An Introduction to Medical Statistics PDF
Document Details
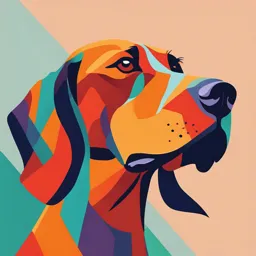
Uploaded by RegalActinium9897
University of Silesia in Katowice
2015
Martin Bland
Tags
Summary
This is a textbook on medical statistics, focusing on the practical application rather than the statistical theory. Written for a wide range of medical professionals, it highlights the importance of study design and data interpretation in medical research through many examples based on real studies. It includes exercises and multiple-choice questions to aid understanding.
Full Transcript
An Introduction to Medical Statistics An Introduction to Medical Statistics FOURTH EDITION Martin Bland Professor of Health Statistics Department of Health Sciences University of York 3 3 Great Clare...
An Introduction to Medical Statistics An Introduction to Medical Statistics FOURTH EDITION Martin Bland Professor of Health Statistics Department of Health Sciences University of York 3 3 Great Clarendon Street, Oxford, OX2 6DP, United Kingdom Oxford University Press is a department of the University of Oxford. It furthers the University’s objective of excellence in research, scholarship, and education by publishing worldwide. Oxford is a registered trade mark of Oxford University Press in the UK and in certain other countries © Oxford University Press 2015 The moral rights of the author have been asserted First Edition published in 1987 Second Edition published in 1995 Third Edition published in 2000 Fourth Edition published in 2015 Impression: 1 All rights reserved. No part of this publication may be reproduced, stored in a retrieval system, or transmitted, in any form or by any means, without the prior permission in writing of Oxford University Press, or as expressly permitted by law, by licence or under terms agreed with the appropriate reprographics rights organization. Enquiries concerning reproduction outside the scope of the above should be sent to the Rights Department, Oxford University Press, at the address above You must not circulate this work in any other form and you must impose this same condition on any acquirer Published in the United States of America by Oxford University Press 198 Madison Avenue, New York, NY 10016, United States of America British Library Cataloguing in Publication Data Data available Library of Congress Control Number: 2014959481 ISBN 978–0–19–958992–0 Printed in Italy by L.E.G.O. S.p.A. Oxford University Press makes no representation, express or implied, that the drug dosages in this book are correct. Readers must therefore always check the product information and clinical procedures with the most up-to-date published product information and data sheets provided by the manufacturers and the most recent codes of conduct and safety regulations. The authors and the publishers do not accept responsibility or legal liability for any errors in the text or for the misuse or misapplication of material in this work. Except where otherwise stated, drug dosages and recommendations are for the non-pregnant adult who is not breast-feeding Links to third party websites are provided by Oxford in good faith and for information only. Oxford disclaims any responsibility for the materials contained in any third party website referenced in this work. To Emily and Nicholas Bland Preface to the Fourth Edition This book is for medical students, doctors, medical I have included some new examples, though many researchers, nurses, members of professions allied to of the old ones remain, being too good to replace, I medicine, and all others concerned with medical data. thought. I have changed most of the exercises, to re- When I wrote the first edition of An Introduction to move all calculations. I never touch a calculator now, so Medical Statistics, I based the contents on the statis- why should my readers? Instead, I have concentrated on tical methods which appeared frequently in the Lancet understanding and interpreting analyses. I have dropped and the British Medical Journal. I continued to do this the stars for sections with material which was beyond the with each succeeding edition. Each time, the range and undergraduate course. I no longer teach medical or nurs- complexity of the methods used increased. There are ing students and I do not have my finger on that pulse. two reasons for this. One is that the size and com- All the graphs have been redrawn using Stata12, except plexity of medical research studies has increased greatly for one pie chart, done using Excel. and, I think, the quality has increased greatly, too. The This is a book about data, not statistical theory. The other reason is that developments in computing have fundamental concepts of study design, data collection, enabled statisticians to develop and bring into use new, and data analysis are explained by illustration and ex- computer-intensive methods of analysis and these have ample. Only enough mathematics and formulae are been applied in medical research. given to make clear what is going on. For those who In this fourth edition, I have added new chapters on wish to go a little further in their understanding, some meta-analysis and on handling missing data by multiple of the more mathematical background to the techniques imputation, methods now seen routinely in major jour- described is given as appendices to the chapters rather nals. I have also added a chapter explaining the Bayesian than in the main text. approach to data, including Markov Chain Monte Carlo The book is firmly grounded in medical data, particu- methods of analysis. I have added a new chapter collect- larly in medical research, and the interpretation of the ing together and expanding the material on time to event results of calculations in their medical context is empha- or survival data. I have also added new sections on allo- sized. Except for a few obviously invented numbers used cation by minimization, bootstrap methods, Poisson and to illustrate the mechanics of calculations, all the data in negative binomial regression, kappa statistics for agree- the examples and exercises are real, from my own re- ment between observers, and the creation of composite search and statistical consultation or from the medical scales using principal components and factor analysis, all literature. things you will see in medical journals. There are two kinds of exercise in this book. Each Apart from changes in the practice of statistics in medi- chapter has a set of multiple choice questions of the ‘true cine in general, I hope that I have changed a bit, too. or false’ type, 122 in all. Multiple choice questions can Since writing the third edition, I have moved to a different cover a large amount of material in a short time, so are university, where I now spend a lot more time on clinical a useful tool for revision. As MCQs are widely used in trials. I have also spent 6 years on the Clinical Evaluation postgraduate examinations, these exercises should also and Trials Board of the Health Technology Assessment be useful to those preparing for memberships. All the programme, reading and criticising hundreds of grant ap- MCQs have solutions, with reference to an appropriate plications. I hope that I have learned something along the part of the text or a detailed explanation for most of the way and I have revised the text accordingly. answers. Each chapter also has a long exercise, also with viii Preface to the Fourth Edition suggested answers, mostly on the interpretation of data Maugdal, Douglas Maxwell, Georgina Morris, Charles in published studies. Mutoka, Tim Northfield, Andreas Papadopoulos, Mo- I wish to thank many people who have contributed to hammed Raja, Paul Richardson, and Alberto Smith. I am the writing of this book. First, there are the many medical particularly indebted to John Morgan, as Chapter 21 is students, doctors, research workers, nurses, physiother- partly based on his work. apists, and radiographers whom it has been my pleasure I thank Douglas Altman, Daniel Heitjan, David Jones, to teach, and from whom I have learned so much. Klim McPherson, Janet Peacock, Stuart Pocock, and Second, the book contains many examples drawn from Robin Prescott for their helpful comments on earlier research carried out with other statisticians, epidemiolo- drafts and Dan Heitjan for finding mistakes in this one. gists, and social scientists, particularly Douglas Altman, I am very grateful to Julian Higgins and Simon Crouch for Ross Anderson, Mike Banks, Barbara Butland, Beulah their comments on my new chapters on meta-analysis Bewley, Nicky Cullum, Jo Dumville, Walter Holland, and and