Cross-Sell on the Web Student Guide PDF
Document Details
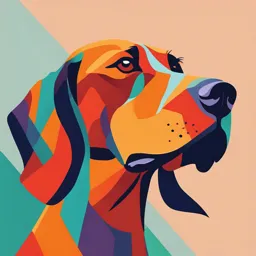
Uploaded by AmazingMoldavite7590
2024
Tags
Related
- PLAY BOOK - Soluções Transporte e Embalagem PDF
- Marketing Strategy and Integrated Marketing PDF
- Chitosan cross-linked poly(acrylic acid) hydrogels: Drug release control and mechanism PDF
- REx Module (1) Effective Selling Skill PDF
- Superabsorbent Cellulose-Based Hydrogels Cross-linked with Borax PDF
- JC-Ordner Fragenkatalog zur JC-Prüfung PDF
Summary
This PDF document is a student guide from Pegasystems Inc. focusing on cross-selling strategies using the Pega Customer Decision Hub. It covers topics such as Next-Best-Action Designer, real-time containers, and customer engagement policies.
Full Transcript
Cross-Sell on the Web STUDENT GUIDE Ó Copyright 2024 Pegasystems Inc., Cambridge, MA All rights reserved. This document describes products and services of Pegasys...
Cross-Sell on the Web STUDENT GUIDE Ó Copyright 2024 Pegasystems Inc., Cambridge, MA All rights reserved. This document describes products and services of Pegasystems Inc. It may contain trade secrets and proprietary information. The document and product are protected by copyright and distributed under licenses restricting their use, copying, distribution, or transmittal in any form without prior written authorization of Pegasystems Inc. This document is current as of the date of publication only. Changes in the document may be made from time to time at the discretion of Pegasystems. This document remains the property of Pegasystems and must be returned to it upon request. This document does not imply any commitment to offer or deliver the products or services provided. This document may include references to Pegasystems product features that have not been licensed by your company. If you have questions about whether a particular capability is included in your installation, please consult your Pegasystems service consultant. PegaRULES, Process Commander, SmartBPM® and the Pegasystems logo are trademarks or registered trademarks of Pegasystems Inc. All other product names, logos and symbols may be registered trademarks of their respective owners. Although Pegasystems Inc. strives for accuracy in its publications, any publication may contain inaccuracies or typographical errors. This document or Help System could contain technical inaccuracies or typographical errors. Changes are periodically added to the information herein. Pegasystems Inc. may make improvements and/or changes in the information described herein at any time. This document is the property of: Pegasystems Inc. 1 Rogers Street Cambridge, MA 02142 Phone: (617) 374-9600 Fax: (617) 374-9620 www.pega.com Mission: Cross-Sell on the Web Product: Pega Customer Decision Hub™ ’24.2 URL: https://academy.pega.com/mission/cross-sell-web/v8 Date: 10 April 2024 Contents Next-Best-Action on digital..............................................................................................................4 Business use case: Cross-sell on the web...................................................................................5 Next-Best-Action Designer...........................................................................................................9 Defining and managing customer actions...................................................................................16 Action hierarchy..........................................................................................................................17 Managing business structure.....................................................................................................22 Creating actions...........................................................................................................................25 Renaming actions........................................................................................................................30 Presenting a single offer on the web............................................................................................33 Real-time containers...................................................................................................................34 Creating and configuring a real-time container.......................................................................38 Presenting a single offer on the web........................................................................................40 Defining customer engagement policies......................................................................................45 Customer engagement policies.................................................................................................46 Defining eligibility, applicability, and suitability Rules.............................................................51 Creating and testing personas......................................................................................................56 Testing Next-Best-Action with Persona and Channel Context...............................................57 Testing the Engagement Policy configurations with Persona Test Cases.............................66 Avoiding overexposure of actions.................................................................................................70 Contact policies...........................................................................................................................71 Defining action suppression rules.............................................................................................73 Arbitrating between actions..........................................................................................................77 Action arbitration........................................................................................................................78 Prioritizing actions with business levers...................................................................................84 Next-Best-Action on digital Description Learn about a typical cross-selling use case on the web channel and its four phases of implementation to improve 1-to-1 customer engagement, drive sales, and deliver Next- Best-Actions in real time. Learning objectives Describe a typical cross-selling use case on the web channel List the four phases of use case implementation 4 Business use case: Cross-sell on the web Introduction Pega Customer Decision Hub’s Next-Best-Action Designer lets you configure how you want the always-on brain to select the best offer for a customer. The best offer is the result of a series of decisions that are executed in a hierarchical fashion by the brain. Cross-selling on the web channel will help you improve 1-to-1 customer engagement, drive sales, and deliver Next-Best-Actions in real-time. Transcript This video describes a typical cross-selling use case on the web channel. U+ is a retail bank. The bank would like to leverage its website as a marketing channel to improve 1-to-1 customer engagement, drive sales, and deliver Next-Best-Actions in real- time. The bank has decided to use the Pega Customer Decision Hub™ to recommend more relevant banner ads to its customers when they visit their personal portal. Banner ads are shown on various pages throughout the website. For example, on the home page, U+ can display a Hero banner at the top of the page, which is typically a larger image with bigger typeface. Below that, there is space to display several Tile banners, which are typically smaller. 5 When customers log in to their personal portal, they also see a Tile banner on the ‘Account overview’ page. The main intent of U+ at this stage is to increase their web engagement. This can be measured by click-through rate. A Click-through is recorded when the customer clicks on the ‘Learn more’ link. 6 The bank would like to use these banners on the ‘Account overview’ page, to display offers that are more relevant and likely to receive a positive response. The offers will be selected by a combination of artificial intelligence (AI) and other business rules. The AI and business rules are defined in the Pega Customer Decision Hub. The Pega Customer Decision Hub is the always-on customer brain that acts as a single, centralized decision authority. The always-on customer brain selects the right offer to be displayed to each customer who visits the bank’s website. Next-Best-Action Designer lets you configure how you want the always-on brain to select the best offer for a customer. The best offer is the result of a series of decisions that are executed in a hierarchical fashion by the brain. The bank plans to implement the requirement in multiple phases. The first phase is a proof-of-concept phase. In this phase, the goal is to display a credit card offer on the U+ website. This requires getting the basic environment up and running, setting up the business structure, defining an Action and a Treatment, and enabling channels and triggers for Next-Best-Action. As a result of this phase, a credit card offer will be displayed on the ‘Account overview’ page to all customers who visit the U+ web site. For example, if customer Troy logs in to his account, the ‘Cash back’ offer is displayed. If another user logs in, they will see the same offer. However, in practice, more offers should be displayed. Also, not all offers may be available to a customer for various reasons. The next phase is to add customer engagement policies. Engagement policies are the set of conditions such as eligibility, applicability, and suitability that qualify an offer, or a group of offers for a customer. As a result of engagement rules, customers will see only those offers that the organization believes they should be exposed to. For example, Troy logs in, he sees 7 the Rewards Card, but for Barbara this is not applicable, so it will never appear; instead, she sees the Rewards Plus Card. Too many contact attempts over a short period of time can have a negative impact on a customer's attitude toward further offers by your company. Therefore, in the next phase, U+ implements some contact policies using suppression rules, which allow an offer to be put on hold after a specific number of outcomes. For example, if Troy ignores an ad a few times, then the ad will no longer be shown to him over a period of time. Instead, his ‘Account overview’ page will show a different ad. Basically, from a set of all available offers, the choice is narrowed down by engagement policies. Then the selection is further narrowed down by suppression rules. After the engagement policies and suppression rules have “whittled down” the total possible offers to a few, Arbitration is used to choose the top offer based on what is relevant for the customer right NOW. Arbitration is the last phase of cross-sell in the U+ web use case. Arbitration aims at balancing customer relevance with business priorities. Specifically, Propensity, Context Weighting, Business Value, and Business Levers are given numerical values. A simple formula is then used to arrive at a prioritization value, which is used to select the top offer. For example, Troy qualifies for three credit card offers. When he logs in, he sees the top offer for him, the Standard Card. This offer is the Top 1 because the priority value is the highest among all other offers. This video has concluded. It showed you a typical cross-selling use case on the web channel and its four phases of implementation. 