Descriptive Statistics PDF
Document Details
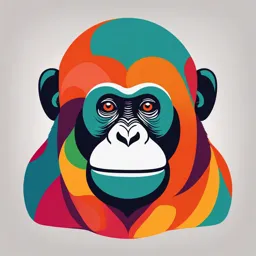
Uploaded by SofterRutherfordium7525
Cairo University
Tags
Summary
This document provides an overview of descriptive statistics, including measures of central tendency (mean, median, mode, quartiles) and measures of variation (range, interquartile range, standard deviation, variance, coefficient of variation). It explains how to use these measures in different contexts, such as data analysis and quality control.
Full Transcript
Descriptive Statistics ( What happened ? ) Descriptive Statistical : is the statistical methods that can be used to summarize data and audit التاكد من جوده البياناتthe data. Descriptive Statistics Measures...
Descriptive Statistics ( What happened ? ) Descriptive Statistical : is the statistical methods that can be used to summarize data and audit التاكد من جوده البياناتthe data. Descriptive Statistics Measures of Central Tendency Mean (μ) : The average of a set of values.\ Median: The middle value when data is ordered. Mode: The most frequently occurring value in a dataset. Quartiles (Q1, Q2, Q3): Descriptive Statistics 1 Q1 (First Quartile): The value that separates the lowest 25% of the data. Q2 (Median): The second quartile, which separates the lower 50% of the data. Q3 (Third Quartile): The value that separates the lowest 75% of the data. The importance of using the measure of central tendency to check data Summarize data Understanding data Compatibility of the data with reality Comparison Study the effect of case variation on the target variable Explains in Diagnostic Identify outlier values For clustering For modeling Measures of Variation Measures of variation describe the spread and dispersion of data points within a dataset. Understanding these variations helps us analyze and interpret data effectively. Statistical Measures all of Measures of Variation ≥ 0 ( ، كان التشتت أقل،هذه المقاييس تشير إلى أنه كلما كانت النتيجة أقرب إلى الصفر مما يدل على تجانس البيانات. ) 1. Range Descriptive Statistics 2 When to Use: To quickly assess the span between the lowest and highest values in a dataset. How to Use: Calculate it when you need a simple overview of the data spread, such as in initial data analysis. 2. Interquartile Range (IQR) When to Use: When you want to understand the dispersion of the middle 50% of your data, especially to identify outliers. How to Use: Use IQR in box plot creation or when comparing the spread of multiple datasets. 3. Standard Deviation When to Use: To determine how much individual data points deviate from the mean, providing insights into variability. How to Use: Apply it in contexts where understanding data consistency is crucial, such as in quality control or finance. 4. Variance When to Use: Similar to standard deviation, but useful for theoretical calculations in statistical models and analyses. How to Use: Use variance when performing operations that require squared variability, or in formal statistical tests. 5. Coefficient of Variation (CV) What it is: The coefficient of variation (CV) is a statistical measure that expresses the ratio of the standard deviation to the mean of a dataset. It is used to indicate the relative variability or dispersion of the data relative to its average value. The CV is usually expressed as a percentage, making it easier to compare variability across different datasets or distributions. Descriptive Statistics 3 When to Use: Comparing Datasets: Use CV when you want to compare the degree of variation between two or more datasets that have different units or significantly different means. Risk Assessment in Finance: Apply CV to assess the risk of investments by comparing the standard deviation of returns with the average return; a higher CV indicates higher risk relative to return. Quality Control: Utilize CV in manufacturing or quality assurance processes to evaluate the consistency of measurements or product attributes. Data Standardization: When dealing with homogenous data sets, CV aids in understanding variability in a standardized way. In summary, CV is a valuable tool for assessing and comparing the relative variability of data, particularly useful in contexts like finance and quality control. 6. Variation Ratio ( كيف ُت حسب نسبة التباينVariation Ratio) ? نسبة التباين تحسب نسبة القيم التي لا تنتمي إلى الفئة الأكثر شيوًع ا أو تكراًر ا ( الفئة الأكثر تكراًر ا ُت سمى المنوال- Mode). تفسير نسبة التباين فهذا يعني أن نسبة كبيرة من القيم تنتمي،0 إذا كانت نسبة التباين قريبة من وبالتالي يكون التشتت قلياًل،إلى الفئة الأكثر تكراًر ا. فهذا يعني أن معظم القيم لا تنتمي إلى،1 إذا كانت نسبة التباين قريبة من وبالتالي يكون التشتت أكبر،الفئة الأكثر تكراًر ا. The "Variation Ratio" is a measure used only with categorical data and is not used with quantitative data. الخالصة: كلما كانت.