Bayesian methods, respectively. I am grateful to John David Torgerson. These studies could not have been Blase for help with converting my only Excel graphic. done without the assistance of Patsy Bailey, Bob Harris, I have corrected a number of errors from earlier edi- Rebecca McNair, Janet Peacock, Swatee Patel, and Vir- tions, and I am grateful to colleagues who have pointed ginia Pollard. Third, the clinicians and scientists with them out to me. Most of all I thank Pauline Bland for her whom I have collaborated or who have come to me for unfailing confidence and encouragement. statistical advice not only taught me about medical data Since the last edition of this book, my children, Nick but many of them have left me with data which are used and Em, have grown up and have both become health here, including Naib Al-Saady, Thomas Bewley, Frances researchers. It is to them I dedicate this fourth edition. Boa, Nigel Brown, Jan Davies, Caroline Flint, Nick Hall, Tessi Hanid, Michael Hutt, Riahd Jasrawi, Ian Johnston, M.B. Moses Kapembwa, Pam Luthra, Hugh Mather, Daram York, April 2015 Contents Detailed Contents xi Chapter 1 Introduction 1 Chapter 2 The design of experiments 5 Chapter 3 Sampling and observational studies 25 Chapter 4 Summarizing data 41 Chapter 5 Presenting data 57 Chapter 6 Probability 73 Chapter 7 The Normal distribution 85 Chapter 8 Estimation 101 Chapter 9 Significance tests 115 Chapter 10 Comparing the means of small samples 131 Chapter 11 Regression and correlation 159 Chapter 12 Methods based on rank order 177 Chapter 13 The analysis of cross-tabulations 193 Chapter 14 Choosing the statistical method 213 Chapter 15 Multifactorial methods 223 Chapter 16 Time to event data 251 Chapter 17 Meta-analysis 265 Chapter 18 Determination of sample size 295 Chapter 19 Missing data 305 Chapter 20 Clinical measurement 313 Chapter 21 Mortality statistics and population structure 347 Chapter 22 The Bayesian approach 357 Appendix 1: Suggested answers to multiple choice questions and exercises 367 References 397 Index 411 Detailed Contents Chapter 1 Introduction 1 1.1 Statistics and medicine 1 1.2 Statistics and mathematics 1 1.3 Statistics and computing 2 1.4 Assumptions and approximations 2 1.5 The scope of this book 3 Chapter 2 The design of experiments 5 2.1 Comparing treatments 5 2.2 Random allocation 6 2.3 Stratification 10 2.4 Methods of allocation without random numbers 10 2.5 Volunteer bias 12 2.6 Intention to treat 13 2.7 Cross-over designs 13 2.8 Selection of subjects for clinical trials 15 2.9 Response bias and placebos 15 2.10 Assessment bias and double blind studies 17 2.11 Laboratory experiments 18 2.12 Experimental units and cluster randomized trials 18 2.13 Consent in clinical trials 20 2.14 Minimization 21 2.15 Multiple choice questions: Clinical trials 23 2.16 Exercise: The ‘Know Your Midwife’ trial 23 Chapter 3 Sampling and observational studies 25 3.1 Observational studies 25 3.2 Censuses 26 3.3 Sampling 26 3.4 Random sampling 27 3.5 Sampling in clinical and epidemiological studies 29 3.6 Cross-sectional studies 31 3.7 Cohort studies 32 3.8 Case–control studies 33 xii Detailed Contents 3.9 Questionnaire bias in observational studies 35 3.10 Ecological studies 36 3.11 Multiple choice questions: Observational studies 37 3.12 Exercise: Campylobacter jejuni infection 38 Chapter 4 Summarizing data 41 4.1 Types of data 41 4.2 Frequency distributions 41 4.3 Histograms and other frequency graphs 44 4.4 Shapes of frequency distribution 47 4.5 Medians and quantiles 49 4.6 The mean 50 4.7 Variance, range, and interquartile range 51 4.8 Standard deviation 52 4.9 Multiple choice questions: Summarizing data 53 4.10 Exercise: Student measurements and a graph of study numbers 54 Appendix 4A: The divisor for the variance 55 Appendix 4B: Formulae for the sum of squares 56 Chapter 5 Presenting data 57 5.1 Rates and proportions 57 5.2 Significant figures 58 5.3 Presenting tables 60 5.4 Pie charts 61 5.5 Bar charts 61 5.6 Scatter diagrams 63 5.7 Line graphs and time series 65 5.8 Misleading graphs 66 5.9 Using different colours 68 5.10 Logarithmic scales 68 5.11 Multiple choice questions: Data presentation 69 5.12 Exercise: Creating presentation graphs 70 Appendix 5A: Logarithms 70 Chapter 6 Probability 73 6.1 Probability 73 6.2 Properties of probability 73 6.3 Probability distributions and random variables 74 6.4 The Binomial distribution 75 6.5 Mean and variance 77 6.6 Properties of means and variances 77 Detailed Contents xiii 6.7 The Poisson distribution 79 6.8 Conditional probability 79 6.9 Multiple choice questions: Probability 81 6.10 Exercise: Probability in court 81 Appendix 6A: Permutations and combinations 82 Appendix 6B: Expected value of a sum of squares 82 Chapter 7 The Normal distribution 85 7.1 Probability for continuous variables 85 7.2 The Normal distribution 86 7.3 Properties of the Normal distribution 89 7.4 Variables which follow a Normal distribution 92 7.5 The Normal plot 93 7.6 Multiple choice questions: The Normal distribution 96 7.7 Exercise: Distribution of some measurements obtained by students 97 Appendix 7A: Chi-squared, t, and F 98 Chapter 8 Estimation 101 8.1 Sampling distributions 101 8.2 Standard error of a sample mean 102 8.3 Confidence intervals 104 8.4 Standard error and confidence interval for a proportion 105 8.5 The difference between two means 105 8.6 Comparison of two proportions 106 8.7 Number needed to treat 108 8.8 Standard error of a sample standard deviation 109 8.9 Confidence interval for a proportion when numbers are small 109 8.10 Confidence interval for a median and other quantiles 110 8.11 Bootstrap or resampling methods 111 8.12 What is the correct confidence interval? 112 8.13 Multiple choice questions: Confidence intervals 112 8.14 Exercise: Confidence intervals in two acupuncture studies 113 Appendix 8A: Standard error of a mean 114 Chapter 9 Significance tests 115 9.1 Testing a hypothesis 115 9.2 An example: the sign test 116 9.3 Principles of significance tests 116 9.4 Significance levels and types of error 117 9.5 One and two sided tests of significance 118 9.6 Significant, real, and important 119 xiv Detailed Contents 9.7 Comparing the means of large samples 120 9.8 Comparison of two proportions 121 9.9 The power of a test 122 9.10 Multiple significance tests 123 9.11 Repeated significance tests and sequential analysis 125 9.12 Significance tests and confidence intervals 126 9.13 Multiple choice questions: Significance tests 126 9.14 Exercise: Crohn’s disease and cornflakes 127 Chapter 10 Comparing the means of small samples 131 10.1 The t distribution 131 10.2 The one sample t method 134 10.3 The means of two independent samples 136 10.4 The use of transformations 138 10.5 Deviations from the assumptions of t methods 141 10.6 What is a large sample? 142 10.7 Serial data 142 10.8 Comparing two variances by the F test 144 10.9 Comparing several means using analysis of variance 145 10.10 Assumptions of the analysis of variance 147 10.11 Comparison of means after analysis of variance 148 10.12 Random effects in analysis of variance 150 10.13 Units of analysis and cluster randomized trials 152 10.14 Multiple choice questions: Comparisons of means 153 10.15 Exercise: Some analyses comparing means 155 Appendix 10A: The ratio mean/standard error 156 Chapter 11 Regression and correlation 159 11.1 Scatter diagrams 159 11.2 Regression 160 11.3 The method of least squares 160 11.4 The regression of X on Y 162 11.5 The standard error of the regression coefficient 163 11.6 Using the regression line for prediction 164 11.7 Analysis of residuals 165 11.8 Deviations from assumptions in regression 166 11.9 Correlation 167 11.10 Significance test and confidence interval for r 169 11.11 Uses of the correlation coefficient 170 11.12 Using repeated observations 171 11.13 Intraclass correlation 172 11.14 Multiple choice questions: Regression and correlation 173 Detailed Contents xv 11.15 Exercise: Serum potassium and ambient temperature 174 Appendix 11A: The least squares estimates 174 Appendix 11B: Variance about the regression line 175 Appendix 11C: The standard error of b 175 Chapter 12 Methods based on rank order 177 12.1 Non-parametric methods 177 12.2 The Mann–Whitney U test 177 12.3 The Wilcoxon matched pairs test 182 12.4 Spearman’s rank correlation coefficient, ρ 185 12.5 Kendall’s rank correlation coefficient, τ 187 12.6 Continuity corrections 188 12.7 Parametric or non-parametric methods? 