Google Data Analytics Course Module 1 Notes PDF
Document Details
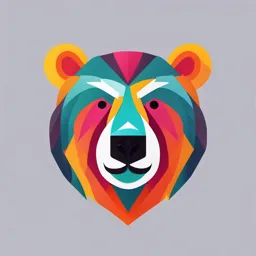
Uploaded by NiceChrysoprase4713
2025
Tags
Summary
This document is a set of notes from a Google Data Analytics course module 1, covering data analysis and data analytics. It includes definitions, lecture notes, summaries, and questions.
Full Transcript
Transform data into insights Saturday, February 1, 2025 12:55 PM Today's Topics: Section: _____________________________________...
Transform data into insights Saturday, February 1, 2025 12:55 PM Today's Topics: Section: ________________________________________________________________________________________________________________________________________________________________ Definitions: ________________________________________________________________________________________________________________________________________________________________ Lecture Notes: During the lecture, take notes here. ________________________________________________________________________________________________________________________________________________________________ Summary: After class, use this space to summarize the main points of this Lecture Topic. ________________________________________________________________________________________________________________________________________________________________ Study Questions After the lecture, create questions based on the notes. Google Data Analytics - Coursera Page 1 Data analytics in everyday life Sunday, January 26, 2025 1:28 PM Today's Topics: Section: Introduction to Data Analytics Data analytics in everyday life Everyday Use of Data Analysis Role of Data in Businesses Definition of Data Analysis Impact of Data Insights Significance of Data Analysts Upcoming Topics ________________________________________________________________________________________________________________________________________________________________ Definitions: Data analysis - The collection, transformation, and organization of data in order to draw conclusions, make predictions, and drive informed decision-making Analysts - convert raw data into actionable insights. ________________________________________________________________________________________________________________________________________________________________ Lecture Notes: During the lecture, take notes here. We use data every day in various aspects of our lives. For example, we analyze our sleep schedules to determine what works best for us or assess how we feel after eating certain foods. This data helps us make decisions, such as when to go to bed, when to wake up, or which foods to avoid to feel our best. Summary: After class, use this space to summarize the main points of this Lecture Topic. Introduction to Data Analytics: Data analytics plays a critical role in decision-making and achieving success across various fields and industries. At Google, data-driven decision-making is integral from concept to product launch. Everyday Use of Data Analysis: People naturally analyze patterns and relationships in their lives to make predictions (e.g., sleep cycles, food effects, or workout times). This personal process mirrors professional data analysis by identifying patterns and making informed decisions. Role of Data in Businesses: Businesses collect massive amounts of data daily and need analysts to organize, interpret, and derive insights from it. Analysts help businesses improve processes, identify trends, launch new products, and enhance customer experiences. Definition of Data Analysis: Data analysis involves collecting, transforming, and organizing data to draw conclusions, make predictions, and drive informed decisions. Analysts convert raw data into actionable insights. Impact of Data Insights: Insights derived from data lead to informed decisions and prompt businesses to take action. Data can inspire businesses to rethink strategies, introduce new products, or deliver better customer experiences. Significance of Data Analysts: Analysts are vital for turning data into insights and helping organizations achieve growth and innovation. The demand for data analysts is high due to the increasing reliance on data-driven strategies. Upcoming Topics: The course will continue exploring how analysts transform data into actionable insights for organizations. ________________________________________________________________________________________________________________________________________________________________ Study Questions After the lecture, create questions based on the notes. 1. Introduction to Data Analytics: ○ What role does data analytics play in decision-making across various fields and industries? ○ How is data-driven decision-making integral to Google's processes from concept to product launch? 2. Everyday Use of Data Analysis: ○ How do people naturally analyze patterns and relationships in their lives to make predictions? ○ In what ways does this personal process mirror professional data analysis? 3. Role of Data in Businesses: ○ Why do businesses need analysts to organize, interpret, and derive insights from the data they collect daily? ○ How do analysts help businesses improve processes, identify trends, launch new products, and enhance customer experiences? 4. Definition of Data Analysis: ○ What are the key steps involved in data analysis? ○ How do analysts convert raw data into actionable insights? 5. Impact of Data Insights: ○ How do insights derived from data lead to informed decisions and prompt businesses to take action? What are some examples of how data can inspire businesses to rethink strategies, introduce new products, or deliver better customer experiences? Google Data Analytics - Coursera Page 2 ○ What are some examples of how data can inspire businesses to rethink strategies, introduce new products, or deliver better customer experiences? 6. Significance of Data Analysts: ○ Why are analysts vital for turning data into insights and helping organizations achieve growth and innovation? ○ What is driving the high demand for data analysts in today's market? Google Data Analytics - Coursera Page 3 New data perspectives Thursday, January 30, 2025 9:03 PM Today's Topics: Section: Using Data Analytics to Improve Employee Retention New data perspectives ________________________________________________________________________________________________________________________________________________________________ Definitions: Data Analysis Process: The six-step process used to analyze data, which includes Ask, Prepare, Process, Analyze, Share, and Act. Employee Retention: The ability of a company to retain its employees and reduce turnover rates. People Analytics: The use of data analysis to improve employee experiences and business efficiency. Employee Satisfaction Survey: A survey designed to gather feedback from employees about their experiences, particularly focusing on hiring, onboarding, and compensation. Data Privacy: Measures taken to protect sensitive information and ensure ethical data collection. Hiring Process: The steps involved in recruiting and selecting new employees. Evaluation Process: The method used to assess employee performance and provide feedback. Standardized Processes: Implementing consistent procedures for hiring and evaluation to improve efficiency and outcomes. Annual Survey: A yearly survey conducted to track progress and gather feedback from employees ________________________________________________________________________________________________________________________________________________________________ Lecture Notes: During the lecture, take notes here. Summary: After class, use this space to summarize the main points of this Lecture Topic. Data is everywhere, and analyzing it can provide insights that drive meaningful changes. A group of people analysts applied the six-step data analysis process—Ask, Prepare, Process, Analyze, Share, and Act—to solve a business problem related to employee retention. The Business Problem A company was experiencing high turnover among new hires, with many leaving within the first year. The analysts sought to determine how to improve retention rates. The Six Steps of Data Analysis in Action 1. Ask: ○ Defined project goals and success criteria. ○ Asked key questions about new employee experiences, existing data, and suspected causes of dissatisfaction. 2. Prepare: ○ Established a three-month timeline. ○ Designed an employee satisfaction survey focusing on hiring, onboarding, and compensation. ○ Set data access rules to protect sensitive information. 3. Process: ○ Ensured ethical data collection, with employee consent. ○ Restricted data access and cleaned data for accuracy. ○ Uploaded raw data to a secure data warehouse. 4. Analyze: ○ Found two key insights: A long and complex hiring process led to higher turnover. An efficient and transparent evaluation process increased employee retention. 5. Share: ○ Presented findings to managers. ○ Encouraged managers to communicate results to teams for productive discussions. 6. Act: ○ Standardized hiring and evaluation processes for efficiency. ○ Implemented an annual survey to track progress. ○ Results showed that these changes successfully improved new hire retention rates. The Impact of People Analytics This case highlights how data analytics can transform workplaces by improving employee experience and business efficiency. If you’re interested in people analytics, it may be worth exploring how data-driven decisions can enhance workplace culture and operations. ________________________________________________________________________________________________________________________________________________________________ Study Questions After the lecture, create questions based on the notes. 1. What are the six steps of the data analysis process mentioned in the lecture? 2. What business problem was the company facing, and what was the goal of the data analysis project? 3. What key questions were asked during the "Ask" step of the data analysis process? Google Data Analytics - Coursera Page 4 4. What measures were taken during the "Prepare" step to ensure data privacy and accuracy? 5. What were the two key insights found during the "Analyze" step? 6. How were the findings shared with managers, and what actions were encouraged? 7. What changes were implemented as a result of the data analysis, and what were the outcomes? 8. How did the use of people analytics impact the company's employee retention rates? 9. Why is it important to have a standardized hiring and evaluation process? 