paired t-test I Unpaired t-test I Repeated measures ANOVA I One-way ANOVA 35/152 One-sample t-test eerst toetsen we hierbij hypothese etc One-sample t-test is a parametric test used to test whether the population mean µ is equal to a specific value µ0 based on a sample X1 ,... , Xn. Experiment: The average birthweight for women living in poverty in the UK is µ0 = 2800 grams. An innovative new prenatal care program is introduced to reduce the number of low birthweight babies born in poverty. In total, n = 25 mothers, all of whom living in poverty, participated in the program. Research question: Is the program effective at improving the birthweights of babies born to poor women? 36/152 One-sample t-test 1. formuleren van toetsen vd hypothese I One- or two-sided test hypotheses: H0 : µ = µ0 gemiddeld geboortegewicht vd babies na interventie is 2800 gram H1 : µ < µ0 OR µ > µ0 OR µ 6= µ0 we gaan ervan uit dat interventie werkt en geboortegwicht gaat vergroten I Test statistic: n is klein en sigma kwadraat kennen we niet X µ 0 H0 T = q ⇠ t(n 1) aantal vrijheidsgraden = 24 S2 n I Sample mean X and sample variance S 2 are unbiased estimators for the population mean µ and variance 2 I For large n 30, Student’s t-distribution can be approximated by a standard normal distribution (one-sample z-test) als n groot zou zijn, word deze t verdeling een standaard normaal verdeling en zitten we met Z-test I Birthweights are assumed to be normally distributed 37/152 One-sample t-test Normal Q−Q Plot 3200 Sample Quantiles 3000 2800 2600 −2 −1 0 1 2 Theoretical Quantiles 38/152 One-sample t-test is maar een schatting v geboortegewicht vd babies na interventie, er is ook onzekerheid I Observed data: the sample mean is equal to x = 2925 grams and the standard deviation of the birthweights is s = 200 grams (n = 25) I Value of the test statistic: t = 3.125 > t0.95,24 = 1.711 VG (one-sided t-test) kp bepalen uit T-verdeling met 24 VG I p-value = P(T t) < 0.0023 < ↵ = 0.05 X - 1,711 3,125 Answer: The average birthweight is significantly different from the hypothesized value at significance level ↵ = 0.05 implying that the new program is effective in increasing the birthweight. 39/152 One-sample t-test in R t.test2 0 OR 6= 0 mediaan niet gelijk aan nul I Calculate differences di = xi yi and absolute values |di | I Determine ranks ri based on |di | in ascending order P I Define Wilcoxon statistic W = ni=1 I {di > 0} ri , i.e., sum of the ranks ri from the group of positive differences di > 0 I Test statistic with continuity correction: formule toetsingsgrootheid ⇣ ⌘ W n(n+1) 4 ± 0.5 H0 W⇤ = q ⇡ N(0, 1) Pn ri2 i=1 4 I Normal approximation is valid when ne 15 (ne = # of non-zero di ), otherwise exact distribution of W (table) 89/152 Wilcoxon signed-rank test I Observed data: ne = 40 since 5 di ’s are equal to 0 we gaan absolute Negative Positive waardes nemen van scores, max verschil in |di | di fi di fi+ fi = fi + fi+ ri score is eig max 9 dus 10 hier niet van 10 10 0 10 0 0 toepassing. Als scores hetzelfde zijn draagt he 9 9 0 9 0 0 tni bij aan vergelijking A 8 8 1 8 0 1 40.0 en B scores kunnen pos of neg zijn 7 7 3 7 0 3 38.0 6 6 2 6 0 2 35.5 absolute frequenties: hoe vaak word een 5 5 2 5 0 2 33.5 verschil geobserveerd 4 4 1 4 0 1 32.0 3 3 5 3 2 7 28.0 observaties met absoluut 2 2 4 2 6 10 19.5 verschil aan 2 1 absolute waarde 1 komt 14 keer 1 4 1 10 14 7.5 voor dus rangnummer 7,5 I w = 10 ⇥ 7.5 + 6 ⇥ 19.5 + 2 ⇥ 28 = 248, w ⇤ = 2.188 w = rangnummer x aantal keer dat bepaald I Normal approximation (ne 15): verschil voorkomt two-sided p-value = 2P(W ⇤ 2.188) = 0.0286 < ↵ Als we sign test gebruikt: niet echt significant verschil maar als je signed rank test gebruikt wel 90/152 Wilcoxon signed-rank test Answer: There exists a significant difference between sunscreen A and B at significance level ↵ = 0.05. Assumptions & Remarks: I Dependent samples with ordinal response values I Wicoxon signed-rank test can be used in case of continuous data ) does not require normality assumption when sample size is small (cfr. paired t-test) 91/152 hebbn het nu gebruikt vr alle