Sampling Techniques PDF
Document Details
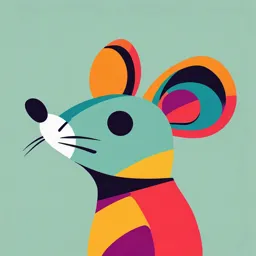
Uploaded by ReliableHarmonica
Saint Louis University
Tags
Summary
This document discusses sampling techniques and criteria for determining sample size in research. It covers concepts like level of precision, confidence levels, and variability. The document also provides examples and formulas for calculating sample sizes for proportions and means.
Full Transcript
MODULE 3: SAMPLING AND DATA COLLECTION UNIT 1: SAMPLING TECHNIQUES Learning Outcome: Distinguish between various sampling techniques and identify how to sample populations correctly using acce...
MODULE 3: SAMPLING AND DATA COLLECTION UNIT 1: SAMPLING TECHNIQUES Learning Outcome: Distinguish between various sampling techniques and identify how to sample populations correctly using acceptable statistical processes to further reliability of research and minimize bias. After going through this unit, you should be familiar with the different techniques on how to determine subjects or respondents with the objective of minimizing bias and guarantee reliability of data in a given research undertaking. SAMPLING Sampling is the process of selecting units, like people, organizations, or objects from a population of interest in order to study and fairly generalize the results back to the population from which the sample was taken.A sample should have the same characteristics as the population it is representing. The purpose of sampling is to make reliable estimates about a population. It is usually impossible, and too expensive, to sample the whole population so that when a sampling experiment is developed it should as closely as possible parallel the population conditions. Sample Size Criteria Usually, there are three criteria that need to be specified to determine the appropriate sample size: the level of precision, the level of confidence or risk, and the degree of variability in the attributes being measured (Miaoulis and Michener, 1976). Level of Precision – it is also referred to as the sampling error or margin of error. It is a range of values in which the true value of the population parameter is estimated to be (Israel, 1992). The level of precision if often expressed in percentage points (e.g. ± 5%).Hence, if a researcher finds that 75% of accountants in the sample have adopted a recommended accounting system with a precision rate of ± 5%, then it can be concluded that between 70% and 80% of accountants in the population have adopted the said system. In educational and social researches, the generally acceptable margin of error is 5% (or 0.05) for categorical data and 3% (0.03) for continuous data (Krejcie and Morgan, 1970 as quoted in Bartlett et al., 2001). Property of and for the exclusive use of SLU. Reproduction, storing in a retrieval system, distributing, uploading or posting online, or transmitting in any form or by any means, electronic, mechanical, photocopying, recording, or otherwise of any part of this document, without the prior written permission of SLU, is strictly prohibited.12 Confidence Level – This is also called risk level. The key idea is encompassed in what is called the Central Limit Theorem (CLT) where when a population is repeatedly sampled, the average value of the attribute obtained by those samples is equal to the true population value (Israel, 1992). Commonly used values for the confidence level are 95% and 99%. If a 95% confidence level is used, it implies that 95 out of 100 samples will have the true population value within the range of precision specified. There is always a chance that the sample you obtain does not represent the true population value. Degree of Variability - It refers to the distribution of attributes in the population. According to Israel (1992), the more heterogeneous (or spread out) the population is, the larger the sample size required in order to achieve a given level of precision. The more homogeneous (or less spread out) the population is, the smaller the sample size. Determining the Sample Size Several formulas to calculate the sample size for a certain study has been developed and suggested such as Parten’s formula (1950), Lehr’s rule (1992), the formula by Berlowitz, Watson’s formula (2001), and Cochran’s formula (1963) with most of these formulas considering the case where the population distribution is approximately normal. The following are some formulas that are used to calculate the size of a sample: Calculating the Size of a Sample for Proportions When the variable of interest in your study is a categorical variable, we use the following formulas in computing for the sample size. For populations that are large, Cochran (1963) developed the following equation to yield a representative sample for proportions. 