Review of Basic Statistics (AMAT 131) PDF
Document Details
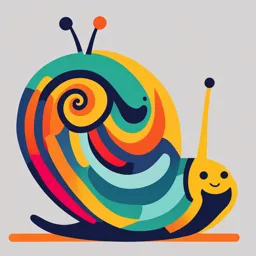
Uploaded by EnrapturedSard4691
University of the Philippines Mindanao
Tags
Summary
This document covers review material for the AMAT 131 course, focusing on basic concepts in statistics, such as probability distributions, hypothesis testing, and experimental design. It includes numerous examples to illustrate these concepts, as well as introductions to R and R Studio.
Full Transcript
Review of Basic Statistics Week 2: AMAT 131 Statistical Methods and Experimental Design EODiamante DMPCS, CSM, UP Mindanao STAT 1 Elementary Statistics Introd...
Review of Basic Statistics Week 2: AMAT 131 Statistical Methods and Experimental Design EODiamante DMPCS, CSM, UP Mindanao STAT 1 Elementary Statistics Introduction to Statistics Hypothesis Testing Hypothesis Testing Part 1 Part 2 A. Introduction to Statistics B. Methods of Data H. Parameter L. Correlation Presentation Estimation Analysis C. Descriptive Statistics I. Hypothesis Testing: M. Regression D. Probability and Counting Rules One Mean Analysis E. Discrete Probability J. Hypothesis Testing: N. Chi-Square Tests Distributions Two Means F. The Normal Probability K. Analysis of Variance Distribution G. The Central Limit Theorem Long Exam 1 Long Exam 2 Long Exam 3 Assumptions for Parametric Tests 1. The distribution is approximately normally distributed. https://www.w3schools.com/statistics/img_normal_distribution.svg Assumptions for Parametric Tests 2. For some tests, the equality (homogeneity) of variance assumption https://uc- r.github.io/public/images/analytics/homogeneity/assumption_homogeneity_main_pic.png What to do if the assumptions are not met? 1. Check and remove outliers An outlier may be due to a variability in the measurement, an indication of novel data, or it may be the result of experimental error; What to do if the assumptions are not met? 2. Transform data Logarithmic transformation, log 𝑥 Square Root transformation, 𝑥 Reciprocal transformation, 1/𝑥 Power transformation, 𝑥 𝑘 What to do if the assumptions are not met? 3. Consider non-parametric alternatives Non-parametric tests do not make assumptions on the distribution, “distribution-free” Performs rank transformation on the dataset during computation BUT…not as powerful as parametric tests Choosing which model to use Probability Distributions Experimental Design Relationship and and Comparison of Two Association Populations Experimental Design: Single Relationship and Factor Experiment Probability Distributions Association A. Introduction to Experimental A. Review of Basic Statistics A. Simple Correlation Design B. Introduction to Probability Analysis: Parametric B. Principles of Experimental Distributions B. Simple Correlation Design C. Binomial Distribution Analysis: Non- C. Analysis of Variance (ANOVA) D. Multinomial Distribution Parametric D. Assumptions of ANOVA: E. Poisson Distribution AMAT 131 F. Other Probability Distributions G. Normal Distribution Violations and Remedies E. Completely Randomized C. Simple Linear Regression Analysis Statistical Methods and Comparison of Two Populations Design (CRD) D. Multiple Linear Experimental Design F. Kruskal Wallis Test Regression Analysis A. Independent Samples: G. Randomized Complete Block Parametric Design (RCBD) B. Related Samples: Parametric H. Friedman Test C. Independent Samples: Non- I. Latin Square Design (LSD) Parametric Factorial Design D. Related Samples: Non- A. Two Factor Factorial Design Parametric B. Split-Plot Design Long Exam 1 Long Exam 2 Long Exam 3 Navigating our course on UVLE 1. Go to uvle.upmin.edu.ph 2. Log in to your account using your UP Email address. 3. Navigate to the course you are currently assigned to: AMAT 131. 