Module 9 - Ch04 Sampling Distribution PDF
Document Details
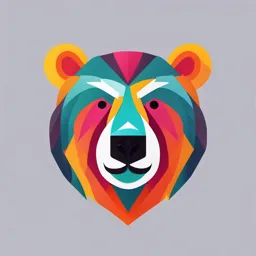
Uploaded by ConsistentDenouement2299
Lee Shau Kee School of Business and Administration, Hong Kong Metropolitan University
Tags
Summary
This document provides an overview of sampling distributions, specifically focusing on the sampling distribution of the mean. It covers topics such as theoretical probability distribution of a sample statistic, the calculation of the sampling distribution of the mean, and an illustrative example. The document also discusses how to compare population and sampling distributions and the Central Limit Theorem. It details situations where the sampling distribution can be approximated by a normal distribution; for example, if the sample size is at least 30. It concludes with an example of a sampling distribution.
Full Transcript
# BUS 2000BEF Integrated Business Foundation ## Module 9 - Decision Making Skills ### Sampling Distribution - **Topics** - Sampling distribution - Sampling distribution of the mean - Sampling distribution of the proportion - Sampling distribution of the difference between two means...
# BUS 2000BEF Integrated Business Foundation ## Module 9 - Decision Making Skills ### Sampling Distribution - **Topics** - Sampling distribution - Sampling distribution of the mean - Sampling distribution of the proportion - Sampling distribution of the difference between two means - **Sampling Distribution** - Theoretical probability distribution of a sample statistic. - Sample statistic is a random variable, such as the sample mean, sample proportion, etc. - The sampling distribution is calculated using the theory of drawing all possible samples of the same size from a population. - **Why Study Sampling Distributions** - Sample statistics are used to estimate population parameters. - Different samples provide different estimations. - The study of sampling distributions can help us answer the following questions: - How good (accurate) is the estimate provided by a sample? We know that larger samples provide better estimates, but are they cost effective? - How large a sample (n) is enough to get a representative result? To answer these questions, we need to have a better understanding of the theoretical basis of sampling - the **Sampling Distribution**. ### Sampling Distribution of the Mean **Overview** - When performing statistical inference, we draw a sample from the population to obtain the sample mean (X̄) and use it to estimate the population mean (μ). - The value of X varies among different samples. - This means that X̄, the sample mean, is a random variable. Its probability distribution is called the **sampling distribution of the mean**. **Calculations** - The sampling distribution of the mean can be calculated by drawing all possible distinct samples from the population. - If there are *m* distinct samples that can be drawn, the possible values of the random variable X̄ are X1, X2, X3,..., Xm and they form a population of sample mean values. - The population mean (μx̄) of this distribution is calculated by adding all the sample means and dividing by the number of samples *(m)*. - The standard deviation (σx̄) of this distribution is calculated by taking the square root of the variance (σ²x̄). -**An Example** - Let's imagine a population of 4 individuals, with ages: 18, 20, 22, 24 (years old). - Population mean (μ): 21 - Population standard deviation (σ): 2.236 In this example, we can calculate the sampling distribution of the mean by drawing all possible samples of size 2 (with replacement) from the population. This will result in 16 different possible samples. For each of these samples, we can calculate the mean. The probability distribution of these sample means is the sampling distribution of the mean. - **Developing Sampling Distributions** The sampling distribution of the mean for the example above demonstrates the following: - The mean of the sampling distribution (μx̄) is equal to the population mean (μ). - The standard error (σx̄), which is the standard deviation of the sampling distribution of the mean, is less than the population standard deviation (σ). This is because the sampling distribution is more concentrated around the population mean, hence the standard error is much smaller than the population standard deviation. ### **Comparing the Population with its Sampling Distribution** - The sampling distribution tends to follow a symmetric bell-shaped distribution. - The population mean equals the mean of the sample mean: μx̄ = μ - The distribution of the sample mean is more packed together than the distribution of the population, it has a smaller variance or standard deviation. - The standard error decreases with larger sample sizes. ### Sampling Distribution of the Mean: When the Population is Normal - **Central Tendency** - μx̄ = μ - **Variation** - σx̄ = σ/√n - The larger the sample size, the more closely the sampling distribution resembles a normal distribution. ### Sampling Distribution of the Mean: When the Population is Not Normal - **Central Tendency** - μx̄ = μ - **Variation** - σx̄ = σ/√n - The distribution of the sample mean will still be approximately normal for larger sample sizes. ### **Central Limit Theorem** - The sampling distribution of the sample mean approaches a normal distribution, regardless of the shape of the population distribution, as the sample size gets larger. - As n increases, the sampling distribution of the mean becomes more and more normal, regardless of the underlying population distribution. ### **How large is “large enough"?