10. How can data-driven decisions enhance workplace culture and operations? Google Data Analytics - Coursera Page 5 How data analysts approach tasks Thursday, January 30, 2025 9:06 PM Today's Topics: Section: The Six Phases of Data Analysis in Action How data analysts approach tasks ________________________________________________________________________________________________________________________________________________________________ Definitions: Data Analysis Process: The six-step process used to analyze data, which includes Ask, Prepare, Process, Analyze, Share, and Act. Ask: Define the problem or question to be answered by gathering input from stakeholders. Prepare: Identify and collect relevant data sources, ensuring the data is accurate and useful. Process: Clean and organize the data by removing inconsistencies, filling in missing values, and formatting it for analysis. Analyze: Perform statistical calculations, identify trends, and extract insights from the data. Share: Present findings using reports, visualizations, or presentations to help stakeholders interpret the results. Act: Implement changes based on the insights to solve the initial business problem. Iteration in Data Analysis: The process is not always linear—analysts may need to revisit previous phases if they find errors or need to refine their approach. ________________________________________________________________________________________________________________________________________________________________ Lecture Notes: During the lecture, take notes here. Summary: After class, use this space to summarize the main points of this Lecture Topic. The data analysis process consists of six key phases: Ask, Prepare, Process, Analyze, Share, and Act. This structured approach helps analysts break down complex business problems into manageable tasks and drive informed decision-making. The Six Phases of Data Analysis 1. Ask: Define the problem or question to be answered by gathering input from stakeholders. 2. Prepare: Identify and collect relevant data sources, ensuring the data is accurate and useful. 3. Process: Clean and organize the data by removing inconsistencies, filling in missing values, and formatting it for analysis. 4. Analyze: Perform statistical calculations, identify trends, and extract insights from the data. 5. Share: Present findings using reports, visualizations, or presentations to help stakeholders interpret the results. 6. Act: Implement changes based on the insights to solve the initial business problem. Case Study: Improving Retirement Contributions at Geo-Flow, Inc. A fictional company, Geo-Flow, Inc., faced an issue where employee participation in their retirement program was lower than expected. They used the six-phase framework to analyze the problem and determine whether an educational training program would help. Ask: Analysts defined the problem by asking if employees were investing in the program and whether education could improve participation. Prepare: They gathered HR data, including demographics, salary levels, and retirement contributions. Process: The data was cleaned and sorted to remove errors, duplicates, and records of former employees. Analyze: The team found that certain employee groups were unaware of the company’s matching contributions and were not fully utilizing the program. Share: Findings were presented through visualizations (bar and pie charts) to help decision-makers understand the issue. Act: Based on the results, the company implemented a targeted educational program. A few months later, contributions significantly increased among the previously low-participating employee groups. Iteration in Data Analysis The process is not always linear—analysts may need to revisit previous phases if they find errors or need to refine their approach. The biggest mistake in data analysis is seeking quick and easy answers instead of following the structured process thoroughly. Key Takeaways The six-phase framework helps analysts tackle business challenges with a structured, data-driven approach. Iteration and constant review are essential for producing quality results. Data insights lead to informed decisions that can improve business operations, such as increasing retirement contributions through targeted education. ________________________________________________________________________________________________________________________________________________________________ Study Questions After the lecture, create questions based on the notes. 1. What are the six phases of the data analysis process? 2. What is the purpose of the "Ask" phase in the data analysis process? 3. During the "Prepare" phase, what steps are taken to ensure the data is accurate and useful? 4. What activities are involved in the "Process" phase of data analysis? 5. How do analysts extract insights during the "Analyze" phase? 6. What methods can be used to present findings in the "Share" phase? 7. What actions might be taken during the "Act" phase to address the initial business problem? Google Data Analytics - Coursera Page 6 7. What actions might be taken during the "Act" phase to address the initial business problem? 8. How did Geo-Flow, Inc. use the six-phase framework to improve employee participation in their retirement program? 9. Why is iteration important in the data analysis process? 10. What are some key takeaways from the six-phase framework for data analysis? Google Data Analytics - Coursera Page 7 Cassie: Dimensions of data analytics Thursday, January 30, 2025 9:19 PM Today's Topics: Section: Choosing Your Path in Data Science: Statistics, Machine Learning, or Dimensions of data analytics Analytics ________________________________________________________________________________________________________________________________________________________________ Definitions: ________________________________________________________________________________________________________________________________________________________________ Lecture Notes: During the lecture, take notes here. Summary: After class, use this space to summarize the main points of this Lecture Topic. Cassie, the leader of Decision Intelligence at Google Cloud, discusses the importance of data analysis and its three key specializations: statistics, machine learning, and analytics. She emphasizes that data analysts must explore and discover insights, rather than expecting predefined answers. Each specialization aligns with different personality traits—statisticians prioritize rigor, machine learning engineers focus on performance, and analysts excel in speed and exploration. She encourages newcomers to embrace curiosity, avoid perfectionism, and enjoy the adventure of working with data. ________________________________________________________________________________________________________________________________________________________________ Study Questions After the lecture, create questions based on the notes. Google Data Analytics - Coursera Page 8 Understand the data ecosystem Saturday, February 1, 2025 12:55 PM Today's Topics: Section: ________________________________________________________________________________________________________________________________________________________________ Definitions: ________________________________________________________________________________________________________________________________________________________________ Lecture Notes: During the lecture, take notes here. ________________________________________________________________________________________________________________________________________________________________ Summary: After class, use this space to summarize the main points of this Lecture Topic. ________________________________________________________________________________________________________________________________________________________________ Study Questions After the lecture, create questions based on the notes. Google Data Analytics - Coursera Page 9 Origins of the data analysis process Thursday, January 30, 2025 9:25 PM Today's Topics: Section: The Evolution and Frameworks of Data Analysis Origins of the data analysis process ________________________________________________________________________________________________________________________________________________________________ Definitions: ________________________________________________________________________________________________________________________________________________________________ Lecture Notes: During the lecture, take notes here. It is time to enter the data analysis process—how industry professionals move from data to decision. All team members can drive success by planning work both upfront and at the end of the data analysis process. While the data analysis process is well known among experts, there isn't a single defined structure or single architecture that’s uniformly followed by every data analyst. But there are some shared fundamentals. This reading provides an overview of several processes, starting with the one that forms the foundation of the Google Data Analytics Certificate. The process presented as part of the Google Data Analytics Certificate is one that will be valuable to you as you keep moving forward in your career: 1. Ask: business challenge, objective, or question 2. Prepare: data generation, collection, storage, and data management 3. Process: data cleaning and data integrity 4. Analyze: data exploration, visualization, and analysis 5. Share: communicating and interpreting results 6. Act: putting insights to work to solve the problem Understanding this process—and all of the iterations that helped make it popular—will be a big part of guiding your own analysis and your work in this program. Let’s go over a few other variations of the data analysis process. EMC's data analysis process EMC Corporation's data analytics process is cyclical with six steps: 7. Discovery 8. Pre-processing data 9. Model planning 10. Model building 11. Communicate results 12. Operationalize EMC Corporation is now Dell EMC. This model, created by David Dietrich, reflects the cyclical nature of typical business projects. The phases aren’t static milestones; each step connects and leads to the next, and eventually repeats. Key questions help analysts test whether they have accomplished enough to move forward and ensure that teams have spent enough time on each of the phases and don’t start modeling before the data is ready. It is a little different from the data analysis process on which this program is based on, but it has some core ideas in common: the first phase is interested in discovering and asking questions; data