Psych 102: Intro to Methods (1) NOBA Reading A PDF
Document Details
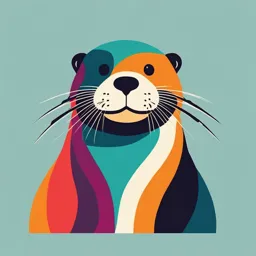
Uploaded by EliteViolet6744
UBC
Tags
Summary
This document appears to be part of a course textbook about introductory psychology, focusing on research methods. It details key concepts such as systematic observation, ethical considerations in research, and how psychological science has developed.
Full Transcript
PSYCH 102: Intro and Methods (1) NOBA READING A Ch.1 Why Science? - Terminology Term Definition Empirical Methods Approaches to inquiry that are tied to actual measurement and observation. Ethics Professional...
PSYCH 102: Intro and Methods (1) NOBA READING A Ch.1 Why Science? - Terminology Term Definition Empirical Methods Approaches to inquiry that are tied to actual measurement and observation. Ethics Professional guidelines offer researchers a template for making decisions that protect research participants from potential harm and that help steer scientists away from conflicts of interest or other situations that might compromise the integrity of their research. Hypotheses A logical idea that can be tested. Systematic Observation The careful observation of the natural world with the aim of better understanding it. Observations provide the basic data that allow scientists to track, tally, or otherwise organize information about the natural world. Theories Groups of closely related phenomena or observations. Learning Objectives Describe how scientific research has changed the world. Identify the key characteristics of the scientific approach. Discuss benefits and problems created by science. Explain how psychological science has improved the world. Outline ethical guidelines psychologists follow. Essential Elements of Science 1. Systematic Observation Science relies on organized, controlled observations and measurements to minimize bias. Varying conditions systematically helps identify when phenomena occur and do not occur. 2. Testable Hypotheses Observations lead to hypotheses and theories that can be tested. Example: Comparing burn speeds of paraffin and beeswax candles. 3. Democratic Nature Science encourages debate and skepticism, with conclusions based on evidence rather than authority. Competing findings help the best data emerge. 4. Cumulative Knowledge Scientific progress builds on prior discoveries, advancing knowledge over time. Example: Physicists build on Newton’s work to achieve modern understanding. Psychology as a Science Skepticism about psychology as a science often arises because thoughts and feelings are invisible, unlike physical phenomena. Early psychologists focused on observable behaviors, but modern methods allow measurement of internal experiences. Francis Galton’s Contributions Invented the self-report questionnaire to assess individual differences. Studied genetic and environmental contributions to personality using twin studies, addressing the “nature vs. nurture” question. Advancements in Psychological Research Psychology’s Growth: At just 150 years old, psychology is a young science. Improved methods, study designs, and statistical tools now allow for sophisticated research. → Example – Measuring Happiness Self-Reports: Participants rate happiness on scales (limited by dishonesty or inconsistent use). Peer Reports: Friends and family provide ratings to cross-check self-reports. Memory Measures: Positive individuals recall pleasant events more easily, while negative individuals recall unpleasant events. Biological Measures: Techniques like cortisol sampling (stress hormone) and fMRI scans (brain activity linked to good moods). Psychological Science in Practice Therapies for Disorders: Cognitive Behavioral Therapy (CBT) is effective for depression and anxiety Some therapies, however, may be harmful (e.g., Lilienfeld, 2007). Organizational Psychology: Alphonse Chapanis redesigned aircraft cockpits, reducing pilot errors and crashes. Forensic Sciences: Elizabeth Loftus’ research revealed the unreliability of eyewitness testimony, impacting courtroom decisions. Ethics in Psychological Research → Psychologists follow strict ethical guidelines to protect participants. 1. Informed Consent: Participants must understand the study and voluntarily agree to take part. 2. Confidentiality: Personal information must remain private without participant consent. 3. Privacy: No observations in private spaces or collection of confidential information without consent. 4. Benefits vs. Risks: Risks must be outweighed by benefits and clearly explained to participants. 5. Deception: Deception may be used when necessary but must be followed by debriefing to explain the study’s true purpose. Ch.2 Research Designs - Terminology Term Definition Confounds Factors that undermine the ability to draw causal inferences from an experiment. Correlation Measures the association between two variables, or how they go together. Independent Variable The variable the researcher manipulates and controls in an experiment. Dependent Variable The variable the researcher measures but does not manipulate in an experiment. Experimenter Expectations When the experimenter’s expectations influence the outcome of a study. Longitudinal Study A study that follows the same group of individuals over time. Operational Definitions How researchers specifically measure a concept. Participant Demand When participants behave in a way that they think the experimenter wants them to behave. Placebo Effect When receiving special treatment or something new, it affects human behavior. Quasi-Experimental Design An experiment that does not require random assignment to conditions. Random Assignment Assigning participants to receive different conditions of an experiment by chance. Learning