8 Next-Best-Action Designer Introduction Next-Best-Action Designer guides you through the creation of a Next-Best-Action strategy for your business. Its intuitive interface, proven best practices, and sophisticated underlying decisioning technology enable you to automatically deliver personalized customer experiences across inbound, outbound, and paid channels. Next-Best-Action Designer is organized according to the high-level sequence of steps needed to configure the next-best- action strategy for your organization. Transcript Next-Best-Action Designer guides you through the creation of a next-best-action strategy for your business. Its intuitive interface, proven best practices, and sophisticated underlying decisioning technology enable you to automatically deliver personalized customer experiences across inbound, outbound, and paid channels. Next-Best-Action Designer user interface allows you to easily define, manage, and monitor next best actions. The tabs across the top of the user interface represent the steps to complete to define next best actions. Use the Taxonomy component to define the business structure for your organization. Use the Constraints component to implement channel limits and constraints. Use the Engagement policy component to define the rules that control which actions are offered to which customers. Use the Arbitration component to configure the prioritization of actions. Use the Channels component to configure when and where the next best actions initiate. 9 The system uses these definitions to create an underlying Next-Best-Action Strategy framework. This framework leverages best practices to generate next-best-actions decision strategies at the enterprise level. These decision strategies are a combination of the business rules and AI models that form the core of the Pega Customer Decision Hub, which determines the personalized set of next best actions for each customer. Use the Taxonomy tab to define the hierarchy of business issues and groups to which an action belongs and create and manage customer journeys. 10 A business issue is the purpose behind the actions you offer to customers. For example, actions with the purpose of acquiring new customers belong to the business Issue of Acquire. Actions with the purpose of retaining existing customers are grouped under the business issue of Retain. Business groups organize customer actions into categories. For example, as part of the Acquire business Issue, you can create groups for products like credit cards, mortgages, or auto loans, with the intention of offering these to potential customers. Customer journeys are the sum of the phases that customers go through when interacting with an organization. You can create customer journeys in Next-Best-Action Designer, and then map customer actions to different journey stages. Each stage represents an experience that a customer goes through while interacting with an organization. On the Properties tab, you can define, manage, and view all the strategy result properties and action properties that are available in the application, including both default system properties and custom properties that you define for the outbound treatments or real-time containers. Use Constraints to specify outbound contact limits and limit overexposure to a specific action or group of actions. 11 Outbound channel limits allow you to limit the number of outbound communications that a customer can receive over a given period of time on a specific channel. For example, you can decide that you do not want your customers to receive more than two emails per week or two SMS messages per week. With action limits (outbound action limits and inbound action limits), you limit the number of actions that the customer sees during an interaction on an inbound or outbound channel. On the Constraints tab of Next-Best-Action Designer, you can define more extensive suppression rules by creating Contact Policy rules in the library. Contact Policy rules are reusable across all Business Issues and Groups. In the Contact Policy library, you define suppression rules that automatically put an action on hold after a specific number of outcomes are recorded for some or all channels. For example, an action can be suppressed for a customer for seven days after the customer has seen an ad for that action five times. Suppressing or pausing an action prevents over- exposure by limiting the number of times a customer is exposed to the same action. Use Engagement policies to define when specific actions or groups of actions are appropriate for customers. 12 There are four types of engagement policies: Eligibility determines whether or not a customer qualifies for an action or group of actions. For example, an action may only be available for customers over a specific age or living in a specific geographic location. Applicability determines if an action or group of actions is relevant for a customer at a particular point in time. For example, a discount on a specific credit card may not be relevant for a customer who already owns a card. Suitability determines if an action or group of actions is appropriate for a customer for ethical or empathetic reasons. For example, a new loan offer may not be appropriate for a customer whose credit score is low, even though it might be profitable for the bank. Contact Policies determine when an action or group of actions should be suppressed and for how long. For example, you can suppress an action after a specific number of promotional messages have been sent to the customer. To activate Contact Policy rules created in the library on the Constraints tab, add them to the Engagement Policy tab. Arbitration determines how Customer Decision Hub prioritizes the list of eligible and appropriate actions that come out of each group. 13 The factors weighed in arbitration are Propensity, Context weighting, Business value, and Business levers, each represented by numerical values. Propensity is the likelihood of a customer responding positively to an action. The propensity is calculated by AI. For example, clicking an offer banner or accepting an offer in the contact center are considered positive behaviors. Real-time contextual data is an important part of making highly relevant recommendations. Context weighting allows you to assign a weighting to a specific context value for all actions within an Issue or Group. For example, if a customer contacts the bank to change their address, the weight of the Service context increases, and the highest priority action is to ensure that the relevant service is delivered to the customer. Business value enables you to assign a financial value to an action and prioritize high-value actions over low-value ones. For example, promoting an unlimited data plan might be more profitable for the company than a limited data plan. Action values are typically normalized across Issues and Groups. Business levers enable you to accommodate ad hoc business priorities by specifying a weight for an action or group of actions and/or its associated business issue. A simple formula determines a prioritization value, which is used to select the top actions. 14 The final priority indicates the top action selected from the list of eligible and appropriate actions for the customer. You can explore the arbitration matrix in Customer Profile Viewer to understand what type of actions are displayed to the customer. You can use Customer Profile Viewer to examine specific profile and behavioral data, including previous next-best-action decisions and interaction history. Next-Best-Action Designer enables the delivery of next best actions through inbound, outbound, and paid channels. You can turn these channels on or off. If you turn off a channel, the next best actions are not delivered to that channel. An external real-time channel is any channel that displays the actions that Customer Decision Hub selects for a customer. These channels can include a website, a call-center, or a mobile application. A real-time container is a placeholder for content in an external real- time channel. A trigger is a mechanism whereby an external channel invokes the execution of a Next- Best-Action decisioning process for specific Issues and Groups. The result returns to the invoking channel. For example, when a real-time container called TopOffers is configured, the website invokes this real-time container before loading the account landing page. As you have seen in this video, Next-Best-Action Designer is organized according to the high-level sequence of steps needed to configure the Next-Best-Action strategy for your organization. These steps involve: 15 Defining the business structure for your organization. Implementing the channel limits and constraints. Defining the contact policies that control for which actions a customer qualifies. Configuring the prioritization of actions. Configuring when and where to initiate next best actions. You have reached the end of this video. 