verschillen die niet gelijk zijn aan nul, maar hebben 45 observaties waarbij 5 gelijk zijn aan nul, Wilcoxon signed-rank test waarde van nul moet ook een waarde hebben, doen we door extra rij toe te kennen met verschil 0 I Use a correction for the number of zero differences I Wilcoxon signed-rank test statistic: n(n+1) t0 (t0 +1) ⇤ W 4 H0 W = p ⇡ N(0, 1), Var (W ) where 1 Var (W ) = [n(n + 1)(2n + 1) t0 (t0 + 1)(2t0 + 1)] 24 1 X ti (ti + 1)(ti 1) 48 i>0 and I t0 is the number of zero differences, I ti for i > 0 represents the number of values in the ith group of nonzero signed ranks (no ties, ti = 1) 92/152 Wilcoxon signed-rank test I Use a correction for the number of zero differences Negative Positive |di | di fi di fi+ fi = fi + fi+ ri 8 8 1 8 0 1 45.0 7 7 3 7 0 3 43.0 6 6 2 6 0 2 40.5 5 5 2 5 0 2 38.5 4 4 1 4 0 1 37.0 3 3 5 3 2 7 33.0 2 2 4 2 6 10 24.5 1 1 4 1 10 14 12.5 0 0 0 5 3.0 I w = 10 ⇥ 12.5 + 6 ⇥ 24.5 + 2 ⇥ 33 = 338, w ⇤ = 1.954 I Normal approximation (n 15): two-sided p-value = 2P(W ⇤ 1.954) = 0.0507 < ↵ p significant gaat nr borderline signifact (rond de 0,05) 93/152 2 x 2 tabel Chi-square test of independence MI geen MI vergelijkt deze tabel met de tabel die je zou verwachten onder de OCPs 13 4987 5000 nulhypothese. Onder de nulhypothese: kans op hartinfarct in OCPgroep = · kans op hartinfarct in niet OCP groep gn OCPs 7 9993 H0: pi_OCP = pi_nOCP 10000 pi 'streep' = (13+7)/15000 onder H0 pi 'streep' x 50000 * Chi-square test of independence determines whether the occurrence of two nominal outcomes (with two or more levels) is statistically independent. verwachte waarden onder de H0 Experiment: A researcher is interested whether the measles-mumps-rubella (MMR) vaccine location (thigh vs. arm) is independent of severity (no severe vs. severe reaction) of the vaccine reaction for infants aged 1 year (first MMR dosis). Research question: Are the vaccine location and the severity of the reaction on the vaccine independent? 94/152 verwachte waarden onder de H0 MI gn MI OCP 6,67* gn OCP 13,33 Chi-square test of independence I Observed data: 2 ⇥ 2 contingency table No severe reaction Severe reaction Total Arm n11 = 255 n12 = 25 n1. = 280 Thigh n21 = 125 n22 = 15 n2. = 140 Total n.1 = 380 n.2 = 40 n = 420 I Test hypotheses: H0 : Severity and vaccine location are independent H1 : Severity and vaccine location are dependent I Chi-square test statistic: 2 X X 2 2 2 Oij Eij H0 2 = ⇠ (1) Eij i=1 j=1 I Observed frequencies Oij = nij , expected frequencies Eij = npi. p.j , where pi. = Oi. /n and p.j = O.j /n 95/152 Chi-square test of independence I Expected data under H0 : No severe reaction Severe reaction Total Arm E11 = 253.333 E12 = 26.667 n1. = 280 Thigh E21 = 126.667 E22 = 13.333 n2. = 140 Total n.1 = 380 n.2 = 40 n = 420 I 2 = 0.345 < 2 0.95,1 = 3.841, p-value= 0.557 > ↵ = 0.05 Answer: We can not reject the null hypothesis of independence between vaccine location and severity at significance level ↵ = 0.05. 0,34 is niet groter dan kp 3,8 dus verwerpen niet want geen significant verschil vr kans op neveneffectten 96/152 Chi-square test of independence in R dat 5, 8i, j (Yates’s correction) I Independence between the observations, otherwise, McNemar’s test I Generalization to more than two levels (r rows, c columns in r ⇥ c table) vr algemen tabel r X X c 2 2 Oij Eij H0 2 = ⇠ ((r 1)(c 1)) Eij i=1 j=1 98/152 alternatief vr Z test vr 2 proporties = fishers exact Wilcoxon rank-sum test alternatief vr ongepaarde t-test Wilcoxon rank-sum test is used to test for differences between two independent samples X1 ,... , Xn1 and Y1 ,... , Yn2 of ordinal or continuous data. Experiment: A Phase II clinical trial is performed to assess the effectiveness of a new drug designed to reduce symptoms of asthma in children. A total of n = 12 participants are randomized in a treatment (n1 = 7) or placebo group (n2 = 5). Participants are asked to record the number of asthma episodes over a one week period following receipt of the assigned treatment. Research question: Is there a significant treatment effect for the new asthma drug? 99/152 Wilcoxon rank-sum