Objectives Articulate the difference between correlational and experimental designs. Understand how to interpret correlations. Understand how experiments help us to infer causality. Understand how surveys relate to correlational and experimental research. Explain what a longitudinal study is. List a strength and weakness of different research designs. Experimental Research Example: Elizabeth Dunn (2008) conducted an experiment at UBC to study the relationship between spending and happiness. Participants received $20 to spend by the end of the day. ○ Group 1: Spend on themselves. ○ Group 2: Spend on others (e.g., charity or gifts). Happiness levels were measured using a self-report questionnaire. Result: Participants who spent money on others reported higher happiness levels than those who spent on themselves. Experiment Design Concepts 1. Independent Variable (IV): ○ The variable manipulated by the researcher. ○ In this case: whether money was spent on oneself or others. 2. Dependent Variable (DV): ○ The variable measured or observed. ○ In this case: participants’ happiness. ○ The DV "depends" on the changes in the IV. 3. Random Assignment: ○ Participants are randomly assigned to conditions (e.g., self-spending vs. other-spending). ○ Ensures groups are similar on all other characteristics (e.g., childhood happiness, daily mood). ○ Reduces biases and external factors. 4. Cause and Effect: ○ If the only difference between groups is the IV, any change in the DV can be attributed to the IV. Importance of Random Assignment: Helps distribute characteristics evenly across groups (e.g., mood, past experiences). Example: Forming basketball teams. Random assignment creates balanced teams by distributing tall and short players equally. Avoiding Confounds: 1. Confounds: Factors that undermine the ability to establish causality. Example: Placebo effect—participants may feel happier just because they expect to feel better. 2. Participant Demand: Participants behave in ways they think the researcher wants. 3. Experimenter Expectations: Experimenter may unintentionally perceive results that align with their expectations. Double-Blind Procedure Definition: Both participants and researchers are unaware of group assignments (e.g., happy pill vs. placebo). Reduces biases from participant demand and researcher expectations. Ensures differences between groups are due to the IV. Key Takeaways for Experimental Research Experiments rely on manipulating IVs and observing changes in DVs. Random assignment and double-blind procedures strengthen the reliability of conclusions. Avoiding confounds is crucial to establishing causality. Correlational Designs 1. Definition: Correlational research involves passive observation and measurement of phenomena. Scientists do not intervene or change behavior as they do in experiments. The focus is on identifying patterns of relationships. 2. Causation: Correlational research cannot establish causality (i.e., what causes what). 3. Variables: Examines the relationship between exactly two variables at a time. Example (Professor Dunn): Hypothesis: Spending on others is related to happiness. Method: Asked participants how much of their income they spent on others or donated to charity and then assessed their happiness levels. Finding: The more money people spend on others, the happier they reported being. Correlations Understanding Correlations 1. Scatterplots: ○ Visual representation of the relationship between two variables. ○ Each dot represents a data point with two values (e.g., X-axis and Y-axis). ○ The pattern of dots indicates the direction and strength of the relationship. 2. Correlation Coefficient (r): ○ A statistical measure summarizing the direction and strength of a relationship. ○ Ranges from -1.0 to +1.0: Positive r: As one variable increases, the other also increases. Negative r: As one variable increases, the other decreases. Zero r: No relationship between the variables. Types of Correlations 1. Positive Correlation: ○ Variables move in the same direction (e.g., the better the month, the happier a person feels). ○ Scatterplot: Dots form a pattern extending from bottom-left to top-right. ○ Example: r = +0.81 (strong positive correlation). 2. Negative Correlation: ○ Variables move in opposite directions (e.g., higher pathogen prevalence relates to shorter average male height). ○ Scatterplot: Dots form a pattern extending from top-left to bottom-right. ○ Example: r = -0.83 (strong negative correlation). 3. Weak Correlation: ○ Variables have a weak association; many exceptions exist. ○ Scatterplot: Dots are loosely scattered without a clear pattern. ○ Example: Valuing happiness and GPA (low absolute r value). 4. Perfect Correlation: ○ No exceptions in the pattern; absolute r value is 1.0. ○ Example: Age and year of birth have a strong negative perfect correlation. Strength of Correlations Strong Correlation: ○ Tight clusters of dots along a sloped line. ○ The absolute value of R is close to 1 (e.g., r=±0.81r = \pm 0.81r=±0.81). ○ Example: Height and pathogen prevalence (r=−0.83r = -0.83r=−0.83). Weak Correlation: ○ Dots are more dispersed; R has a low absolute value. ○ Example: Valuing happiness and GPA. Uncorrelated Variables: ○ No meaningful relationship; R is near zero. Key Insights 1. Direction: ○ Positive or negative depends on whether the variables move together or in opposite directions. 2. Strength: ○ Determined by the absolute value of R: higher absolute values = stronger relationships. 3. Exceptions: ○ More exceptions = weaker correlation. ○ Example: Generous people being unhappy or stingy people being happy are exceptions to the happiness-spending correlation. 