16 Defining and managing customer actions Description In Pega Customer Decision Hub™, learn how to use Next-Best-Action Designer to build the business issue/group hierarchies for your organization. Create an action under a specific business issue/group hierarchy and learn to rename an action to better reflect its business context. Learning objectives Explain an actions hierarchy Configure a business structure with issues and groups Create actions Rename actions 17 Action hierarchy Customer action introduction In Pega Customer Decision Hub™, next-best-action customer recommendations can take many forms, such as a banner advertisement, a retention offer, or a service message. Learn how to recognize different types of actions in your organization and organize similar actions into action groups. Customer actions in your organization In Pega Customer Decision Hub, customer actions are the specific actions that the system or application can take based on customer interactions and decisions. These actions can include sending personalized messages, offers, recommendations, or any other form of engagement with the customer. The actions are determined by the decisioning capabilities of Customer Decision Hub, which utilize analytics, business rules, and customer data to make intelligent decisions and drive personalized customer experiences. Consider the following scenarios in various channels: contact center, mobile, and web. Scenario 1: Contact center: Upsell A customer, Peter, has a subscription with a Telco company. A Customer Service Representative (CSR) calls Peter to encourage him to purchase an upgraded version of the subscription. This scenario is an upsell offer made in a contact center and is a valid customer action. 18 Scenario 2: SMS: Retention A customer, Troy, doesn’t use his credit card that often anymore and has decided to cancel the contract with U+ Bank. The Bank sends him an SMS message with the discount retention offer. This scenario is a retention offer made on the mobile channel to retain a customer and is a valid customer action. 19 Scenario 3: Inbound-web: cross-sell A customer, Arnold, searches for a new phone subscription on a Telco company website. He finds an advertisement for a monthly subscription with unlimited mobile internet and calls. This scenario is a banner advertisement on a website and is a valid customer action. 20 Customer action properties Every customer action has properties that define its characteristics. For example, a banner advertisement on a website usually consists of several elements, which help customers quickly understand the offer. 21 Image represents the visualization that is used for the banner. Price is a numeric property that represents the price of the customer action. Benefit represents the value proposition to the customer. Short title is a property that represents a short description of the customer action. Business hierarchy In Pega Customer Decision Hub, customer actions apply to various business issues and are organized into a three-level hierarchy. The business issue is the purpose of the actions that you offer to your customers. Each action that you prepare for your customers is associated with an issue and a group. Because of the association, you must always define the hierarchy before creating actions. In Pega Customer Decision Hub, a Business Issue represents the business area for which a customer action is applicable (for example, Grow, Retention, Collections, and Service), a Group organizes actions into logical categories (for example, Credit Cards or Mortgages), and an offer, which is referred to as an Action (for example, a Reward card or 30-year fixed rate). Each action that you prepare for your customers is associated with an issue and a group. You have reached the end of this video. What did it show you? - What are the customer actions in Customer Decision Hub. - What are the customer action properties. - Business hierarchy in Customer Decision Hub. 22 23 Managing business structure In Pega Customer Decision Hub™, you can use Next-Best-Action Designer to build the business issue and group hierarchies for your organization. Business issues and groups collectively form the organizing structure for your customer interactions. Each next best action that the system displays to a customer is associated with a business issue and group. Transcript This demo shows you how to manage your business structure in Next-Best-Action Designer. This is the Pega Customer Decision Hub portal. To manage the business structure, navigate to Next-Best-Action Designer. Customer actions are organized using a hierarchical business structure known as Issues and Groups. On the Taxonomy tab, you can view the issues and groups defined during the set up of the Financial Services (FS) framework. You can also define new Issues and Groups that will contribute to the next-best-action decisioning process. 24 Click Edit > Configure issues to add, remove, or create new Issues. The Configure Business Issues windowthat were shows the issues created at the earlier sstage. The issues marked blue are already active. Click the Collections issue to turn it off. The deactivated issue is automatically removed from a business hierarchy. Similarly, you can add, remove, or create business groups. To add more groups, configure the groups under the selected issue. Create a new Debit cards group. To complete the configuration, save the changes. 25 Note that you can disable only the issues and groups that do not have any references. For example, if there are actions or other references in the Grow issue, Credit cards group, you can not disable the issue or group. You have reached the end of this video. You have learned: - How to remove an existing issue from the business hierarchy. - How to add a new group to an existing Issue. 26 Creating actions Introduction An action holds various details about a particular offering, such as Start Date, End Date, and Expected Revenue. Each action is backed by a decisioning proposition. The system automatically manages this relationship by creating, deploying, and deleting the proposition instances as needed. Since an action is closely tied to a proposition, it must always be created in the context of an issue and group. Transcript This demo will show you how to create an Action. It will also explain how to create Actions in bulk. In Pega Customer Decision Hub, actions are customer recommendations that can take many forms, such as a banner advertisement, a retention offer, a service message, an e- mail message with the new offer, or different kinds of announcements. All these actions fall into one of the two categories: Always-on or One-time actions. Always-on actions are available for the Next-Best-Action framework to select and deliver the appropriate communication to the customer. They can be promotional, transactional, or mandatory. One-time actions are batch communication that all customers receive at a specific date and time. These actions are executed in a Business Operations Environment. No engagement policies or constraints are applied to these actions, and everyone receives the same action. U+, a retail bank, recently introduced several new credit card offers that they would like to display to their customers. As a decisioning consultant, you have been tasked with creating these offers in the Grow Issue and CreditCards Group as per the pre-defined business hierarchy. This is the Pega Customer Decision Hub™ portal. Actions are created and managed on the Actions landing page. 27 You can either create a single Action or Actions in bulk. To create a single Action, enter a short description of the new Action. Select the appropriate Issue and Group. Now, open the Action. The attributes of an Action distinguish it from other Actions. Attributes are used by the Customer Decision Hub to select the right Action for a customer. Fill in the attributes relevant to this Action. For example, provide a Description that explains its purpose and Benefits that describe how this Action will benefit the customer. Bear in mind that some of the values you enter may be customer-facing information. For example, U+ wants to display the content of the Benefits attribute when this Action is presented on its website. An Action can be associated to a Context, and it will be evaluated as part of the Next-Best- Action for that Context. In this case, Customer is the primary context. 28 To complete the configuration, save. To view Actions within a certain Group, you can filter by a specific Issue and Group. This is the Action you just created. Thus far you have created a single Action. However, sometimes you may want to create multiple Actions at once. You can do that by uploading a list of Actions from a Comma Separated Value (CSV) file. To create such a CSV file you need to download a CSV template and fill it in. Open the downloaded CSV template. You can use this template to add/update Actions. Add new Actions with corresponding attributes to the file. Properties that start with py, such as pyIssue, pyName, pyGroup, pyLabel and pyIsPropositionActive are mandatory internal properties. It is important that you input these values in the correct format into the CSV file. 29 The property pyIsPropositionActive corresponds to the Availability property, which is visible on the Details tab of an Action. Possible values for this property are 'Always', 'Never' and 'Date'. Choose 'Always' to ensure that the Action is selected during the Next-Best-Action decisioning process by the Customer Decision Hub. Set it to 'Never' if you never want the Action to be selected, for example when you want to retire the Action If you set the value to 'Date', you should enter a date range in the 'StartDate' and 'EndDate' fields. The Customer Decision Hub will select this Action only during that time period. pyIssue corresponds to the Issue the Action was created for. pyLabel corresponds to a short description of the Action. pyName corresponds to the Action name. pyGroup is the Group to which the Action belongs. Save and close the CSV file. Now, import Actions from the file. Select the CSV file that contains the list of Actions you want to import. If you want to delete existing Actions from this Issue/Group that have been created in the system but not in the CSV file, you can select this option. For now, you are only interested in adding new Actions. 