2 0 = 2 where 0 is the sample size, is the abscissa of the normal curve that cuts off an area at the tails (1 − equals the desired confidence level), is the desired level of precision, is the estimated proportion of items in the population that exhibits the attribute of interest, and is the estimated proportion of items in the population that does not exhibit the attribute of interest, determined by = 1 −. Here are values of for commonly used confidence levels, as taken from the statistical table of Areas under the Normal Curve. Property of and for the exclusive use of SLU. Reproduction, storing in a retrieval system, distributing, uploading or posting online, or transmitting in any form or by any means, electronic, mechanical, photocopying, recording, or otherwise of any part of this document, without the prior written permission of SLU, is strictly prohibited.13 Confidence Level Z value 90% 1.645 95% 1.96 99% 2.576 The values and are taken from previous surveys or from similar studies. Now, when these values are not known, indicating that we do not know about the variability in the proportion, we assume both to be equal to 0.5. Assuming and to be equal to 0.5 provides us with the maximum variability in the proportion and hence, a conservative estimate of the sample size. Example 1: As an illustration, suppose you wish to evaluate the implementation of a new accounting system in which accountants are encouraged to adopt the system. Suppose the population of accountants is large and we have no information as to the variability in the proportion of accountants that will adopt the new system. With this, we assume = 0.5 and we desire to use a 95% confidence level and ± 3% precision. For a 95% confidence level, the corresponding value is 1.645. The required sample size would be 2 1.96 2 0.5 (0.5) 0 = = = 1067.111 ≈ 1068 2 (0.03)2 For this case, you would need a sample of 1,068 accountants. An important rule for you to take note of is this: when the calculated sample size is not a whole number, it should always be rounded up to the next higher whole number. When the sample size is rounded- off to the nearest whole number, then you exceed the maximum margin of error (level of precision) of your estimate in some cases. Finite Population Correction for Proportions If the population is small, then we can slightly reduce the sample size. The formula for the sample size is given by 0 = −1 1 + 0 where is the sample size while is the population size. Property of and for the exclusive use of SLU. Reproduction, storing in a retrieval system, distributing, uploading or posting online, or transmitting in any form or by any means, electronic, mechanical, photocopying, recording, or otherwise of any part of this document, without the prior written permission of SLU, is strictly prohibited.14 Example 2: Suppose that in the previous example, there is information that only 3,000 accountants were affected by the implementation of a new accounting system. The necessary sample size for the study, based on the information about the affected population, will now be: 0 1068 = 0 −1 = 1068−1 = 787.80 ≈ 789. 1+ 1+ 3000 This finite population correction is seen to substantially reduce the needed sample size for small populations. The Yamane’s formula This is a simplified formula for computing sample sizes for proportions by Yamane (1967). Yamane’s formula assumes a 95% confidence level and a value of 0.5: = 1 + 2 where is the sample size, is the population size, and is the level of precision. Example 3: Now, applying this formula to our preceding example, we have the sample size required as 2000 = = = 714.29 ≈ 715 1 + 2 1 + (2000)(0.032 ) Thus, the sample size will be 715. Calculating the Size of a Sample for the Mean The preceding formulas that we have seen used to compute for sample sizes for proportions consider variables that assume a dichotomous response for the attribute of interest (e.g. gender – male or female; agreement – yes or no, and the like). How about if the variable assumes a polytomous response or if the variable is quantitative? When the variable of interest is polytomous or is quantitative, one way of computing for the sample size is to combine the responses into two categories and use the formula for proportions. The other way, which applies to quantitative variables only, is to use the formula for the sample size for the mean which is given by Property of and for the exclusive use of SLU. Reproduction, storing in a retrieval system, distributing, uploading or posting online, or transmitting in any form or by any means, electronic, mechanical, photocopying, recording, or otherwise of any part of this document, without the prior written permission of SLU, is strictly prohibited.15 2 2 = 2 where 0 is the sample size, is the abscissa of the normal curve that cuts off an area at the tails, and is the desired level of precision (in the same unit of measure as the variance) and 2 is the variance of the attribute in the population. A good estimate of the