Statistics for Business Analytics & Data Science PDF
Document Details
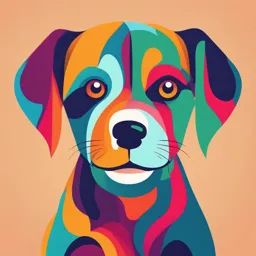
Uploaded by WinningSodalite2051
Zarqa University
Dr. Mais Haj Qasem
Tags
Summary
This document is a chapter on statistics for business analytics and data science. It covers topics like the Central Limit Theorem, sampling distributions, and Z-scores. The chapter is aimed at an undergraduate level and provides examples and definitions of statistical concepts.
Full Transcript
Statistics for Business Analytics & Data Science Part -2 Prepared by: Dr. Mais Haj Qasem Outline What we will learn : 1. Population and Samples 2. Sampling Distribution 3. Central Limit Theorem 4. Central Limit Theorem - Visualiz...
Statistics for Business Analytics & Data Science Part -2 Prepared by: Dr. Mais Haj Qasem Outline What we will learn : 1. Population and Samples 2. Sampling Distribution 3. Central Limit Theorem 4. Central Limit Theorem - Visualization 5. Z-Score 6. Hands-On CLT: An Analytics Challenge Prepared by: Dr. Mais Haj Qasem Population and Samples Population: In general, population refers to the number of individuals living in a specific location at a given moment. However, the population in statistics refers to data on what you've chosen to study. A population in research does not always relate to people. It may mean a collection of any kind of subject you like to study, including objects, events, groups, countries, animals, plants, and so on. ❖ Example : if you want a statistics about school, the population will be number of student and teacher in school. ❖ Important parameter for any population: ▪ 𝑁 = number of observations or the total number of the population; it can be age, height, or weight for a person, and it can be for some object or even balance. ▪ 𝜇 = Mean ▪ 𝜎 = Standard Deviation Prepared by: Dr. Mais Haj Qasem Samples: A part of a larger population that has characteristics from the original population. When the population is too big for all of its subjects or observations to be included in the test, a sample is used in statistical testing. ❖ Samples have statistics, not parameters like population: ▪ 𝑛 = number of observations that take form the sample ▪ 𝑥ҧ = Mean ▪ 𝑠 = Standard Deviation Sometimes, you can gather data from a subset of your population and use it as the general standard in order to overcome the limitations of a population. Reliability of the data is increased by gathering the subset data from the study participant groups. It is possible to extend the study's results for the different participant populations to the general population. Prepared by: Dr. Mais Haj Qasem Sampling Distribution Data helps academics, researchers, marketers, analysts, statisticians, and researchers to draw significant conclusions about particular subjects and information. It may help governments in planning for services that a particular population needs or it can help businesses in making future decisions and improving their performance. Instead of dealing with populations, many of the data that are gathered and used are samples. Subsets of populations are called samples. A sample, in its simplest shape, is a subset of a larger group. For that reason, this smaller segment was created to be representative of the entire population. The probability distribution of a statistic obtained by selecting random samples from a particular population is known as a sampling distribution. In sampling distribution, we take a huge number of samples that will be around 25% or less of the population, it could be 10%. Prepared by: Dr. Mais Haj Qasem Sampling Distribution How Does It Work? ❖ Choose particular sizes of random samples from the population. ❖ calculate the mean for each sample. In general, sampling distribution is the process of taking many samples from the population to prepare for the Central Limit Theorem. Prepared by: Dr. Mais Haj Qasem Central Limit Theorem The Central Limit Theorem: is based on the idea of a sampling distribution, which is the statistical probability distribution for a large number of samples drawn from a population. ❖ For example, Let us consider that class X consists