Advanced Statistical Analysis - Week 1 Lecture 2
Document Details
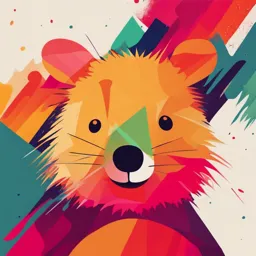
Uploaded by ClearerKoala
University of Groningen
2023
Mark van Duijn
Tags
Related
- Advanced Statistical Analysis Lecture Notes - University of Groningen
- Advanced Statistical Analysis Lecture Notes (Week 4)
- Module 1 : Régression Linéaire Simple PDF
- Financial Econometrics Exercise Sheet 4 Solutions PDF
- Mestrado em Matemática Financeira - Econometria dos Mercados Financeiros (FCUL e ISCTE) PDF
- Evidenziato Econometria A.A. 23/24 PDF
Summary
This document is the lecture notes for a course on advanced statistical analysis. It covers linear regression, the ordinary least squares method (OLS), assumptions behind OLS, different types of independent variables, and model fit statistics. The lecture was given by Dr. Mark van Duijn at the University of Groningen on February 9, 2023.
Full Transcript
Advanced Statistical Analysis Introduction & Practical issues Dr. Mark van Duijn Department of Economic Geography Department of DemographyUniversity of Groningen [email protected] 6 Feb, 2023 Introduction B. Exploring relationships C. Illustration Conclusions Today Introduction A. Motivation an...
Advanced Statistical Analysis Introduction & Practical issues Dr. Mark van Duijn Department of Economic Geography Department of DemographyUniversity of Groningen [email protected] 6 Feb, 2023 Introduction B. Exploring relationships C. Illustration Conclusions Today Introduction A. Motivation and practical information B. Exploring relationships C. Illustration using STATA Dr. Mark van Duijn (RUG) Advanced Statistical Analysis 6 Feb 20232 / 31 Introduction B. Exploring relationships C. Illustration Conclusions Motivation Wide knowledge of a variety of academic research methods (balance between qualitative and quantitative methods) Multidisciplinary field asks for objective analysis and systematic relationships Increasing demand for students with modelling skills (e.g. ”big data” specialists and forecasting specialists) Employers expect a certain ”level of thinking”: Critical thinking + analytic thinking Data availability Statistical methodologies and programmes: Excel/SPSS/STATA/R/others Dr. Mark van Duijn (RUG) Advanced Statistical Analysis 6 Feb 20233 / 31 Introduction B. Exploring relationships C. Illustration Conclusions Contact Coordinator: Dr. Mark van Duijn Lecturer event history analysis: Prof. dr. Clara Mulder Computer labs: Lara Bister MSc. Xiuxiang Pan MSc. Please contact us using e-mail subject:ASA Dr. Mark van Duijn (RUG) Advanced Statistical Analysis 6 Feb 20234 / 31 Introduction B. Exploring relationships C. Illustration Conclusions Schedule Lectures on Mondays: 11.00 - 13.00 by M. van Duijn & C.H. Mulder Lectures on Thursdays: 11.00 - 13.00 by M. van Duijn & C.H. Mulder Computer lab sessions on Thursdays: 15.00 - 17.00 (+extra hour) by Lara Bister & Xiuxiang Pan Always check rooster.rug.nl for up-to-date information! See the course guide on Brightspace for more information! Dr. Mark van Duijn (RUG) Advanced Statistical Analysis 6 Feb 20235 / 31 Introduction B. Exploring relationships C. Illustration Conclusions Assessment Digital exam where you need to use any statistical programme (Stata is default) (100%) April 4 from 15.00 - 18.00 (computer room: 5415.0032 + 42) Requirements: 5 out of 7 assignments must be submitted on time and must be graded as ’satisfactory’, and 5 out of 7 practicals need to be attended Attendence list during computer lab sessions See the course guide on NESTOR for more information! Dr. Mark van Duijn (RUG) Advanced Statistical Analysis 6 Feb 20236 / 31 Introduction B. Exploring relationships C. Illustration Conclusions Course material for the exam See the course guide on Brightspace for more information! Dr. Mark van Duijn (RUG) Advanced Statistical Analysis 6 Feb 20237 / 31 Introduction B. Exploring relationships C. Illustration Conclusions Getting to