30 Notice that the window displays a summary of the result of the import. For example, when a duplicate Action is added, the Errors count is incremented, and you can download the error file to learn the exact issue. Complete the import. Notice that the new Actions are now listed on the Actions page. This demo has concluded. What did it show you? - How to create an Action. - How to set Action attributes. - The most important properties of Actions in a CSV file. - How to create multiple Actions using a CSV file. 31 Renaming actions Introduction By default, Pega Customer Decision Hub uses the term ‘actions’ to refer to messages delivered during a customer interaction. If needed, you can change this to a more appropriate term, such as Promotions or Nudges. Actions can be renamed with different terms based on the business issue and group to which the product belongs. Transcript This demo will show you how to change the default naming of Actions to something that better reflects their business context. This is the Pega Customer Decision Hub™ portal. On the Actions landing page, you can view all the Actions that have been created. To view Actions within a certain Group, you can filter by a specific Issue and Group. In this case, filter Actions in the Grow Issue and CreditCards Group. Open an Action to view the name. Notice that the Grow/CreditCards Group uses the default terminology ‘Action’. U+ bank wants to rename Actions under the Grow Issue and CreditCards Group to better suit their business purpose. The Actions under the CreditCards Group are always promotional credit card offers, therefore the bank wants to change the naming convention from ‘Action’ to ‘Offer’. You can change the default naming convention of Actions in Next-Best-Action Designer. To manage the business structure, navigate to the Taxonomy tab of Next-Best-Action Designer. The ‘Action naming’ convention is set in a business Group’s configuration settings. This can be set for each group in the business hierarchy. Edit the hierarchy to modify the Group attributes. 32 In this case, change the Action naming convention to Offer. To complete the configuration, save the changes. Verify that the Action is renamed. Open any Action under the Grow Issue and CreditCards Group to view the effect of renaming. 33 From now on, Actions under Grow/CreditCards will be referred to as Offers. This demo has concluded. What did it show you? - How to change the default naming convention of Actions, to a name that better reflects their business context. 34 Presenting a single offer on the web Description Learn about real-time containers and how they are used to display actions selected by Pega Customer Decision Hub in real-time channels. Gain experience creating and configuring real-time containers by learning how to configure Next-Best-Action Designer to select a single offer that will be displayed on a website. Learning objectives Describe the concept of real-time containers Explain how to create and configure a real-time container Explain how to configure Next-Best-Action designer to display an offer on the web 35 Real-time containers Introduction A real-time container is a service that manages communication between Pega Customer Decision Hub and external channels. An external real-time channel is any channel that presents actions selected by the Customer Decision Hub to a user or customer. For example, a website, a call-center application, or a mobile application. Transcript This video explains the concept of real-time containers, which manage communication between the Pega Customer Decision Hub™ and external channels. This is the website of a retail bank called U+ Bank. The bank plans to promote new offers on the account page, which is displayed when customers log in. 36 The marketing department wants to leverage the Customer Decision Hub’s Next-Best- Action capability to display the right offer for each customer. The Customer Decision Hub’s real-time container functionality is used to implement this requirement. A real-time container is a placeholder for content in an external real-time channel. An external real-time channel is any channel that presents actions selected by the Customer Decision Hub to a user or customer. For example, a website, a call-center application, or a mobile application. 37 Here’s how the website invokes the real-time container to present credit card offers on the account page. In the Customer Decision Hub’s Next-Best-Action Designer, a real-time container called “Account Page Container” is configured. The website invokes this real-time container before loading the account page. The Customer Decision Hub then evaluates the actions from the associated Issue/Group, which in this case is Sales/CreditCards and returns the resulting offer details back to the website. The website then loads the account page with the content returned by the Customer Decision Hub, such as the offer image, description and other relevant attributes. 38 Meanwhile, the Customer Decision Hub records these customer interactions in the Interaction History. An Impression is recorded to indicate that the action was shown to the customer, a Click-through is recorded when the customer clicks on the action. Marketers use these metrics, i.e. Impressions and Click-throughs, to measure the level of customer engagement, and therefore, the success of the marketing effort. 39 Creating and configuring a real-time container Introduction Learn how to create and configure a real-time container which allows you to manage communication between Pega Customer Decision Hub and external channels. Transcript This demo will show you how to create and configure a real-time container. To create real-time containers, navigate to the Real-Time Artifacts landing page. Enter a short description of the new real-time container. An external channel uses the real-time container’s identifier when it makes a request to the Pega Customer Decision Hub. You can change the identifier to a value that the external channel in your environment expects. For example, the U+ Bank website is pre-configured to call a real-time container with the identifier Ubank_home_banner. Now, open the real-time container. Here you can turn the real-time container’s ability to respond to requests from external channels on or off. Keeping the default value will allow the Customer Decision Hub to serve requests from the website. ‘Impression capture’ allows the external channel to control how the Customer Decision Hub records impressions. 40 ‘Captured on retrieval’ means that the Customer Decision Hub records an impression in the Interaction History immediately after sending the action details to the external channel. ‘Captured by channel’ means that the Customer Decision Hub does not immediately record an impression. Instead, the external channel can explicitly request that the Customer Decision Hub records an impression at a later point in time. In this case, the bank wants the impressions not followed by a click to be captured, so keep the default value. To record a click in the Interaction History without initiating an action flow for the customer, keep the default value. This option is suitable for actions that do not have a defined flow, for example, on the Web channel when a customer clicking on an ad does not trigger any follow-up steps. Here you can view the list of Next-Best-Action strategies that you have associated with this real-time container. Save the configuration. Navigate to the Containers tab to see the newly created real-time container. This demo has concluded. What did it show you? - How to create a real-time container. - How to configure the real-time container settings. 41 Presenting a single offer on the web Introduction Pega Customer Decision Hub is the always-on customer brain used to select the right offer to be presented to each customer in any real-time channel. Learn how to configure Next- Best-Action Designer to select a single offer that will be displayed on a website. Transcript This demo will show you how to configure Next-Best-Action Designer to select a single offer that will be displayed on a website. U+, a retail bank, would like to use the Pega Customer Decision Hub™ to display a single offer on its website. U+ bank wants to make offers related to credit cards and display the same ‘Cash back’ offer to every customer who logs in to the web site. For example, if customer Troy logs in to his Accounts page, the ‘Cash back’ offer is displayed. If Troy clicks on the ‘Learn more’ button, it takes him to the Offers page. This page shows the offer details. 42 To implement this business requirement, navigate to Next-Best-Action designer. Here you can configure business rules to define when specific actions or groups of actions are appropriate for customers. Notice that the NBA hierarchy currently has three Business issues with Groups under them. In this case, U+ wants to promote credit card offers. So, open the CreditCards Group. You can configure the actions here. A consultant has already created a few actions under Sales/CreditCards. However, in this scenario, the bank wants to show only the ‘Cash back’ card. Now, open on the ‘Cash back’ offer. To display an offer, you need to add a treatment that is specific to the channel. In this scenario, U+ bank wants to present the offer on their website, so select the right treatment type. 43 A web treatment represents the message that you want to communicate visually to your customer on the web channel. You can either use an existing treatment or create one here. Now, fill in the required fields for the web treatment. Here you provide a link to an image that will display the action for this treatment. Once you provide the link, a preview of the content is displayed. This is the URL that you want the customer to go to when they click on the action. 