test I Let 1 and 2 denote the median number of asthma episodes during a week in the placebo and treatment group I One-sided test problem H0 : 1 = 2 H1 : 1 > 2 mediane aantal asthmas is groter in placebogroep dan in behandelde groep I Similar to Wilcoxon signed-rank test: use of ranks I Observed data and corresponding ranks: Number of asthma episodes 6 komy drie keer vr dus gemiddelde 8, 9 en 10 Placebo 7 5 6 4 10 Treatment 3 6 5 4 2 1 6 Corresponding ranks Placebo 11 6.5 9 4.5 12 Treatment 3 9 6.5 4.5 2 1 9 100/152 Wilcoxon rank-sum test I Define W1G = sum of the ranks in group 1 (placebo group) I Test statistic (n large, i.e., min (n1 , n2 ) 10): ⇣ ⌘ n1 (n1 +n2 +1) W1G 2 ± 0.5 H0 W⇤ = v " # ⇡ N(0, 1) u Pg ⇣ ⌘ un n t j=1 j tj2 1 t 1 2 n1 + n2 + 1 12 (n1 +n2 )(n1 +n2 1) I tj represents the number of observations for response value j and g the total number of different response values I Wilcoxon rank-sum test in literature also referred to as Mann-Whitney U test I Small sample size: exact values based on Mann-Whitney U g statistic (table), U = n1 n2 + [n1 (n1 + 1)/2] W1 101/152 Wilcoxon rank-sum test Experiment: I w1g = 11 + 6.6 + 9 + 4.5 + 12 = 43, w ⇤ = 1.641 I Small sample size: u = n1 n2 + [n1 (n1 + 1)/2] w1g = 7 I Exact one-sided p-value = P(U 7) = 0.053 > ↵ = 0.05 niet veel bewijs tegen nul hypothese, er is geen significatn effect Answer: We can not reject the null hypothesis of no treatment effect at significance level ↵ = 0.05. 102/152 Kruskal-Wallis H test slaan we even over Kruskal-Wallis H test used to determine if there are statistically significant differences between two or more independent samples (ordinal or continuous dependent variables), and is an extension of the Mann-Whitney U (Wilcoxon rank-sum) test. Experiment: One wants to study whether test performance (on a continuous scale ranging from 0 to 100) is different for different test anxiety levels (i.e., low, medium or high levels). Research question: Is there a significant difference in test performance between students with low, medium or high test anxiety levels? 103/152 Kruskal-Wallis H test I Observed data and corresponding ranks: anxiety Test performance score low 64 68 72 83 84 91 94 97 medium 25 37 49 54 59 81 82 high 13 41 49 52 55 82 Corresponding ranks Rij R̄i. low 11 12 13 17 18 19 20 21 16.4 medium 2 3 5.5 8 10 14 15.5 8.3 high 1 4 5.5 7 9 15.5 7.0 I Test hypotheses: 1 – low, 2 – medium, 3 – high H0 : 1 = 2 = 3 H1 : 9(j, k) : j 6= k Pni I Kruskal-Wallis test statistic (R̄i. = j=1 Rij /ni ): Pg [12/n(n + 1)] i=1 ni R̄i.2 3(n + 1) H0 2 H= PG ⇠ (g 1) 1 3 ti )/(n3 n) i=1 (ti I Tie correction: ti number of values in tie-group i = 1,... , G 104/152 Kruskal-Wallis H test I h = 10.063 > 20.95,2 = 5.991, p-value = 0.0065 < ↵ = 0.05 I Small sample size, i.e., not all ni > 5: exact probabilities from statistical table Answer: We reject the null hypothesis of equal medians in the three groups at significance level ↵ = 0.05. 105/152 Kruskal-Wallis H test Assumptions & Remarks: I Ordinal or continuous dependent variables are required I Two or more categorical independent groups I Independence of observations within and between groups I Distributions of response variable in each group of the independent variable should have the same shape (and variability) I Parametric equivalent of the Kruskal-Wallis H test = one-way analysis of variance (ANOVA) I Kruskal-Wallis H test does not assume normality in the data and is less sensitive to outliers as compared to one-way ANOVA I Account for ordinal nature Jonckheere-Terpstra test I Post-hoc multiple comparison tests 106/152 welke type test je wnr kan gebruken Which test do we have to use? Ordinal or continuous data? Ordinal Continuous Tests in 1 group, or compare 2 groups? Tests in 1 group, or compare 2 groups? 1 group 2 groups 2 groups 1 group Paired measurements or independent measurements Paired measurements or independent measurements Paired Independent Independent Paired Proceed with ≠ Only info about better/worse, or 𝑛1 and 𝑛2 ≥ 30? 