has to be prepared before it can be analyzed and used; and then findings should be shared and acted on. For more information, refer to this e-book, Data Science & Big Data Analytics. Select PDF under the section that reads Free Access. SAS's iterative process An iterative data analysis process was created by a company called SAS, a leading data analytics solutions provider. It can be used to produce repeatable, reliable, and predictive results: 13. Ask 14. Prepare 15. Explore 16. Model 17. Implement 18. Act 19. Evaluate The SAS model emphasizes the cyclical nature of their model by visualizing it as an infinity symbol. Its process has seven steps, many of which mirror the other models, like ask, prepare, model, and act. But this process is also a little different; it includes a step after the act phase designed to help analysts evaluate their solutions and potentially return to the ask phase again. Project-based data analytics process A project-based data analytics process has five simple steps: 20. Identifying the problem 21. Designing data requirements 22. Pre-processing data 23. Performing data analysis 24. Visualizing data This data analytics project process was developed by Vignesh Prajapati. It doesn’t include the sixth phase, or the act phase. However, it still covers a lot of the same steps described. It begins with identifying the problem, preparing and processing data before analysis, and ends with data visualization. For more information, refer to Understanding the data analytics project life cycle. Big data analytics process Authors Thomas Erl, Wajid Khattak, and Paul Buhler proposed a big data analytics process in their book, Big Data Fundamentals: Concepts, Drivers & Techniques. Their process suggests phases divided into nine steps: 25. Business case evaluation 26. Data identification Google Data Analytics - Coursera Page 10 26. Data identification 27. Data acquisition and filtering 28. Data extraction 29. Data validation and cleaning 30. Data aggregation and representation 31. Data analysis 32. Data visualization 33. Utilization of analysis results This process appears to have three or four more steps than the previous models. But in reality, they have just broken down what has been referred to as prepare and process into smaller steps. It emphasizes the individual tasks required for gathering, preparing, and cleaning data before the analysis phase. For more information, refer to Big Data Adoption and Planning Considerations. From Summary: After class, use this space to summarize the main points of this Lecture Topic. The origins of data analysis trace back to ancient Egypt, where early statisticians recorded data on papyri, laying the foundation for modern analytical processes. While there is no single universally followed data analysis process, several frameworks exist. The Google Data Analytics Certificate follows a six-step approach: Ask, Prepare, Process, Analyze, Share, and Act. Other models, such as EMC’s cyclical process, SAS’s iterative approach, project-based analytics, and big data analytics, share core steps like problem identification, data preparation, analysis, and action. Despite variations, these frameworks emphasize the importance of structuring data-driven decisions. ________________________________________________________________________________________________________________________________________________________________ Study Questions After the lecture, create questions based on the notes. 1. What are the origins of data analysis, and how did early statisticians in ancient Egypt contribute to its development? 2. What is the six-step data analysis process followed by the Google Data Analytics Certificate? 3. How does EMC Corporation's data analytics process differ from the Google Data Analytics Certificate's process? 4. What are the key steps in SAS's iterative data analysis process, and how does it emphasize the cyclical nature of data analysis? 5. Describe the five steps involved in the project-based data analytics process developed by Vignesh Prajapati. 6. What are the nine steps in the big data analytics process proposed by Thomas Erl, Wajid Khattak, and Paul Buhler, and how do they break down the prepare and process phases? 7. Why is it important to have a structured data analysis process, and how does it help in making data-driven decisions? 8. How do the different data analysis frameworks mentioned in the lecture share core steps like problem identification, data preparation, analysis, and action? 9. What are some of the variations in the data analysis processes discussed, and how do they reflect the needs of different business projects? 10. How can understanding various data analysis processes guide your own analysis and work in the Google Data Analytics program? Google Data Analytics - Coursera Page 11 What is the data ecosystem? Thursday, January 30, 2025 9:31 PM Today's Topics: Section: Understanding the Data Ecosystem and the Role of a Data Analyst What is the data ecosystem? ________________________________________________________________________________________________________________________________________________________________ Definitions: Data Ecosystem – A system composed of hardware, software, tools, and people that interact to produce, manage, store, analyze, and share data. The Cloud – A virtual storage location where data is kept online instead of on a local hard drive or an organization's internal network. Data Analyst – A professional who analyzes structured data to answer specific business questions, identify trends, and generate insights for decision-making. Data Scientist – A professional who creates models and algorithms to explore raw data, develop new questions, and solve complex problems using machine learning and AI. Data Analysis – The collection, transformation, and organization of data to find insights, make predictions, and drive informed decision-making. Data Analytics – The broader field that includes data management, tools, techniques, and analysis methods used to extract insights and improve decision-making. Big Data – Large and complex datasets that require advanced tools and techniques to store, process, and analyze efficiently. Data-Driven Decision-Making (DDDM) – The practice of basing decisions on data analysis and interpretation rather than intuition or guesswork. Structured Data – Organized data stored in rows and columns, such as databases and spreadsheets, making it easy to search and analyze. Unstructured Data – Data that does not follow a predefined format, such as emails, social media posts, videos, or images, requiring advanced techniques to analyze. Data Cleaning – The process of removing errors, duplicates, and inconsistencies in a dataset to ensure accuracy and reliability. Data Visualization – The use of charts, graphs, and dashboards to represent data in a visual format for easier interpretation and insights. Predictive Analytics – Using historical data, machine learning, and statistical models to forecast future trends or outcomes. Business Intelligence (BI) – A set of technologies and strategies used to analyze business data and support decision-making. Machine Learning (ML) – A subset of AI where computers learn from data and improve performance without being explicitly programmed. ________________________________________________________________________________________________________________________________________________________________ Lecture Notes: During the lecture, take notes here. The data ecosystem encompasses much more than just the data and the tools used for analysis. It includes: Data Sources: Where the data originates from (e.g., databases, APIs, etc.). Data Management: Processes for storing, organizing, and maintaining data. Data Analysis: The methods and tools used to analyze the data. Data Sharing: How data is communicated and shared across different stakeholders. Data Governance: Policies and standards that ensure data quality and security. From Summary: After class, use this space to summarize the main points of this Lecture Topic. 1. What is a Data Ecosystem? A data ecosystem consists of various elements that interact to produce, manage, store, organize, analyze, and share data. Includes hardware, software tools, and the people who use them (e.g., data analysts). Data can be stored locally or in the cloud, which provides internet-based storage and access. 2. Real-World Examples of Data Ecosystems: Retail: Using customer data to predict future purchases and manage inventory. Human Resources: Analyzing labor market data to improve hiring and employee retention. Agriculture: Tracking geological and weather patterns to predict crop yields. Environmental Conservation: Monitoring coral reefs digitally to track ecosystem changes. 3. Difference Between Data Analysts and Data Scientists: Data Analysts: Find answers to existing business questions using structured data. Data Scientists: Create new questions by developing models and working with raw data. 4. Difference Between Data Analysis and Data Analytics: Data Analysis: The collection, transformation, and organization of data to draw conclusions and make informed decisions. Data Analytics: A broader term encompassing all aspects of managing and using data, including tools, methods, and processes. 