Lesson 2 Part 2.pdf
Document Details
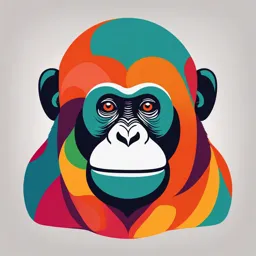
Uploaded by CooperativeJacksonville
Nanyang Technological University
Tags
Related
- Cybersecurity Technician Network Security Controls PDF
- Some Current Dimensions of Applied Behavior Analysis PDF 1968
- Baer et al. (1968) Some Current Dimensions of Applied Behavior Analysis PDF
- Insider Threat Analyst Training Lesson 2 Part 1 PDF
- Chapter 7 - 08 - Discuss Other Network Security Controls_fax_ocred.pdf
- CYB. Defensive AI (part 4) - Master's in Artificial Intelligence 2024/25
Full Transcript
Lesson 2 Part 2 As defined before, technical data is characterized by its digital origin, generated by computer systems and networks, and we will be diving into some of the examples of technical data sources, starting with user activity logs. User activity logs are fundamental records of what action...
Lesson 2 Part 2 As defined before, technical data is characterized by its digital origin, generated by computer systems and networks, and we will be diving into some of the examples of technical data sources, starting with user activity logs. User activity logs are fundamental records of what actions an employee has taken within our corporate systems. These logs typically include information on every login attempt, file access, system commands, and more. They are crucial for proving innocence or guilt and are an essential tool for any insider threat analyst. Monitoring these logs is an essential duty of a full-time insider threat analyst, as these are used to detect and mitigate potential insider threat activities. Think of this as the cold hard evidence you need to prove innocence or guilt in an active investigation. For example, if a user was accused of not logging into their corporate machine for an extended amount of time, user activity logs could be retrieved to prove whether the user had or had not been logged in and working during the suggested time frame. Moving on to endpoint data, this involves information gathered from each device within your network, from system configurations to software inventories. Endpoint data provides a detailed look at the health and security of each device. We will discuss the importance of this data in spotting unauthorized changes and installations, which might be indicative of insider threats or system compromises. Network traffic analysis is another critical data source for insider threats detection. By examining how data moves through your network, you can identify unusual patterns that may indicate malicious activities, such as data exfiltration or unauthorized access. We will explore typical anomalies detected through this method and discuss the tools and techniques used to monitor and analyze network traffic. Effectively, behavior analytics represents the cutting edge of insider threats detection. By using machine learning algorithms to analyze patterns of user behavior, we can identify activities that deviate from the norm and might indicate a threat. In this part of the lecture, we will look into how these systems are trained, the data they analyze, and how they can be tuned to better predict and prevent insider threats. Behavioral analytics involves the study and analysis of patterns in human behavior to predict and understand actions within a specific context. This translates to analyzing employee behaviors to detect anomalies that may indicate insider threats. We will be highlighting key behavioral indicators of insider threats, which are patterns of behavior, psychological factors, and physical actions. Patterns of behavior involves regular monitoring of behaviors, such as access patterns, working hours, and network activity that can reveal deviations that suggest malicious intent. Psychological factors, on the other hand, include changes in mood, attitude, or behavior that deviates from an individual's baseline and can serve as an early indicator of disgruntlement or malicious intent. And lastly, physical actions. Unusual physical access to restricted areas or improper handling of sensitive information can be detected through physical security measures. There are three methodologies for behavioral analysis, which includes data collection, pattern recognition, and contextual analysis. Data collection involves gathering data from various sources, including access logs, email traffic, and HR reports. And this is the first step in behavioral analysis. Secondly, we have pattern recognition, which involves using statistical models and machine learning techniques to identify patterns that deviate from the norm. Lastly, we have contextual analysis, which is the understanding of the context behind behaviors and behaviors, which is crucial for accurate interpretation. And this also involves considering personal circumstances and environmental factors. So what are the applications in cybersecurity? Continuous monitoring. Implementing systems that continuously monitor behavior to quickly detect and respond to anomalies. Risk assessment involves using behavioral analytics to assess the risk level of various behaviors, categorizing them based on potential threat levels. Insider threat programs. Integrating behavioral analytics into insider threat programs to enhance detection capabilities and improve response strategies. There are some challenges in behavioral analytics, which involves or which includes privacy concerns. Balancing security needs with individual privacy rights is a significant challenge, requiring clear policies and legal compliance. Second, we have data accuracy. Ensuring the accuracy and relevance of the data used for behavioral analytics is critical, as incorrect data can lead to false positives. And lastly, interpretation difficulties. Analyzing behavior can be subjective and differentiating between benign and malicious intent requires expertise and context. There are also ethical and legal considerations. The ethical usage implies employing behavioral analytics must be done ethically, respecting the dignity and privacy of individuals while protecting organizational security. Legal compliance, on the other hand, means adhering to legal standards regarding data protection and employee privacy is mandatory, with clear communication of monitoring practices to all stakeholders. Behavioral analytics is a powerful tool in the arsenal of cybersecurity, particularly effective in the realm of insider threat detection. By understanding and analyzing the nuances of human behavior, security professionals can preemptively address potential threats. The ethical, legal, and practical aspects of behavioral analytics must be carefully managed to ensure its effective and responsible use. Strengths and weaknesses of data sources. Each data source, while invaluable, also has its own set of challenges. We will examine the strengths that make these data sources indispensable tools for insider threat analysts, as well as the weaknesses that can limit their effectiveness, such as data volume, management challenges, privacy concerns, and legal restrictions.