𝑛 ≥ 30, or 𝑛 < 30 how much better/worse no yes How much Only better/worse 𝑛 < 30 𝑛 ≥ 30 Both groups normally distributed Normally distributed data? no yes Not normal Normal Signed-rank test Sign test Rank-sum test 2 sample t-test Signed-rank test 1 sample t-test 107/152 Which test do we have to use? Ordinal data ) non-parametric I Paired observations: I Sign test: I Uses only information: A is better than B or B is better than A I Normal approximation is valid when n 30 I Exact method can be used when n < 30 I Wilcoxon signed-rank test: I Used different categories that require ordening I Normal approximation is valid when n 15 I Tables can be used when n < 15 in the absence of ties I Independent observations: I Wilcoxon rank-sum test: I Two groups with measurements that can be ordered I Normal approximation can be used when min(n1 , n2 ) 10 I Tables can be used when min(n1 , n2 ) < 10 in the absence of ties I Kruskal-Wallis H test: I Three or more groups with ordinal response variables I Tables can be used when not all ni > 5 108/152 Which test do we have to use? Quantitative data ) (non-)parametric I 1 sample: I n 30 or population is normally distributed: I parametric tests : normal approximation or one-sample t test I non-parametric tests : signed-rank with normal approximation I n < 30 and population is not normally distributed: I signed-rank test with normal approximation when n 16 I signed-rank test with tables when n < 16 and no ties I 2 samples: I paired measurements: one sample (paired t-test, see 1 sample) I independent measurements: I n1 and n2 large or normally distributed populations: ! parametric tests: normal approximation or unpaired t-test ! non-parametric tests: rank-sum test with normal approximation I n1 or n2 small and non-normally distributed populations: ! rank-sum test with normal approximation when min(n1 , n2 ) 10 ! rank-sum test with tables when min(n1 , n2 ) < 10 and no ties 109/152 Which test do we have to use? Quantitative data ) (non-)parametric I 3 samples: I paired measurements: I Normally distributed populations with sphericity: ! parametric tests: one-way repeated measured ANOVA ! non-parametric tests: Friedman test I Non-normally distributed populations or lack of sphericity: ! non-parametric tests: Friedman test I independent measurements: I Normally distributed populations with homogeneity of variances: ! parametric tests: one-way ANOVA ! non-parametric tests: Kruskal-Wallis H test I Non-normally distributed populations or heteroscedasticity: ! non-parametric tests: Kruskal-Wallis H test 110/152 Case study hier gestopt bij les 1 vanaf hier niet meer bekeken 111/152 Case study I – Leprosy in rats Experiment In an experiment reported in the Lancet on the effective- ness of the antibiotic isoniazid as a treatment for leprosy in rats, one studies the survival times (in weeks) for n = 20 rats randomized in a treatment and control group. 112/152 Case study I – Leprosy in rats Untreated rats Treated rats 4.055 4.953 4.306 5.155 4.393 5.529 4.572 5.585 4.618 6.234 4.618 6.360 4.759 6.360 4.855 6.424 5.053 6.424 5.207 6.686 113/152 Case study I – Leprosy in rats I Normality assumption: positive time variables ) logarithmic data transformation Untreated rats Treated rats 1.40 1.60 1.46 1.64 1.48 1.71 1.52 1.72 1.53 1.83 1.53 1.85 1.56 1.85 1.58 1.86 1.62 1.86 1.65 1.90 I Shapiro-Wilk test & F -test for normality & homoscedasticity I Unpaired t-test in case of normality & homoscedasticity I Small sample sizes & non-normality ) non-parametric Wilcoxon rank-sum test 114/152 Case study II – Influenza vaccine Experiment In order to evaluate the effect of a new influenza vaccine, one offers n = 1000 inhabitants of a village the opportu- nity to use the vaccine freely. More specifically, administra- tion of 2 doses of the vaccine within 2 weeks was recom- mended. However, some individuals declined the invitation, some participated only once and others were vaccinated twice. During the next influenza season, one reports the presence of influenza for all these individuals and studies dependence between vaccination schedule and probability to acquire influenza. 