189 12.8 Multiple choice questions: Rank-based methods 190 12.9 Exercise: Some applications of rank-based methods 190 Chapter 13 The analysis of cross-tabulations 193 13.1 The chi-squared test for association 193 13.2 Tests for 2 by 2 tables 195 13.3 The chi-squared test for small samples 196 13.4 Fisher’s exact test 197 13.5 Yates’ continuity correction for the 2 by 2 table 199 13.6 The validity of Fisher’s and Yates’ methods 199 13.7 Odds and odds ratios 200 13.8 The chi-squared test for trend 202 13.9 Methods for matched samples 204 13.10 The chi-squared goodness of fit test 205 13.11 Multiple choice questions: Categorical data 207 13.12 Exercise: Some analyses of categorical data 208 Appendix 13A: Why the chi-squared test works 209 Appendix 13B: The formula for Fisher’s exact test 210 Appendix 13C: Standard error for the log odds ratio 211 Chapter 14 Choosing the statistical method 213 14.1 Method oriented and problem oriented teaching 213 14.2 Types of data 213 14.3 Comparing two groups 214 14.4 One sample and paired samples 215 14.5 Relationship between two variables 216 14.6 Multiple choice questions: Choice of statistical method 218 14.7 Exercise: Choosing a statistical method 218 xvi Detailed Contents Chapter 15 Multifactorial methods 223 15.1 Multiple regression 223 15.2 Significance tests and estimation in multiple regression 225 15.3 Using multiple regression for adjustment 227 15.4 Transformations in multiple regression 228 15.5 Interaction in multiple regression 230 15.6 Polynomial regression 231 15.7 Assumptions of multiple regression 232 15.8 Qualitative predictor variables 233 15.9 Multi-way analysis of variance 234 15.10 Logistic regression 237 15.11 Stepwise regression 239 15.12 Seasonal effects 239 15.13 Dealing with counts: Poisson regression and negative binomial regression 240 15.14 Other regression methods 244 15.15 Data where observations are not independent 244 15.16 Multiple choice questions: Multifactorial methods 245 15.17 Exercise: A multiple regression analysis 246 Chapter 16 Time to event data 251 16.1 Time to event data 251 16.2 Kaplan–Meier survival curves 251 16.3 The logrank test 256 16.4 The hazard ratio 258 16.5 Cox regression 259 16.6 Multiple choice questions: Time to event data 261 16.7 Exercise: Survival after retirement 263 Chapter 17 Meta-analysis 265 17.1 What is a meta-analysis? 265 17.2 The forest plot 265 17.3 Getting a pooled estimate 267 17.4 Heterogeneity 268 17.5 Measuring heterogeneity 268 17.6 Investigating sources of heterogeneity 270 17.7 Random effects models 272 17.8 Continuous outcome variables 274 17.9 Dichotomous outcome variables 279 17.10 Time to event outcome variables 282 17.11 Individual participant data meta-analysis 283 17.12 Publication bias 284 Detailed Contents xvii 17.13 Network meta-analysis 289 17.14 Multiple choice questions: Meta-analysis 290 17.15 Exercise: Dietary sugars and body weight 292 Chapter 18 Determination of sample size 295 18.1 Estimation of a population mean 295 18.2 Estimation of a population proportion 296 18.3 Sample size for significance tests 296 18.4 Comparison of two means 297 18.5 Comparison of two proportions 299 18.6 Detecting a correlation 300 18.7 Accuracy of the estimated sample size 301 18.8 Trials randomized in clusters 302 18.9 Multiple choice questions: Sample size 303 18.10 Exercise: Estimation of sample sizes 304 Chapter 19 Missing data 305 19.1 The problem of missing data 305 19.2 Types of missing data 306 19.3 Using the sample mean 307 19.4 Last observation carried forward 307 19.5 Simple imputation 308 19.6 Multiple imputation 309 19.7 Why we should not ignore missing data 310 19.8 Multiple choice questions: Missing data 311 19.9 Exercise: Last observation carried forward 312 Chapter 20 Clinical measurement 313 20.1 Making measurements 313 20.2 Repeatability and measurement error 315 20.3 Assessing agreement using Cohen’s kappa 317 20.4 Weighted kappa 322 20.5 Comparing two methods of measurement 324 20.6 Sensitivity and specificity 326 20.7 Normal range or reference interval 329 20.8 Centile charts 331 20.9 Combining variables using principal components analysis 332 20.10 Composite scales and subscales 335 20.11 Internal consistency of scales and Cronbach’s alpha 341 20.12 Presenting composite scales 341 20.13 Multiple choice questions: Measurement 342 20.14 Exercise: Two measurement studies 344 xviii Detailed Contents Chapter 21 Mortality statistics and population structure 347 21.1 Mortality rates 347 21.2 Age standardization using the direct method 348 21.3 Age standardization by the indirect method 349 21.4 Demographic life tables 350 21.5 Vital statistics 353 21.6 The population pyramid 354 21.7 Multiple choice questions: Population and mortality 355 21.8 Exercise: Mortality and type 1 diabetes 356 Chapter 22 The Bayesian approach 357 22.1 Bayesians and Frequentists 357 22.2 Bayes’ theorem 357 22.3 An example: the Bayesian approach to computer- aided diagnosis 357 22.4 The Bayesian and frequency views of probability 358 22.5 An example of Bayesian estimation 358 22.6 Prior distributions 361 22.7 Maximum likelihood 361 22.8 Markov Chain Monte Carlo methods 362 22.9 Bayesian or Frequentist? 364 22.10 Multiple choice questions: Bayesian methods 364 22.11 Exercise: A Bayesian network meta-analysis 365 Appendix 1: Suggested answers to multiple choice questions and exercises 367 References 397 Index 411 1 1 Introduction 1.1 Statistics and medicine based on sampling theory, which had been developed by Fisher and others, were introduced into medical research, Evidence-based practice is the watchword in every pro- notably by Bradford Hill. It rapidly became apparent that fession concerned with the treatment and prevention research in medicine raised many new problems in both of disease and the promotion of health and well-being. design and analysis, and much work has been done This requires both the gathering of evidence and its crit- since towards solving these by clinicians, statisticians, and ical interpretation. The former is bringing more people epidemiologists. into the practice of research, and the latter is requir- Although considerable progress has been made in ing of all health professionals the ability to evaluate the such fields as the design of clinical trials, there remains research carried out. Much of this evidence is in the much to be done in developing research methodology form of numerical data. The essential skill required for in medicine. It seems likely that this will always be so, the collection, analysis, and evaluation of numerical data for every research project is something new, something is Statistics. Thus Statistics, the science of assembling which has never been done before. Under these circum- and interpreting numerical data, is the core science of stances we make mistakes. No piece of research can be evidence-based practice. perfect and there will always be something which, with In the past 40 years, medical research has become hindsight, we would have changed. Furthermore, it is of- deeply involved with the techniques of statistical infer- ten from the flaws in a study that we can learn most ence. The work published in medical journals is full of about research methods. For this reason, the work of statistical jargon and the results of statistical calculations. several researchers is described in this book to illustrate This acceptance of statistics, though gratifying to the the problems into which their designs or analyses led medical statistician, may even have gone too far. More them. I do not wish to imply that these people were than once I have told a colleague that he did not need any more prone to error than the rest of the human me to prove that his difference existed, as anyone could race, or that their work was not a valuable and serious see it, only to be told in turn that without the magic of undertaking. Rather I want to learn from their experi- the P value he could not have his paper published. ence of attempting something extremely difficult, trying Statistics has not always been so popular with the to extend our knowledge, so that researchers and con- medical profession. Statistical methods were first used in sumers of research may avoid these particular pitfalls in medical research in the 19th century by workers such the future. as Pierre-Charles-Alexandre Louis, William Farr, Flor- ence Nightingale, and John Snow. Snow’s studies of the modes of communication of cholera, for example, made use of epidemiological techniques upon which 1.2 Statistics and mathematics we have still made little improvement. Despite the work Many people are discouraged from the study of Statis- of these pioneers, however, statistical methods did not tics by a fear of being overwhelmed by mathematics. It become widely used in