Descriptive Statistics PDF
Document Details
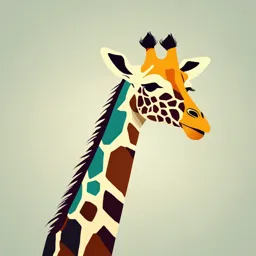
Uploaded by LovableUkulele9075
De La Salle Medical and Health Sciences Institute
Tags
Summary
This document presents an overview of descriptive statistics, encompassing methods of summarizing and analyzing numerical data. It covers measures of central tendency (mean, median, mode), measures of variability, and techniques for data presentation. The document also includes worked examples and tables.
Full Transcript
Descriptive Statistics Learning Objectives: At the end of the session, the participants shall be able to: 1. Determine ways of summarizing data using descriptive statistics 2. Explain the purpose of descriptive statistics and its role in summarizing data 3. Define and perform calculatio...
Descriptive Statistics Learning Objectives: At the end of the session, the participants shall be able to: 1. Determine ways of summarizing data using descriptive statistics 2. Explain the purpose of descriptive statistics and its role in summarizing data 3. Define and perform calculation of the different descriptive statistics 4. Interpret and communicate the insights derived from descriptive statistics in the context of real-world data THE DOMAIN OF THE SCIENCE OF STATISTICS Descriptive Statistics: Data Summarization: Measure of central tendency Measures of location Measures of variability Data Presentation: Narratives Tabular Presentation Graphical Presentation Measures of Central Tendency A measure of central tendency is a typical value or a representative value of a set of data. Measure of central tendency provides a very convenient way of describing a set of scores with a single number that describes the PERFORMANCE of the group. It is also defined as a single value that is used to describe the “center” of the data. There are three common measures of central tendency: Mean, Median, Mode MEAN (𝑥̅) It is the most commonly used measure of the center of data It is also referred as the “arithmetic average” ∑ 𝑥𝑖 ∑ 𝑓𝑥 𝑖 𝑥̅ = 𝑥̅ = ∑𝑓 𝑁 Example Scores of 15 students in Mathematics I quiz consist of 25 items. The highest score is 25 and the lowest score is 10. Here are the scores: 25, 20, 18, 18, 17, 15, 15, 15, 14, 14, 13, 12, 12, 10, 10. Find the mean in the following scores. ∑ 𝑥𝑖 𝑥̅ = 𝑁 𝑥̅ = 25+20+18+18+17+15+15+15+14+14+13+12+12+10+10 15 𝒙! = 𝟏𝟓. 𝟐 Interpretation: The average performance of 15 students who participated in mathematics quiz consisting of 25 items is 15.20. The implication of this is that student who got scores below 15.2 did not perform well in the said examination. Students who got scores higher than 15.2 performed well in the examination compared to the performance of the whole class. Example Find the Grade Point Average (GPA) of Mr. Cruz for the first semester of the school year 2023-2024. Use the table below: Subjects Grade Unit BM112 1.25 3 BM102 1.00 3 AC103 1.25 6 EC111 1.00 3 MG101 1.50 3 MK101 1.25 3 FM111 1.50 3 PE2 1.00 2 Subjects Grade (𝒙𝒊) Unit (𝒇) 𝑓 (𝑥𝑖) BM112 1.25 3 3.75 BM102 1.00 3 3.00 AC103 1.25 6 7.50 EC111 1.00 3 3.00 MG101 1.50 3 4.50 MK101 1.25 3 3.75 FM111 1.50 3 4.50 PE2 1.00 2 2.00 ' 𝑓 = 𝟐𝟔 ' 𝑓 (𝑥𝑖) = 𝟑𝟐. 𝟎𝟎 ∑ 𝑓𝑥𝑖 32.00 𝑥̅ = 𝑥̅ = ∑𝑓 26 𝑥̅ = 1.23 Properties of the Mean Itmeasures stability. Mean is the most stable among other measures of central tendency because every score contributes to the value of the mean. The sum of each score’s distance from the mean is zero. It may easily affected by the extreme scores. It can be applied to interval level of measurement. It may not be an actual score in the distribution. It is very easy to compute. When to Use the Mean Sampling stability is desired. Other measures are to be computed such as standard deviation, coefficient of variation and skewness. MEDIAN Median is what divides the scores in the distribution into two equal parts. Fifty percent (50%) lies below the median value and 50% lies above the median value. It is also known as the middle score or the 50th percentile. Steps in Solving Median of Ungrouped Data 1. Arrange the scores (from lowest to highest) 2. Determine the middle most score in a distribution if n is an odd number and get the average of the two middle most scores if n is an even number. Example: Find the median score of 7 students in an English class. 19, 16, 2, 10, 15, 5, 17 2 5 10 15 16 17 19 Example Find the median score of 8 students in an English class. 