of 15 sections, with 50 students in each section. ▪ We have to figure out what class X student's average grades are. ▪ The standard approach will be to simply compute the average. ▪ But what happens if there is a lot of data? Is this a good approach? No way, figuring out each student's grade will be a difficult and time-consuming procedure. What then are the options? The solution will be to use the Central Limit Theorem. Prepared by: Dr. Mais Haj Qasem The Central Limit Theorem has two main Theorems: 1. The First Theorem in Central Limit Theorem: Any population via sampling distribution should end with a normal distribution. When the sample size increases, wherever the final distribution for sampling is closer to the normal distribution, until we reach a certain point, the distribution will be exactly the same as the normal distribution. ❖ For example, if we have an x and y axis graph below, and whenever we take a sample from the population and calculate their mean, we drop this value into the graph, that will take us to a normal distribution over time. ഥഥ 𝒙 ഥ 𝒙 𝒙 𝒙 ഥ 𝒙ഥ ഥ 𝒙 𝒙 ഥ 𝒙 ഥ 𝒙 ഥ ഥ 𝒙 ഥ 𝒙 ഥ 𝒙 𝒙ഥ ഥ Y 𝒙 ഥ 𝒙 𝒙 ഥ 𝒙 ഥ 𝒙 ഥ ഥ ഥ 𝒙 ഥ𝒙ഥ 𝒙 ഥ 𝒙 𝒙 ഥ 𝒙 𝒙 ഥ X Prepared by: Dr. Mais Haj Qasem 2. The Second Theorem in Central Limit Theorem: According to the central limit theorem, even in cases where a population isn't normally distributed, the means of samples taken from it will follow a normal distribution if the samples are large enough. ❖ For example, If we take 10,000 samples from the population, each with a sample size of 50, the sample means follow a normal distribution, as predicted by the central limit theorem in the right image. Even the population in the left image does not follow a normal distribution. Prepared by: Dr. Mais Haj Qasem The idea for transforming the population or random distribution into a normal distribution is that a normal distribution is the most important distribution in the world and reflects many theorems because each population will end up with a normal distribution. Research in many fields with different cases has found that it ends up with a normal distribution, and this is the normal distribution for any experiment in the world. ❖ For example, If we take a book with 500 pages and every page in this book is considered a sample, I need to know the average size of the word size for each sample. I have words that contain 1 letter, and others have 50 letters in the word. If I calculate the average size of letters for words on each page, I will have the 𝒙 ഥ , and if I sum 500 samples, it will end up with a normal distribution eventually. Prepared by: Dr. Mais Haj Qasem 3. The Third Theorem in Central Limit Theorem: The shape of the sampling distribution may be determined without actually sampling the population multiple times. The properties of the population dictate the parameters of the mean sampling distribution: ❖ The mean of the sampling distribution is the mean of the population. 𝑚𝑥= ҧ 𝑚 , where 𝑚 is the mean of whole population, and 𝑚𝑥ҧ is the average mean of all samples ❖ The standard deviation of the sampling distribution is the standard deviation of the population divided by the square root of the sample size. 𝜎 𝑥= ҧ 𝑛 , where 𝜎 is the standard deviation of whole population, 𝜎𝑥ҧ is the average mean of all samples, and 𝑛 is the number of point in each samples Prepared by: Dr. Mais Haj Qasem Sample size and the Central Limit Theorem : The number of observations taken from the population for each sample is known as the sample size (n). Every sample has the same sample size. There are two ways that the sample size affects the sampling distribution of the mean. 1. Sample size and normality: The sampling distribution will match a normal distribution more closely the larger the sample size. When the sample size is small in the sampling distribution, the mean will be non-normal. The central limit theorem only applies in cases where the sample size is sufficiently large. 