clinical medicine until the mid- is true that many professional statisticians are also math- dle of the 20th century. It was then that the methods ematicians, but not all are, and there are many very able of randomized experimentation and statistical analysis appliers of statistics to their own fields. It is possible, 2 Chapter 1 Introduction though perhaps not very useful, to study statistics simply There is therefore no need to consider the prob- as a part of mathematics, with no concern for its ap- lems of manual calculation in detail. The important plication at all. Statistics may also be discussed without thing is to know why particular calculations should be appearing to use any mathematics at all (e.g. Huff 1954). done and what the results of these calculations ac- The aspects of statistics described in this book can tually mean. Indeed, the danger in the computer age be understood and applied with the use of simple al- is not so much that people carry out complex cal- gebra. Only the algebra which is essential for explaining culations wrongly, but that they apply very compli- the most important concepts is given in the main text. cated statistical methods without knowing why or what This means that several of the theoretical results used are the computer output means. More than once I have stated without a discussion of their mathematical basis. been approached by a researcher, bearing a two inch This is done when the derivation of the result would not thick computer printout and asking what it all means. aid much in understanding the application. For many Sadly, too often, it means that another tree has died readers the reasoning behind these results is not of in vain. great interest. For the reader who does not wish to take The widespread availability of computers means that these results on trust, several chapters have appendices more calculations are being done, and being published, in which simple mathematical proofs are given. These than ever before, and the chance of inappropriate statis- appendices are designed to help increase the under- tical methods being applied may actually have increased. standing of the more mathematically inclined reader and This misuse arises partly because people regard their data to be omitted by those who find that the mathematics analysis problems as computing problems, not statistical serves only to confuse. ones, and seek advice from computer experts rather than statisticians. They often get good advice on how to do it, but rather poor advice about what to do, why to do it, 1.3 Statistics and computing and how to interpret the results afterwards. It is therefore more important than ever that the consumers of research Practical statistics has always involved large amounts of understand something about the uses and limitations of calculation. When the methods of statistical inference statistical techniques. were being developed in the first half of the 20th cen- tury, calculations were done using pencil, paper, tables, slide rules, and, with luck, a very expensive mechanical 1.4 Assumptions adding machine. Older books on statistics spend much and approximations time on the details of carrying out calculations and any reference to a ‘computer’ means a person who computes, Many statistical calculations give answers which are ap- not an electronic device. The development of the digital proximate rather than exact. For example, if we carry computer has brought changes to statistics as to many out a clinical trial and obtain a difference in outcome other fields. Calculations can be done quickly, easily, between two treatment groups, this applies only to the and, we hope, accurately with a range of machines from people in the trial. It is people not in the trial, those who pocket calculators with built-in statistical functions to are yet to come and to be eligible for the trial treatments, powerful computers analysing data on many thousands for whom we want to know the difference. Our trial can of subjects. Many statistical methods would not be con- only give an approximate estimate of what that might be. templated without computers, and the development of As we shall see, statistical methods enable us to get an new methods goes hand in hand with the development idea of how precise our estimate could be, but this, too, of software to carry them out. The theory of multilevel is only approximate. It depends on some assumptions modelling (Goldstein 1995) and the programs MLn and about how the data behave. We have to assume that MLWin are a good example. Most of the calculations in our data fit some kind of idealized mathematical model. this book were done using a computer and the graphs The great statistician George Box said that ‘essentially, all were produced with one. models are wrong, but some are useful’. We might add 1.5 The scope of this book 3 that, in medical statistics, all answers are approximate, covered. Like most such books, this one has exer- but some approximations are useful. cises at the end of each chapter, but to ease the te- The important thing will be to have an idea of how dium many of these are of the multiple choice type. good our approximations are. We shall spend quite a There is also a long exercise, usually involving inter- lot of time investigating the assumptions underlying our pretation of results rather than calculations, for each statistical methods, to see how plausible they are for chapter. The exercises can be completed quite quickly our data. and the reader is advised to try them. Solutions are given at the end of the book, in full for the long ex- ercises and as brief notes with references to the rele- vant sections in the text for MCQs. Readers who would 1.5 The scope of this book like more numerical exercises are recommended to This book is intended as an introduction to some of see Osborn (1979). For a wealth of exercises in the the statistical ideas important to medicine. It does not understanding and interpretation of statistics in med- tell you all you need to know to do medical research. ical research, drawn from the published literature and Once you have understood the concepts discussed here, popular media, you should try the companion volume to it is much easier to learn about the techniques of study this one, Statistical Questions in Evidence-based Medicine design and statistical analysis required to answer any (Bland and Peacock 2000). particular question. There are several excellent standard If you would like to try some of the analyses described, works which describe the solutions to problems in the you can download some of the datasets from my website analysis of data (Armitage et al. 2002; Altman 1991) and (martinbland.co.uk). also more specialized books to which reference will be Finally, a question many students of medicine ask as made where required. It is also worth noting that, just they struggle with statistics: is it worth it? As Altman like Medicine, Statistics does not stand still. Statisticians (1982) has argued, bad statistics leads to bad research and other researchers are continually trying to develop and bad research is unethical. Not only may it give mis- new methods, often exploiting advances in computing, leading results, which can result in good therapies being to give better answers to questions than those we have abandoned and bad ones adopted, but it means that now. The basic principles explained in this book should patients may have been exposed to potentially harm- apply to all of them. ful new treatments for no good reason. Medicine is a What I hope the book will do is to give enough rapidly changing field. In 10 years’ time, many of the ther- understanding of the statistical ideas commonly used in apies currently prescribed and many of our ideas about medicine to enable the health professional to read the the causes and prevention of disease will be obsolete. medical literature competently and critically. It covers They will be replaced by new therapies and new theor- enough material (and more) for an undergraduate course ies, supported by research studies and data of the kind in Statistics for students of medicine, nursing, physio- described in this book, and probably presenting many of therapy, etc. At the time of writing, as far as can be the same problems in interpretation. The practitioner will established, it covers the material required to answer stat- be expected to decide for her- or himself what to pre- istical questions set in the examinations of most of the scribe or advise based on these studies. So a knowledge Royal Colleges. of medical statistics is one of the most useful things any When working through a textbook, it is useful to doctor, nurse, dentist, or physiotherapist could acquire be able to check your understanding of the material during her or his training. 