19, 16, 2, 10, 15, 5, 17, 30 2 5 10 15 16 17 19 30 15+16 = 2 = 𝟏𝟓. 𝟓 Properties of the Median It may not be an actual observation in the data set. It can be applied in ordinal level. It is not affected by extreme values because median is a positional measure. When to Use the Median The exact midpoint of the score distribution is desired. There are extreme scores in the distribution. MODE The mode or the modal score is a score or scores that occurred most in the distribution. It is classified as unimodal, bimodal, trimodal or multimodal. Unimodal is a distribution of scores that consists of only one mode. Bimodal is a distribution of scores that consists of two modes. Trimodal is a distribution of scores that consists of three modes or multimodal is a distribution of scores that consists of more than three modes. Example Scores of 10 students in Section A, Section B and Section C. Section A Section B Section C 25 25 25 24 24 25 24 24 25 20 20 22 20 18 21 20 18 21 16 17 21 12 10 18 10 9 18 7 7 18 The score that appeared most in Section A is 20, hence, the mode of Section A is 20. There is only one mode, therefore, score distribution is called unimodal. The modes of Section B are 18 and 24, since both 18 and 24 appeared twice. There are two modes in Section B, hence, the distribution is a bimodal distribution. The modes for Section C are 18, 21, and 25. There are three modes for Section C, therefore, it is called a trimodal distribution. Properties of the Mode It can be used when the data are qualitative as well as quantitative. It may not be unique. It is affected by extreme values. It may not exist. When to Use the Mode When the “typical” value is desired. When the data set is measured on a nominal scale. Measures of Central Location Tell where a specific data value falls within the data set or its relative position in comparison with other data values. The most common measures of position are quartiles, deciles, and percentiles. Quartiles Q1, Q2, Q3 divides ranked scores into four equal parts Q1 Q2 Q3 Example: Find the quartiles Q1, Q2, and Q3 of the following data 20, 30, 25, 23, 22, 32, 36. Solution: Arrange data in ascending form, and n = 7 20 22 23 25 30 32 36 Example: Find the quartiles Q1, Q2, and Q3 of the ffg. data 20, 30, 25, 23, 22, 32, 36, 18 Solution: Arrange data in ascending form, and n = 8 18 20 22 23 25 30 32 36 Deciles D1, D2, D3, D4, D5, D6, D7, D8, D9 divides ranked data into ten equal parts Percentiles P1, P2, P3, P4, P5, P6, P7, P8,… P99 divides ranked data into one hundred equal parts 99 Percentiles or 𝑖th Percentiles (for a given number 𝑖) Quartiles, Deciles, Percentiles FRACTILES partitions data into approximately equal parts Measures of Variability Measures of variability describe the spread or the dispersion of a set of data. A measure of variability usually accompanies a measure of central tendency as basic descriptive statistics for a set of scores. Central Tendency vs Variability Central tendency describes the central point of the distribution, and variability describes how the scores are scattered around that central point. Together, central tendency and variability are the two primary values that are used to describe a distribution of scores. Variability Variability serves both as a descriptive measure and as an important component of most inferential statistics. As a descriptive statistic, variability measures the degree to which the scores are spread out or clustered together in a distribution. In the context of inferential statistics, variability provides a measure of how accurately any individual score or sample represents the entire population. Variability When the population variability is small, all of the scores are clustered close together and any individual score or sample will necessarily provide a good representation of the entire set. On the other hand, when variability is large and scores are widely spread, it is easy for one or two extreme scores to give a distorted picture of the general population. Common Measures of Variability ◦ Range ◦ Interquartile Range ◦ Mean Absolute Deviation ◦ Variance ◦ Standard Deviation ◦ Z scores ◦ Coefficient of Variation Range The difference between the largest and the smallest values in a set of data 𝑅𝑎𝑛𝑔𝑒 = 𝐻𝑉 − 𝐿𝑉 Example: Find the Range of the given set of data. 35, 37, 37, 39, 40, 40, 41, 41, 43, 43, 43, 43, 44, 44, 44, 44, 44, 45, 45, 46, 46, 46, 46, 48 𝑅 = 48 − 35 = 𝟏𝟑 Interquartile Range Range of values between the first and third quartiles Range of the “middle half” Less influenced by extremes Example: Find the interquartile quartile of the following data 20, 30, 25, 23, 22, 32, 36. 