نسبة التباين تساعد في فهم مدى تركز البيانات حول الفئة الأكثر تكراًر ا مما يعني أن عدًد ا كبيًر ا من القيم ينتمي إلى الفئة، كان التشتت أقل،النسبة أصغر الأكثر شيوًع ا. Descriptive Statistics 4 7. Measures of position a. Percentiles: These indicate the value below which a given percentage of observations falls. For example, the 25th percentile (Q1) is the value below which 25% of the data points lie. b. Quartiles: These are specific percentiles that divide the data into four equal parts. The first quartile (Q1) represents the 25th percentile, the median (Q2) is the 50th percentile, and the third quartile (Q3) is the 75th percentile. c. Deciles: These are similar to quartiles but divide the dataset into ten equal parts. Each decile represents 10% of the data. d. Z-scores: This measure indicates how many standard deviations a value is from the mean. It helps to understand how unusual or typical a value is in the context of the dataset. e. Standard Scores: These are various measures that standardize values to allow comparisons across different datasets or distributions. f. Interquartile Range (IQR): This measure represents the range between the first and third quartiles (Q3 - Q1) and is useful for understanding the spread of the middle 50% of the data. Understanding these measures helps in analyzing data distributions, identifying outliers, and making informed decisions based on relative standings of values. 8. Skewness & Kurtosis Skewness Descriptive Statistics 5 Skewness > 0 Skewness = 0 Skewness < 0 الرسوم البيانية .التوزيع المائل إيجابًي ا :يظهر تأثير القيم العالية .التوزيع المتناظر :يعكس توازن البيانات .التوزيع المائل سلبًي ا :يظهر تأثير القيم المنخفضة Kurtosis كل ما اتضغط يكون تشتت البيانات اكبر وكل ما علي زاد تركيز البيانات في مكان واحد )Visualization (Box Plots Descriptive Statistics 6 أو مخطط الصندوق هو أداة رسومية ُت ستخدم لعرض توزيع مجموعة من Box Plot البياناتُ.ي ظهر هذا المخطط معلومات مهمة مثل الوسيط ،والرباعيات ،والقيم الشاذة. Box Plot:إليك مكونات (Median):الوسيط 1. ُ.ي مثل الخط العمودي داخل الصندوقُ.ي قسم البيانات إلى نصفين متساويين (Quartiles):الرباعيات 2. .يمثل %25من البياناتُ.ي حدد الحد الأدنى للصندوق ):الرباعية الأولى( Q1 .يمثل %75من البياناتُ.ي حدد الحد الأقصى للصندوق ):الرباعية الثالثة( Q3 Descriptive Statistics 7 Box Plotكيفية قراءة Q3.و Q1الصندوق :يمثل نطاق البيانات بين .الخط العمودي داخل الصندوق :يمثل الوسيط تمتد من الصندوق إلى القيم القصوى التي لا ُت عتبر (Whiskers):الخطوط الأفقية .شاذة النقاط خارج الصندوق :تمثل القيم الشاذة التي قد تشير إلى تباين كبير في .البيانات Box Plotأهمية ُ.ي ساعد في فهم توزيع البيانات بشكل سريع ُ.ي ظهر التباين والتماثل في البيانات ُ.ي ستخدم في مقارنة مجموعات بيانات متعددة .هو أداة فعالة لتحليل البيانات وتقديم رؤى سريعة حول توزيعها ، Box Plotباختصار أنواع البيانات( Data Types and Analysis )والتحليل Descriptive Statistics 8 Numbers ( )الأرقامare generally classified as: 1. Continuous Examples: Height, weight Includes decimal values ()توجد أرقام عشرية 2. Discrete Examples: Goals, number of people No decimal values ()لا توجد أرقام عشرية Data Analysis Types & Actions Descriptive Statistics 9 1. Descriptive Analysis ()التحليل الوصفي Question: What happened? Purpose: Summarizes past data to understand events or trends. 2. Diagnostic Analysis ()التحليل التشخيصي Question: Why did it happen? Purpose: Identifies causes and factors behind past events. 3. Predictive Analysis ()التحليل التبوئية Question: What is likely to happen? Purpose: Forecasts future outcomes based on patterns and data. 4. Prescriptive Analysis ()التحليالت الإرشادية Question: What action should we take? Purpose: Recommends next steps to optimize outcomes. Levels of Measurement ()مستويات القياس 1. Categorical Descriptive Statistics 10 Nominal Characteristics ()خصائصها: Shared traits for classification ()حاجات مشتركة في التصنيف Different variable values ()قيم مختلفة No ranking order ()لا يوجد ترتيب أفضلية No numerical values ()لا توجد أرقام Example: Categories like gender, types of fruits, etc. Ordinal Characteristics ()خصائصها: Shared traits for classification ()حاجات مشتركة في التصنيف Different variable values ()قيم مختلفة Ranking order exists ()يوجد ترتيب أفضلية No numerical values ()لا توجد أرقام Descriptive Statistics 11 Example: Grading system (A, B, B+), satisfaction levels (low, medium, high). 2. Quantitative Interval Characteristics ()خصائصها: Shared traits for classification ()حاجات مشتركة في التصنيف Different variable values ()قيم مختلفة Ranking order exists ()يوجد ترتيب أفضلية Numerical values ()توجد أرقام Zero represents a value, not the absence of something ()الصفر له قيمة. Example: Body temperature of 0°C, academic score of 0. Descriptive Statistics 12 Ratio Characteristics ()خصائصها: Shared traits for classification ()حاجات مشتركة في التصنيف Different variable values ()قيم مختلفة Ranking order exists ()يوجد ترتيب أفضلية Numerical values ()توجد أرقام Zero represents the absence of value (الصفر يعني عدم )وجود قيمة. Example: Weight of 0, height of 0—no weight or height. Descriptive Statistics 13