4. Real-World Application: ○ Helps predict patterns but does not establish causation. For example, spending on others is correlated with happiness, but we cannot claim spending directly causes happiness. Problems with Correlation Key Limitation: Correlation ≠ Causation 1. Directionality Problem: ○ Correlation cannot determine which variable influences the other. ○ Example: Does generosity cause happiness, or does happiness cause generosity? Does pathogen prevalence cause short stature, or does short stature affect pathogen prevalence? 2. Third Variable Problem: ○ A third factor might influence both variables, creating an illusion of a direct link. ○ Example: In the generosity-happiness correlation, wealth could be the third variable. Wealthier individuals may: Have more resources to be generous. Experience higher levels of happiness due to financial security. For the pathogen prevalence-height correlation, nutrition or healthcare access could be the third variable affecting both. 3. Spurious Correlations: ○ Correlations might occur by chance or due to unrelated variables. ○ Without further investigation, conclusions based on correlation alone can be misleading. Qualitative Designs in Research Qualitative research designs enable the study of topics that are challenging to manipulate or quantify. These methodologies focus on exploring deeper insights, experiences, and perspectives. Three common qualitative designs are: 1. Participant Observation Definition: Involves the researcher immersing themselves in a group to observe its behaviors, dynamics, and culture. Example: Festinger, Riecken, and Schacter (1956) infiltrated a cult by pretending to be members. This allowed them to study the cult's behavior and psychology from within. Key Features: ○ Observing participants in their natural environment. ○ Participants typically know the researcher is observing, though some cases involve disguised observation. Strengths: ○ Provides in-depth, contextual insights. ○ Captures group dynamics in real time. Limitations: ○ Observer bias may influence findings. ○ Ethical concerns arise if participants are unaware of the researcher's intentions. 2. Case Study Definition: Involves an intensive, detailed examination of a single individual, group, or specific context. Example: Researchers studying the effects of rare brain injuries on happiness might focus on one person due to the limited number of cases. Key Features: ○ Focuses on unique or rare phenomena. ○ Uses extensive tests, interviews, or observations. Strengths: ○ Ideal for studying rare or complex issues. ○ Provides rich, detailed data. Limitations: ○ Findings may not generalize to others. ○ Unique characteristics of the individual or context may skew results. 3. Narrative Analysis Definition: Centers on analyzing stories and personal accounts to understand individuals, groups, or cultures. Key Features: ○ Explores themes, structure, and dialogue within narratives. ○ Data sources can include written, audio-recorded, or video-recorded stories. Example: Analyzing personal testimonies to understand how individuals cope with trauma. Strengths: ○ Captures participants' unique perspectives and emotional depth. ○ Provides insight into both what is said and how it is conveyed. Limitations: ○ Interpretation may be subjective. ○ Requires significant time and effort to analyze. Quasi-Experimental Designs Quasi-experimental designs allow researchers to study variables when random assignment is not possible. These designs are similar to experimental research but lack the key element of random assignment, making causal inference more challenging. Key Features No Random Assignment: Participants are assigned to groups based on preexisting characteristics (e.g., married vs. single, professor preference). Independent Variables: Existing group memberships are treated as the independent variable, but the researcher does not manipulate these conditions. Causal Inference Challenges: Differences between groups may be influenced by preexisting factors, not the independent variable. Examples of Quasi-Experimental Designs 1. Effects of Marriage on Happiness: ○ Research Question: Does marriage make people happier? ○ Design: Compare happiness levels between married and single individuals. ○ Challenge: Married participants may already differ from single participants (e.g., being happier or having more social support before marriage), making it difficult to conclude that marriage causes happiness. 2. Professor Effectiveness: ○ Research Question: Who is a better professor, Dr. Smith or Dr. Khan? ○ Design: Compare students’ final grades in their classes. ○ Challenge: Students self-selecting into classes introduces a confounding variable, such as motivation or intelligence, which affects grades independently of teaching quality. Limitations of Quasi-Experimental Designs Confounding Variables: Differences between groups may result from factors other than the independent variable. Weaker Causal Claims: While associations can be identified, establishing causation is less reliable compared to experimental designs. Longitudinal Studies A longitudinal study is a research design in which the same individuals are tracked and studied over a period of time. These studies are particularly valuable for observing changes, trends, and developmental patterns within individuals or groups. Key Features Tracking Over Time: Participants are studied at multiple points, ranging from weeks to decades. Same Participants: The same individuals or groups are repeatedly measured, providing insight into changes within subjects rather than between different groups. Wide Applications: Useful for testing theories in psychology and other fields, particularly when studying development, behavior, or long-term effects. Example — Study of Happiness and Marriage: Research: Psychologist Rich Lucas (2003) conducted a longitudinal study that followed more than 20,000 Germans over two decades. Findings: People who eventually got married were generally happier than their peers who never married, even before marriage. This demonstrates how longitudinal studies can provide nuanced insights that cross-sectional studies cannot. Advantages of Longitudinal Studies 1. Identifies Patterns of Change: Tracks development, behavioral trends, or outcomes over time. ○ Example: Studying how personality traits evolve with age. 2. Minimizes Individual Differences: By studying the same people, researchers control for variability between individuals. 