2. Sample size and standard deviations: The sampling distribution's standard deviation is affected by the sample size. The standard deviation is used as an indicator for the distribution's variability, or how wide or narrow it is. ❖ When 𝑛 is low, the standard deviation will be high, which is because there’s a lot of spread in the samples’ means and it will not estimate the population’s mean precisely. ❖ When 𝑛 is high, the standard deviation will be low because there’s not much spread in the samples’ means, and it will estimate the population’s mean precisely. Prepared by: Dr. Mais Haj Qasem Central Limit Theorem Examples: Let's say that you're interested in the average age at which Americans quit their jobs. All of the people in the population are Americans who have stopped working and their distribution could look like this: The distribution of retirement age is left-skewed. At 65 years old, which is the average retirement age, most people retire in five years or less. A "long tail" of people, on the other hand, retire considerably earlier—at 50 or even 40 years old. The standard deviation of the population is 6 years. Prepared by: Dr. Mais Haj Qasem Assume you select just a small sample of the general population. You ask five retirees, chosen at random when they retired. sample of n = 5 68, 73,70, 62,63 𝟔𝟖 + 𝟕𝟑 + 𝟕𝟎 + 𝟔𝟐 + 𝟔𝟑 𝑴𝒆𝒂𝒏 = = 𝟔𝟕. 𝟐 𝒚𝒆𝒂𝒓𝒔 𝟓 Let's say you repeat this process 10 times, collecting samples from five retirees each time, and figuring out the average for each sample. This is the mean sampling distribution : 60.8, 57.8, 62.2, 68.6, 67.4, 67.8, 68.3, 65.6, 66.5, 62.1 Prepared by: Dr. Mais Haj Qasem A histogram of the sample means it will look like this if the process is repeated multiple times: Although this sampling distribution is more normally distributed than the population, it still has a bit of a left skew. Prepared by: Dr. Mais Haj Qasem Central Limit Theorem - Visualization You can try the Central Limit Theorem by visualization to see the importance and effect of sampling distribution online at the following link: https://www.onlinestatbook.com/stat_sim/sampling_dist/index.html Prepared by: Dr. Mais Haj Qasem Z-Score Z- Score : The relationship between a value and the mean of a set of values is described statistically by the Z-score. Standard deviations from the mean are a measure of measurement for Z-score. The Z-score of means that the mean score and the data point's scores are the same. One standard deviation from the mean would be indicated by a Z- score of 1.0. Z-scores can be positive or negative, where a positive number means the score is higher than the mean and a negative value means it is lower. You can plot a z-score on a normal distribution curve. Z-scores range from -3 standard deviations (left of the normal distribution curve) up to +3 standard deviations (right of the normal distribution curve). You require knowledge of the population standard deviation (𝜎) and mean (𝜇) in order to use a z-score. Prepared by: Dr. Mais Haj Qasem Approximately 68% of the elements in a given set with a large number have a z-score between -1 and 1; approximately 95% have a z-score between -2 and 2; and approximately 99% have a z-score between -3 and 3. This is seen in the image below and is known as the Empirical Rule or the 68-95-99.7 Rule. Prepared by: Dr. Mais Haj Qasem Z-score Calculate outliers for the dataset that we work on, where there is a single data point that goes far outside the average value of a group of statistics. Outliers may be exceptions that stand outside individual samples of populations as well. The dataset that contains the outliers is first gathered, and its mean and standard deviation is then calculated. These numbers will be used to determine each data point's z- score value. We'll figure out the z-score cutoff value, which will indicate whether a data point can be classified as an outlier. As a hyperparameter, this cutoff value indicates which data point we do not require for our project. We choose it based on our needs. A data point does not fall inside the dataset's 99.73 % point if its z-score value is larger than 3. An outlier is any data point whose z-score exceeds the cutoff value that we have determined. Later, we will learn how to use the Z-score to normalize the value in the dataset in the data- preprocessing