Sampling Design & Data Collection PDF
Document Details
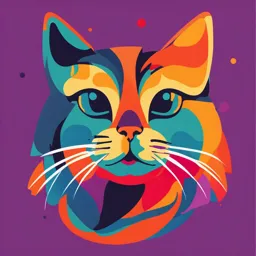
Uploaded by AvidMossAgate1502
Saint Joseph School, Addis Ababa
Tags
Summary
This document provides an overview of sampling design and data collection methods. It explains various sampling techniques, including probability and non-probability sampling. It also discusses the importance of sample size determination and different types of data collection methods, such as primary and secondary data collection.
Full Transcript
CHAPTER-4 Sampling Design& Data Collection The sampling is selecting a subset of individuals, events, or elements from a larger population to represent the whole. This process ensures that the research findings are generalizable and valid while saving time and resources. ...
CHAPTER-4 Sampling Design& Data Collection The sampling is selecting a subset of individuals, events, or elements from a larger population to represent the whole. This process ensures that the research findings are generalizable and valid while saving time and resources. The Sampling Process Plan procedure for selecting sampling units 4 Select Sampling Method: Probability or non-probability 5 3 Determine sample size Sampling method Select a Sampling Frame Select actual sampling units 6 2 Define the Target 7 Conduct experiments population 1 Defining the Target Population The entire group of individuals or elements relevant to the research problem. Example For a study on online banking users, the target population might be all users of online banking services in Ethiopia Determine the Sampling Frame A list or database that includes all elements of the target population from which the sample will be drawn. Example A list of registered users from a bank’s database. 5 Choose the Sampling Technique There are two basic/general types of sampling techniques: ◦ Probability Sampling: Each member of the population has a known and non-zero chance of being selected (e.g., Simple Random Sampling, Stratified Sampling, Cluster Sampling). 6 Common types of Probability sampling 1. Simple random sampling (SRS) The required number of individuals are selected at random from the sampling frame. Each member of a population has an equal chance of being included in the sample. It uses Lottery method To use a SRS method: Make a numbered list of all the units in the population Each unit should be numbered from 1 to N (where N is the size of the population) Select the required number. Example Advantages :no bias disadvantage: Requires a sampling frame. Difficult if the reference population is dispersed. 2. Systematic random sampling Sometimes called interval sampling Selection of individuals from the sampling frame systematically rather than randomly Individuals are taken at regular intervals down the list The starting point is chosen at random Taking individuals at fixed intervals (every k th) based on the sampling fraction. Steps in systematic random sampling 1. Number the units on your frame from 1 to N (where N is the total population size). 2. Determine the sampling interval (K) by dividing the number of units in the population by the desired sample size. 3. Select a number between one and K at random. This number is called the random start and would be the first number included in your sample. 4. Select every Kth unit after that first number e.g, To select a sample of 100 from a population of 400, you would need a sampling interval of 400 ÷ 100 = 4. Therefore, K = 4. You will need to select one unit out of every four units to end up with a total of 100 units in your sample. Select a number between 1 and 4 from a table of random numbers. If you choose 3, the third unit on your frame would be the first unit included in your sample. The sample might consist of the following units to make up a sample of 100: 3 (the random start), 7, 11, 15, 19...395, 399 (up to N, which is 400 in this case). A. 1, 5, 9, 13...393, 397 B. 2, 6, 10, 14...394, 398 C. 3, 7, 11, 15...395, 399 D. 4, 8, 12, 16...396, 400 11 3. Stratified random sampling It is done when the population is known to be have heterogeneity with regard to some factors and those factors are used for stratification Using stratified sampling, the population is divided into homogeneous, mutually exclusive groups called strata, and A population can be stratified by any variable that is available for all units prior to sampling (e.g., age, sex, income, etc.). The population are stratified on the bases of common characteristics Among strata there is heterogeneity and within each strata units are homogeneous three questions are highly relevant in the context of stratified sampling: How to form strata?(it can be formed on the basis of common characteristic(s) of the items to be put in each stratum.) How should items be selected from each stratum? Any of the sampling methods mentioned in this section (and others that exist) can be used to sample within each stratum. How many items be selected from each stratum or how to allocate the sample size of each stratum? A separate sample is taken independently from each stratum by proportion To determine the sample size drawn from each stratum, proportional allocation is commonly used. Proportional allocation ensures that the sample size from each stratum is proportional to the size of that stratum in the population. E.g., we want a sample of size n = 30 to be drawn from a population of size N = 8000 which is divided into three strata of size N1 = 4000, N2 = 2400 and N3 = 1600. what is the sample size drown from each stratum Dividing the population into subgroups (strata) and sampling proportionally from each stratum. ◦ Identify strata based on characteristics (e.g., age, role). ◦ Draw a random sample from each stratum proportional to its size in the population. Example Sampling 30% programmers, 50% testers, and 20% designers from a tech company to evaluate a development tool. ◦ Increases accuracy and representativeness. ◦ Requires detailed knowledge of the population. 