44 Now, select the intended location and style of the treatment design. For example, the treatment can appear as a large central banner, a rotating strip of images, or a footer on the website. In this scenario, select Tile to display the ‘Cash back’ offer on the top right of the Account page. You can specify the language of the treatment if required. Specifying the treatment language allows Pega Customer Decision Hub to consider the customer’s preferred language when selecting the treatment. Add the web treatment you just created. In this phase, U+ does not have any further eligibility or prioritization requirements for this action. Save the changes. Here, you can enable the channels and triggers that will invoke Next-Best-Actions. As U+ wants to display the offer on the web, enable the web channel. Now, configure the real-time container that the U+ website will use to display the offer banner on the account page. The real-time container manages communication between the Pega Customer Decision Hub and external channels such as the web and call center. A decisioning consultant has already configured a real-time container for you, so select it to be added to your configuration. Once the real-time container is added, configure it to select the results from an appropriate Business issue and Group. In this case, U+ bank wants to display the ‘Cash back’ offer, which is under Sales/CreditCards. 45 With that, all the necessary configuration for this scenario is complete. Save the changes for the configuration to take effect. The account page on the U+ Bank website has been configured to use the real-time container with the name 'SalesCreditCards' and with Placement type 'Tile'. The web treatment is shown as a tile on the top right of the page. When Troy clicks on the ‘Learn more’ button, it takes him to the URL that was configured as the click-through URL for the treatment. If another user logs in, they will see the same offer. This demo has concluded. What did it show you? - How to configure Next-Best-Action designer to display a web treatment on the U+ bank website. 46 Defining customer engagement policies Description Engagement policies are the set of conditions such as eligibility, applicability, and suitability that qualify an offer or a group of offers for a customer. As a result of engagement rules, customers will see only those offers that the organization believes they should be exposed to. Learning objectives Define engagement policy conditions in Next-Best-Action Designer at the action group level Define engagement policy conditions at the action level Differentiate between eligibility, applicability and suitability conditions 47 Customer engagement policies Introduction Engagement policies are a set of business rules and practices used by the organization to determine which customers are eligible for which Next-Best-Actions. These policies allow you to specify the conditions under which an action or group of actions are eligible for a customer. To learn more about the three types of engagement policy conditions, see Understanding engagement policies. Transcript This video explains the concept of customer engagement policies. The Pega Customer Decision Hub™ combines analytics, business rules, customer data, and data collected during each customer interaction to create a set of actionable insights that it uses to make intelligent decisions. These decisions are known as the Next-Best-Action. Every Next-Best-Action weighs customer needs against business objectives to optimize decisions based on priorities set by the business manager. Typically, the business defines a set of rules that make certain actions available to certain customers. This set of rules is called an engagement policy. 48 As part of an engagement policy, three types of conditions are defined – Eligibility, Applicability, and Suitability. Consider the following examples: a retail bank is promoting a Gold Credit Card; a telco is offering a new iPhone upgrade with an unlimited data plan; and a communications and media company is promoting a new bundle of HD channels. Let’s see how to define engagement policy conditions that will ensure the bank’s Next-Best- Action decisions support these promotions. In Eligibility, you define strict rules for what is legal, and even possible, to offer customers. For example, to be eligible for the Gold Card offer, customers must be 18 years or older. Similarly, for the iPhone upgrade offer, customers are eligible for a new contract only if their old contract ends in less than three months. For the TV channels offer, customers must already own a TV subscription. This offer is not available for customers who have only mobile or landline subscriptions. In Applicability, you specify rules for limiting what to offer based on a customer’s current situation, which is often defined by the products they currently have. These rules are not as rigid as those for Eligibility. 49 For example, a Gold Card is not applicable if the customer already has a higher value card, such as a Platinum Credit Card. If a customer already has a Platinum Card, they might be eligible for the Gold Card, but the Gold Card is not applicable to them. If they ask for it, they may get it, but the business would prefer not to present them with the Gold Card offer. Similarly, with the iPhone upgrade offer, if a customer explicitly expressed in the last survey that they weren’t interested in an iPhone, this action is not applicable to them. For the TV channels offer, the business does not want to advertise HD channels to a customer who has recently bought a set top box that is not capable of HD. In Suitability, you specify conditions that define an offer as appropriate for a customer. Suitability rules are in place to promote the concept of empathy. That is, to help an enterprise be empathetic toward their customers and refrain from making offers that may not be a good fit. For example, as the Gold Card is a high value card, it is only suitable for a customer whose debt-to-income ratio is below a certain threshold. Although a customer might be eligible for it, and the offer might be applicable to them, it would be inappropriate to market it to them, as there is a risk of default. Similarly, an unlimited data plan is not suitable to be offered to a customer with low Internet usage. In the last example, if the customer's favorite TV shows are not available in HD, then it’s not empathetic to offer them the new HD channels package. 50 U+, a retail bank, has configured its engagement policy to suit its own business objectives as well as the needs of its customers. In this scenario, a marketer for U+ has designed 200 actions that could be presented to customers. To select the Next-Best-Actions from these, the Pega Customer Decision Hub first checks eligibility conditions and filters the actions. Then, the applicability conditions are run to filter them further. Next, the suitability conditions are checked to derive the final set of available actions. These actions will go through one final stage before being presented to customers: the arbitration stage. Arbitration is used to prioritize and choose the Next-Best-Actions based on what is relevant for each customer right now. This is decided by considering factors such as AI-calculated propensity, the action value, and various business levers. 51 52 Defining eligibility, applicability, and suitability Rules You define engagement policies in Next-Best-Action Designer. Engagement policies specify the conditions under which an action or group of actions is available for a customer. Configure these policies in the following categories: eligibility, applicability, and suitability, which represent the true nature of the associated conditions. Transcript This demo shows you how to define engagement policy conditions such as eligibility, applicability, and suitability by using Next-Best-Action Designer. U+ Bank, a retail bank, has introduced two new credit cards and wants to offer them to customers based on certain criteria. First, all credit cards are eligible only for existing U+ customers who are at least 18 years old. In addition, the business wants to ensure that these two new credit cards are available for new customers who do not yet have a credit card. Last, the business understands that not all credit cards are suitable for everyone. Because of the credit limits of each card, the business wants to offer the Rewards Card to customers with a credit score higher than 500 and the Rewards Plus Card to customers with a credit score higher than 750. 53 In the Pega Customer Decision Hub™ portal, you define engagement policies in Next-Best- Action Designer. Engagement policies specify the conditions under which an action or group of actions is available for a customer. 54 Now, you set up the engagement policies to reflect U+ Bank’s requirements. You can define the engagement policies for a specific group within an issue and for individual actions. Edit to configure the engagement policies. Notice that the two actions are displayed in this group. As a best practice, use one of the following categories to define your engagement policies: Eligibility, Applicability, and Suitability. These categories represent the true nature of the associated conditions. First, define the group-level eligibility condition to ensure that only customers who are at least 18 years old are considered for the actions. 55 Then, define the group-level applicability condition so that only customers who currently do not have a credit card qualify for the actions. If you wish to reuse a specific condition or a set of conditions in the future, there is an option to save them to a library. This feature helps to avoid recreating identical conditions repeatedly. Saved conditions are available on the Intelligence>Engagement policies tab, where you can modify and prepare them for reuse. The Save to library option is unavailable if the conditions include either strategy Rules or When conditions with parameters. The option is also inactive for eligibility conditions, which include default criteria created with the Use advanced logic option. Lastly, U+ Bank wants different suitability conditions for individual cards depending on the limit. Because the conditions are specific to each credit card, you must define the suitability condition for each at the action level. Open the Rewards Card action. This card comes with a certain credit limit. Therefore, U+ Bank believes that this card is only suitable for customers with a credit score higher than 500, even though customers may satisfy the eligibility and applicability conditions. Thus, define the suitability condition accordingly. 