3. Causal Inference: While not as definitive as experimental designs, longitudinal data can suggest causation more strongly than correlational or cross-sectional studies. ○ Example: Observing how early childhood experiences predict adult mental health. 4. Rich Data: Provides extensive and detailed data on long-term processes. Challenges and Limitations 1. Cost and Time: These studies can be expensive and time-consuming, especially when conducted over decades. 2. Attrition: Participants dropping out over time can skew results and reduce the representativeness of the sample. 3. Cohort Effects: Findings may be influenced by unique characteristics of the cohort being studied, limiting generalizability to other populations. Surveys in Research Key Features Data Collection: Surveys use structured questions to collect self-reported data on attitudes, behaviors, or characteristics. Scalability: Surveys allow researchers to reach a large number of participants quickly. Versatility: Although commonly associated with correlational research, surveys can also be used in experimental designs. Example of an Experimental Survey Study by King and Napa (1998): ○ Research Question: Do people perceive happy individuals as more likely to get into heaven compared to unhappy individuals? ○ Method: Participants were shown surveys completed by a "happy person" or an "unhappy person" and asked to judge their likelihood of getting into heaven. ○ Variables: Independent Variable: The perceived happiness of the individual (happy vs. unhappy person). Dependent Variable: Judgments about the likelihood of getting into heaven. ○ Findings: Participants judged happy people as more likely to get into heaven. Advantages of Surveys 1. Efficient Data Collection: Surveys can gather information from hundreds or thousands of participants in a short time. 2. Cost-Effectiveness: Compared to laboratory experiments, surveys are significantly less expensive to administer. 3. Flexibility: Surveys can be adapted for various research designs, including experiments, correlational studies, and descriptive research. 4. Anonymity: Online surveys, in particular, allow participants to respond honestly without fear of judgment. Challenges and Limitations 1. Self-Report Bias: Participants may provide socially desirable answers instead of honest ones. 2. Question Design: Poorly phrased or leading questions can influence responses and compromise data quality. 3. Non-Response Bias: Certain groups may be less likely to respond, resulting in an unrepresentative sample. 4. Superficial Insights: Surveys often provide surface-level information and lack the depth of qualitative research methods. Ch.3 Statistical Thinking - Terminology Term Definition Cause-and-effect Related to whether we say one variable is causing changes in the other variable, versus other variables that may be related to these two variables. Confidence interval An interval of plausible values for a population parameter; the interval of values within the margin of error of a statistic. Distribution The pattern of variation in data. Generalizability Related to whether the results from the sample can be generalized to a larger population. Margin of error The expected amount of random variation in a statistic; is often defined for a 95% confidence level. Parameter A numerical result summarizing a population (e.g., mean, proportion). Population A larger collection of individuals that we would like to generalize our results to. P-value The probability of observing a particular outcome in a sample, or more extreme, under a conjecture about the larger population or process. Random assignment Using a probability-based method to divide a sample into treatment groups. Random sampling Using a probability-based method to select a subset of individuals for the sample from the population. Sample The collection of individuals on which we collect data. Statistic A numerical result computed from a sample (e.g., mean, proportion). Statistical significance A result is statistically significant if it is unlikely to arise by chance alone. Learning Objectives Define the basic elements of a statistical investigation. Describe the role of p-values and confidence intervals in statistical inference. Describe the role of random sampling in generalizing conclusions from a sample to a population. Describe the role of random assignment in drawing cause-and-effect conclusions. Critique statistical studies. Basic Elements of Statistical Investigation 1. Planning the Study: ○ Define a testable question and determine data collection methods (e.g., duration, participant demographics, and other variables like smoking habits). 2. Examining the Data: ○ Analyze data using graphs and descriptive statistics to identify patterns and deviations (e.g., comparing smokers and non-smokers). 3. Inferring from the Data: ○ Use statistical methods (e.g., p-values, confidence intervals) to determine if results are significant and not due to chance. 