5 2 The design of experiments 2.1 Comparing treatments disease itself may change. All these factors may produce changes in the patients’ apparent response to treatment. There are two broad types of study in medical research: For example, Christie (1979) showed this by studying the observational and experimental. In observational studies, survival of stroke patients in 1978, after the introduction aspects of an existing situation are observed, as in a sur- of a C-T head scanner, with that of patients treated in vey or a clinical case report. We then try to interpret our 1974, before the introduction of the scanner. He took the data to give an explanation of how the observed state records of a group of patients treated in 1978, who re- of affairs has come about. In experimental studies, we ceived a C-T scan, and matched each of them with a pa- do something, such as giving a drug, so that we can ob- tient treated in 1974 of the same age, diagnosis, and level serve the result of our action. This chapter is concerned of consciousness on admission. As the first column of with the way statistical thinking is involved in the design Table 2.1 shows, patients in 1978 clearly tended to have of experiments. In particular, it deals with comparative better survival than similar patients in 1974. The scanned experiments where we wish to study the difference be- 1978 patient did better than the unscanned 1974 patient tween the effects of two or more treatments. These in 31% of pairs, whereas the unscanned 1974 patient experiments may be carried out in the laboratory in vitro did better than the scanned 1978 patient in only 7% of or on animals or human volunteers, in the hospital or pairs. However, he also compared the survival of patients community on human patients, or, for trials of prevent- in 1978 who did not receive a C-T scan with matched ive interventions, on currently healthy people. We call patients in 1974. These patients also showed a marked trials of treatments on human subjects clinical trials. improvement in survival from 1974 to 1978 (Table 2.1). The general principles of experimental design are the The 1978 patient did better in 38% of pairs and the same, although there are special precautions which must 1974 patients in only 19% of pairs. There was a general be taken when experimenting with human subjects. The improvement in outcome over a fairly short period of experiments whose results most concern clinicians are time. If we did not have the data on the unscanned clinical trials, so the discussion will deal mainly with them. Suppose we want to know whether a new treatment is more effective than the present standard treatment. We Table 2.1 Analysis of the difference in survival for matched pairs of stroke patients (data from Christie 1979) could approach this in a number of ways. First, we could compare the results of the new treat- C-T scan No C-T scan ment on new patients with records of previous results in 1978 in 1978 using the old treatment. This is seldom convincing because there may be many differences between the Pairs with 1978 better 9 (31%) 34 (38%) patients who received the old treatment and the patients than 1974 who will receive the new. As time passes, the general Pairs with same 18 (62%) 38 (43%) population from which patients are drawn may become outcome healthier, standards of ancillary treatment and nursing Pairs with 1978 worse 2 (7%) 17 (19%) care may improve, or the social mix in the catchment than 1974 area of the hospital may change. The nature of the 6 Chapter 2 The design of experiments patients from 1978, we might be tempted to interpret were not vaccinated. Between 1927 and 1932, physicians these data as evidence for the effectiveness of the C-T vaccinated half the children, the choice of which chil- scanner. Historical controls like this are seldom very con- dren to vaccinate being left to them. There was a clear vincing, and usually favour the new treatment. We need advantage in survival for the BCG group (Table 2.2). How- to compare the old and new treatments concurrently. ever, there was also a clear tendency for the physician Second, we could obtain concurrent groups by com- to vaccinate the children of more cooperative parents, paring our own patients, given the new treatment, with and to leave those of less cooperative parents as con- patients given the standard treatment in another hospital trols. From 1933, allocation to treatment or control was or clinic, or by another clinician in our own institution. done centrally, alternate children being assigned to con- Again, there may be differences between the patient trol and vaccine. The difference in degree of cooperation groups due to catchment, diagnostic accuracy, prefer- between the parents of the two groups of children largely ence by patients for a particular clinician, or you might disappeared, and so did the difference in mortality. Note just be a better therapist. We cannot separate these that these were a special group of children, from families differences from the treatment effect. where there was tuberculosis. In large trials using children Third, we could ask people to volunteer for the new drawn from the general population, BCG was shown to treatment and give the standard treatment to those who be effective in greatly reducing deaths from tuberculosis do not volunteer. The difficulty here is that people who (Hart and Sutherland 1977). volunteer and people who do not volunteer are likely Different methods of allocation to treatment can prod- to be different in many ways, apart from the treatments uce different results. This is because the method of we give them. Volunteers might be more likely to fol- allocation may not produce groups of subjects which low medical advice, for example. We will consider an are comparable, similar in every respect except the treat- example of the effects of volunteer bias in Section 2.5. ment. We need a method of allocation to treatments in Fourth, we can allocate patients to the new treatment which the characteristics of subjects will not affect their or the standard treatment and observe the outcome. The chance of being put into any particular group. This can way in which patients are allocated to treatments can in- be done using random allocation. fluence the results enormously, as the following example (Hill 1962) shows. Between 1927 and 1944, a series of trials of BCG vaccine were carried out in New York (Lev- 2.2 Random allocation ine and Sackett 1946). Children from families where there was a case of tuberculosis were allocated to a vaccination If we want to decide which of two people receive an ad- group and given BCG vaccine, or to a control group who vantage, in such a way that each has an equal chance Table 2.2 Results of studies of BCG vaccine in New York City (data from Hill 1962) Period No. of No. of Death Average no. of visits to Proportion of parents of trial children deaths rate clinic during 1st year of giving good cooperation from TB follow-up as judged by visiting nurses 1927–32 Selection made by physician: BCG group 445 3 0.67% 3.6 43% Control group 545 18 3.30% 1.7 24% 1933–44 Alternate selection carried out centrally: BCG group 566 8 1.41% 2.8 40% Control group 528 8 1.52% 2.4 34% Table 2.3 1 040 random digits Column Row 1–4 5–8 9–12 13–16 17–20 21–24 25–28 29–32 33–36 37–40 41–44 45–48 49–52 53–56 57–60 61–64 65–68 69–72 73–76 77–80 1 36 45 88 31 28 73 59 43 46 32 00 32 67 15 32 49 54 55 75 17 90 51 40 66 18 46 95 54 65 89 16 80 95 33 15 88 18 60 56 46 2 98 41 90 22 48 37 80 31 91 39 33 80 40 82 38 26 20 39 71 82 55 25 71 27 14 68 64 04 99 24 82 30 73 43 92 68 18 99 47 54 3 02 99 10 75 77 21 88 55 79 97 70 32 59 87 75 35 18 34 62 53 79 85 55 66 63 84 08 63 04 00 18 34 53 94 58 01 55 05 90 99 4 33 53 95 28 06 81 34 95 13 93 37 16 95 06 15 91 89 99 37 16 74 75 13 13 22 16 37 76 15 57 42 38 96 23 90 24 58 26 71 46 5 06 66 30 43 00 66 32 60 36 60 46 05 17 31 66 80 91 01 62 35 92 83 31 60 87 30 76 83 17 85 31 48 13 23 17 32 68 14 84 96 6 61 21 31 49 98 29 77 70 72 11 35 23 69 47 14 27 14 74 52 35 27 82 01 01 74 41 38 77 53 68 53 26 55 16 35 66 31 87 82 09 7 61 05 50 10 94 85 86 32 10 72 95 67 88 21 72 09 48 73 03 97 11 57 85 67 94 91 49 48 35 49 39 41 80 17 54 45 23 66 82 60 8 15 16 08 90 92 86 13 32 26 01 20 02 72 45 94 74 97 19 99 46 22 09 29 66 15 44 76 74 94 92 48 13 75 85 81 28 95 41 36 30 9 69 13 53 55 35 87 43 23 83 32 79 40 92 20 83 76 82 61 24 20 