115/152 Case study II – Influenza vaccine Number of doses Influenza No dose 1 dose 2 doses Total Yes 24 9 13 46 No 289 100 565 954 Total 313 109 578 1000 116/152 Case study II – Influenza vaccine I Chi-square test of independence H0 : Vaccine schedule and influenza are independent H1 : Vaccine schedule and influenza are dependent I Calculate expected cell counts Eij in each cell Number of doses Influenza No dose 1 dose 2 doses Yes 14.398 5.014 26.588 No 298.602 103.986 551.412 I 2 contributions implying 2 = 17.314 > 2 0.95,2 = 5.99 Number of doses Influenza No dose 1 dose 2 doses Yes 6.404 3.169 6.944 No 0.309 0.153 0.335 I We reject H0 at significance level ↵ = 0.05 117/152 Case study III – Sore throat Experiment In a study, one wants to investigate whether a patient expe- rienced a sore throat on waking after surgery with general anesthesia (Y with 0 = no, 1 = yes), and how this is af- fected by the duration of the surgery (D in minutes) and the type of device used to secure the airway (T with 0 = laryn- geal mask airway, 1 = tracheal tube). 118/152 Case study III – Sore throat Patient D T Y Patient D T Y Patient D T Y 1 45 0 0 13 50 1 0 25 20 1 0 2 15 0 0 14 75 1 1 26 45 0 1 3 40 0 1 15 30 0 0 27 15 1 0 4 83 1 1 16 25 0 1 28 25 0 1 5 90 1 1 17 20 1 0 29 15 1 0 6 25 1 1 18 60 1 1 30 30 0 1 7 35 0 1 19 70 1 1 31 40 0 1 8 65 0 1 20 30 0 1 32 15 1 0 9 95 0 1 21 60 0 1 33 135 1 1 10 35 0 1 22 61 0 0 34 20 1 0 11 75 0 1 23 65 0 1 35 40 1 0 12 45 1 1 24 15 1 0 Source: Data from D. Collett, in Encyclopedia of Biostatistics (New York, Wiley: 1998), p. 350–358. 119/152 Case study III – Sore throat I How to analyse these data? Answer the research question? 120/152 Case study III – Sore throat I Logistic regression model for binary response variable Y Call: glm(formula = response ˜ duration + type, family = binomial(link = logit), data = airway) Deviance Residuals: Min 1Q Median 3Q Max -2.3802 -0.5358 0.3047 0.7308 1.7821 Coefficients: Estimate Std. Error z value Pr(>|z|) (Intercept) -1.41734 1.09457 -1.295 0.19536 duration 0.06868 0.02641 2.600 0.00931 ** type1 -1.65895 0.92285 -1.798 0.07224. --- Signif. codes: 0 ‘***’ 0.001 ‘**’ 0.01 ‘*’ 0.05 ‘.’ 0.1 ‘ ’ 1 (Dispersion parameter for binomial family taken to be 1) Null deviance: 46.180 on 34 degrees of freedom Residual deviance: 30.138 on 32 degrees of freedom AIC: 36.138 121/152 Appendix 122/152 Binomial test Experiment: We investigate whether n = 12 laboratory rats choose the ‘correct’ door (behind which food is left) out of 4 possible doors in a maze. Research question: Do these rats significantly prefer the correct door (at significance level ↵ = 0.05)? I Define X = number of rats choosing the ‘correct’ door I H0 : µX = 3 versus H1 : µX > 3 (one-sided test) H I X ⇠0 B (n, p), where p = 1/4 I Observed data: x = 5 rats I Hence, p-value is P (X 5) = 1 P (X < 5) = 0.158 > ↵ Answer: The rats do not show a significant preference for the correct door at significance level 5%. 123/152 Chi-square goodness-of-fit test Chi-square goodness-of-fit test is used to test whether the observed proportions for a categorical (nominal) variable with k levels differ from hypothesized proportions. Experiment: A company claims that 10% of their medical devices breaks down in less than 1 year, 25% between 1 and 2 years, 45% between 2 and 3 years, and 20% after at least 3 years. A random sample of n = 156 medical devices is studied. Research question: Is the observed data consistent with the specified distribution? 124/152 Chi-square goodness-of-fit test I Test hypotheses: H0 : Proportions of devices are 10%, 25%, 45% and 20% for the < 1, 1 2, 2 3 and > 3 groups, respectively H1 : Proportions do not follow the specified distribution I Test statistic: k 2 X (Oi Ei )2 H0 2 = ⇠ (k 1) Ei i=1 I Oi observed frequencies, Ei = n⇡i expected frequencies, and ⇡i the hypothesized proportions 125/152 Chi-square goodness-of-fit test I Observed data: 3 Oi 20.0 32.0 75.0 29.0 Ei 15.6 39.0 70.2 31.2 2 1.24 1.26 0.33 0.16 i I 2 = 2.98 with p-value = 0.39 > ↵ = 0.05 Answer: The observed proportions are not significantly different from the ones reported by the manufacturer at significance level ↵ = 0.05. 