population variance is necessary and, oftentimes, this estimate is not available which makes it a disadvantage. This is the reason why according to Israel (1992), the sample size for the proportion is frequently preferred. Other Strategies Using a census for small populations. Use the entire population as the sample. This would eliminate sampling error and it would provide data for all items or individuals in the population. Use the sample size of a similar study. Reviewing these studies in your discipline or area of research can provide guidance as to the typical sample sizes which are used. SAMPLING TECHNIQUES There are two types of samples: Random Samples. A random sample is a sample drawn in such a way that each member of the population has some chance of being selected in the sample. Non-random Samples. In a non-random sample, some members of the population may not have any chance of being selected in the sample. Nonrandom sampling uses some criteria for choosing the sample. The results obtained from a sample survey may contain two types of errors: Sampling errors: The sampling error is also called the chance error. The sampling error is the difference between the result obtained from a sample survey and the result that would have been obtained if the whole population had been included in the survey. The actual process of sampling causes sampling errors. The sampling error occurs because of chance, and it cannot be avoided. A sampling error can occur only in a sample survey. Non-sampling errors. Non-sampling errors are also called the systematic errors. Factors not related to the sampling process cause non-sampling errors. These are errors that occur in the collection, recording, and tabulation of data. Non-sampling errors occur Property of and for the exclusive use of SLU. Reproduction, storing in a retrieval system, distributing, uploading or posting online, or transmitting in any form or by any means, electronic, mechanical, photocopying, recording, or otherwise of any part of this document, without the prior written permission of SLU, is strictly prohibited.16 because of human mistakes and not chance. Non-sampling errors can be minimized if questions are prepared carefully and data are handled cautiously. Many types of systematic errors or biases can occur in a survey, including selection error, non-response error, response error, and voluntary response error. The following chart shows the types of errors. A defective counting device can cause a non-sampling error. The list of members of the target population that is used to select a sample is called the sampling frame. The error that occurs because the sampling frame is not representative of the population is called the selection error. Even if our sampling frame and, consequently, the sample are representative of the population, nonresponse error may occur because many of the people included in the sample did not respond to the survey. The response error occurs when the answer given by a person included in the survey is not correct. This may happen for many reasons. One reason is that the respondent may not have understood the question. It has been observed that when the same question is worded differently, many people do not respond the same way. Usually such an error on the part of respondents is not intentional. Voluntary response error occurs when a survey is not conducted on a randomly selected sample but a questionnaire is published in a magazine or newspaper and people are invited to respond to that questionnaire. RANDOM SAMPLING TECHNIQUES Members from the population are selected in such a way that each individual member in the population has an equal chance of being selected. Property of and for the exclusive use of SLU. Reproduction, storing in a retrieval system, distributing, uploading or posting online, or transmitting in any form or by any means, electronic, mechanical, photocopying, recording, or otherwise of any part of this document, without the prior written permission of SLU, is strictly prohibited.17 Simple Random Sampling (SRS) – the most basic and well-known type of random sampling technique. Every case in the population being sampled has an equal chance of being chosen. It is an equal probability sampling method (EPSEM). EPSEMs are important because they produce representative samples, i.e., it reflects or exhibits the characteristic of the population. Basic Steps: 1. Construct the sampling frame. Make a list of the population units and number them from 1 to , where is the population size. 2. Determine the sample size. 3. Select random numbers from 1 to using some random process. Employ any of the following selection procedure: Draw lots Lottery Usage of gadgets like the calculator or computer to generate Random Numbers Table of Random Numbers Systematic Sampling - consists of randomly selecting one unit and choosing additional elements at equal intervals until the desired sample size is achieved. Basic Steps: 1. Construct the sampling frame. 