08 29 79 37 00 33 35 34 86 55 10 91 18 86 43 50 67 79 33 58 10 37 29 99 85 55 63 32 66 71 98 85 20 31 93 63 91 77 21 99 62 65 11 14 04 88 86 28 92 04 03 42 99 87 08 20 55 30 53 82 24 11 66 22 81 58 30 80 21 10 15 53 26 90 33 77 51 19 17 49 27 14 37 21 77 13 69 31 20 22 67 13 46 29 75 32 69 79 39 23 32 43 12 51 43 09 72 68 38 05 77 14 62 89 07 37 89 25 30 92 09 06 92 31 59 37 83 92 55 15 31 21 24 03 93 35 97 84 61 96 85 45 51 13 79 05 43 69 52 93 00 77 44 82 91 65 11 71 25 37 89 13 63 87 04 30 69 08 33 81 34 92 69 86 35 37 51 81 47 95 13 55 48 33 8 Chapter 2 The design of experiments of receiving it, we can use a simple, widely accepted odd digits to group A and those corresponding to even method. We toss a coin. This is used to decide the way digits to B. The first digit, 6, is even, so the first subject football matches begin, for example, and all appear to goes into group B. The second digit, 0, is also even, so agree that it is fair. So if we want to decide which of the second subject goes into group B, the third, 1, is odd two subjects should receive a vaccine, we can toss a giving group A, and so on. We get the allocation shown coin. Heads and the first subject receives the vaccine, in Table 2.4. We could allocate into three groups by as- tails and the second receives it. If we do this for each signing to A if the digit is 1, 2, or 3, to B if 4, 5, or 6, and to pair of subjects, we build up two groups which have C if 7, 8, or 9, ignoring 0. We could allocate in a 2:1 ratio been assembled without any characteristics of the sub- by putting 1 to 6 in A, 7 to 9 in B, and ignoring zeros. jects themselves being involved in the allocation. The There are many possibilities. only differences between the groups will be those due The system described above gave us unequal numbers to chance. As we shall see later (Chapters 8 and 9), stat- in the two groups, 8 in A and 12 in B. We sometimes istical methods enable us to measure the likely effects want the groups to be of equal size. One way to do this of chance. Any difference between the groups which is would be to proceed as above until either A or B has larger than this should be due to the treatment, since 10 subjects in it, all the remaining subjects going into there will be no other differences between the groups. the other groups. This is satisfactory in that each sub- This method of dividing subjects into groups is called ject has an equal chance of being allocated to A or B, random allocation or randomization. but it has a disadvantage. There is a tendency for the last Several methods of randomizing have been in use for few subjects all to have the same treatment. This charac- centuries, including coins, dice, cards, lots, and spinning teristic sometimes worries researchers, who feel that the wheels. Some of the theory of probability which we shall randomization is not quite right. In statistical terms, the use later to compare randomized groups, was first de- possible allocations are not equally likely. If we use this veloped as an aid to gambling. For large randomizations method for the random allocation described above, the we use a different, non-physical randomizing method: 10th subject in group B would be reached at subject 18 random numbers generated by a mathematical process. and the last two subjects would both be in group A. We Table 2.3 provides an example, a table of 1 040 random can ensure that all randomizations are equally likely by digits. These are more properly called pseudo-random using the table of random numbers in a different way. numbers, as they are generated by a mathematical pro- cess. They are available in printed tables (Kendall and Table 2.4 Allocation of 20 subjects to two groups Babington Smith 1971) or can be produced by com- subject digit group subject digit group puter and calculators. Random allocation is now usually done by generating the numbers fresh each time, but the 1 6 B 11 9 A table will be used to illustrate the principle. We can use 2 0 B 12 2 B tables of random numbers in several ways to achieve ran- dom allocation. For example, let us randomly allocate 3 1 A 13 8 B 20 subjects to two groups, which I shall label A and B. 4 5 A 14 6 B We choose a random starting point in the table, using 5 1 A 15 1 A one of the physical methods described previously. (I used decimal dice. These are 20-sided dice, numbered 0 to 9 6 6 B 16 3 A twice, which fit our number system more conveniently 7 0 B 17 3 A than the traditional cube. Two such dice give a random 8 8 B 18 2 B number between 1 and 100, counting ‘0,0’ as 100.) The random starting point was row 7, column 79, and the first 9 9 A 19 2 B twenty digits were 6, 0, 1, 5, 1, 6, 0, 8, 9, 0, 9, 2, 8, 6, 1, 3, 10 0 B 20 6 B 3, 2, 2, and 6. We now allocate subjects corresponding to 2.2 Random allocation 9 For example, we can use the table to draw a random Table 2.5 Condition of patients on admission to trial of sample of 10 subjects from 20, as described in Sec- streptomycin (data from MRC 1948) tion 3.4. These would form group A, and the remaining 10 group B. Another way is to put our subjects into small Group equal-sized groups, called blocks, and within each block S C to allocate equal numbers to A and B. This gives ap- proximately equal numbers on the two treatments and General condition Good 8 8 Fair 17 20 will do so whenever the trial stops. We can also have Poor 30 24 blocks which vary in size, the size of block being chosen randomly. Max. evening temperature 98–98.9 4 4 The use of random numbers and the generation of the in first week (◦ F) 99–99.9 13 12 random numbers themselves are simple mathematical 100–100.9 15 17 operations well suited to the computers which are now 101+ 24 19 readily available to researchers. It is very easy to program Sedimentation rate 0–10 0 0 a computer to carry out random allocation, and once a 11–20 3 2 program is available it can be used over and over again 21–50 16 20 for further experiments. There are several programs avail- 51+ 36 29 able, both free and commercial, which will do random allocations of different types. There is a directory on my website, martinbland.co.uk. advantage to the streptomycin group. The relationship of The trial carried out by the Medical Research Coun- survival to initial condition is shown in Table 2.6. Survival cil (MRC 1948) to test the efficacy of streptomycin for was more likely for patients with lower temperatures, but the treatment of pulmonary tuberculosis is generally con- the difference in survival between the S and C groups is sidered to have been the first randomized experiment in clearly present within each temperature category where medicine. There are other contenders for this crown, but deaths occurred. this is generally regarded as the trial that inspired oth- Randomized trials are not restricted to two treatments. ers to follow in their footsteps. In this study, the target We can compare several treatments. A drug trial might population was patients with acute progressive bilateral include the new drug, a rival drug, and no drug at all. pulmonary tuberculosis, aged 15–30 years. All cases were We can carry out experiments to compare several factors bacteriologically proved and were considered unsuitable at once. For example, we might wish to study the effect for other treatments then available. The trial took place of a drug at different doses in the presence or absence in three centres and allocation was by a series of ran- of a second drug, with the subject standing or supine. dom numbers, drawn up for each sex at each centre. This is usually designed as a factorial experiment, where The streptomycin group contained 55 patients and the every possible combination of treatments is used. These control group 52 cases. The condition of the patients on designs are unusual in clinical research but are some- admission is shown in Table 2.5. The frequency distribu- times used in laboratory work. They are described in tions of temperature and sedimentation rate were similar more advanced texts (e.g. Armitage et al. 2002). For more for the two groups; if anything the treated (S) group were on randomized trials in general, see Pocock (1983) and slightly worse. However, this difference is no greater than Johnson and Johnson (1977). could have arisen by chance, which, of course, is how it Randomized experimentation may be criticised be- arose. The two groups are certain to be slightly different cause we are withholding a potentially beneficial treat- in some characteristics, especially with a fairly small sam- ment from patients. Any biologically active treatment ple, and we can take account of this in