Module 2 Handout - Comparison Experiments and Analysis of Single Factor Experiments PDF
Document Details
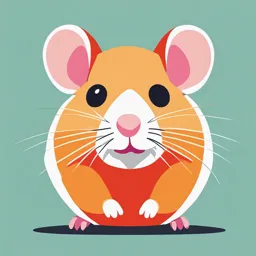
Uploaded by EncouragingMonkey
University of the Philippines Los Baños
Tags
Summary
This document is a module handout on comparison experiments and single factor experiments, covering hypothesis testing and analysis of variance (ANOVA). It provides an overview, topics, and introductory information on inferential statistics, which are crucial concepts for statistical analysis. The document also introduces the formulation of statistical hypotheses and associated principles, demonstrating statistical concepts and procedures. The document is aimed at individuals studying experimental designs and statistical methods.
Full Transcript
MODULE 2: COMPARISON EXPERIMENTS AND ANALYSIS OF SINGLE FACTOR EXPERIMENTS MODULE OVERVIEW As learned from the previous module, the motivation in implementing experimental designs is to provide statistically sound inferences which could lead to new discoveries. To co...
MODULE 2: COMPARISON EXPERIMENTS AND ANALYSIS OF SINGLE FACTOR EXPERIMENTS MODULE OVERVIEW As learned from the previous module, the motivation in implementing experimental designs is to provide statistically sound inferences which could lead to new discoveries. To come up with valid inferences, you must follow appropriate procedure in hypothesis testing. In this module, we will learn the steps in hypothesis testing, apply the steps in relation to experiments and understand one of the most utilized method in analyzing experimental data — the analysis of variance (ANOVA). At the end of this module, you should be able to perform test of hypotheses for a single population and comparing experiments, conduct one-factor ANOVA, and test its assumption. MODULE TOPICS I. Steps in Hypothesis Testing II. Test of Hypothesis for Comparison Experiments III. Analysis of Variance and its Assumptions TOPIC 1: Steps in Hypothesis Testing In statistics, we deal with a lot of data. To give meaning to the data, we present and analyze them in a manner that can be understood by many. The use of statistics in dealing with these data is divided into two broad categories: descriptive and inferential statistics. Descriptive statistics is concerned with summarizing obtained data while inferential statistics is focused on generalizing about a characteristic of a population where only a part was examined. Shown in the slide is the process of inferential statistics. In making valid conclusions about the characteristic of interest, a proper procedure called hypothesis testing must be followed. Hypothesis testing is an area in inferential statistics which evaluates a conjecture about some characteristic of the parent population based on the information contained in the random sample. Usually, the conjecture concerns one of the unknown parameters (e.g. mean, proportion, variance) of the population. The usual steps followed in hypothesis testing are given below: Page | 1 STAT 162: Experimental Designs Institute of Statistics University of the Philippines Los Baños MODULE 2 Comparison Experiments and Analysis of Single Factor Experiments The first step in hypothesis testing is the formulation of a statistical hypothesis. A statistical hypothesis is a conjecture about one or more populations whose accuracy can be established using sample data. There are two types of statistical hypothesis, the null hypothesis and alternative hypothesis. The null hypothesis is usually denoted as Ho. It is the statement being tested or the statement that the experimenter doubts to be true. Moreover, null hypothesis always pertains to a statement of equality, no difference, no change, or status quo. Thus, it is written using any one of the these symbols: =, ≤, or ≥. STAT 162: Experimental Designs Page | 2 Institute of Statistics University of the Philippines Los Baños MODULE 2 Comparison Experiments and Analysis of Single Factor Experiments On the other hand, the alternative hypothesis denoted by Ha or H1, is a statement that must be true if the null hypothesis is false. Usually, this is an operational statement of the theory that the experimenter believes to be true or wishes to prove. That is why it is often referred to as the “researcher’s hypothesis.” It is written using any of the following symbols: ≠,. In formulating the null and alternative hypothesis, you must first identify the unit of interest is an individual or an object where the response will be measured. Next, identify the variable of interest which refers to the information evaluated from the unit of interest. Note that one can also refer the unit of interest as the sampling unit while the variable of interest as the response variable. Lastly, identify the parameter of interest (i.e. based on the variable of interest) which is the unknown quantity to be tested. Some common parameters of interests are the mean (μ), proportion (P), and variance (σ2). STAT 162: Experimental Designs Page | 3 Institute of Statistics University of the Philippines Los Baños MODULE 2 Comparison Experiments and Analysis of Single Factor Experiments The statistical hypothesis in symbols is stated in the form:. For example, in the one population test for the mean where the hypothesized value is equal to 370, we can state the null hypothesis as Ho: μ = 370, and the alternative hypothesis as the opposite which is Ha: μ ≠ 370. In this example, “μ” (i.e. the population mean as an average) is the parameter of interest, “=” is the symbol, and “370” is the hypothesized value. The hypotheses to be formulated often come in pairs (Ho and Ha), but the identification of the appropriate hypothesis depends on the actual problem. Thus, we have some conventions in formulating a hypothesis. These conventions are shown in the slide. Let us have this example. STAT 162: Experimental Designs Page | 4 Institute of Statistics University of the Philippines Los Baños MODULE 2 Comparison Experiments and Analysis of Single Factor Experiments Next, the claim or statement to be tested is “if the life expectancy lasts longer than the mean 13 years”. This statement is not a statement of equality. Thus, according to our convention this will be the alternative hypothesis. Since we want to test if the life expectancy will be longer, then the symbol we will use is “>”, and the hypothesized value is 13 which makes our alternative hypothesis as Ha: μ > 13. Finally, our null hypothesis will be “=” following the second convention (i.e. Ho: μ = 13). Our null and alternative hypothesis in symbols and in words would be: Symbol Words Ho: μ = 13 The mean life expectancy of the refrigerator is equal to 13. Ha: μ > 13 The mean life expectancy of the refrigerator is greater than 13. Here is another example with the following details. Then, the statement/claim to be tested is “if the proportion of drivers who admit to running the red light is at least 50%”. At least means greater than or equal, thus, it is a statement of equality. This means that this will be our null hypothesis. And the opposite, which is “