4. Drawing Conclusions: ○ Draw conclusions about the findings, considering who they apply to and whether a cause-and-effect relationship can be claimed (e.g., does coffee drinking cause lower mortality?). Distributional Thinking Data Variation: Data vary, and understanding this variation is key to statistical analysis. Research Example: A study compared the reading ability of 63 cancer patients to the readability of 30 cancer pamphlets. Both were measured in grade levels. Key Insights: 1. Data vary: Reading levels of both patients and pamphlets differ. 2. Distribution: Analyzing the full distribution, not just measures like medians, reveals deeper insights. Conclusion: A graph showed 27% of patients have a reading level below the pamphlet's readability, highlighting the need for patient assistance. This insight comes from analyzing the entire distribution. Statistical Significance Uncertainty in Data: Even when patterns appear in data, there may be uncertainty due to measurement errors, limited data, or small sample sizes. To determine if observed patterns are genuine or just due to chance, statistical methods are applied. Example (Hamlin, Wynn, & Bloom, 2007): ○ Study Goal: Investigate if infants prefer a "helper" toy over a "hinderer" toy after observing a character being helped or hindered. ○ Results: Of the 16 infants who made a clear choice, 14 chose the helper toy. ○ Potential Other Factors: Could other factors, like toy color or handedness, affect the choice? The researchers controlled for these variables. Randomness: Despite controlling for variables, there's still randomness in the data. Could the 14 out of 16 choices just be due to random chance? Probability Model: ○ Assumption: If infants have no preference, each has a 50% chance of choosing either toy. ○ p-value Calculation: The chance of 14 or more infants choosing the helper toy by random chance is 0.0021 (p-value). Conclusion: ○ A p-value of 0.0021 is very small, meaning the result is unlikely to be due to chance. ○ Since the p-value is smaller than the typical significance level of 0.05, the researchers concluded that there is strong evidence that the infants have a genuine preference for the helper toy. p-value: A measure of how often a result as extreme as the one observed would occur if random chance were the only factor. If the p-value is below the significance level (usually 0.05), we reject the hypothesis that the result is due to chance. Generalizability Limitation: Conclusions from small studies, like the 16 infants in Example 2, may not be generalizable to larger populations, as we don’t know how those infants were selected. Challenge: How can conclusions from a sample be generalized to a larger population? Pollsters face this question daily. → Margin of Error What It Is: The margin of error is the range within which the true population value is likely to fall. It accounts for the variability that comes with selecting a sample rather than surveying the entire population. How It Works: In surveys, random sampling introduces variability in the results. The margin of error helps estimate how much the sample result could differ from the true population value just due to random chance. For example, if 83.6% of respondents say they feel rushed, the margin of error might be 3%, meaning the true percentage in the population could be between 80.6% and 86.6%. Why We Use It: Since a sample is just a subset of the population, we can't be 100% sure that the sample's results reflect the true population values. The margin of error provides a range that helps quantify uncertainty and shows where the true population value is likely to fall, given the sample data. Non-Random Sampling: Non-random methods often introduce bias, over- or under-representing certain groups within the population. The margin of error does not account for such biases. Other Biases: Issues like dishonest responses or non-responses can also affect survey results, but these sources of error are not captured by the margin of error. Cause and Effect Conclusions The Key Question: In research, the main question is often whether differences between groups are caused by a specific factor or whether they are due to the way the groups were formed. Group Formation: If groups are formed based on certain characteristics (e.g., people who drink coffee vs. those who don’t), there’s a possibility that the differences observed between them might be due to those characteristics rather than the variable being studied. Random Assignment: In experimental studies, researchers aim to assign participants to groups in a way that minimizes bias. Random assignment is crucial because it balances out other variables that could influence the results. For example, if the groups were formed without random assignment, we might not know if any differences in creativity scores between groups were due to the motivation type or some other factor (like gender or age). Random assignment helps to control for such variables. Random Assignment Process: Even though random assignment minimizes bias, there’s still a chance that by "luck of the draw," the groups might be slightly different. In this case, we need to ask: could the observed differences be due to random chance? This is where statistical models come into play to test whether the observed effect is large enough to be meaningful or if it’s likely to have occurred by random chance. Statistical Significance (p-value): In the study example, researchers simulate many random assignments to see how often they would get a difference as large as the one observed. When only 2 out of 1,000 simulated random assignments produced a difference as large or larger, the small p-value (0.002) suggests that the observed difference is highly unlikely to have happened just by chance. Cause and Effect Conclusion: Since random assignment controls for other variables