56 Next, open the Rewards Plus Card action. This card has an even higher credit limit than the Rewards card. Thus, this card is suitable for customers with a credit score higher than 750. To complete the configuration, save the changes. Log in as Troy, a 25-year-old chef who became a customer of U+ Bank three months ago, and his credit score is 600. Notice that the Rewards Card offer is displayed because Troy satisfies all eligibility and applicability conditions. Troy receives this card only because his credit score is higher than 500 but lower than 750. 57 Now, log in as Barbara, a 40-year-old engineer who became a customer of U+ Bank one month ago; her credit score is 800. Notice that the Rewards Plus Card offer is displayed because Barbara also satisfies all eligibility and applicability conditions. Barbara, on the other hand, receives offers for both cards because her credit score is higher than 750. You have reached the end of this video. You have learned: How to define group-level engagement policy conditions in Next-Best-Action Designer. How to define action-level engagement policy conditions. How to differentiate between eligibility, applicability, and suitability conditions. 58 Creating and testing personas Description Learn how to create personas and channel contexts in Pega Customer Decision Hub™, and how to use them for next-best-action testing. Explore the reasons behind action filtering through persona-based testing. Examine the results of the persona test cases and analyze how changes in the engagement policies affect the test results. Learning objectives Create personas and channel context. Use personas for next-best-action testing. Create and analyze persona test cases. 59 Testing Next-Best-Action with Persona and Channel Context In Pega Customer Decision Hub™ (CDH), after applying engagement policies and suppression rules to actions, it is necessary to test the settings to ensure that customers receive the expected and desired results. There are two different types of tests that the Decisioning Architect can perform: Next-Best-Action testing in Customer Profile Viewer. Persona testing in Next-Best-Action Designer. Transcript This video shows you how to create Personas and Channel Contexts and how to use them for next best action testing. U+Bank, a retail bank, is cross-selling on the web. The bank wants to test the recent next best action configurations in Customer Profile Viewer. To generate the test results of the next best action strategy framework, the bank needs to create personas and channel context. The dedicated space for creating, updating, and managing the Personas and Channel Contexts in Customer Decision Hub is the Persona Management landing page. A persona is a profile of a fictitious person, representing a group of customers with a similar set of attributes. There are three types of personas you can create in CDH: New persona, where you input the customer properties manually, Persona from file, when creating Personas in bulk, using an external file, Persona from the existing customer. 60 To test the next best action configurations, U+Bank decided to use the Persona created based on existing customer Troy Murphy. Troy is a 26-year-old chef, who has been a customer of the bank for over three months, with a high credit risk. Troy is also considered financially vulnerable. It is a good practice to use such customer information to name your Persona. The descriptive naming convention helps users to recognize the profile faster and to avoid repetitive Persona profiles. Following this rule, you can name the persona created based on Troy’s profile: Young High Risk Financially Vulnerable. When you submit the new persona, the system auto-populates the actual customer information and organizes it in the customer attributes tree, which represents the Customer Insights cache. All the customer details are editable. You use this option to create 61 the persona based on the customer, and then adjust it to specific industry needs. In the Persona profile, you can also add a Persona description and tags. Tags are the labels that you add to personas for the purpose of identification and easier searching. Tags can consider customer properties like Credit risk, Age, and Relationship length in days and so on, as well as engagement policy rules. For example, if the applicability rule is Relationship length in days is =< 90, and Troy's relationship with the bank is 88 days, we can tag him as a new_customer. The tag Financially_vulnerable means that Troy does not meet the suitability condition of Is financially vulnerable=false. Low_debt means that the Debt-to-income ratio for Troy is less than or equal to 48. Personas include only raw information about the customer. Therefore, to run the tests in context, an additional factor that contains information about the Direction, Channel, and 62 Real-time container is necessary. This factor is called Channel context and it can also be created on the Persona Management Landing Page. U+Bank is cross-selling on the web, therefore there is a need to perform a test for the direction Inbound and the channel Web. The real-time container for this channel context is Top_Offers. When you create the channel context, the system automatically displays it on the Channel Context tab of the Persona Management landing page. Creating personas using Pega GenAI features Pega introduces new features that use the power of Pega GenAI™ to simplify your daily tasks in Pega Customer Decision Hub. With Pega GenAI, creating personas is effortless and efficient. When creating a new persona, you can describe the characteristics you want to include in natural language. It will allow you to create a diverse set of personas quickly that accurately represent your target audience. 63 In this case, you can generate customer details by typing your persona characteristics in the text box. Create a mature, low-debt, financially invulnerable customer, who lives in New York. Based on the given details, the system generates the Customer data for the new persona. In this case, the age information provided is automatically set to 45, "low debt" corresponds to a low debt-to-income ratio, and "financially vulnerable" is translated to the "isFinanciallyVulnerable" flag being set to false. 64 The remaining properties are randomly filled and can be reviewed and updated as necessary. Testing next best actions with newly created persona and channel context U+Bank wants to test the next best actions using the newly created Persona and Channel context. The objective is to identify whether any actions have been filtered out for the Young High Risk Financially Vulnerable Persona and determine the reasons why. You can proceed with next best action testing in the Customer Profile Viewer. Search for Persona Young High Risk Financially Vulnerable profile, and on the next best actions tab, make the decision for the Web Inbound Channel context. 65 In the action results table, you can observe that two out of four credit cards were filtered out, which means that they will not be presented to the customer. You can explore the reasons behind this decision by utilizing the "Filtering by feature" option. The action results table has been enhanced with multiple categories that might serve as the underlying cause for excluding certain actions. For instance, actions may be excluded based on eligibility, applicability, suitability, contact policy suppression, or if no treatment has been defined. When the labels in the table columns display "false," it indicates that the action is not excluded and can be presented to the customer. In the case of Persona Young, High Risk, Financially Vulnerable, two actions have been filtered out due to the Suitability condition. The label in the Excluded by suitability column of the action results table indicates ‘true’. Next to the label is an information icon that provides an explanation of the decision. The Rewards Plus card and the Premier Rewards card were excluded because of the suitability condition: Is financially vulnerable=false. Young High Risk Financially Vulnerable Persona is financially vulnerable, he does not meet this condition and therefore, the two actions cannot be presented to him. 66 Additionally, in CPV, another GenAI feature: the AI Insight is available. It provides the summary of the tested configuration. In this example, you receive a clear explanation that there are four candidate offers for the Persona. However, only 2 actions passed, and 2 actions were filtered out due to the Suitability conditions. By clicking on the Polaris icon for each row in the Results column, you can access an explanation of the action results in the Action results explanation window, specific to the action. In this example, you will learn that the RewardsPlusCard is filtered out due to the suitability condition. 67 You have reached the end of this video. What did it show you? - What is Persona and Channel context. - How to create a Persona based on a customer profile. - How to create a Persona using the Pega GenAI feature - How to create a channel context. 68 - How to test the next best actions using Personas. - How AI insights are generated using Pega GenAI. 69 Testing the Engagement Policy configurations with Persona Test Cases In Customer Decision Hub, after applying engagement policies and suppression rules to actions, you need to perform tests to ensure that customers receive the expected and desired results. Next-Best-Action testing in Customer Profile Viewer. Persona testing in Next-Best-Action Designer. Transcript This video shows you how to conduct a persona test to evaluate the next-best-action strategy results. U+ Bank, wants to verify that customers receive the correct offers from the engagement strategy and that the offers are in line with the business requirements of the bank. Persona-based tests use customer personas with specific characteristics to evaluate next- best-action engagement strategy results. To test the configurations, the bank decides to use a Mature Low Risk Financially Invulnerable and a Young Low Risk Financially Vulnerable personas which are created based on customers Barbara and Troy profiles. You can see the attributes of each persona in the Persona Management landing page: Mature Low Risk Financially Invulnerable is a 32-year-old engineer who became a customer of U+ bank a year ago, and her annual income is 14452. Young Low Risk Financially Vulnerable persona is a 26-year-old chef who became a customer of U+ Bank 3 months ago, and his annual income is 13652. 