5. Importance of Data in Decision-Making: Data ecosystems help organizations make data-driven decisions across various industries. Analysts play a key role in extracting insights from data to guide business strategies. This foundation sets the stage for understanding how data is used for decision-making and how you, as a future data analyst, will contribute to the process. ________________________________________________________________________________________________________________________________________________________________ Study Questions After the lecture, create questions based on the notes. Google Data Analytics - Coursera Page 12 How data informs better decisions Saturday, February 1, 2025 10:49 AM Today's Topics: Section: Data-Driven Decision-Making How data informs better decisions ________________________________________________________________________________________________________________________________________________________________ Definitions: Data-Driven Decision-Making (DDDM) – The practice of using data, facts, and insights to guide business strategy and make informed decisions. Business Need – A specific problem or goal that an organization wants to address using data analysis. Data Insights – Meaningful patterns, trends, or relationships discovered through data analysis that help drive decision-making. Subject Matter Experts (SMEs) – Individuals with deep knowledge and experience in a particular business area who help interpret and validate data insights. Data Strategy – A company's plan for collecting, analyzing, and using data to improve decision-making and business outcomes. Predictive Analytics – A type of data analysis that uses historical data and patterns to make future predictions about business trends, customer behavior, or market changes. Business Intelligence (BI) – The process of analyzing business data and presenting actionable insights to support decision-making. E-commerce – A business model that allows consumers to buy and sell products or services online without a physical store. Streaming Services – Platforms that use data analytics to recommend music, movies, or shows based on user preferences and viewing history. Industry Disruption – When data insights lead to major changes in business models, making older methods or products obsolete (e.g., mobile phones replacing corded phones). ________________________________________________________________________________________________________________________________________________________________ Lecture Notes: During the lecture, take notes here. Summary: After class, use this space to summarize the main points of this Lecture Topic. Everyday Use of Data ○ We use data in daily life, such as fitness trackers and product reviews. ○ Businesses use data to understand customers, improve processes, and enhance employee performance. What is Data-Driven Decision-Making (DDDM)? ○ The process of using facts and data insights to guide business strategy. ○ Helps organizations in various industries make better, informed decisions. Steps in Data-Driven Decision-Making: ○ Identify the business need or problem (e.g., brand recognition, product improvement, employee satisfaction). ○ Collect and analyze data to find trends, patterns, and relationships. ○ Use insights to guide strategy, either building on past successes or exploring new directions. Real-World Examples: ○ Streaming services analyze listening and viewing habits to make personalized recommendations. ○ E-commerce growth was driven by data showing consumer preference for online shopping. ○ Industry shifts, such as the transition from corded phones to mobile devices, were guided by data insights. The Role of Human Expertise in DDDM: ○ Data alone isn’t enough—it must be combined with human experience, observation, and intuition. ○ Subject Matter Experts (SMEs) help validate data insights by identifying inconsistencies and interpreting results. The Role of a Data Analyst: ○ Empower organizations by integrating data into business strategy. ○ Ensure that decision-making is a balance of data insights and human expertise for the best outcomes. Key Takeaway: Data-driven decision-making transforms businesses, but the best decisions combine data insights with human knowledge and experience. ________________________________________________________________________________________________________________________________________________________________ Study Questions After the lecture, create questions based on the notes. Google Data Analytics - Coursera Page 13 Data and gut instinct Saturday, February 1, 2025 10:55 AM Today's Topics: Section: Balancing Data and Gut Instinct in Decision-Making Data and gut instinct ________________________________________________________________________________________________________________________________________________________________ Definitions: Data-Driven Decision-Making (DDDM) – The process of using data, facts, and analysis to guide business strategies and choices. Gut Instinct – An intuitive understanding or feeling about something without concrete data or explanation. Bias – A preconceived notion or preference that can lead to misinterpretations of data or incorrect decisions. Business Knowledge – Understanding the industry, market, and organization to provide context to data insights. Data Analysis Process – The structured approach to analyzing data, including: Ask: Define the problem. Prepare: Collect and store relevant data. Process: Clean and check data for accuracy. Analyze: Identify trends and patterns. Share: Present findings effectively. Act: Use insights to make decisions. Project Constraints – Factors such as time limits, available resources, and business needs that affect how much data analysis can be performed. ________________________________________________________________________________________________________________________________________________________________ Lecture Notes: During the lecture, take notes here. Detectives and data analysts have a lot in common. Both depend on facts and clues to make decisions. Both collect and look at the evidence. Both talk to people who know part of the story. And both might even follow some footprints to see where they lead. Whether you’re a detective or a data analyst, your job is all about following steps to collect and understand facts. Analysts use data-driven decision-making and follow a step-by-step process. You have learned that there are six steps to this process: 1. Ask questions and define the problem. 2. Prepare data by collecting and storing the information. 3. Process data by cleaning and checking the information. 4. Analyze data to find patterns, relationships, and trends. 5. Share data with your audience. 6. Act on the data and use the analysis results. But there are other factors that influence the decision-making process. You may have read mysteries where the detective used their gut instinct, and followed a hunch that helped them solve the case. Gut instinct is an intuitive understanding of something with little or no explanation. This isn’t always something conscious; we often pick up on signals without even realizing. You just have a “feeling” it’s right. Why gut instinct can be a problem At the heart of data-driven decision making is data. Therefore, it's essential that data analysts focus on the data to ensure they make informed decisions. If you ignore data by preferring to make decisions based on your own experience, your decisions may be biased. But even worse, decisions based on gut instinct without any data to back them up can cause mistakes. Consider an example of a restaurant entrepreneur, partnering with a well-known chef to develop a new restaurant in a bustling part of the city’s central shopping district. The chef has several restaurants across the city. Banking on their reputation, the restaurant entrepreneur and chef followed gut instinct and created another uniquely themed restaurant. However, fundraising efforts fell short to fund the opening of the restaurant after months of planning and preparation. The property will go back on the market to be sold at a loss. Had the entrepreneur done more research, they would've found data showing prospective customers in this new restaurant location were very different from the chef's other restaurants. The more you understand the data related to a project, the easier it will be to figure out what is required. These efforts will also help you identify errors and gaps in your data so you can communicate your findings more effectively. Sometimes past experience helps you make a connection that no one else would notice. For example, a detective might be able to crack open a case because they remember an old case just like the one they’re solving today. It's not just gut instinct. Data + business knowledge = mystery solved Blending data with business knowledge, plus maybe a touch of gut instinct, will be a common part of your process as a junior data analyst. The key is figuring out the exact mix for each particular project. A lot of times, it will depend on the goals of your analysis. That is why analysts often ask, “How do I define success for this project?” Google Data Analytics - Coursera Page 14 project?” In addition, try asking yourself these questions about a project to help find the perfect balance: What kind of results are needed? Who will be informed? Am I answering the question being asked? How quickly does a decision need to be made? For instance, if you are working on a rush project, you might need to rely on your own knowledge and experience more than usual. There just isn’t enough time to thoroughly analyze all of the available data. But if you get a project that involves plenty of time and resources, then the best strategy is to be more data-driven. It’s up to you, the data analyst, to make the best possible choice. You will probably blend data and knowledge a million different ways over the course of your data analytics career. And the more you practice, the better you will get at finding that perfect blend. Key takeaways Data analysts and detectives share a similar approach to problem-solving, both relying on evidence and facts to make decisions. Data-driven decision-making is essential for analysts, but gut instinct can also play a role in identifying patterns and connections. Balancing data and gut instinct is crucial for making informed decisions, and the right mix depends on the project's goals and time constraints. From ________________________________________________________________________________________________________________________________________________________________ Summary: After class, use this space to summarize the main points of this Lecture Topic. Data analysts and detectives have a lot in common—they both gather evidence, analyze patterns, and make decisions based on facts. The data-driven decision- making process involves six steps: Ask, Prepare, Process, Analyze, Share, and Act. However, gut instinct—an intuitive feeling without direct evidence—can sometimes influence decision-making. While intuition can help, relying solely on it can lead to mistakes, as seen in the restaurant example where lack of data analysis led to financial failure. The best approach is to blend data insights with business knowledge and, when necessary, intuition. The right balance depends on project constraints, such as available time and required outcomes. The more analysts practice, the better they become at finding this balance. ________________________________________________________________________________________________________________________________________________________________ Study Questions After the lecture, create questions based on the notes. Google Data Analytics - Coursera Page 15 analytical thinking self-assessment Conducting an analytical thinking self-assessment is significant because it helps individuals evaluate their own analytical skills and thought processes. This can include: Identifying Strengths and Weaknesses: Understanding where you excel in data analysis and where you may need improvement. Enhancing Problem-Solving Skills: Reflecting on how you approach data-related problems can lead to better strategies for analysis. Building Confidence: Recognizing your capabilities can boost your confidence in tackling data challenges. This self-assessment is a valuable tool for personal and professional growth in the field of data analytics. From Strengths: 1. Clear understanding of the data life cycle and its parallels to project management. 