and the p-value is small, researchers can reasonably conclude that the type of motivation (intrinsic vs. extrinsic) likely caused the difference in creativity scores. This gives us stronger evidence for a cause-and-effect relationship between motivation and creativity. Generalizability: Although the conclusion about motivation and creativity is supported by strong evidence, the study only involved people with extensive experience in creative writing. Therefore, while the findings might apply to similar individuals (i.e., those with similar creative writing experience), we can't automatically generalize these results to everyone. Further studies would be needed to explore how the findings might apply to different groups of people. Ch.4 Thinking like a Psychological Scientist - Terminology Term Definition Anecdotal evidence A piece of biased evidence, usually drawn from personal experience, is used to support a conclusion that may or may not be correct. Causality In research, the determination that one variable causes—is responsible for—an effect. Correlation In statistics, the measure of relatedness of two or more variables. Data/ observations In research, information is systematically collected for analysis and interpretation. Deductive reasoning A form of reasoning in which a given premise determines the interpretation of specific observations (e.g., All birds have feathers; since a duck is a bird, it has feathers). Distribution In statistics, the relative frequency that a particular value occurs for each possible value of a given variable. Empirical Concerned with observation and/or the ability to verify a claim. Fact Objective information about the world. Falsify In science, the ability of a claim to be tested and—possibly—refuted; is a defining feature of science. Generalize In research, the degree to which one can extend conclusions drawn from the findings of a study to other groups or situations not included in the study. Hypothesis A tentative explanation that is subject to testing. Induction To draw general conclusions from specific observations. Inductive reasoning A form of reasoning in which a general conclusion is inferred from a set of observations (e.g., noting that “the driver in that car was texting; he just cut me off then ran a red light!” (a specific observation), which leads to the general conclusion that texting while driving is dangerous). Levels of analysis In science, there are complementary understandings and explanations of phenomena. Null-hypothesis significance In statistics, a test is created to determine the chances that an alternative testing (NHST) hypothesis would produce a result as extreme as the one observed if the null hypothesis were actually true. Objective Being free of personal bias. Population In research, all the people belonging to a particular group (e.g., the population of left handed people). Probability A measure of the degree of certainty of the occurrence of an event. Probability values In statistics, the established threshold for determining whether a given value occurs by chance. Pseudoscience Beliefs or practices that are presented as being scientific, or which are mistaken for being scientific, but which are not scientific (e.g., astrology, the use of celestial bodies to make predictions about human behaviors, and which presents itself as founded in astronomy, the actual scientific study of celestial objects. Astrology is a pseudoscience unable to be falsified, whereas astronomy is a legitimate scientific discipline). Representative In research, the degree to which a sample is a typical example of the population from which it is drawn. Sample In research, a number of people selected from a population to serve as an example of that population. Scientific theory An explanation for observed phenomena that is empirically well-supported, consistent, and fruitful (predictive). Type I error In statistics, the error of rejecting the null hypothesis when it is true. Type II error In statistics, the error of failing to reject the null hypothesis when it is false. Value Belief about the way things should be. Learning Objectives Compare and contrast conclusions based on scientific and everyday inductive reasoning. Understand why scientific conclusions and theories are trustworthy, even if they are not able to be proven. Articulate what it means to think like a psychological scientist, considering the qualities of good scientific explanations and theories. Discuss science as a social activity, comparing and contrasting facts and values. Scientific vs. Everyday Reasoning 1. Everyday Reasoning Definition: Conclusions or statements based on personal experiences or observations. Example: ○ "It looks like rain today" (based on one's observations of the sky). ○ "Dogs are very loyal" (based on personal experience with dogs). Induction: Everyday reasoning often involves drawing conclusions from a limited sample of observations or personal experiences. Certainty: Everyday statements tend to be more certain (e.g., "Dogs are loyal"). Limitations: May not be supported by systematic evidence or broad data, and often lacks testing of alternative explanations. 2. Scientific Reasoning Definition: Systematic, evidence-based reasoning used to draw conclusions about the world. Example: ○ "There is an 80% chance of rain today" (based on meteorological data). ○ "Dogs tend to protect their human companions" (based on research). Induction: Scientists also use induction, but they draw conclusions from a broader range of data and systematically test hypotheses. Certainty: Scientific statements are often presented with less certainty (e.g., using probabilities like "80% chance"). Falsifiability: A core feature of scientific reasoning—claims must be testable and potentially falsifiable. 