70 With persona-based tests, you verify that the next-best-action strategy gives the expected results. On the Engagement Policy tab of Next-Best-Action Designer, you can create test cases for a specific group or all groups. Running test cases for all groups implies that you run the next-best-action strategy for all issues and groups in your business structure. As the bank wants to target the credit cards group, create a persona test case at the group level. To configure a persona test case, select the action and treatment that you expect the test persona to receive according to your next-best-action strategy. In this case , Young Low Risk Financially Vulnerable Persona is supposed to receive the Standard card and Rewards card actions. In the Persona (Data transform) field, select the persona against which you want to test the strategy. Select Young Low Risk Financially Vulnerable. 71 In the Channel context field, select WebInbound channel context, which defines the Direction, channel and the Real-time container for the test. In the Next-Best-Action scope section, select whether the test checks only the engagement policy configuration or includes additional elements such as constraints and arbitration. Selecting Engagement policies only validates that your policy conditions provide the desired results. The selection ensures that the test looks at the eligibility, applicability, and suitability. Selecting Engagement policies and arbitration validates the effectiveness of your policies when you arbitrate across all actions. The test considers arbitration, adaptive analytics, treatment and channel processing, and constraints. In this case, select Engagement policies only. Mature Low Risk Financially Invulnerable Persona is eligible for the Rewards Plus card and the Premier Rewards card. Create a test case for this persona. Run the tests to confirm that they pass. The bank now wants to offer the Standard card to customers who have an annual income greater than 14000. See how this engagement policy change affects these test results. To define an engagement policy, click Standard card. Define a suitability condition so that only customers who have a minimum annual income of 14000 qualify for the actions. Run both tests to see how this engagement policy configuration affects the test cases. Observe that Young Low Risk Financially Vulnerable persona fails the test. The failure means that customers with a profile similar to Troy does not satisfy all the existing engagement policies. Analyze the results to see why the test did not give the expected outcome. Based on the report, Young Low Risk Financially Vulnerable persona is not eligible for the Standard card. 72 Young Low Risk Financially Vulnerable persona’s annual income is 13652, which is less than the defined applicability condition. Mature Low Risk Financially Invulnerable persona’s annual income is 14452, which is higher than the defined applicability condition. Although the Young Low Risk Financially Vulnerable persona fails the test, the Mature Low Risk Financially Invulnerable persona passes it. Now, edit the test for the Young Low Risk Financially Vulnerable persona to delete the Standard card assertion, and then run the test. You can design the personas according to the business requirements and test them every time an engagement policy changes. You have reached the end of this video. What did it show you? - How to create persona test cases. - How engagement policy changes affect the persona test results. - How to edit persona test cases. 73 Avoiding overexposure of actions Description Learn about contact policies and how they are used in Pega Customer Decision Hub. Gain experience defining contact policy rules to suppress actions after a specific number of outcomes. Learning objectives Describe contact policy requirements Explain how to define contact policy rules to suppress a single action or group of actions for a limited time period 74 Contact policies Understanding contact policy requirements Too many contact attempts over a short period of time can have a negative impact on a customer's attitude toward further actions by your company. To maximize the lifetime value of every customer relationship, organizations must prevent outreach fatigue by optimizing the number of actions taken. In the Pega Customer Decision Hub, contact policies allow you to suppress actions after a specific number of outcomes. Suppressing or pausing an action prevents oversaturation by limiting the number of times a customer is exposed to the same action. Defining contact policies Contact policies determine when and for how long an Action or group of Actions should be shown to a customer. Contact policies track responses to Actions over a specific period of time, allowing you to implement rules such as the following: Do not show an ad to a customer for two weeks if the customer ignores the ad five times in a one-week timeframe. Note: If outcomes are tracked for an individual action, then the action is not shown once the suppression criteria are met. Do not show a group of ads for six months if a customer clicks on any ad in the group 3 times over a period of 30 days. Note: If outcomes are tracked for all actions in the group, then all of these actions are not shown once the suppression criteria are met. An Interaction History Summary rule is used to determine the number of impressions and clicks generated by a customer over a period of time. The default time periods are 7 and 30 days. There might be business requirements to track a customer’s response to an offer over different time periods, for example, 14 days. 75 You can add more tracking periods by creating a new Interaction History Summary rule for the required time period and then updating the part of the Next-Best-Action strategy that references it. 76 Defining action suppression rules Introduction Suppression rules determine when and for how long an action or group of actions should not be shown to a customer. These suppression rules put an action on hold after a specific number of outcomes are recorded for some or all channels. Transcript This demo will show you how to suppress a single action or group of actions for a limited time period. U+, a retail bank, currently displays various credit card offers to each customer who logs in to the website. For example, every time Troy logs in to his accounts page, a credit card offer is shown. Sometimes the same offer is shown multiple times. For a limited time period, the bank wants to automatically suppress offers that are shown or clicked too often. In this scenario, the bank has two requirements. First, do not show a credit card for ten days if the card was shown three times in the last seven days. Second, do not show any credit cards for ten days if a user has clicked on a credit card five times in the last seven days. Contact policies are used to implement these business requirements. You create contact policies in Next-Best-Action Designer. 77 On the Constraints tab, you can define the suppression rules by creating contact policy rules. For the first requirement, showing a credit card a maximum of three times, configure a contact policy to track Impressions at the Action level. Provide a name for the contact policy. Then select the type of outcome that will be tracked by the contact policy, in this case Impressions. You can specify whether the responses are tracked for one specific action, or for all actions in the group. Track the first requirement at the Action level, since you want to show one specific card a maximum of three times. You can select the time period over which the responses should be tracked. In this case, responses should be tracked over a period of seven days. The newly configured contact policy has been added. The first business requirement is to suppress the action for ten days if there are three impressions for the web treatment, so fill in the details accordingly. Enter the number of responses required to fulfill the suppression criteria. Select the channel for which the responses are tracked. Note that if you want to track impressions across multiple channels, you can select Any. 78 Enter the number of days for which an action should be paused after the suppression criteria are met. The next business requirement is to suppress the entire group of actions if there are five clicks for web treatments. The first contact policy is configured to track Impressions, so add another contact policy. For this requirement you will be tracking Clicks for all actions in the group. This because you want to hide all credit cards if there are five clicks on any one credit card. Once the contact policy is created, fill in the suppression rule details. If there are five clicks on web treatments, suppress the group of actions for ten days. Save the changes. The contact policy rules are reusable as policy rules across all business issues and groups. As the bank wants to suppress credit card offers, open the CreditCards group. Add the contact policy rules you just created. With that, all the necessary configuration for this scenario is complete. Save the changes. When customer Troy logs in to his accounts page, the Premier Rewards Card offer is displayed. 79 After showing him this offer three times, it is automatically suppressed, and a different credit card offer is shown. When customer Barbara logs in to her accounts page, a credit card offer is displayed. After clicking on any credit card offer five times, the credit card offers are not shown again. This demo has concluded. What did it show you? - How to define contact policy rules to suppress a single action or group of actions. 