2. Determine the sample size. 3. Determine the sampling interval, : =. 4. Identify the random start, , using any of the selection procedures under SRS where 1 ≤ ≤. 5. The random start identifies the first sampling unit.Commencing on the random start, select every th item until the desired sample size is reached. Property of and for the exclusive use of SLU. Reproduction, storing in a retrieval system, distributing, uploading or posting online, or transmitting in any form or by any means, electronic, mechanical, photocopying, recording, or otherwise of any part of this document, without the prior written permission of SLU, is strictly prohibited.18 Stratified Random Sampling - this involves dividing the potential samples into two or more mutually exclusive groups based on strata/categories of interest in the research so that each population item belongs to only one stratum. The objective is to form strata such that the population values of interest within each stratum are as much alike as possible. The purpose is to organize the potential samples into homogenous subsets before sampling. Sample items are selected from each stratum using the simple random sampling method. For example, you could divide the potential samples based on gender, race or occupation. You then draw a random sample from each subset. Stratified random sampling is common because it ensures that each subgroup of the larger group is adequately represented in the sample. We use proportional allocation to ensure that the percentage of each strata in the population is the same in the resulting sample, using the following formula: ℎ ℎ = ( ) where: ℎ = sample size for each stratum ℎ = stratum size = population size = sample size Example 4: Suppose a school has five departments composed of the following number of students. Determine the number of students to be part of the sample when the researcher needs 363 respondents. Department ℎ ℎ Solution: 1500 Business Administration (BA) 1,500 140 nBA 363 139.62140 3900 1200 Management(M) 1,200 112 nM 363 111.69112 3900 Property of and for the exclusive use of SLU. Reproduction, storing in a retrieval system, distributing, uploading or posting online, or transmitting in any form or by any means, electronic, mechanical, photocopying, recording, or otherwise of any part of this document, without the prior written permission of SLU, is strictly prohibited.19 850 Finance(F) 850 80 nF 363 79.1280 3900 200 Entrepreneurship(E) 200 19 nE 363 18.6219 3900 1500 Culinary Arts(CA) 150 14 nCA 363 13.9614 3900 Total 3,900 Hence, 140 students from Business Administration, 112 students from Management, 80 students from Finance, 19 students from Entrepreneurship, and 14 students from Culinary Arts are part of the sample (we round up each value to the next higher integer). In this case, our sample will contain 365 students, which is greater than the original intended sample size of 363 (but that’s OK!). Cluster Random Sampling - In cluster random sampling, you randomly select clusters instead of individual samples in the first stage of sampling and include every unit from the cluster in the sample. For example, a cluster might be a school, a team or a village. This technique is used when no list of individual samples is available. Usually, the way this type of sampling is done is by starting at the higher level clusters and then sampling at subsequent levels until individual samples are reached. Multi-Stage Sampling - This method uses several stages or phases in getting random samples from the general population. It is commonly used if the research is of national scope. We divide the country into Regions Regions into Municipalities and Cities Municipalities and Cities into barangays Barangays into Sitios or sections Property of and for the exclusive use of SLU. Reproduction, storing in a retrieval system, distributing, uploading or posting online, or transmitting in any form or by any means, electronic, mechanical, photocopying, recording, or otherwise of any part of this document, without the prior written permission of SLU, is strictly prohibited.20 NON PROBABILITY SAMPLING TECHNIQUES These are sampling methods that do not involve random selection of samples. With non- probability samples, the population may or may not be represented well, and it will often be difficult to know how well the population has been represented. Accidental or Haphazard or Convenience Sampling - one of the most common methods of sampling where methods done are normally biased since the researcher considers his/her convenience in the collection of the data. Purposive Sampling - sampling is based on certain criteria laid down by the researcher. People who satisfy the criteria are interviewed. Subcategories of Purposive sampling Modal instance sampling When we do modal instance sampling, we are sampling the most frequent case. The problem with modal instance sampling is identifying the “modal” case. Modal instance sampling is only sensible for informal sampling contexts. Expert sampling Involves the assembling of a sample of persons with known or demonstrable experience and expertise in some area. Two reasons we might do expert sampling: 1. It would be the best way to elicit the views of persons who have specific expertise. 