2. Insightful recognition of the role of data visualization in communication. 3. Strong understanding of data cleaning and its importance. 4. Good grasp of the role of data visualization in communicating insights. Areas for Further Practice: 1. Review the specific terminology related to data analysis processes to strengthen your vocabulary. 2. Explore more about data-driven decision-making practices in e-commerce settings. 3. Review the components of the data ecosystem to solidify your understanding. 4. Explore more about analytical thinking and its application in data analytics. From Difference Between Data Analysis and Data Analytics Although the terms data analysis and data analytics sound similar, they have distinct meanings in the field of data science. Category Data Analysis Data Analytics Definition The process of collecting, A broader discipline that includes transforming, and organizing data managing, analyzing, and applying data to draw conclusions and make to solve problems and improve decision- decisions. making. Scope A specific subset of data analytics An umbrella term that includes data focused on examining past data for analysis, as well as predictive modeling, insights. machine learning, and automation. Purpose To interpret existing data, identify To apply various techniques, tools, and trends, and answer specific business strategies to process and utilize data questions. effectively. Approach Typically descriptive(e.g., "What Can be descriptive, diagnostic, happened?"). predictive, or prescriptive(e.g., "What will happen?" or "What should we do?"). Examples - Summarizing sales data to identify best-selling products. Google Data Analytics - Coursera Page 16 best-selling products. Cleaning and structuring a dataset for reporting. | - Using machine learning to predict customer churn. Automating real-time data collection and visualization. | Key Takeaway: Data analysis is a subset of data analytics, focused on interpreting and examining data. Data analytics is a broader field that includes managing, analyzing, predicting, and optimizing data for decision-making. Google Data Analytics - Coursera Page 17 Test 1 Saturday, February 1, 2025 11:35 AM first test Google Data Analytics - Coursera Page 18 Google Data Analytics - Coursera Page 19 Embrace your data analyst skills Saturday, February 1, 2025 12:57 PM Today's Topics: Section: ________________________________________________________________________________________________________________________________________________________________ Definitions: ________________________________________________________________________________________________________________________________________________________________ Lecture Notes: During the lecture, take notes here. ________________________________________________________________________________________________________________________________________________________________ Summary: After class, use this space to summarize the main points of this Lecture Topic. ________________________________________________________________________________________________________________________________________________________________ Study Questions After the lecture, create questions based on the notes. Google Data Analytics - Coursera Page 20 Key data analyst skills Saturday, February 1, 2025 11:40 AM Today's Topics: Section: Essential Analytical Skills for Data Analysts Key data analyst skills ________________________________________________________________________________________________________________________________________________________________ Definitions: Analytical Skills – The ability to solve problems using facts, logic, and structured thinking. Curiosity – The drive to ask questions and seek new knowledge. Context – The surrounding information or conditions that help give meaning to data. Technical Mindset – The ability to break down problems into smaller, logical steps for better problem-solving. Data Design – The process of organizing and structuring data for efficiency and clarity. Data Strategy – The management of people, processes, and tools to optimize data analysis and decision-making. ________________________________________________________________________________________________________________________________________________________________ Lecture Notes: During the lecture, take notes here. Analytical skills, such as curiosity, understanding context, technical thinking, data design, and data strategy, are essential for data analysts and can be developed through daily practice. 🚀 ________________________________________________________________________________________________________________________________________________________________ Summary: After class, use this space to summarize the main points of this Lecture Topic. Many people already have analytical skills without realizing it. Analytical skills involve solving problems using facts and are crucial for data analysts. The five essential skills are: 1. Curiosity – The desire to learn and explore new information, which drives analytical thinking. 2. Understanding Context – Recognizing how information fits into a larger structure or environment to ensure accuracy and relevance. 3. Technical Mindset – The ability to break down complex tasks into smaller, logical steps, such as organizing and prioritizing bills. 4. Data Design – Organizing information in a clear and logical way, like sorting phone contacts or structuring a database. 5. Data Strategy – Managing people, processes, and tools in data analysis, ensuring the right data and resources are used effectively. These skills are applied in everyday life, such as grouping groceries, organizing contacts, and following step-by-step processes for tasks like paying bills or mowing a lawn. By recognizing and practicing these skills, aspiring data analysts can improve their ability to analyze and interpret data effectively. ________________________________________________________________________________________________________________________________________________________________ Study Questions After the lecture, create questions based on the notes. Google Data Analytics - Coursera Page 21 Use data analytics skills in a business scenario Saturday, February 1, 2025 11:52 AM Today's Topics: Section: Applying Analytical Skills in Data Analysis: A Case Study Use data analytics skills in a business scenario ________________________________________________________________________________________________________________________________________________________________ Definitions: Analytical Skills – The ability to solve problems using logical reasoning, data, and structured thinking. Exploratory Data Analysis (EDA) – A method of examining datasets to find patterns, trends, and relationships before drawing conclusions. Curiosity – The drive to ask questions, explore new possibilities, and seek deeper insights in data. Context – The environment, structure, or conditions that give meaning to data and influence decision-making. Technical Mindset – The skill of breaking down complex problems into smaller, logical steps for analysis. Data Design – The process of structuring and organizing data to enhance analysis and reveal patterns. Data Strategy – The management of tools, processes, and people used in data analysis to optimize outcomes. Resource Allocation – Distributing time, tools, and effort based on project priorities and goals. ________________________________________________________________________________________________________________________________________________________________ Lecture Notes: During the lecture, take notes here. As you’ve been learning, data analysts have inherent analytical skills, whether they know it or not. In other words, the interests that have led you to pursue a career as a data analyst create a foundation that you will build on throughout your career. To review, these skills are: Curiosity Understanding of context Technical mindset Data design Data strategy It’s important to recognize these skills and begin applying them to all sorts of situations. In this reading, you’ll do that through a case study based on a fictitious film production company, Mega-Pik International. Scenario: Use data to create better movies The movies Mega-Pik has released recently aren’t having the impact they used to. Five of their last six releases barely broke even at the box office, and the sixth film lost a lot of money. The lead executives at Mega-Pik have noticed that their competitors went through a similar slump, but recovered when they started producing remakes of past successes and marketing them to a new audience. Mega-Pik is interested in following this trend. They want to do this based on data-driven strategies, so they hire your analytics company to help them make popular movies again. Specifically, they ask for exploratory data analysis (EDA) to help them understand what audiences have liked in the past and determine if the successes of those films can be replicated. You and your team develop the following objectives for Mega-Pik’s EDA: Identify key factors that contribute to a movie's opening weekend success. Understand the relationship between a movie's budget and its revenue. Determine which genres are most successful. The right dataset Your company collects, cleans, and organizes the following relevant information into a dataset: Movie name Release date Opening night revenue Opening weekend revenue Budget (cost to create) Marketing costs Ratings Genre Use your skills Now you’ll examine how inherent data analysis skills can help you guide Mega-Pik to make data-driven decisions about which movies to produce. Curiosity Google Data Analytics - Coursera Page 22 If you worked for the company performing this data analysis, what kinds of questions would you ask based on the data and how it relates to the objectives of the EDA? Curiosity is critical here, because it will help you come up with questions you can answer For example, you might wonder if there’s a relationship between a movie’s budget and the