Sampling Techniques & Sample Size Estimation PDF
Document Details
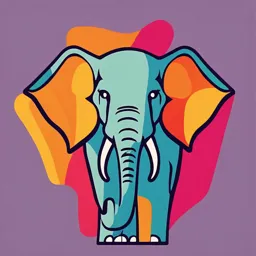
Uploaded by StrongTajMahal8964
Al-Arish University
Alshimaa Hosseny
Tags
Summary
This document covers various sampling techniques, including probability and non-probability methods, with a focus on how to determine an appropriate sample size for research studies. It also discusses the importance of sampling, statistical inference and common considerations. The document uses clear examples and diagrams.
Full Transcript
Sampling Techniques & Sample Size Estimation Alshimaa Hosseny Teaching Assistant Faculty of Medicine Arish University Objectives ▪ Introduction to sampling and definitions ▪ Why sampling is important ▪ Types of sampling methods ▪ Sampl...
Sampling Techniques & Sample Size Estimation Alshimaa Hosseny Teaching Assistant Faculty of Medicine Arish University Objectives ▪ Introduction to sampling and definitions ▪ Why sampling is important ▪ Types of sampling methods ▪ Sample size calculation Census A complete enumeration of all items in the population. ▪ In census - No chance - highest accuracy ▪ In practice - Slightest element of bias---become larger - To check the element of bias----resurvey. - A great deal of time, money and energy. Population: ▪ A set which includes all measurements of interest to the researcher ▪ (The collection of all responses, measurements, or counts that are of interest) Sample: ▪ A subset of the population A population could be: ▪ All individuals, families, groups, organizations, events, objects, or items from which samples are taken for measurement. ▪ For example, a population of presidents, professors, books or students, RBCs. Sampling: ▪ The process of selecting a group of items from a population. ▪ The process of sampling has 3 elements ▪ Selecting the sample ▪ Collecting information ▪ Making inference about population Statistical inference is a method of making decisions about the parameters of a population, based on sampling. Importance of sampling Get information about large populations ▪ Less costs (Economy) it is cheaper to observe a part rather than the whole population), ▪ Less field time ▪ More accuracy ▪ The large size of many populations, when it’s impossible to study the whole population Statistical Terms ▪ Target Population: The population to be studied/to which the investigator wants to generalize his results. ▪ Sampling frame List of all the sampling units from which sample is drawn ▪ Sampling Unit: The people or items that have been sampled. The target population is the collection of sampling units. ▪ Sampling fraction Ratio between sample size and population size Example: 100 out of 2000 (5%). ▪ Sample criteria Determine the inclusion and exclusion criteria. ▪ Sampling scheme Method of selecting sampling units from sampling frame. Steps of the sampling process 1. Identify the target population, 2. Identify the accessible population to be sampled (N) (S. frame), 3. Determine the size of the sample needed (n) and sample criteria, 4. Select the sampling technique, 5. Implement the plan. Sampling Sampling methods Sample size (How can you (How many) choose) Accuracy Representativeness It refers to how close the It refers to how sample’s statistic is to accurately this sample’s the true population’s members resemble the value it represents. members of the entire population it represents. Sampling methods ▪ Probability samples: Samples in which each members of the population have a known equal nonzero chance (probability) of being selected in the study ▪ Non-probability samples: Samples in which the chances (probability) of selecting members from the population are unknown probability samples ▪ Each subject has a known probability of being selected ▪ Allows generalization of results ▪ Probability samples are the best ▪ Ensure ✓ Representativeness ✓ Precision 1. Simple Random Sampling ▪ Procedure defining the population choosing the sample size; Need listing of all sampling units (“sampling frame”) Number all units Randomly draw units ▪ Methods Lottery method Table of random numbers Computer generated random numbers Simple Random Sampling Advantages: Disadvantages: Known and equal Require knowledge chance of selection of the complete Simple process and sampling frame easy Not great for diverse groups 2. Systematic sampling ▪ Procedure 1. Select a suitable sampling frame 2. Each element is assigned a number from 1 to N (pop. size) 3. Determine the sampling interval I=N/n. If k is a fraction, round to the nearest integer 4. Select a random number, s, between 1 and k, as explained in simple random sampling 5. The elements with the following numbers will comprise the systematic random sample: s, s+k,s+2k,s+3k,s+4k,...,s+(n-1)k. N = 600 and n = 200 sampling fraction = 600/ 200 = 3 List persons from 1 to 600 Randomly select a number between 1 and 3 (ex : 2) 1st person selected = the 2nd on the list 2nd person = 2 + 3 = the 5th etc..... 200th person = 2 + (200 – 1)*3 = 599th. Systematic sampling Advantages: Disadvantages: Known and Small loss in equal chance of sampling any element precision being selected Less expensive, fast 3. Stratified sampling ▪ Principle A two-step process in which the population is partitioned into subpopulations, or strata. Elements are selected from each stratum by a random procedure. ▪ Procedure 1. Identify and define the population. 