Module 1-3 Part A STAT 151 PDF
Document Details
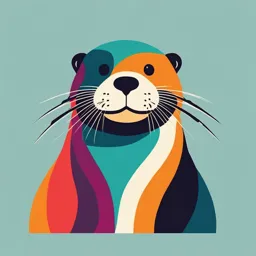
Uploaded by HardWorkingLute
Tags
Summary
This document discusses different methods of sampling, including simple random sampling, stratified random sampling, and cluster sampling. It explains how to choose representative samples from a population and avoid biases. The document includes examples and illustrations in the context of statistics.
Full Transcript
Module 1-3 Part A (Chapter 9: Sample Surveys) One way to gather information about a population is to conduct a census, that is, collect data from the entire population. There are many problems associated with trying to conduct a census: the population may be too large to carry one out i...
Module 1-3 Part A (Chapter 9: Sample Surveys) One way to gather information about a population is to conduct a census, that is, collect data from the entire population. There are many problems associated with trying to conduct a census: the population may be too large to carry one out it may be difficult to find every individual in a population it may be impractical – for example, it would be impractical to collect data about the amount of damage that occurs on all vehicles of a specific model moving a particular speed by subjecting every one of those vehicles to a collision. costly takes a long time to complete the population characteristics being studied may change while the census is taking place complex process Instead, we can take a sample from the population and use the sample to make inferences about the population. (Strictly speaking, a census may be considered as a sample in which the entire population is selected.) For example, at some point you may have been asked by a company to participate in a sample survey (a study that asks questions of a sample selected from a population with the intension of learning something about the entire population). Companies use this information to make decisions about their products and services. To apply inferential statistic, we require a representative sample, that is, a sample that reflects, as closely as possible, the characteristics of the population being studied. Samples that are not accurate representations of the population are said to be biased. Bias is any systematic failure of a sampling method to accurately represent a population. It is the tendency of a sample statistic to overestimate or underestimate a population parameter. To try to avoid bias and obtain a representative sample, we use random sampling, that is, we randomly select individuals from the population to be included in a sample. In general, when selecting a random sample, it is the sample size, that is, the number of individ- uals in the sample, not the fraction of the population that you have sampled, that determines how reliable an estimate will be. If multiple random samples are taken from the same population, the samples will generally be dif- ferent from each other. The values of the variables that we measure for each of these samples will also differ from each other. These sample-to-sample differences are called sampling variability. In order to make selections for a sample, we require a sampling frame. A sampling frame is a list of individuals/items in the population of interest. A sample is taken by selecting items on the list. Any individual/item not on the list has no chance of being selected for the sample. (In addition to lists, a sampling frame could be some other material which displays the individuals/items, such as a Module 1-3 Part A Page 1 of 4 map.) For example, to select a sample of employees at a particular company, we could obtain a list of all employees (a sampling frame) and draw a sample from that list. Random Sampling Methods: a) Simple Random Sampling b) Stratified Random Sampling c) Cluster Sampling d) Systematic Sampling e) Multistage Sampling Simple Random Sampling: a method of sampling in which every sample of size n has the same chance of being selected (that is, every collection of n individuals has the same chance of being selected to be the sample). a sample selected using simple random sampling is called a simple random sample (SRS). this method also ensures that every member of the population has the same chance of being included in the sample. for example, put the name of every member of the population in a container, mix the names up thoroughly, and select n names from the container. this method can be done using random numbers. difficult to perform, in practice. may be difficult to obtain a sampling frame. – for example, it would be impossible to obtain a list all people living in Edmonton (including new arrivals, the homeless, undocumented immigrants, etc.) on a specific day. Simple random sampling is the most basic of the sampling methods. The other methods are more complicated, but may be more efficient, may be less costly, may help to reduce bias, or may improve estimate accuracy. Stratified Random Sampling: a method of sampling in which the population is first partitioned into homogeneous groups, called strata, and then simple random samples are drawn from each group/stratum. a sample selected using stratified random sampling is called a stratified random sample. one way to