revenue it generates on opening night or over the opening weekend. You might also be curious about combining columns to make new metrics, such as which genres tend to perform better on opening weekend—both overall and in the seasons in which the movies were released. You might even ask if there should be additional columns of data that you don’t already have, such as audience demographics. Curiosity is a skill that drives analysts to discover just how much information they can coax out of the data in expected or unexpected ways. Keep in mind that curiosity isn’t the only skill that compels analysts to ask probing questions about their data. Understanding context Context is crucial for any kind of meaningful data analysis. By contextualizing data, you start to understand why the data shows what it does. Factors including the time of year a movie is released, holidays, and competing events can all have an effect on revenue, which is the gauge Mega-Pik uses to determine success. Audience demographics such as age, gender, education, and income levels can help you understand who is going to the movies. This context might clarify which genres or storylines are most interesting to movie-goers. Analysts determine context by looking for patterns or anomalies in a dataset. It also helps to understand the entertainment industry, which provides a whole other set of contextual clues. For example, family films typically generate more revenue when children are on vacation from school. This provides important context about the relationship between genre and revenue over a short timeframe. To understand the relationship between family films and revenue, you might have to search over a time period of more than one year to avoid inaccurate conclusions based on school schedule. Further the “season” in which children are on vacation from school differs by country, which is another contextual clue you have to take into account. An accurate analysis of this data needs to come from cross-referencing all of the various contexts, including external data or historical trends. Understanding context helps you solve problems by narrowing down variables that are most likely to influence the outcome, which in turn enables you to come up with more meaningful insights. Technical mindset Google Data Analytics - Coursera Page 23 As you have discovered, having a technical mindset means approaching problems (and datasets) in a systematic and logical manner. This starts with the way you clean, organize, and prepare your data. It can also guide the tools or software you use to break down data and help you identify and fix incorrect data that can skew your analysis. Remember that problems aren't always technical, but a technical mindset is the skill that you use to break down any complex issue into manageable parts. Focusing on implementing a process, regardless of what that looks like, is a great first step to exercising your technical mindset. Data design The skill of data design is an extension of your technical mindset. It deals with how information is organized. Suppose the dataset here is presented in a spreadsheet. You would be able to shift the cells to organize the data to find different patterns. For example, you might organize the data by revenue and then by genre, which could reveal that comedies are more profitable than dramas. Basically, how you choose to structure your data makes analysis easier and more insightful. Data strategy Google Data Analytics - Coursera Page 24 Data strategy is the management of the people, processes, and tools used in data analysis. In this scenario, think of it as the approach you use to analyze your dataset. One element might be the tools you use. If Mega-Pik wants a relatively simple dashboard, you might use Google Sheets or Excel because there are only a few columns of data. On the other hand, if Mega-Pik wants a dashboard where information updates every time new data comes in, you’d need a robust tool like Tableau. The data strategy you select should be based on the dataset and the deliverables. Think about a data strategy as a kind of resource allocation—the tools, time, and effort that you put into a project will vary based on what you need to accomplish. One strategy you might use for this case study is to prioritize any analyses that would directly affect the next quarter's revenue. The way you allocate resources can lead you to quicker, more actionable insights. Key takeaways Your inherent analytical skills are essential for conducting data analysis and will be even more critical when you combine them with the tools and techniques from this program. Understanding how to use these skills in business scenarios is the first step toward developing them further and using them effectively in your career. From ________________________________________________________________________________________________________________________________________________________________ Summary: After class, use this space to summarize the main points of this Lecture Topic. Analytical skills are essential for data analysts, and many people already possess them without realizing it. These skills—curiosity, understanding context, a technical mindset, data design, and data strategy—help analysts structure their approach to solving business problems. A case study featuring Mega-Pik International, a struggling film production company, highlights how these skills apply in real-world data analysis. Analysts use Exploratory Data Analysis (EDA) to: 1. Identify key factors for a movie's opening weekend success. 2. Understand the relationship between a movie's budget and revenue. 3. Determine which genres perform best. Each analytical skill plays a role: Curiosity helps analysts ask meaningful questions and explore relationships between data points. Understanding context ensures data is interpreted correctly, considering factors like seasonality, audience demographics, and industry trends. Technical mindset enables analysts to systematically clean, organize, and analyze data. Data design structures the dataset in ways that reveal useful patterns. Data strategy determines the best tools and processes to generate actionable insights. By combining analytical skills, tools, and techniques, data analysts can help businesses make data-driven decisions and improve outcomes. Understanding and applying analytical skills in real-world business scenarios is crucial for making data-driven decisions. These skills, combined with the right tools and techniques, will help you succeed as a data analyst. 🚀 ________________________________________________________________________________________________________________________________________________________________ Study Questions After the lecture, create questions based on the notes. Google Data Analytics - Coursera Page 25 Test 2 Saturday, February 1, 2025 12:04 PM test 2 Google Data Analytics - Coursera Page 26 Google Data Analytics - Coursera Page 27 Analytical thinking for effective outcomes Saturday, February 1, 2025 12:44 PM Today's Topics: Section: ________________________________________________________________________________________________________________________________________________________________ Definitions: ________________________________________________________________________________________________________________________________________________________________ Lecture Notes: During the lecture, take notes here. ________________________________________________________________________________________________________________________________________________________________ Summary: After class, use this space to summarize the main points of this Lecture Topic. ________________________________________________________________________________________________________________________________________________________________ Study Questions After the lecture, create questions based on the notes. Google Data Analytics - Coursera Page 28 All about thinking analytically Saturday, February 1, 2025 12:58 PM Today's Topics: Section: The Five Key Aspects of Analytical Thinking in Data Analysis All about thinking analytically ________________________________________________________________________________________________________________________________________________________________ Definitions: Analytical Thinking – A problem-solving approach that involves structuring, analyzing, and interpreting data to find solutions. Visualization – Using graphs, maps, and charts to represent data in a way that is easier to interpret. Strategy – A planned, goal-oriented approach that helps analysts focus on achieving meaningful insights from data. Problem-Orientation – The practice of keeping the primary problem in focus throughout the entire analysis. Correlation – A relationship between two or more variables, indicating how they move together, but not necessarily implying one causes the other. Causation – When one event directly causes another (unlike correlation, which only shows a relationship). Big-Picture Thinking – The ability to see overarching patterns and trends to drive strategic decision-making. Detail-Oriented Thinking – The ability to focus on small but essential data points that contribute to the overall analysis. ________________________________________________________________________________________________________________________________________________________________ Lecture Notes: During the lecture, take notes here. ________________________________________________________________________________________________________________________________________________________________ Summary: After class, use this space to summarize the main points of this Lecture Topic. Analytical thinking is the ability to identify and define problems, then solve them using data in a structured, step-by-step manner. As a data analyst, developing strong analytical thinking skills is essential. There are five key aspects to analytical thinking: 1. Visualization – The graphical representation of data (e.g., graphs, maps, and charts) helps analysts understand and communicate insights more effectively. 2. Strategy – A structured approach to working with data, ensuring that collected data is valuable and contributes to solving the problem. 3. Problem-Orientation – Keeping the problem at the center of the analysis, using questioning and data-driven methods to find solutions. 4. Correlation – Identifying relationships between different data points, while remembering that correlation does not imply causation. 