𝐼𝑅 = 𝑄3 − 𝑄1 = 32 − 22 𝐼𝑛𝑡𝑒𝑟𝑞𝑢𝑎𝑟𝑡𝑖𝑙𝑒 𝑅𝑎𝑛𝑔𝑒 = 𝟏𝟎 Mean Absolute Deviation Average of the absolute deviations from the mean ∑𝑥– 𝜇 𝑀. 𝐴. 𝐷. = 𝑁 Example: Find the mean absolute deviation of the following data 20, 30, 25, 23, 22, 32, 36. 𝜇 = 26.86 34.86 𝑀. 𝐴. 𝐷. = 7 𝑴. 𝑨. 𝑫. = 𝟒. 𝟗𝟖 Population Variance Average of the squared deviations from the arithmetic mean ∑(𝑥 – 𝜇) 2 𝜎2 = 𝑁 Population Standard Deviation Square root of the variance ∑(𝑥 – 𝜇) 2 𝜎= 𝑁 Example: Find the population variance and population standard deviation of the following data 20, 30, 25, 23, 22, 32, 36. 𝜎 2 = 29.84 𝜎 = 5.46 Sample Variance Average of the squared deviations from the arithmetic mean ∑(𝑥 –𝑥̅ )2 𝑆2 = 𝑛–1 Sample Standard Deviation Square root of the variance ∑(𝑥 –𝑥̅ )2 𝑆= 𝑛–1 Example: Find the sample variance and sample standard deviation of the following data 20, 30, 25, 23, 22, 32, 36. 𝑆2 = 34.81 𝑆 = 5.90 Z - Score A z-score represents the number of standard deviations a data value falls above or below the mean. It is used as a way to measure relative position. Z-Score Formula (value − mean) z − score = st.deviation x−x z= s Please round z − scores to 2 decimal places. Can a z-score be negative? YES A positive z-score means that a score is above the mean. A negative z-score means that a score is below the mean. A z-score of 0 means that a score is the exact same as the mean. Example A student scored a 65 on a math test that had a mean of 50 and a standard deviation of 10. She scored 30 on a history test with a mean of 25 and a standard deviation of 5. Compare her relative position on the two tests. Math: z = (65-50)/10= 15/10 = 1.5 History: z = (30-25)/5 = 5/5 = 1 The student did better in math because the z-score was higher. Example Find the z-score for each test and state which test is better. Test A: x= 38 x= 40 s= 5 Test B: x = 94 x = 100 s = 10 Test A: z = (38-40)/5 = -0.4 Test B: z = (94-100)/10 = -0.6 Which is higher? Test A is higher, therefore it is better. It has a higher relative position. Example A sample has a mean of 200 and a standard deviation of 25. Find the value of x that corresponds to a z-score of 2.35. z= (x − x) s x − 200 2.35 = 25 x − 200 = (2.35)(25) x − 200 = 58.75 x = 258.75 Coefficient of Variation Ratio of the standard deviation to the mean, expressed as a percentage Measurement of relative dispersion Example: Find the coefficient of variation of the following data 20, 30, 25, 23, 22, 32, 36. σ 𝐶. 𝑉. = 100 𝜇 𝐶. 𝑉. = 21.96% Measures of Shape Skewness ◦Absence of symmetry ◦Extreme values in one side of a distribution Kurtosis ◦Peakedness of a distribution Skewness Coefficient of Skewness 3( − M d ) Sk = If Sk < 0, the distribution is negatively skewed (skewed to the left). If Sk = 0, the distribution is symmetric (not skewed). If Sk > 0, the distribution is positively skewed (skewed to the right). Example Kurtosis Peakedness of a distribution ◦ Leptokurtic: high and thin ◦ Mesokurtic: normal in shape ◦ Platykurtic: flat and spread out Data Presentation 1. Narratives 2. Tabular Presentation 3. Graphical Presentation Data Presentation: Examples of One Way Tables Table 1. Distribution of Patients Table 2. Distribution of Patients According to BMI According to COVID-19 Severity BMI Number Percentage COVID-19 Number Percentage SEVERITY Underweight 18 3.0 Normal 235 39.8 Mild 401 67.7 Overweight 201 33.9 Moderate 151 25.5 Obese 138 23.3 Severe 40 6.8 Total 592 100.0 Total 592 100.0 Example of Cross Tabulation Table 3. Distribution of Patients According to BMI and COVID-19 Severity BMI COVID-19 Severity Total Mild Moderate Severe Underweight 18 0 0 18 Normal 210 20 5 235 Overweight 128 68 14 201 Obese 45 63 30 138 Total 401 151 49 592 Example of Graphs: (Bar Graph) Figure 1. TB Cure Rates (in %) of Provinces in Region Y: 2014 Example of Graphs: (Line Graph) Figure 2. TB Cure Rates (in %) of Figure 3. TB Cure Rates (in %) of Municipality X: 2008 - 2012 Municipality X: 2008 - 2012 Example of Graphs: (Pie Chart) Figure 4. Percentage Distribution of Notified TB Cases According to Type Credits to CHED seminar facilitators for most of the contents on the slides. THANK YOU..