ensure that certain demographic groups are included in the sample. for example, a Canadian government survey trying gauge national support for a particular policy may divide the country up into geographic regions and then take an SRS in each region (to guarantee region representation in the survey). Module 1-3 Part A Page 2 of 4 benefits over SRS: – may help to reduce sample variability – increased flexibility, may use different methods to sample each stratum – gives estimates for each stratum, as well as the entire population Cluster Sampling: a method of sampling in which the population is partitioned into groups, called clusters, and then a simple random sample of clusters is selected from the population. For each cluster selected, a census is conducted of that cluster (that is, every individual in the cluster is included in the sample). a sample selected using cluster sampling is called a cluster sample. for example, if the City of Edmonton wants to conduct a survey on transit use by its residents, it could view each residential city block as a cluster and then randomly select a certain number of blocks to survey. may be less precise than using stratified / simple random sampling, but is more practical, more convenient, and may be less costly. Systematic Sampling: a method of sampling in which individuals are selected systematically from a sampling frame. a sample selected using systematic sampling is called a systematic sample. for example, starting from a randomly selected individual, select every 5th individual on an alphabetical list of employees. start the selection process at a randomly selected individual. If you want to select every k th individual in a list, then randomly choose an individual from number 1 to k on the list to start. results similar to an SRS if there is no relationship between the order of the list and the variable of interest. may be less costly and easier to carry out than an SRS. Multistage Sampling: a method of sampling which involves multiple stages of random sampling. a sample selected using multistage sampling is called a multistage sample. for example, if the City of Edmonton wants to conduct a survey on transit use by its residents, it could use cluster sampling to randomly select a certain number of residential city blocks, then use systematic sampling to select the 4th house on each block (on each side of the street), and then conduct a census of each household selected. may use a different method at each stage. involves smaller and smaller sampling units at each successive stage. Module 1-3 Part A Page 3 of 4 There are other types of samples which do not involve random selection, that is, they come from non-random sampling. Non-random sampling leads to biased results. These samples cannot be used to make inferences about a population: a) Voluntary Response Sample: a sample in which individuals can decide on their own whether to participate. A large group of individuals are invited to respond. Those who choose to respond are counted. for example, Internet polls often only individuals with very negative opinions or very positive opinions will respond unlikely that the sample will be a good representation of the population survey results are invalid b) Convenience Sample: a sample of individuals who are easy to reach (conveniently available). someone goes to a convenient location and samples individuals who walk by. for example, a sample resulting from survey conducted a shopping mall. unlikely that the sample will be a good representation of a population. If a sample is obtained using a biased method, then any conclusions made based upon the sample will be flawed and unreliable. Once a sample is collected, there is usually no way to correct for the bias. It is important to carefully consider your sampling methods and survey designs and to try to reduce biases as much as possible before collecting any data. It is essential that samples are selected at random. Types of Bias: a) Voluntary Response Bias: a type of bias introduced to a sample when individuals can decide on their own whether to participate in the sample. This type of bias invalidates the results of the survey. b) Undercoverage: occurs when some portion(s) of the population are not sampled at all or have smaller representation in the sample than they have in the population. excluded individuals may have characteristics that differ from the rest of the population. for example, an opinion poll conducted by calling landlines will exclude anyone without a landline. c) Nonresponse Bias: occurs when not all individuals selected for a sample respond. individuals who don’t respond may have characteristics that differ from the rest of the population. of particular concern is when a large proportion of those sampled do not respond. d) Response Bias: refers to anything in the survey design that influences the responses. occurs when an individual’s responses are affected by external influences, such as the wording of the questions or the behaviour of the interviewer. the questions may be misleading, confusing, or of a sensitive nature. for example, a respondent may not answer truthfully to a question such as “Have you ever lied on your income tax return?” Module 1-3 Part A Page 4 of 4