3. Key Differences Between Scientific and Everyday Reasoning Induction: ○ Everyday Reasoning: Draws conclusions from limited personal observations (e.g., passing an exam after cramming). ○ Scientific Reasoning: Draws conclusions from broader, controlled samples of data collected through systematic methods. Certainty: ○ Everyday Reasoning: Often more certain, with statements like “dogs are loyal” based on personal experience. ○ Scientific Reasoning: Less certain and often presented with probabilities (e.g., "80% chance of rain"). Falsifiability (Karl Popper’s Concept): ○ Definition: A claim must be testable and have the potential to be proven false. ○ Example: Scientific: "All people are right-handed" (can be falsified by showing left-handed individuals). Unscientific: "A magician can teach people to move objects with their minds" (unfalsifiable claim, can't be disproven). Testable Hypotheses: ○ Scientific Reasoning: Generates hypotheses that are clear, testable, and falsifiable. The scientist tests various possible explanations and aims to falsify the incorrect ones. ○ Everyday Reasoning: Often involves conclusions drawn from personal experiences that cannot be rigorously tested. 4. Popper’s Critique of Non-Scientific Claims Example: Freud's theories on mental illness. ○ Freud suggested that mental illnesses could be explained by childhood experiences (e.g., obsessive perfectionism stemming from messy or orderly parents). ○ His theories are not falsifiable because they can explain any situation, making it impossible to test or disprove them. Popper’s Argument: Theories that cannot be disproven hinder scientific progress because they block further investigation and refinement. 5. The Role of Falsification in Science Testing All Possible Explanations: ○ Scientists aim to test all potential explanations for a phenomenon, ruling out those that are incorrect. ○ Example: Studying car accidents involves testing multiple factors (e.g., alcohol consumption, speeding, using a mobile phone). ○ Only after testing various hypotheses can the true causes be identified. Falsifiability: A key principle that allows scientific knowledge to progress by eliminating false claims. 6. The Evolving Role of Falsifiability in Modern Science Beyond Falsification: ○ While falsifiability remains a cornerstone of scientific reasoning, modern scientists are also interested in describing and explaining phenomena. ○ For example, research might explore when young children begin speaking in full sentences or how exercise affects depression. Interpretation and Probability: Data are not always clear-cut and may require probabilistic reasoning, where conclusions are drawn from limited data samples, and interpretations are made based on the probability of the outcomes. Why We Can Never "Prove" Anything 1. Proof in Science vs. Certainty: Science does not prove anything. It offers evidence for or against hypotheses. Inductive reasoning is used to draw conclusions based on observations. It doesn’t prove but suggests likelihoods. Even large, replicated studies cannot guarantee the same outcome every time, leaving room for uncertainty and future findings. 2. Inductive Reasoning: In the caffeine-memory study, the researcher uses inductive reasoning: ○ Previous studies show a possible relationship between caffeine and memory. ○ Based on these findings, she forms a hypothesis: Caffeine enhances memory. Inductive reasoning draws general conclusions from specific examples (e.g., caffeine improving memory in some studies). It never proves something definitively, only suggests the probability of something being true. 3. Deductive Reasoning: Deductive reasoning starts with a general principle and applies it to specific situations. Example: "All living cells contain DNA, so any cell will contain DNA." In the caffeine study, deductive reasoning isn’t as useful because the hypothesis involves complex, variable factors (e.g., individual differences in caffeine tolerance). 4. The Role of Probabilities: Science deals with probabilities, not certainties. The caffeine study could show that caffeine improves memory in many cases, but there will always be exceptions or new data that could contradict this. Research findings suggest likelihoods of an outcome but never guarantee it. Probabilities express the strength of evidence, but not absolute truth. 5. Anecdotal Evidence: Anecdotal evidence refers to personal experiences or casual observations, which are unreliable in scientific research. Example: Someone claims caffeine always helps their memory based on personal experience—this is not scientifically valid. Anecdotal evidence is prone to bias, such as selective memory (remembering times caffeine worked) and not accounting for other influencing factors. 6. Scientific Research vs. Anecdotal Evidence: In the caffeine-memory study, a well-designed experiment uses control, randomization, and systematic observation to gather data. In contrast, anecdotal evidence lacks these controls and is often subjective, making it less reliable than scientific research. 