15 4. Cluster sampling Cluster sampling is the most widely used to reduce the cost The clusters should be homogeneous Steps in cluster sampling Cluster sampling divides the population into groups or clusters. A number of clusters are selected randomly to represent the total population, and then all units within selected clusters are included in the sample. No units from non-selected clusters are included in the sample-they are represented by those from selected clusters. This differs from stratified sampling, where some units are selected from each group. Dividing the population into clusters and randomly selecting entire clusters for the sample. ◦ Divide the population into clusters (e.g., departments, offices, or schools). ◦ Randomly select clusters and include all individuals within selected clusters. ◦ Cost-effective for geographically dispersed populations. ◦ Risk of less diversity if clusters are homogeneous. Example A company has 50 branches nationwide. Randomly select 5 branches and survey all employees in those branches about their software usage. 17 B. Non-probability sampling In non-probability sampling, every item has an unknown chance of being selected. The most common types of non-probability sampling Convenience Volunteer sampling Judgment sampling Quota sampling Snowball sampling technique 1. Convenience sampling Selecting samples based on ease of access. Quick and inexpensive. Simple and fast. Limited generalizability. May lead to biased results. Example 2.Volunteer sampling As the term implies, this type of sampling occurs when people volunteer to be involved in the study. In psychological experiments or pharmaceutical trials (drug testing), for example, it would be difficult and unethical to enlist random participants from the general public. In these instances, the sample is taken from a group of volunteers. Sometimes, the researcher offers payment to attract respondents. In exchange, the volunteers accept the possibility of a lengthy, demanding or sometimes unpleasant process Example A university’s computer science department posts an open call for students to participate in usability testing for a new e-learning platform. Students who volunteer are included in the sample. 22 3. Judgment/purposive sampling The researcher uses their expertise to select the sample they believe is most representative. Relies on the researcher's judgment. Targeted and efficient. Prone to researcher bias. In the case of investigating the information System development trends and challenges in Ethiopian software companies, we may purposely select participants and companies that align with the research goal/s. 4. Quota sampling Ensuring the sample reflects specific characteristics in the population. Divide the population into categories. Select participants to meet quotas for each category. Ensures representation of subgroups. Not random, risk of bias. done until a specific number of units (quotas) for various sub-populations have been selected. Since there are no rules as to how these quotas are to be filled, quota sampling is really a means for satisfying sample size objectives for certain sub-populations. Example Surveying 50% software developers and 50% UI/UX designers to evaluate a software tool. Snowball Sampling Participants recruit other participants from their networks. ◦ Useful for hard-to-reach populations. Builds connections to hard-to-access groups. May lead to homogeneous samples. Example Collecting data from ethical hackers by asking one hacker to recommend others. 25 Sample size determination The size of the sample is one of the most important determinants of the accuracy of survey estimates. Samples size is estimated using formulae The selection of a formula depends on: Sampling strategies (cluster Vs simple random sampling) Population size The type of variable being studied Design type (experimental vs non-experimental ) Type of statistical comparison planned. Data Collection Methods/Techniques The process of gathering data for analysis to address research questions. There are two types of data Primary Data: Are those data, which are collected by the investigator himself for the purpose of a specific inquiry or study. Such data are original in character and are mostly generated by surveys conducted by individuals or research institutions. Secondary Data: Are those data which have already been collected and analyzed by27 Secondary data can be obtained from journals, reports, government publications, publications of professionals and research organizations. Secondary data are less expensive to collect both in money and time. 28 Methods of Primary Data Collection Primary data obtained either through observation or through direct communication with respondents in one form or another or through personal interviews. There are several methods of collecting primary data, particularly in surveys and descriptive researches. Important ones are: Observation method, Interview method, Questionnaires 29 Observation method It is the gathering of primary data by investigator’s own direct observation of relevant situations without asking from the respondent. The aim is to gather data or information about the world as it is. So you hope the act of studying doesn't substantially modify the thing you are interested in. Observation can yield information which people are normally unwilling or unable to provide. 30 Advantage The main advantage of this method is that subjective bias is eliminated, if observation is done accurately. Secondly, the information obtained under this method relates to what is currently happening; it is not complicated by either the past behavior or future intentions or attitudes. Thirdly, this method is independent of respondents’ willingness to respond Limitations feelings, beliefs and attitudes cannot be observed. expensive method 31 Interview method It is a verbal face-to-face discussion or communication via some technology like the telephone or computer between an interviewer and a respondent. Unstructured: It allows a free flow of communication in the course of the interview or questionnaire administration. Structured: where the information that needs to be collected from the respondents is already decided. Semi-structured: Restricts certain kinds of communications but allows maneuvering freedom on the discussion of certain topics. 32 Questionnaires This method of data collection is quite popular, particularly in case of big inve stigations. The questionnaire is mailed (usually by post) to respondents who are expected to read, understand and write down the reply in the space meant for the purpose in the questionnaire itself. Type of Question Open-ended questions: Type of questions that do not have pre-coded options (answers). Dichotomous questions: Dichotomous questions have two possible answers like yes/no, true/false or agree/disagree responses. Multiple-response questions: Type of questions has many probable answers. 33