2. To provide evidence for the validity of another sampling approach you’ve chosen. Quota sampling Select items non-randomly according to some fixed quota. Snowball sampling Begin by identifying someone who meets the criteria for inclusion in your study. You then ask them to recommend others who they may know who also meet the criteria. Property of and for the exclusive use of SLU. Reproduction, storing in a retrieval system, distributing, uploading or posting online, or transmitting in any form or by any means, electronic, mechanical, photocopying, recording, or otherwise of any part of this document, without the prior written permission of SLU, is strictly prohibited.21 Learning Reinforcement Activity No. 3: SAMPLING TECHNIQUES Work on the following problems using short bond papers with 1-inch margin on all sides. You may not copy the problems. Your answers can be handwritten be computerized. If it is computerized, save it as a single PDF file; if it is handwritten, scan or take a photo/picture of each page, copy and paste the photo/picture to a WORD document and save it as a single PDF file (or you can use any means, like a mobile App, to scan and save it as a single PDF file). The Filename should be: For example, BALLENAJaime_Reinforcement3 will be my output for Learning Reinforcement 3. 1. Which of the following situations will result in probability or non-probability sampling? a. Population: All residents of Baguio City Sampling Technique: For a week the researcher stops and interviews every fourteenth person who passes by a particular spot at lower Session Road. b. Population: All students of a large public high school. Sampling Technique: Researcher selects the first 50 students who report to school on a Monday morning and gathers data from them. c. Population: All 100 guests/participants at a business convention. Sampling Technique: The name of each person is written on a slip of paper then all slips of paper are placed in a box, mixed, then drawn one after the other for the available ten door prizes. d. Population: Business owners with less than 20 employees. Sampling Technique: Researcher gets information from DTI on barangays with small businesses (less than 20 employees), randomly selects 5 barangays, and takes all small business from selected barangays for the study. e. Population: Online live sellers in Baguio City utilizing Facebook for selling. Sampling Technique: The researcher monitors Facebook Buy and Sell groups for a particular time duration in the evening. Through Facebook messenger, the researcher notes all the online sellers he/she chances upon who are currently “live” selling and interviews them right after they have been online. 2. In each of the following situations, a random sample must be obtained. Determine whether a cluster, stratified, or systematic random sampling would be appropriate. Explain in brief detail how the sampling is to be conducted. Do not discuss expected results or conclusions. Property of and for the exclusive use of SLU. Reproduction, storing in a retrieval system, distributing, uploading or posting online, or transmitting in any form or by any means, electronic, mechanical, photocopying, recording, or otherwise of any part of this document, without the prior written permission of SLU, is strictly prohibited.22 a. A large convenience store chain wishes to determine its customers’ level of satisfaction with regard to their service. b. A nationwide survey on charter change is to be conducted. (Note: there are seventeen regions in the Philippines.) c. An educational researcher wants to compare the difference in career goals between male and female students of Maryheights College having 10,000 students. d. A social researcher wants to determine whether accountants who work in corporate offices earn more than accountants who work in government offices. e. A market analyst would like to compare the durability, in terms of the mean time before wear, of two leading brands of car tires. Congratulations! You just completed Module 3 Unit 1. Let’s learn about data collection in the next unit. Property of and for the exclusive use of SLU. Reproduction, storing in a retrieval system, distributing, uploading or posting online, or transmitting in any form or by any means, electronic, mechanical, photocopying, recording, or otherwise of any part of this document, without the prior written permission of SLU, is strictly prohibited.23