the analysis using is potentially harmful, however, and we are surely not multifactorial methods (Chapter 15). justified in giving potentially harmful treatments to pa- After six months, 93% of the S group survived, com- tients before the benefits have been demonstrated pared with 73% of the control group. There was a clear conclusively. Without properly conducted controlled 10 Chapter 2 The design of experiments Table 2.6 Survival at six months in the MRC streptomycin trial, stratified by initial condition (data from MRC 1948) Maximum evening Group temperature during first Outcome Streptomycin Control observation week group group 98–98.9◦ F Alive 3 4 Dead 0 0 99–99.9◦ F Alive 13 11 Dead 0 1 100–100.9◦ F Alive 15 12 Dead 0 5 101◦ F and above Alive 20 11 Dead 4 8 clinical trials to support it, each administration of a treat- sexes. There is no point in using a stratification variable ment to a patient becomes an uncontrolled experiment, which we do not know without great effort. It means whose outcome, good or bad, cannot be predicted. that the recruitment process takes longer and potential participants may be lost. Some statisticians and triallists, myself included, think 2.3 Stratification that stratification is often a waste of time. We need a large sample and a large sample will give similar groups Researchers sometimes worry that the randomization anyway. We should adjust treatment estimates for partici- process will not produce balanced groups. Just by pant characteristics which affect the outcome whether chance, we might have all the men allocated to one the groups are exactly balanced or not (Section 15.3). group and all the women allocated to the other. This is Stratification is usually more to make the researchers feel possible, of course, but extremely unlikely. However, to secure than for any practical benefit. be sure that it does not happen, we can stratify our allocation. We divide the sample to be allocated into separate, mutually exclusive groups, called strata. We 2.4 Methods of allocation then allocate participants to trial groups using a separate blocked allocation within each stratum. This guarantees without random numbers that within each stratum we will have similar numbers of In the second stage of the New York studies of BCG vac- participants on each treatment. There must be enough cine, the children were allocated to treatment or control participants in each stratum for more than one block alternately. Researchers often ask why this method can- if this is to work. This makes stratified allocation suit- not be used instead of randomization, arguing that the able only for large trials. For small trials, we can consider order in which patients arrive is random, so the groups minimization (Section 2.14) instead. thus formed will be comparable. First, although the pa- Age and sex are often used to stratify. Each stratum is tients may appear to be in a random order, there is no then an age group of one sex, e.g. women aged under guarantee that this is the case. We could never be sure 40 years. For stratification, we choose a variable which that the groups are comparable. Second, this method predicts the outcome well and is easy to observe. There is very susceptible to mistakes, or even to cheating in is no point in stratifying by sex if the thing we are trying the patients’ perceived interest. The experimenter knows to influence by our treatment will not differ between the what treatment the subject will receive before the subject 2.4 Methods of allocation without random numbers 11 is admitted to the trial. This knowledge may influence Other methods of allocation set out to be random the decision to admit the subject, and so lead to biased but can fall into this sort of difficulty. For example, we groups. For example, an experimenter might be more could use physical mixing to achieve randomization. This prepared to admit a frail patient if the patient will be on is quite difficult to do. As an experiment, take a deck of the control treatment than if the patient would be ex- cards and order them in suits from ace of clubs to king of posed to the risk of the new treatment. This objection spades. Now shuffle them in the usual way and examine applies to using the last digit of the hospital number for them. You will probably see many runs of several cards allocation. which remain together in order. Cards must be shuffled Knowledge of what treatment the next patient will very thoroughly indeed before the ordering ceases to be receive can certainly lead to bias. For example, Schulz apparent. The physical randomization method can be et al. (1995) looked at 250 controlled trials. They com- applied to an experiment by marking equal numbers on pared trials where treatment allocation was not ad- slips of paper with the names of the treatments, sealing equately concealed from researchers, with trials where them into envelopes and shuffling them. The treatment there was adequate concealment. They found an average for a subject is decided by withdrawing an envelope. treatment effect 41% larger in the trials with inadequate This method was used in another study of anticoagulant concealment. therapy by Carleton et al. (1960). These authors reported There are several examples reported in the literature that in the latter stages of the trial some of the clinicians of alterations to treatment allocations. Holten (1951) re- involved had attempted to read the contents of the enve- ported a trial of anticoagulant therapy for patients with lopes by holding them up to the light, in order to allocate coronary thrombosis. Patients who presented on even patients to their own preferred treatment. dates were to be treated and patients arriving on odd Interfering with the randomization can actually be dates were to form the control group. The author reports built into the allocation procedure, with equally disas- that some of the clinicians involved found it ‘difficult to trous results. In the Lanarkshire Milk Experiment, dis- remember’ the criterion for allocation. Overall the treated cussed by ‘Student’ (1931), 10 000 school children re- patients did better than the controls (Table 2.7). Curi- ceived three-quarters of a pint of milk per day and 10 000 ously, the controls on the even dates (wrongly allocated) children acted as controls. The children were weighed did considerably better than control patients on the odd and measured at the beginning and end of the six-month dates (correctly allocated), and even managed to do mar- experiment. The object was to see whether the milk im- ginally better than those who received the treatment. The proved the growth of children. The allocation to the ‘milk’ best outcome, treated or not, was for those who were in- or control group was done as follows: correctly allocated. Allocation in this trial appears to have The teachers selected the two classes of pupils, been rather selective. those getting milk and those acting as controls, in two different ways. In certain cases they Table 2.7 Outcome of a clinical trial using systematic alloca- selected them by ballot and in others on an tion, with errors in allocation (data from Holten 1951) alphabetical system. In any particular school where there was any group to which these Even dates Odd dates methods had given an undue proportion of well-fed or ill-nourished children, others were Outcome Treated Control Treated Control substituted to obtain a more level selection. Survived 125 39 10 125 The result of this was that the control group had a mark- Died 39 (25%) 11 (22%) 0 (0%) 81 (36%) edly greater average height and weight at the start of the Total 164 50 10 206 experiment than did the milk group. ‘Student’ interpreted this as follows: 12 Chapter 2 The design of experiments Presumably this discrimination in height and first and third grade left unvaccinated as controls. The weight was not made deliberately, but it would argument against this ‘observed control’ approach was seem probable that the teachers, swayed by the that the groups may not be comparable, whereas the very human feeling that the poorer children argument against the randomized control method was needed the milk more than the comparatively that the saline injection could provoke paralysis in in- well to do, must have unconsciously made fected children. The results are shown in Table 2.8. In the too large a substitution for the ill-nourished randomized control areas the vaccinated group clearly among the (milk group) and too few among experienced far less polio than the control group. Since the controls and that this unconscious selection these were randomly