** - The larger the sample size, the more nearly normally distributed is the population of all possible sample means. - When the sample size is at least 30, the sampling distribution of the mean will be approximately normal. **Example 1:** - Assume variable X follows a normal distribution with a mean of 8 and a variance of 4. - A sample of size 25 is taken from this population. - The mean of the sampling distribution of the mean (μx̄) is 8. - The standard error (σx̄) = 0.4 (square root of (4/25)). - The sampling distribution of the mean is X ~N(8, 4/25). **Exercise 1:** - It is known that the monthly salary of graphic designers graduated last year is normally distributed with a mean of $15,500 and a standard deviation of $2,000. - The probability can be calculated for a variety of scenarios: - What is the probability that the monthly salary of a randomly selected graphic designer graduated last year exceeds $16,200? - If 5 graphic designers graduated last year are randomly selected, find the probability that the average of monthly salary exceeds $16,200. - If 64 graphic designers graduated last year are randomly selected, find the probability that the average of monthly salary exceeds $16,200. - If the distribution about the monthly salary of graphic designers is not known, which, if any, of the questions above can you answer? Explain. **Exercise 1 (Answer):** - Let X be monthly salary of graphic designers graduated last year, X ~N(15500, 2000²) - The probability of the monthly salary of a randomly selected graphic designer graduated last year exceeding $16,200 is 0.3632. - The probability that the average of monthly salary of 5 randomly selected graphic designers exceeds $16,200 is 0.2177. - The probability that the average of monthly salary of 64 randomly selected graphic designers exceeds $16,200 is 0.0026. - Only the third question can be answered in this case as n is large - we can use the Central Limit Theorem to assume that X is normally distributed. ### Population Proportions (p) - It represents the proportion of the population that possesses a particular characteristic of interest. - For example, in a population of size N, if the number of males is given as x, the proportion of males is denoted by p and is given by: p = x/N ### Sample Proportion (p) - The sample proportion (p) provides an estimate of the population proportion. - It is calculated by dividing the number of successes (x) in a sample by the sample size (n). - The mean (μp̂) of the sampling distribution of the sample proportion is equal to the population proportion. - The standard error (σp̂) of the sampling distribution of the sample proportion is - σp̂ = √(p(1-p)/n) ### Sampling Distribution of the Sample Proportion - The sampling distribution of the sample proportion can be approximated by a normal distribution when: - np ≥ 5 - n(1-p) ≥ 5 - **Mean:** μp̂ = p - **Standard Error:** σp̂ = √(p(1-p)/n) ### Standardizing Sampling Distribution of the Sample Proportion - The standardized value (Z) for the sampling distribution of the sample proportion is: Z = (p-μp̂) / σp̂ = (p̂ -p) / √(p(1-p)/n) ### Exercise 2 - A random sample of 50 university students was selected for a survey. The key question asked was: "Did you make in-app purchase on mobile game last month?" Assume that the true proportion is 0.4. - Determine the standard error of the proportion of university students who made in-app purchase on a mobile game last month. - Find the probability that the sample proportion of university students who made in-app purchase on a mobile game last month lies between 26% and 36%. - Find the probability that more than half of the sampled students made in-app purchase on a mobile game last month. ### Exercise 2 (Answer) - Let p̂ be the sample proportion of university students who made in-app purchase last month. - The standard error of the proportion is .0693. - The probability is 0.2593 that the sample proportion of university students who made in-app purchase on a mobile game last month lies between 26% and 36%. - The probability is 0.0749 that more than half of the sampled students made in-app purchase on a mobile game last month. ### Sampling Distribution of the Difference Between Two Sample Means - There is often a need to compare the means between two independent populations by studying the difference between two relevant sample means. - Two samples are said to be independent if the selection of items in one sample has no effect on the selection of items in the other sample. - The mean of the sampling distribution of the difference between two sample means is: - μ(X̄ - Ȳ ) = μx - μy - The variance of the sampling distribution of the difference between two sample means is: - σ²(X̄ - Ȳ ) = σ²x / nx + σ²y / ny. - If X and Y are normally distributed, then X - Y will also be normally distributed with the mean and variance as stated. - The standardized variable Z is: Z = (X - Y) - (μx-μy) / √(σ²x/nx + σ²y/ny) - The sampling distribution of the difference between two sample means follows a standard normal distribution. This document will be a valuable resource for understanding and applying sampling distributions. It will enhance your ability to apply these concepts in practical situations.