126/152 One-sample Wilcoxon signed-rank One-sample Wilcoxon signed-rank test is a non-parametric alternative to the one-sample t-test. The test determines whether the median is equal to a value 0 based on a sample X1 ,... , Xn (ordinal or continuous random variables). Experiment: One randomly captures n = 9 adult male great white sharks around Dyer Island, South Africa, and one is interested in their length Xi. According to previous research the median length of male great white sharks is 4.5m. Research question: Does the median length of these sharks significantly different from the hypothesized value of 4.5m? 127/152 One-sample Wilcoxon signed-rank I One- or two-sided test hypotheses: H0 : = 0 H1 : < 0 OR > 0 OR 6= 0 I Calculate differences di = xi 0 and absolute values |di | I Determine ranks ri based on |di | in ascending order P I Define Wilcoxon statistic W = ni=1 I {di > 0} ri , i.e., sum of the ranks ri from the group of positive differences di > 0 I Test statistic with continuity correction: n(n+1) W 4 ± 0.5 H0 ⇤ W = q ⇡ N(0, 1) Pn ri2 i=1 4 I Normal approximation is valid when ne 16 (ne = # of non-zero di ), otherwise exact distribution of W (table) 128/152 One-sample Wilcoxon signed-rank I Observed data: ne = 8 since one di equal to 0 xi di |di | ri I {di > 0} 4.2 0.3 0.3 4 0 3.8 0.7 0.7 8 0 4.5 +0.0 0.0 4.9 +0.4 0.4 5.5 1 4.3 0.2 0.2 2.5 0 4.7 +0.2 0.2 2.5 1 4.1 0.4 0.4 5.5 0 4.4 0.1 0.1 1 0 5.0 +0.5 0.5 7 1 I w = 5.5 + 2.5 + 7 = 15 < E(W ) = [ne (ne + 1)] /2 = 18 I ne < 16: exact 2-sided p-value = 2P(W 15) = 0.7422 Answer: The median length of these sharks does not differ significantly from 4.5m at significance level ↵ = 0.05 129/152 McNemar test Experiment: A researcher attempts to determine whether a drug has an effect on a particular disease based on subjects with before-and-after measurements (matched pairs). I X /Y = diagnosis before/after treatment I Results 2 ⇥ 2 – contingency table: After treatment (0/1: absent/present) Y =0 Y =1 Total Before X =0 n00 = 33 n01 = 59 n00 + n01 = 92 treatment X =1 n10 = 121 n11 = 101 n10 + n11 = 222 Total n00 + n10 = 154 n01 + n11 = 160 n = 314 I Null hypothesis of marginal homogeneity: H0 : ⇡01 = ⇡10 H1 : ⇡01 6= ⇡10 I McNemar test statistic: 2 (n10 n01 )2 H0 2 = ⇠ (1) n10 + n01 130/152 McNemar test Experiment: A researcher attempts to determine whether a drug has an effect on a particular disease based on subjects with before-and-after measurements (matched pairs). I X /Y = diagnosis before/after treatment I Results 2 ⇥ 2 – contingency table: After treatment (0/1: absent/present) Y =0 Y =1 Total Before X =0 n00 = 33 n01 = 59 n00 + n01 = 92 treatment X =1 n10 = 121 n11 = 101 n10 + n11 = 222 Total n00 + n10 = 154 n01 + n11 = 160 n = 314 I 2 = 21.35 with p-value < 0.001. Answer: Hence, we reject the null hypothesis of no treatment effect at significance level ↵ = 0.05, implying strong evidence in favour of a treatment effect. 131/152 McNemar test Experiment: A researcher attempts to determine whether a drug has an effect on a particular disease based on subjects with before-and-after measurements (matched pairs). I X /Y = diagnosis before/after treatment I If n10 + n01 < 25 then the McNemar statistic 2 is not-well approximated by 2 (1) I Revisited results 2 ⇥ 2 – contingency table: After treatment (0/1: absent/present) Y =0 Y =1 Total Before X =0 n00 = 33 n01 = 16 n00 + n01 = 49 treatment X =1 n10 = 6 n11 = 101 n10 + n11 = 107 Total n00 + n10 = 39 n01 + n11 = 117 n = 156 H I Exact binomial test: N10 ⇠0 B (n10 + n01 , 0.5) I Two-sided p-value: 2P(N10 n10 ) = 2P(N10 6) = 0.0525 I Alternative: continuity corrected version of McNemar test 132/152 Fisher’s exact test Fisher’s exact test is an alternative for the Chi-square test of independence when sample size is small (nominal outcomes): exact probability of the table of observed cell frequencies. Experiment: In a study including 18 patients, 8 women and 10 men, one records the success of a new flu antiviral treatment (success vs. no success). Research question: Is there a difference between the success rate in men and women? 