4. Explore the features of the platform. Free Statistical Software PAST R and R Studio Basic Statistical Terms ▪ Universe: set of all entities or individuals under consideration ▪ Variable: characteristic or attribute that can assume different values. ▪ Two types: qualitative vs. quantitative ▪ Data: values (measurements or observations) that the variables can assume. ▪ Random variable: represents possible outcomes of a process that is NOT deterministic but includes some unpredictable variation Basic Statistical Terms ▪ Two phases of statistical inference: 1. Estimation: determining the true value of parameter thru a sample ▪ Point estimate: a specific numerical value estimate of a parameter (i.e. the point estimate for the population mean is the sample mean) ▪ Interval estimate: an interval or range of values used to estimate the parameter; this may or may not contain the value of the parameter being estimated 2. Hypothesis testing: testing assertions/claims about the population through a sample Basic Statistical Terms ▪ Population: consists of all subjects (human or otherwise) that are being studied; set of ALL possible values of the variable ▪ Sample: group of subjects selected from a population Example: Universe: All households in the Philippines Variable: No. of family members per household in the Philippines Population: 𝐻 = {1,2,3, … } Sample of three households: {3,7,10} Summation Notation ▪ In mathematics, the symbol Σ means to add or find the sum. The summation notation is given as: Summation Notation First Constant Theorem: 𝑛 𝑘 = 𝑛𝑘 𝑖=1 Summation Notation Second Constant Theorem: 𝑛 𝑛 𝑘𝑋𝑖 = 𝑘 𝑋𝑖 𝑖=1 𝑖=1 Summation Notation Third Constant Theorem: 𝑛 𝑛 𝑛 (𝑎𝑋𝑖 + 𝑏𝑌𝑖 ) = 𝑎 𝑋𝑖 + 𝑏 𝑌𝑖 𝑖=1 𝑖=1 𝑖=1 Example: A total of 𝑛 = 7 measurements of the yield of a commercial variety of wheat were obtained from a field trial. The samples were converted into equivalent yields per hectare as: 7,9,6,12,4,6, and 9 tons. Compute the following: ▪ Mean σ𝑛𝑖=1 𝑦𝑖 𝑦ത = 𝑛 ▪ Sample variance, 𝑠 2 σ𝑛 2 𝑖=1 𝑦𝑖 − 𝑦 ത 𝑠2 = 𝑛−1 ▪ Sample standard deviation, 𝑠. σ𝑛𝑖=1 𝑦𝑖 − 𝑦 2 𝑠= 𝑛−1 Probability Distributions The probability structure of a random variable, 𝑦, is described by its probability distribution. ▪ If 𝑦 is discrete, 𝑝(𝑦) is called the probability mass function of y ▪ If 𝑦 is continuous, 𝑝(𝑦) is called the probability density function of y Random variable ▪ A random variable is a numerical variable whose value depends on the outcome of a random experiment. ▪ Associates a numerical value with each outcome in the sample space. ▪ 𝑌 denotes the random variable, 𝑦 represents one of its values, each possible value of 𝑌 represents an event. Types of Random variable 1. Discrete Random Variable ▪ Possible values are whole numbers ▪ Have a finite possible values or infinite number of values that are countable 2. Continuous Random Variable ▪ Can assume all values in the interval between any two given values and can be decimal and fractional values. ▪ Obtained from data that can be measured rather than counted Example – Identify the random variable and the type of random variable 1. Three electronic components are tested and classified as defective or non-defective. Solution: Let the random variable 𝑌 be the number of defective electrical components. The sample space or the outcomes of 𝑌 is 0,1,2, or 3, denoted as 𝑌 = 0,1,2,3. Based on the sample space, 𝑌 is a discrete random variable. Example – Identify the random variable and the type of random variable 2. A die is thrown until a 5 occurs. Solution: Let the random variable 𝑌 be the non-occurrence of 5 when a die is thrown. The sample space is 𝑌 = 1,2,3,4,6. 𝑌 is a discrete random variable Example – Identify the random variable and the type of random variable 3. Effect of height-growing supplement on the height of a 5- year-old kid. Solution: Let the random variable 𝑌 be height difference of 5- year-old kids before and after taking the height-growing supplement. The sample space is 𝑌 = {𝑦 ≥ 0}; thus, 𝑌 is a continuous random variable. Questions? Next meeting: Probability Distributions