80 Arbitrating between actions Description After applying engagement policy rules, a customer may still qualify for more than one action. Learn how every Next-Best-Action weighs customer needs against business objectives to optimize decisions based on priorities set by the business. Learning Objectives Describe what action arbitration is and how it works Explain how customer needs and business objectives are considered during arbitration Prioritize actions based on AI Prioritize actions based on AI & business levers 81 Action arbitration Pega Customer Decision Hub™ (CDH) combines analytics, business rules, customer data, and data collected during each customer interaction to create a set of actionable insights that CDH uses to make intelligent decisions. Arbitration aims to balance customer relevance and business priorities by weighing numerical values for the following factors: propensity, context weighting, business value, and business levers. Learn to create a simple formula for arriving at a prioritization value, which is used to select the top actions. Transcript This video explains the concept of action arbitration in Pega Customer Decision Hub. Pega Customer Decision Hub combines analytics, business rules, customer data, and data collected during each customer interaction to create a set of actionable insights that it uses to make intelligent decisions. These decisions are known as Next-Best-Actions. Every Next- Best-Action weighs customer needs against business objectives to optimize decisions based on priorities set by the business manager. U+, a retail bank, wants to avoid offering random actions to its customers, so it uses Pega CDH to rank and select the next best actions for every customer. All the actions from the Bank’s offer move through several steps that whittle them down until the Next Best Action is selected. 82 The process starts with the catalog full of actions that the organization can offer to its customers. These actions belong to various issues and groups. The first step is to apply the Engagement Policies: Eligibility, Applicability, and Suitability conditions. Eligibility rules are used to determine whether customers are eligible for an action. Users must meet the condition for CDH to consider the action eligible. An example of an eligibility condition is the customer’s age. A customer can be offered a credit card only if they are 18 years old or older. Applicability rules are used to limit what to offer, based on a customer’s current situation. For example, the bank wants to show a retention offer, instead of a credit card offer, to customers who are likely to churn in the near future. Suitability rules are used to define whether an offer is appropriate for a customer. For example, if a customer is in debt and has a lot to pay off, the bank does not want to offer them a 30-year mortgage as it would not be empathetic. 83 The initial catalog of actions has been narrowed down by engagement policies. These engagement policies are a combination of business rules, for example, certain age requirements for a loan or some product compatibility rules, and predictive models which help predict customer behavior as customer churn or probability of default. These predictive models are created by data scientists in Pega, or other tools familiar to data scientists like Python, R, and H2O.AI. Before being presented to customers, the remaining actions go through a final stage known as the arbitration stage. This stage focuses on selecting and prioritizing the best actions that are most relevant for the customer at the present time. Arbitration balances customer relevance with business priorities using four components: Propensity, Context Weighting, Business Value, and Business Levers, each represented by numerical values. 84 Propensity is the predicted likelihood of positive behavior, such as the likelihood of a customer accepting an offer. The propensity value is calculated by AI and it is the foundation of the arbitration process. The higher the likelihood of a customer accepting an offer, the higher the Propensity value for that offer. For Example, when a customer is eligible to receive a Standard Credit Card and clicks the Standard Credit card offer on the Bank’s website the propensity of this action increases. 85 The AI is driven by adaptive models, self-learning mathematical models that use machine learning to make calculations. Adaptive models are a sub-group of predictive models that continuously adapt to new data as it becomes available. This means that the models are updated incrementally in real-time based on incoming data. The Customer Decision Hub is configured to calculate the propensity for each treatment. For the web treatment, Web_Click_Through_Rate is the out-of-the-box adaptive model. It predicts the likelihood of a customer clicking on a banner, which is considered a positive behavior. Context Weighting allows Pega Customer Decision Hub to consider the situational context for each action. For example, if a customer contacts the bank to close their account, the highest-priority action is to ensure that the customer is retained, even if they are eligible for other offers. Business Value enables you to assign a financial value to an action and prioritize high- value actions over low-value ones. This value is typically normalized across Issues and Groups. For example, a 25-year mortgage is more profitable than a 15-year mortgage. So, in a situation where a customer is eligible for both plans, the 25-year mortgage will be ranked higher because of its higher business value. Business Levers allow the business to assert some level of control over the prioritization of actions defined within the system. Levers are used to manually nudge Customer Decision Hub toward Next-Best-Actions based on external factors. For example, the recommended Next-Best-Action might be to offer a credit card to a customer when they visit the home page. However, to meet a business goal, the Mortgage Line of Business favors a mortgage offer, even if that offer is ranked a little lower on the list of possible actions. Pega AI considers all four components to select the top offer for a customer. A simple formula P*C*V*L is used to arrive at a prioritization value, which is used to select the top actions. The action with the highest priority will be presented to a customer. In the current example, there are 5 actions that made it until the arbitration phase. 86 Assume the AI generates a propensity value for each of the actions which is a value between 0 and 1. Each action has a context weight set, which is a percentage value between 0% and 100%, business value in dollars, and business lever value in a percentage between 0% and 100%. Using the priority formula PCVL, with all of these values taken into account, we end up with a computed priority. Finally, the action with the highest priority is selected. In this case, it is a Standard Credit Card. In summary, arbitration is used to select and prioritize the best actions that are most relevant for the customer at the present time. Arbitration balances customer relevance with business priorities using the formula P*C*V*L. The top actions are selected based on the result of this multiplication – the prioritization value. 87 Prioritizing actions with business levers Introduction Often, due to an internal ad-hoc priority, the business would like to boost the chance of certain actions being selected. To achieve this, they would like to present more relevant offers to customers based not only on their behavior but also on business priorities. Learn how to include business requirements in an action prioritization calculation to boost the chance of an action being selected. Transcript This video will show you how to include business requirements in an action prioritization calculation to boost the chances of an action being selected. U+, a retail bank, noticed that one of the offers, the Rewards card offer, was not presented frequently enough due to its low propensity because customers ignored it during the initial launch. For example, Troy, a customer, qualifies for two credit card offers – the Standard Card and the Rewards Card. When he logs in to the bank’s website, he sees the top offer for him, Standard Card. Now, due to an internal ad-hoc priority, the bank wants to boost the chances of the Rewards Card being selected as the top offer. That is, the bank would like to present more relevant offers to customers based on not only their behavior but also on business priorities. 88 To implement this requirement, you must first enable the Action Weighting, a Business Lever, in Next-Best-Action Designer’s Arbitration equation. This ensures that an action’s business weight is used in the priority value calculation. In this case, the bank wants to boost the Rewards Card. So, open the Rewards Card offer. Edit the offer to set a business weight, a value in percentage, that is required to boost the offer. In this case, U+ wants to increase the changes of this action selected by 40%. Save the offer for the changes to take effect. Now, when Troy logs into the website, he will see that Rewards card is the top offer. In Customer Profile Viewer, you can examine the detailed results of the total lever weight after your updates, for the customer Troy. 89 First, view the next-best-action decision to learn more about the details of the actions for which Troy qualifies. For the current use case, the direction is Inbound, and the channel is the Web. TopOffers is the real-time container service that manages communication between Customer Decision Hub and the website of the bank. When you request a decision for Troy, the Customer Profile Viewer shows you the offers for which Troy is eligible. In the Explain prioritization view, you can see how the final Priority is computed, based on 4 factors: Propensity, Context, Business Value, Business levers. In this specific example, you can see how the Total lever weight influences the ranking. Even though the Final Propensity is higher for the Standard Card, the Rewards Card is ranked 1. This is caused by the Total Lever Weight factor, which is now 1.4 for the Rewards Card because the lever for this card was increased by 40%. This demo has concluded. What did it show you? How to include business requirements in action prioritization calculation. How to boost an action using business weight. 90