133/152 Fisher’s exact test I Observed data: 2 ⇥ 2 contingency table No success Success Total Men n11 = 2 n12 = 8 n1. = 10 Women n21 = 3 n22 = 5 n2. = 8 Total n.1 = 5 n.2 = 13 n = 18 I H0 : independence versus H1 : no independence I Probability of observing set of values under H0 is given by the hypergeometric distribution: ✓ ◆✓ ◆ n1. n2. n11 n21 P(Nij = nij , 8i, j) = ✓ ◆ n n.1 I Table probability is equal to 0.294 I Exact one-sided p-value = 0.382 > ↵ = 0.05 134/152 Cochran’s Q test Cochran’s Q test is an extension of the McNemar test for testing for differences between matched sets (blocks) of k 3 dichotomous responses (categories). I Experimental design: Category 1 Category 2... Category k Block 1 Y11 Y12... Y1k Block 2 Y21 Y22... Y2k............... Block n Yn1 Yn2... Ynk Total Y.1 Y.2... Y.k I Test hypotheses: H0 : ⇡1 = ⇡2 =... = ⇡k H1 : 9 (a, b) : ⇡a 6= ⇡b , a 6= b & 1 a < b k I Cochran’s Q statistic: P ⇣ ⌘2 k (k 1) kj=1 Y.j Nk H0 2 Q= P Pn ⇠ (k 1) k ni=1 Yi. Y 2 i=1 i. 135/152 Cochran’s Q test Experiment: A study investigates the performance of three drugs to treat a chronic disease. In total, n = 46 subjects received drugs A, B and C, and the response to each drug is classified as unfavourable (value 0) or favourable (value 1). I Observed data Drug A Drug B Drug C Count 1 1 1 6 1 1 0 16 1 0 1 2 1 0 0 4 0 1 1 2 0 1 0 4 0 0 1 6 0 0 0 6 Total Y.1 = 28 Y.2 = 28 Y.3 = 16 n = 46 I q = 8.4706 with p-value equal to 0.0145 < ↵ = 0.05 Answer: The probability of favourable response is significantly different for the three drugs at ↵ = 0.05 136/152 Cochran’s Q test Assumptions: I Response variables are binary (dichotomous) and belong to k matched or paired samples I Subjects (blocks) are independent and selected randomly from the population I Sample size is sufficiently large, i.e., number of subjects (blocks) with non-trivial results (not all 0’s of 1’s for the k categories) ne 4 and ne k 25 in order to have a good approximation of Q by means of the 2 distribution I McNemar test: k = 2 and ne = n10 + n01 25 (otherwise exact binomial McNemar test) 137/152 Friedman test Friedman test is a non-parametric test to detect differences in k treatments across n matched blocks with ordinal responses Experiment: In a study, 10 patients rated 4 different antidepressiva on a scale from 0 (little effect) to 5 (huge effect), and one is interested whether a specific treatment leads to consistently better results. Research question: Do these treatments have identical effects? 138/152 Friedman test I Observed data and ranking within matched blocks: Subject T1 Rank T2 Rank T3 Rank T4 Rank 1 0 1 5 4 1 2 4 3 2 3 2 4 3 2 1 5 4 3 1 1 4 3.5 3 2 4 3.5 4 4 4 2 1.5 2 1.5 3 3 5 2 1.5 2 1.5 4 4 3 3 6 0 1 3 2 5 3.5 5 3.5 7 3 2.5 1 1 3 2.5 4 4 8 5 3.5 3 2 1 1 5 3.5 9 1 1 5 4 2 2 4 3 10 2 2 4 4 0 1 3 3 R.j 19.5 26.5 20.5 33.5 R̄.j 1.95 2.65 2.05 3.35 R̄ 2.50 I Test statistic (nk30): P [12/(nk (k + 1))] kj=1 n2 R̄.j2 3n(k + 1) H0 2 Q= Pn PGi ⇠ (k 1) 1 (t 3 t )/(nk (k + 1)) i=1 j=1 ij ij 139/152 Friedman test I Gi = number of distinct values in the ith block (i = 1,... , n) I tij = number of observations equal to the jth smallest value (j = 1,... , Gi ) I q = 7.5/(1 36/200) = 9.146 > 20.95,3 = 7.815, p-value = 0.0274 < ↵ = 0.05 Answer: The four treatments have a significantly different effect on the patients at significance level ↵ = 0.05. 140/152 Friedman test Assumptions & Remarks: I Three or more paired samples with at least ordinal responses I Parametric alternative = repeated measures ANOVA I When n or k is small, the chi-square approximation becomes poor ) exact tables for the Friedman statistic I Significant test results can be followed by a post-hoc multiple comparisons tests to decide which groups are significantly different from each other I In case of dichotomous data, one uses Cochran’s Q test 141/152 Jonckheere-Terpstra test Jonckheere trend test or Jonckheere–Terpstra test is a test for an ordered alternative hypothesis for three or more independent samples (ordinal or continuous response variables). Experiment (revisited): One wants to study whether test performance (on a continuous scale ranging from 0 to 100) is different for different test anxiety levels (i.e., low, medium or high levels). Research question (revisited): Is there a significant a priori ordering in test performance implying performance from best to worst for students with low, medium and high test anxiety levels, respectively? 