2. Determine the desired sample size. 3. Identify the variable and subgroups (strata) for which you want to guarantee appropriate, equal representation. 4. Randomly select, number of individuals from each of the stratum, (fixed number or proportional number). Stratified sampling Advantages: Disadvantages: More accurate More complex, sampling plan requiring different sample sizes for each stratum 4. Cluster sampling Cluster: a group of sampling units close to each other i.e., crowding together in the same area or neighborhood. ▪ Principle The target population is first divided into subpopulations, or clusters. Then a random sample of clusters is selected. Elements within a cluster should be as heterogeneous as possible, but clusters themselves should be as homogeneous as possible. Ideally, each cluster should be a small-scale representation of the population. Cluster sampling ▪ Procedure Identify and define the population. Determine the desired sample size. Identify and define a logical cluster. List all clusters. Estimate the average number of population members per cluster. Determine the number of clusters needed by dividing the sample size by the estimated size of a cluster. Randomly select the needed number of clusters. Include in your study all population members in each selected cluster. Cluster sampling Advantages: Time- and cost-efficient, especially for samples that are widely geographically spread High external validity because your sample will reflect the characteristics of the larger population. Disadvantages: More complex to plan Cluster members are more likely to be alike 5. Multi-stage sampling ▪ Principle Sampling repeated at several levels. Nonprobability samples 1. Convenience Sampling ▪ Sample is selected from elements of a population that are easily accessible. ▪ Often, respondents are selected because they happen to be in the right place at the right time. ▪ Also known as accidantal Sampling (ease of access). 2. Judgmental Sampling (Purposive sampling) ▪ Refers to intentionally selecting participants based on their characteristics, knowledge, experiences, or some other criteria. ▪ Convenience sampling involves recruiting individuals primarily because they are available, willing, or easy to access or contact on a practical level. 3.Quota Sampling ▪ Divide population into different strata ( e.g male and female students). ▪ Proportion from each stratum but not random. 4. Snowball Sampling ▪ Referrals from the initial respondents (chain referral, friend to friend). ▪ Also known as Network sampling Nonprobability samples ▪ Advantages: ▪ Disadvantages: 1. Simple and easy 1. Highly vulnerable to to use selection bias 2. Helpful for pilot 2. Generalizability is studies and for unclear. hypothesis 3. High level of generation sampling error. 3. Short duration of time 4. Cost effectiveness Sampling errors Sampling error (random error) ▪ Random difference between sample and population from which sample drawn. ▪ Size of error can be measured in probability samples. ▪ Expressed as “standard error” of mean, proportion… ▪ Standard error (or precision) depends upon: - Size of the sample - Distribution of character of interest in population Systematic error (or bias) ▪ Inaccurate response (information bias) ▪ Selection bias Why Sample Size calculation? With Small S. Size, you can not be sure about your conclusion, or may Scientific reasons mistakenly decide wrong conclusion Undersized study can expose subjects to potentially harms without yielding a solid conclusion. Ethical reasons Oversized study expose unnecessarily large number of subjects to potentially harmful intervention Undersized study is a waste of resources due to its inability to yield Economic reasons useful results Oversized study may result in statistically significant result with doubtful clinical importance leading to waste of resources Factors determining the sample size Significance level (1 - Alpha level) ▪ p-value:The probability that the observed effect or phenomena is due to chance. ▪ Most studies set p-value at 0.05, accepting a chance of 5% of finding a difference not acctually present. ▪ The more stringent significance level (e.g., 1%) the larger required sample size Power (1 - Beta level) ▪ The capacity of the study to detect differences, effect or relationships that exist in the population. ▪ Most studies set power at 0.80, accepting 20% chance of missing a difference that is present. ▪ The greater the power, the larger required sample size Effect size ( difference to be detected) ▪ The minimal difference you consider as clinically significant & you wish to detect. ▪ ex: comparing to anti-hypertensive drugs A, B a difference of 5 mmHg (expected effect) will be clinically relevant. ▪ The smaller effect, the larger sample size will be required. Variability in the population ▪ It is measured by “Variance” ▪ The Larger variability, the larger sample size will be needed. Alternative Study Hypothesis ▪ One-tailed is easier to identify/prove (small required sample size). ▪ Two-tailed is more general and difficult to identify (larger sample size will be needed). Don’t forget to calculate the anticipated drop out and non-response % ? Where do we get the previous information? Previous published studies Pilot studies If information is lacking, there is no good way to calculate the sample size Tools for calculating sample size 1. Use of formulae (for each study design) 2. Nomograms 3. Ready made tables 4. Computer software Descriptive study sample size equation