5. Big-Picture and Detail-Oriented Thinking – The ability to see both the overall goal and the smaller details that contribute to solving the problem. Each of these skills plays a crucial role in making data-driven decisions. Most people are naturally stronger in one area, but by developing all five skills, data analysts can think critically, solve complex problems, and generate valuable insights. Key Takeaway: Analytical thinking in data analysis involves visualization, strategy, problem-orientation, correlation analysis, and balancing big-picture and detail-oriented thinking. Mastering these skills enables analysts to approach problems effectively and generate meaningful insights. 🚀 ________________________________________________________________________________________________________________________________________________________________ Study Questions After the lecture, create questions based on the notes. Google Data Analytics - Coursera Page 29 Explore core analytical skills Saturday, February 1, 2025 1:08 PM Today's Topics: Section: Analytical Thinking and Problem-Solving in Data Analysis Explore core analytical skills ________________________________________________________________________________________________________________________________________________________________ Definitions: Root Cause – The underlying reason why a problem occurs, which must be addressed to prevent future issues. Five Whys – A problem-solving technique that involves asking "why?" five times to reveal the root cause of a problem. Gap Analysis – A method used to compare current vs. desired outcomes to identify missing elements and areas for improvement. Data-Driven Decisions – Business decisions guided by data and analysis, rather than assumptions or intuition. Analytical Thinking – A structured problem-solving approach that involves identifying, analyzing, and interpreting data to find solutions. ________________________________________________________________________________________________________________________________________________________________ Lecture Notes: During the lecture, take notes here. ________________________________________________________________________________________________________________________________________________________________ Summary: After class, use this space to summarize the main points of this Lecture Topic. Analytical thinking consists of five key aspects: visualization, strategy, problem-orientation, correlation, and big-picture/detail-oriented thinking. While people naturally excel in certain types of thinking, they can develop and strengthen their skills in others, making them more versatile thinkers. Data analysts must think critically and creatively to uncover hidden insights and find solutions that are not immediately obvious. They frequently ask key questions to guide their analysis, such as: 1. What is the root cause of a problem? ○ The Five Whys technique helps analysts dig deeper into a problem by asking “why?” five times to uncover its true cause. ○ Example: A late frost damaging mulberry bushes indirectly led to a shortage of blueberries in stores. 2. Where are the gaps in our process? ○ Gap Analysis is used to compare the current state of a process to its ideal future state and identify gaps that need to be filled for improvement. 3. What did we not consider before? ○ Encourages looking beyond obvious factors to discover missing information or overlooked opportunities for better decision-making. By mastering analytical thinking and asking the right questions, data analysts help businesses improve processes, solve problems, and make data-driven decisions that drive success. Key Takeaway: Effective data analysts think critically, ask the right questions, and use structured methods like the Five Whys and Gap Analysis to uncover root causes, identify gaps, and drive data-informed decision-making. 🚀 ________________________________________________________________________________________________________________________________________________________________ Study Questions After the lecture, create questions based on the notes. Google Data Analytics - Coursera Page 30 Use the five whys for root cause analysis Saturday, February 1, 2025 1:13 PM Today's Topics: Section: Use the five whys for root cause analysis Use the five whys for root cause analysis ________________________________________________________________________________________________________________________________________________________________ Definitions: Root Cause – The fundamental issue that leads to a problem occurring. Five Whys – A problem-solving technique that involves asking "why?" five times (or more) to identify the root cause of an issue. Root Cause Analysis (RCA) – The process of investigating and identifying the underlying reason for a problem. Process Improvement – Making changes to workflows, training, or systems to prevent future problems. Business Intelligence – The use of data-driven insights to solve problems and optimize business performance. ________________________________________________________________________________________________________________________________________________________________ Lecture Notes: During the lecture, take notes here. Use the five whys for root cause analysis Recently, you’ve been learning why business solutions almost always require some data detective work. This is one way critical thinking helps data professionals determine the right questions to ask in order to arrive at those solutions. One very common question is, “What is the root cause of the problem?” A root cause is the reason why a problem occurs. So, by identifying and eliminating the root cause, data professionals can help stop that problem from occurring again. The five whys is a simple but effective technique for identifying a root cause. It involves asking "Why?" repeatedly until the answer reveals itself. This often happens at the fifth “why,” but sometimes you’ll need to continue asking more times, sometimes fewer. You recently explored a case involving lacking the necessary ingredients to bake pies; now, you’ll go more in-depth with some business applications of the five whys technique to do root cause analysis. Boost customer service An online grocery store was receiving numerous customer service complaints about poor deliveries. To address this problem, a data analyst at the company asked their first “why?” Why #1. “Customers are complaining about poor grocery deliveries. Why?” The data analyst began by reviewing the customer feedback more closely. They noted the vast majority of complaints dealt with products arriving damaged. So, they asked “why?” again. Why #2. “Products are arriving damaged. Why?” To answer this question, the data analyst continued exploring the customer feedback. It turned out that many customers said products were not packaged properly. Why #3. “Products are not packaged properly. Why?” After asking their third “why,” the data analyst did some further detective work. They ultimately learned that their company’s grocery packers were not adequately trained on packing procedures. Why #4. “Grocery packers are not adequately trained. Why?” This “why” enabled the data analyst to uncover that nearly 35% of all packers were new to the company. They had not yet had the chance to complete all required training, yet they were already being asked to pack groceries for customer orders. Why #5. “Packers have not completed required training. Why?” This final “why?” led the data analyst to find out that the human resources department had not provided necessary training to any newly hired packers. This was because HR was in the middle of reworking the training program. Rather than training new hires using the old Google Data Analytics - Coursera Page 31 hired packers. This was because HR was in the middle of reworking the training program. Rather than training new hires using the old system, they had provided them with a quick one-page guide, which was insufficient. So, in this example, the root cause of the problem was that HR had not completed the training program updates and was using a less- thorough guide to train new packers. Fortunately, this was a problem that the grocer could control. And thanks to the data analyst’s work, they provided more support to the HR department to complete the training and retrain all newly hired grocery packers! Advance quality control An irrigation company was experiencing an increase in the number of defects in their water pumps. The company's data team used the five whys to analyze the situation: Why #1. “There has been an increase in the number of defects in water pumps. Why?” To answer this question, the data team set up a meeting with shop floor engineers. They asked for some insights into machine performance and manufacturing processes. After some exploration, it was discovered that the machines used to produce the pumps were not properly calibrated. Why #2. “The machines are not properly calibrated. Why?” After more brainstorming with the engineering team, it was determined that the machines were miscalibrated during the last maintenance cycle. Why #3. “The machines were miscalibrated during maintenance. Why?” Next, the data team investigated the procedures involved with machine calibration. They found out that the current method was inappropriate for the machines. Why #4. “The calibration method is inappropriate for the machines. Why?” This “why” led them to discover that the company had recently installed new software in their machines. Because it was a minor software upgrade, the engineers didn’t realize it would affect calibration. They didn’t have the information they needed to properly calibrate the upgraded machines. Why #5. “The engineers don’t have the information they need to calibrate the upgraded machines. Why?” The fifth and final “why” turned up even more evidence: The installation team had upgraded machine software, but had failed to share the corresponding calibration procedures with the engineers. So, in this example, the root cause of the problem was that the engineers lacked important information about how to calibrate the machines using the new software system. The solution was found, and the irrigation company was able to implement it right away. Soon, the engineers had the necessary calibration instructions, and the pump defects were eliminated! Key takeaways The five whys is a powerful tool for root cause analysis. It’s simple, effective, and a great way to collaborate with colleagues and learn about other areas of the business. Plus, the five whys can be used to analyze problems in any industry, helping organizations of all kinds identify and fix business problems. As a data professional, you can turn to the five whys whenever you feel stumped by a problem and need to approach it from a different perspective. From ________________________________________________________________________________________________________________________________________________________________ Summary: After class, use this space to summarize the main points of this Lecture Topic. The Five Whys is a simple yet effective technique for identifying the root cause of a problem by repeatedly asking “Why?” until the true issue is revealed. This method is commonly used in business problem-solving and data analysis. Case Study Examples: 1. Customer Service Issue (Online Grocery Store) ○ Problem: Customers complained about damaged grocery deliveries. ○ Root Cause: HR had not provided proper training to new grocery packers due to an incomplete training program update. ○ Solution: HR was given support to complete and implement a new training program. 