142/152 Jonckheere-Terpstra test I Observed data and corresponding ranks: anxiety Test performance score low 64 68 72 83 84 91 94 97 medium 25 37 49 54 59 81 82 high 13 41 49 52 55 82 Corresponding ranks Rij R̄i. low 11 12 13 17 18 19 20 21 16.4 medium 2 3 5.5 8 10 14 15.5 8.3 high 1 4 5.5 7 9 15.5 7.0 I Test hypotheses: 1 – low, 2 – medium, 3 – high H0 : 1 = 2 = 3 H1 : 1 2 3 I Equivalent alternative H1 : 3 2 1 I Generalization to g groups possible 143/152 Jonckheere-Terpstra test ‘Direct counting’ method I Arrange the samples in the predicted order I P = the total number of scores in the samples to the right which are larger than the score in question, for all scores I Q = the total number of scores in the samples to the right which are smaller than the score in question, for all scores ‘Nautical’ method I Ordered contingency table for the observed data: levels of independent variable increasing from left to right + values of the dependent variable increasing from top to bottom I P = the total number of entries that lie to the ‘South East’ of each entry in the table I Q = the total number of entries that lie tot the ‘South West’ of each entry in the table 144/152 Jonckheere-Terpstra test I Jonckheere-Terpstra test statistic: H 2 S=P Q ⇠0 N(0, S) where Pg Pg 2 2 n3 3 i=1 ni + 3 n2 2 i=1 ni S= 18 I Continuity correction: Sc = S sgn(S) ⇥ 1 I Tie correction (cj and ri column and row totals): ⇣ P 3 P 3⌘ ⇣ P 2 P 2⌘ 2 n3 ri cj + 3 n 2 ri cj + 5n 2 S = + ⇣P 18 ⌘⇣ ⌘ P P 3 P ri3 3 ri2 + 2n cj 3 cj2 + 2n + + 9n(n 1)(n 2) ⇣P ⌘⇣ P ⌘ ri2 n cj2 n + 2n(n 1) 145/152 Jonckheere-Terpstra test ‘Direct counting’ method I Samples in predicted order high medium low 13 25 64 41 37 68 49 49 72 52 54 83 55 59 84 82 81 91 82 94 97 I p = 15 + 13 + 12 + 12 + 11 + 8 + 8 + 8 + 8 + 8 + 8 + 5 + 5 = 121 I q = 0 + 2 + 2 + 3 + 4 + 6 + 0 + 0 + 0 + 0 + 0 + 3 + 3 = 23 I s = p q = 98 146/152 Jonckheere-Terpstra test ‘Nautical’ method – Ordered contingency table score high medium low total (ri ) 13 1 0 0 1 25 0 1 0 1 37 0 1 0 1 41 1 0 0 1 19 X 49 52 1 1 1 0 0 0 2 1 ri2 = 27 54 0 1 0 1 i=1 55 1 0 0 1 19 59 0 1 0 1 X 64 0 0 1 1 ri3 = 35 68 0 0 1 1 i=1 72 0 0 1 1 3 81 0 1 0 1 X 82 1 1 0 2 ci2 = 149 83 0 0 1 1 i=1 84 0 0 1 1 3 91 0 0 1 1 X 94 0 0 1 1 ci3 = 1071 97 0 0 1 1 i=1 total (ci ) 6 7 8 21 147/152 Jonckheere-Terpstra test I Standard normal approximation: Sc H0 Z = ⇠ N(0, 1) S I Variance of test statistic S (including tie correction): 2 (9261 35 1071) + 3 (441 27 149) + 105 ˆS2 = + 18 (35 81 + 42) (1071 447 + 42) + + 71820 (27 21) (149 21) + = 956.9883 840 p I Hence, z = (98 1)/ 956.9883 = 3.1356 > z1 ↵ = 1.645 for ↵ = 0.05; p-value = 0.0009 < ↵ = 0.05 Answer: Individuals with increasing anxiety levels perform decreasingly good at the test at ↵ = 0.05. 148/152 Jonckheere-Terpstra test Assumptions & Remarks: I Similar to the Kruskal-Wallis H test in that H0 : 1 = 2 = 3 , however, there is no a priori ordering of the populations from which the samples are drawn I When there is an a priori ordering, the Jonckheere test has more statistical power than the Kruskal-Wallis H test I Exact tables for S exist for small sample sizes 149/152 Part III References 150/152 References 151/152 References Hollander, M., Wolfe, D.A., and Chicken, E. (2014). Nonparametric Statistical Methods. Wiley: New York. Lehmann, E. L. (2006). Nonparametrics: Statistical Methods Based on Ranks. Springer: New York. Rosner, B. (2006). Fundamentals of Biostatistics. Thomson-Brooks/Cole: Duxbury Siegel, S. and Castellan, N.J. (1988). Nonparametric Statistics for the Behavioral Sciences. McGraw-Hill International Editions: New York Vanpaemel, D., Dierckx, G. Beirlant, J., and Hubert, M. (2015). Statistics and Science (in Dutch). Acco: Leuven. 152/152