allocated, the only difference be- affected secondarily, both measurements. tween them should be the treatment, which is clearly preferable to saline. However, the control group also had Whether the bias was conscious or not, it spoiled the ex- more polio than those who had refused to participate periment, despite being from the best possible motives. in the trial. The difference between the control and not There is one non-random method which can be used inoculated groups is in both treatment (saline injection) successfully in clinical trials: minimization (Section 2.14). and selection; they are self-selected as volunteers and In this method, new subjects are allocated to treatments refusers. The observed control areas enable us to dis- so as to make the treatment groups as similar as possible tinguish between these two factors. The polio rates in in terms of the important prognostic factors. the vaccinated children are very similar in both parts of the study, as are the rates in the not inoculated sec- ond grade children. It is the two control groups which 2.5 Volunteer bias differ. These were selected in different ways: in the ran- People who volunteer for new treatments and those domized control areas they were volunteers, whereas in who refuse them may be very different. An illustration the observed control areas they were everybody eligible, is provided by the field trial of Salk poliomyelitis vaccine both potential volunteers and potential refusers. Now carried out in 1954 in the USA (Meier 1977). This was suppose that the vaccine were saline instead, and that carried out using two different designs simultaneously, the randomized vaccinated children had the same polio due to a dispute about the correct method. In some experience as those receiving saline. We would expect districts, second grade school-children were invited to 200 745 × 57/100 000 = 114 cases, instead of the 33 participate in the trial, and randomly allocated to receive observed. The total number of cases in the randomized vaccine or an inert saline injection. In other districts, all areas would be 114 + 115 + 121 = 350 and the rate per second grade children were offered vaccination and the 100 000 would be 47. This compares very closely with the Table 2.8 Result of the field trial of Salk poliomyelitis vaccine (data from Meier 1977) Study group Number in group Paralytic polio Number of cases Rate per 100 000 Randomized control: Vaccinated 200 745 33 16 Control 201 229 115 57 Not inoculated 338 778 121 36 Observed control: Vaccinated 2nd grade 221 998 38 17 Control 1st and 3rd grade 725 173 330 46 Unvaccinated 2nd grade 123 605 43 35 2.7 Cross-over designs 13 rate of 46 in the observed control first and third grade both vaccinated and refused, with the control group. group. Thus it seems that the principal difference be- The rate in the second grade children is 23 per 100 000, tween the saline control group of volunteers and the not which is less than the rate of 46 in the control group, inoculated group of refusers is selection, not treatment. demonstrating the effectiveness of the vaccine. The ‘treat- There is a simple explanation of this. Polio is a viral ment’ which we are evaluating is not vaccination itself, disease transmitted by the faecal–oral route. Before the but a policy of offering vaccination and treating those development of vaccine almost everyone in the popula- who accept. A similar problem can arise in a random- tion was exposed to it at some time, usually in childhood. ized trial, for example in evaluating the effectiveness of In the majority of cases, paralysis does not result and health check-ups (South-east London Screening Study immunity is conferred without the child being aware of Group 1977). Subjects were randomized to a screening having been exposed to polio. In a small minority of group or to a control group. The screening group were cases, about one in 200, paralysis or death occurs and invited to attend for an examination, some accepted and a diagnosis of polio is made. The older the exposed indi- were screened and some refused. When comparing the vidual is, the greater the chance of paralysis developing. results in terms of subsequent mortality, it was essential Hence, children who are protected from infection by to compare the controls to the screening groups contain- high standards of hygiene are likely to be older when ing both screened and refusers. For example, the refusers they are first exposed to polio than those children from may have included people who were already too ill to homes with low standards of hygiene, and thus more come for screening. The important point is that the ran- likely to develop the clinical disease. There are many fac- dom allocation procedure produces comparable groups tors which may influence parents in their decision as to and it is these we must compare, whatever selection may whether to volunteer or refuse their child for a vaccine be made within them. We therefore analyse the data ac- trial. These may include education, personal experience, cording to the way we intended to treat subjects, not the current illness, and others, but certainly include inter- way in which they were actually treated. This is analysis by est in health and hygiene. Thus, in this trial, the high intention to treat. The alternative, analysing by treat- risk children tended to be volunteered and the low risk ment actually received, is called on treatment or per children tended to be refused. The higher risk volun- protocol analysis. teer control children experienced 57 cases of polio per Analysis by intention to treat is not free of bias. As 100 000, compared with 36/100 000 among the lower some patients may receive the other group’s treatment, risk refusers. the difference may be smaller than it should be. We know In most diseases, the effect of volunteer bias is op- that there is a bias and we know that it will make the posite to this. Poor conditions are related both to refusal treatment difference smaller, by an unknown amount. to participate and to high risk, whereas volunteers tend On treatment analyses, on the other hand, are biased in to be low risk. The effect of volunteer bias is then to favour of showing a difference, whether there is one or produce an apparent difference in favour of the treat- not. Statisticians call methods which are biased against ment. We can see that comparisons between volunteers finding any effect conservative. If we must err, we like and other groups can never be reliable indicators of to do so in the conservative direction. treatment effects. 2.7 Cross-over designs 2.6 Intention to treat Sometimes it is possible to use a trial participant as In the observed control areas of the Salk trial (Table 2.8), her or his own control. For example, when compar- quite apart from the non-random age difference, the ing analgesics in the treatment of arthritis, participants vaccinated and control groups are not comparable. may receive in succession a new drug and a control However, it is possible to make a reasonable comparison treatment. The response to the two treatments can then in this study by comparing all second grade children, be compared for each participant. These designs have 14 Chapter 2 The design of experiments the advantage of removing variability between partici- on the control treatment. These periods were in random pants. We can carry out a trial with fewer participants order. The outcome measure was the number of attacks than would be needed for a two group trial. of angina experienced. These were recorded by the pa- Although all subjects receive all treatments, these tient in a diary. Twelve patients took part in the trial. The trials must still be randomized. In the simplest case results are shown in Table 2.9. The advantage in favour of of treatment and control, patients may be given two pronethalol is shown by 11 of the 12 patients reporting different regimes: control followed by treatment or fewer attacks of pain while on pronethalol than while on treatment followed by control. These may not give the the control treatment. If we had obtained the same data same results, e.g. there may be a long-term carry-over from two separate groups of patients instead of the same effect or time trend which makes treatment followed by group under two conditions, it would be far from clear control show less of a difference than control followed that pronethalol is superior because of the huge variation by treatment. Subjects are, therefore, assigned to a between subjects. Using a two group design, we would given order at random. It is possible in the analysi