2. Quality Control Issue (Irrigation Company) ○ Problem: There was an increase in defective water pumps. ○ Root Cause: Engineers lacked updated calibration instructions after a software upgrade. ○ Solution: The installation team provided proper calibration instructions, eliminating defects. The Five Whys is valuable because it: Helps analysts dig deeper into business problems. Encourages cross-team collaboration to find solutions. Can be used in any industry to improve efficiency and prevent recurring issues. Key Takeaway: The Five Whys method is a powerful tool that helps data professionals identify root causes, solve business problems, and prevent issues from recurring. 🚀 ________________________________________________________________________________________________________________________________________________________________ Study Questions After the lecture, create questions based on the notes. Google Data Analytics - Coursera Page 32 Data drives successful outcomes Saturday, February 1, 2025 1:24 PM Today's Topics: Section: How Analytical Skills Support Data-Driven Decision-Making https://www.coursera.org/learn/foundations-data/lecture/YXQHf/data-drives- successful-outcomes# ________________________________________________________________________________________________________________________________________________________________ Definitions: Data-Driven Decision-Making (DDDM) – Using data, facts, and analysis to guide business strategies rather than intuition or assumptions. Curiosity – The drive to ask questions, explore data, and identify meaningful insights. Context – The conditions and background that help analysts interpret data accurately. Technical Mindset – A structured problem-solving approach that involves breaking down data into logical steps. Data Design – The process of structuring and organizing data for easy access and meaningful analysis. Data Strategy – A high-level plan that incorporates people, processes, and tools to solve business problems effectively. ________________________________________________________________________________________________________________________________________________________________ Lecture Notes: During the lecture, take notes here. ________________________________________________________________________________________________________________________________________________________________ Summary: After class, use this space to summarize the main points of this Lecture Topic. Data-driven decision-making (DDDM) involves using facts and data to guide business strategies instead of relying on gut feelings or assumptions. This approach helps businesses gain insights, verify theories, identify opportunities and challenges, and make more informed, confident decisions. Examples of DDDM: A dairy farmer surveys customers to find out which ice cream flavors they prefer instead of guessing. A company president uses an employee survey to determine the most valued workplace perk, rather than relying on an HR director’s assumptions. The five essential analytical skills—curiosity, understanding context, having a technical mindset, data design, and data strategy—play a major role in effective decision-making: 1. Curiosity & Context: Analysts naturally seek patterns, relationships, and insights by exploring data and applying context to make meaningful predictions and conclusions. 2. Technical Mindset: Analysts go beyond gut feelings by seeking out facts, applying structured analysis, and making evidence-based decisions. 3. Data Design: Organizing data logically and efficiently makes it easier to analyze and extract valuable insights. 4. Data Strategy: A structured approach that incorporates people, processes, and tools ensures the success of data-driven decisions. Since DDDM is a collaborative effort, organizations need clear procedures, technology, and alignment with their overall data strategy. By applying these five skills, analysts ensure that businesses make better, more informed decisions. Key Takeaway: Data-driven decision-making, supported by strong analytical skills, leads to better business outcomes by replacing assumptions with factual insights. 🚀 ________________________________________________________________________________________________________________________________________________________________ Study Questions After the lecture, create questions based on the notes. Google Data Analytics - Coursera Page 33 Witness data magic Saturday, February 1, 2025 1:33 PM Today's Topics: Section: Real-World Applications of Data-Driven Decision-Making Witness data magic ________________________________________________________________________________________________________________________________________________________________ Definitions: Data-Driven Decision-Making (DDDM) – Using data, facts, and insights to guide business and strategic decisions. Quartile – A statistical division that splits data into four equal parts to compare trends and distributions. Data Visualization – Representing data graphically (e.g., charts, graphs, and dashboards) to reveal insights. Engagement Metrics – Data points that measure reader interaction, such as clicks, shares, and comments. Performance Analysis – The process of evaluating employee, business, or campaign effectiveness using data insights. ________________________________________________________________________________________________________________________________________________________________ Lecture Notes: During the lecture, take notes here. ________________________________________________________________________________________________________________________________________________________________ Summary: After class, use this space to summarize the main points of this Lecture Topic. Data analysts play a crucial role in helping organizations make data-driven decisions that improve efficiency, effectiveness, and overall success. Two real-world case studies demonstrate how data analysis leads to better business strategies: 1. Google’s HR Analytics ○ Google wanted to determine whether managers were necessary or if employees should work as individual contributors. ○ Data analysts reviewed performance reviews and employee surveys and visualized the data using quartiles. ○ The analysis revealed that teams with the best managers were happier, more productive, and more engaged. ○ Additional data collection, including employee nominations and manager interviews, helped define key management behaviors for success. ○ Google used these insights to improve manager evaluations and create a better company culture. 2. Nonprofit Journalism Analysis ○ Data analysts studied how journalists can make a greater impact when writing about nonprofit causes. ○ They tracked and analyzed story topics, clicks, web traffic, shares, and reader engagement. ○ Insights helped journalists improve their content strategies to better educate and engage audiences. ○ This resulted in greater awareness, increased donations, and more community action toward social causes. These case studies highlight how data analysts drive meaningful change in both corporate and nonprofit settings. The ability to ask the right questions, analyze data effectively, and communicate insights ensures better decision-making across industries. Key Takeaway: Data analysis empowers organizations to make informed, impactful decisions, leading to better management, increased engagement, and stronger business strategies. 🚀 ________________________________________________________________________________________________________________________________________________________________ Study Questions After the lecture, create questions based on the notes. Google Data Analytics - Coursera Page 34 Test 3 Saturday, February 1, 2025 1:38 PM test 3 Google Data Analytics - Coursera Page 35 test 3 retake Google Data Analytics - Coursera Page 36 Google Data Analytics - Coursera Page 37 Glossary terms from course 1, module 1 Saturday, February 1, 2025 1:40 PM Terms and definitions for Course 1, Module 1 Analytical skills: Qualities and characteristics associated with using facts to solve problems Analytical thinking: The process of identifying and defining a problem, then solving it by using data in an organized, step-by-step manner Context: The condition in which something exists or happens Data: A collection of facts Data analysis: The collection, transformation, and organization of data in order to draw conclusions, make predictions, and drive informed decision-making Data analyst: Someone who collects, transforms, and organizes data in order to draw conclusions, make predictions, and drive informed decision-making Data analytics: The science of data Data design: How information is organized Data-driven decision-making: Using facts to guide business strategy Data ecosystem: The various elements that interact with one another in order to produce, manage, store, organize, analyze, and share data Data science: A field of study that uses raw data to create new ways of modeling and understanding the unknown Data strategy: The management of the people, processes, and tools used in data analysis Data visualization: The graphical representation of data Dataset: A collection of data that can be manipulated or analyzed as one unit Gap analysis: A method for examining and evaluating the current state of a process in order to identify opportunities for improvement in the future Root cause: The reason why a problem occurs Technical mindset: The ability to break things down into smaller steps or pieces and work with them in an orderly and logical way Visualization: (Refer to data visualization) From Google Data Analytics - Coursera Page 38