stage. Prepared by: Dr. Mais Haj Qasem The following formula is used to determine the statistical formula for a value's z-score: 𝒛 = (𝒙 − 𝝁)/𝝈 Where: 𝒙 − 𝝁 ❖ 𝒛 = Z-score ❖ 𝒙 = value being evaluated ❖ 𝝁 = mean ❖ 𝝈 = standard deviation X To determine if the provided Z-Score is positive or negative, use the corresponding positive positive Z-Table negative Z-Table or negative Z-Table that may be found online. This is link for positive Z-Table or negative Z- negative Z-Table Table. Prepared by: Dr. Mais Haj Qasem Examples-1 : You take the GATE examination and score 500. The mean score for the GATE is 390 and the standard deviation is 45. How well did you score on the test compared to the average test taker? ❖ 𝒙 =500 ❖ 𝝁 = 390 ❖ 𝝈 = 45 𝒙 − 𝝁 𝟓𝟎𝟎 − 𝟑𝟗𝟎 𝒛 = = = 𝟐. 𝟒𝟒 𝝈 𝟒𝟓 This means that your z-score is 2.44. Since the Z-Score is positive 2.44. Prepared by: Dr. Mais Haj Qasem We will make use of the positive Z-Table. As a result, you will get the final value which is 0.99266. Now, we need to compare how our original score of 500 on the GATE examination compares to the average score of the batch. To do that we need to convert the Z-Score into a percentage value. 𝟎. 𝟗𝟗𝟐𝟔𝟔 ∗ 𝟏𝟎𝟎 = 𝟗𝟗. 𝟐𝟔𝟔% Finally, you can say that you have performed well than almost 99% of other test-takers. Prepared by: Dr. Mais Haj Qasem Examples-2 : What is the probability that a student scores between 350 and 400 if the mean and standard deviation equal: ❖ 𝝁 = 390 ❖ 𝝈 = 45 Min Score = 𝑥1 = 350 Max Score = 𝑥1 = 400 𝒙𝟏 − 𝝁 𝟑𝟓𝟎 − 𝟑𝟗𝟎 𝒛𝒙𝟏 = = = −𝟎. 𝟖𝟖 𝝈 𝟒𝟓 𝒙𝟐 − 𝝁 𝟒𝟎𝟎 − 𝟑𝟗𝟎 𝒛𝟐 = = = 𝟎. 𝟐𝟐 𝝈 𝟒𝟓 Prepared by: Dr. Mais Haj Qasem negative Z-Table Since 𝑧1 is negative, we will have to look at a negative Z-Table and find that 𝑝1 , the first probability, is 0.18943. positive Z-Table 𝑧2 is positive, so we use a positive Z-Table which yields a probability 𝑝2 of 0.58706. The final probability is computed by subtracting 𝑝1 from 𝑝2 : 𝑝 = 𝑝2 − 𝑝1 𝑝 = 0.58706 – 0.18943 = 0.39763 The probability that a student scores between 350 and 400 is 39.763% (0.39763 * 100). Prepared by: Dr. Mais Haj Qasem Analytics Challenge You are a Business Analyst working for Aramex. 1. A business client has requested a large freight to be transported urgently from Amman to Cairo. When asked about the weight of the cargo they could not supply the exact weight. However, they have specified that there are a total of 36 boxes. 2. From prior experience with this client, you know that this type of cargo follows a distribution with a mean = 72 lb. (32.66 kg) and std.dev of 3 lb. (1.36 kg). The only plane Amazon currently have at Denver is a Cessna 208B Grand Caravan with a max cargo weight of 2,630 lb. (1,193 kg) Based on this information what is the probability that all of the cargo can be safely loaded onto the plane and transported? (This is our Target ) Prepared by: Dr. Mais Haj Qasem historical data we have for the clients because we dealt with them before Sample = 36 boxes, but I cannot depend on this to know if the cargo will be safely delivered, so the optimal solution is to work backwards. We know that for any population in the world, if we use the Central Limit Theorem (sampling distribution), it will end up with a normal distribution. Prepared by: Dr. Mais Haj Qasem The goal will be to transfer the original distribution to a normal distribution. Original Distribution 𝝁𝑥ҧ = 𝝁= 72 𝜎 3 𝑥ҧ = = = 0.5 37 6 𝝁 𝑃𝑙𝑎𝑛 𝑐𝑎𝑝𝑎𝑐𝑖𝑡𝑦 = 2,630 Original Distribution , n=36 Here we know the maximum weight for the plan, so we can calculate from the maximum number of boxes 𝑥𝑐𝑟𝑖𝑡 for 𝝈𝒙ഥ weight. 2,630 𝝁𝑥ҧ 𝒙𝒄𝒓𝒊𝒕 𝑥𝑐𝑟𝑖𝑡 = = 73.06 𝑙𝑏/𝑏𝑜𝑥 36 𝑏𝑜𝑥𝑒𝑠 Prepared by: Dr. Mais Haj Qasem Original Distribution 2,630 𝑥𝑐𝑟𝑖𝑡 = = 73.06 𝑙𝑏/𝑏𝑜𝑥 36 𝑏𝑜𝑥𝑒𝑠 𝑥𝑐𝑟𝑖𝑡 − 𝝁𝑥ҧ 73.06 − 72 𝑧= = = 2.12 𝑥ҧ 0.5 𝝁 𝑝 (𝑥 < 𝑥𝑐𝑟𝑖𝑡 ) = 0.9830 = 98.3% Original Distribution , n=36 Here, the probability that the plan goes from Amman to 𝝈𝒙ഥ Cario with 36 boxes without risk is 98.3%, which is the best probability, and the final decision will depend on the manager, but from the data science perspective, it says that the confidence level should be more than 97%, which is very 𝝁𝑥ҧ 𝒙𝒄𝒓𝒊𝒕 good in that case. Prepared by: Dr. Mais Haj Qasem