7. Key Takeaways: Science cannot prove anything; it can only suggest that something is likely true. Inductive reasoning is about making educated guesses based on evidence, but these are always subject to change with new data. Deductive reasoning applies to more certain, structured fields and is not as effective in psychology or biology, where multiple variables can influence outcomes. Probabilities in science help estimate the likelihood of results but cannot provide certainty. Anecdotal evidence is not valid in scientific contexts because it lacks systematic observation and may be influenced by bias. Why We Should Trust Science Despite It Not "Proving" Anything 1. Trusting Science Despite No Absolute Proof: Science doesn’t offer absolute proof. Instead, it focuses on probabilities and likelihoods. NHST is a way to assess the probability that observed data would occur if there were no relationships between the variables being studied. This doesn’t prove that a relationship exists, but it provides evidence for or against it. 2. Null-Hypothesis Significance Testing (NHST): NHST compares two hypotheses: ○ The null hypothesis (H₀) states there is no relationship between two variables (e.g., maturity and academic performance). ○ The alternative hypothesis (H₁) states that there is a relationship. The goal is to determine whether the observed data can reject the null hypothesis in favor of the alternative hypothesis. 3. The Process of NHST: Step 1: The researcher collects data (e.g., student age and academic performance). Step 2: The data is analyzed to see if the observed relationship (e.g., older students performing better) is statistically significant. Step 3: Probability is used to assess whether the data obtained is likely to happen if the null hypothesis is true. 4. What Are the Possible Outcomes of NHST? There are four possible outcomes based on reality and what the researcher finds: 1. Accurate Detection: The researcher finds the correct relationship (either proving the hypothesis or not). 2. Type I Error: The researcher incorrectly rejects the null hypothesis, concluding a relationship exists when there is actually none. This can happen due to random chance in the sample. 3. Type II Error: The researcher fails to reject the null hypothesis, missing a real relationship between variables due to sample peculiarities. 4. True Negative: The researcher correctly concludes that there is no relationship, and none exists. 5. Type I Error (False Positive): This occurs when the researcher mistakenly concludes that there is a relationship between two variables, even when there is none. Example: The researcher might find that older students seem to perform better, but this could be due to better study habits, not maturity. 6. Type II Error (False Negative): This occurs when the researcher fails to detect a real relationship that actually exists. Example: The researcher may not find a connection between maturity and academic performance, even though older students perform better. 7. Understanding p-values and Probability: Researchers use p-values to determine the likelihood of their results occurring by chance. A p-value less than 0.05 (p < 0.05) means there is a less than 5% chance that the result is due to random chance, making it statistically significant. P-value threshold: ○ p < 0.05: There is a 5% chance of error (Type I). ○ p < 0.01: There is a 1% chance of error. ○ p < 0.001: There is a 0.1% chance of error. 8. Why NHST is Important: NHST helps researchers evaluate whether their results are likely to reflect a real relationship or if they could have arisen by chance. It enables objectivity, ensuring that conclusions are based on data, not personal bias or assumptions. Scientific Theories 1. Definition of Scientific Theory: A scientific theory is a comprehensive framework that organizes evidence related to a particular phenomenon. Unlike everyday usage, where a theory might be just a guess or a belief, a scientific theory is supported by substantial research and evidence. In everyday language: "Theory" is often used as an educated guess or personal belief. For instance, predicting which team will make the playoffs is not based on empirical evidence, but is a speculation. In science: A scientific theory has undergone extensive testing, critique, and empirical validation, making it reliable for explaining, describing, and predicting phenomena. 2. Characteristics of a Good Scientific Theory: Describes: A good theory explains what we observe in the world. Explains: It provides a coherent rationale for why these observations occur. Predicts: It makes predictions that can be tested in future research. Empirically Tested: The theory must be supported by empirical evidence—data collected through systematic observation or experimentation. Falsifiable: A theory must be falsifiable—meaning it can be proven false through evidence. This is a key feature that distinguishes scientific theories from non-scientific ones. 3. The Role of Evidence in Scientific Theories: Scientific theories are developed and supported by research studies. Over time, theories accumulate evidence that supports them, while competing theories are challenged and falsified based on new data. Falsification: Theories are not about proving something absolutely true but about disproving competing explanations. For example, theories about the Sun’s motion evolved as more evidence was collected, leading to the heliocentric theory. 4. Example of Scientific Theory Evolution: Old Theory: In ancient times, people believed that the Sun revolved around the Earth (geocentric theory), as it seemed to fit observable phenomena. New Theory: In the 16th century, astronomers like Copernicus provided evidence through systematic observation and charting of the sky, suggesting the heliocentric theory—that the Earth and other celestial objects revolve around the Sun. The new theory was revised after further evidence came in, and it became the prevailing explanation for planetary motion. 5. Revision of Theories: Open to Change: Scientific theories must be open to revision if new evidence arises that challenges existing explanations. This flexibility is crucial to the advancement of science. Evidence-Driven: The process of theory development is driven by evidence, not just personal beliefs or intuitions. If better data comes along, theories are revised to fit the new understanding.