know you... Where are you from? Dr. Mark van Duijn (RUG) Advanced Statistical Analysis 6 Feb 20238 / 31 Introduction B. Exploring relationships C. Illustration Conclusions This classroom Knowledge spillovers We have a lot to share with you in a limited amount of time We like interacting with you . . . so do not be afraid to interrupt us during the lecture Talk to us before or after the lecture or during the break as much as possible Do not be afraid to make mistakes I hope you like puzzles . . . Dr. Mark van Duijn (RUG) Advanced Statistical Analysis 6 Feb 20239 / 31 Introduction B. Exploring relationships C. Illustration Conclusions Starting level: Week 1 Prior knowledge: Two statistics courses (280 hours) and one academic methods course (140 hours): statistics, basic linear regression, basic logistic regression Questions? Try to come to me for questions before or after class as much as possible! For questions outside the classroom: [email protected] Dr. Mark van Duijn (RUG) Advanced Statistical Analysis 6 Feb 202310 / 31 Introduction B. Exploring relationships C. Illustration Conclusions Continuing Introduction A. Motivation and practical information B. Exploring relationships C. Illustration using STATA Dr. Mark van Duijn (RUG) Advanced Statistical Analysis 6 Feb 202311 / 31 Introduction B. Exploring relationships C. Illustration Conclusions Research questions Dr. Mark van Duijn (RUG) Advanced Statistical Analysis 6 Feb 202312 / 31 Introduction B. Exploring relationships C. Illustration Conclusions Formulate research questions Relationship? Motivation / Rationale / Importance? Theory? What has been done before? Company reports? Policy reports? Academic literature? What is still unknown? How to operationalize key concepts/variables? Let us practice . . . Dr. Mark van Duijn (RUG) Advanced Statistical Analysis 6 Feb 202313 / 31 Introduction B. Exploring relationships C. Illustration Conclusions Coronavirus Dr. Mark van Duijn (RUG) Advanced Statistical Analysis 6 Feb 202314 / 31 Introduction B. Exploring relationships C. Illustration Conclusions Earthquakes in Groningen Dr. Mark van Duijn (RUG) Advanced Statistical Analysis 6 Feb 202315 / 31 Introduction B. Exploring relationships C. Illustration Conclusions Critical thinking and reflection Is the relationship a causal one? Impact/effect/influence vs. association What empirical model is valid to use? Qualitative/quantitative? Linear regression/Logistic regression? How do we get data? Do we observe all relevant variables? What is the quality of the data? Are the results intuitive / in line with theory? Are deviations of the model results structural or temporary (noise in market)? Model results vs. expert opinions Dr. Mark van Duijn (RUG) Advanced Statistical Analysis 6 Feb 202316 / 31 Introduction B. Exploring relationships C. Illustration Conclusions Systematic model of doing academic research Quantitative research: Aim is to provide insights into (new) empirical relationships using quantitative data! Dr. Mark van Duijn (RUG) Advanced Statistical Analysis 6 Feb 202317 / 31 Introduction B. Exploring relationships C. Illustration Conclusions Intuitive illustration For example, we gathered a lot of data on the height of mothers and daughters Survey questions: What is your height in cms? What is your mother’s height in cms? In which country are you born? A bold observation is that the daughter’s height seems to have a relationship with her mother’s heigth Step 1: ? Plot the height of daughters (y-axis) on the height of mothers (x-axis) Scatterplot: Dr. Mark van Duijn (RUG) Advanced Statistical Analysis 6 Feb 202318 / 31 Introduction B. Exploring relationships C. Illustration Conclusions Intuitive illustration For example, we gathered a lot of data on the height of mothers and daughters Survey questions: What is your height in cms? What is your mother’s height in cms? In which country are you born? A bold observation is that the daughter’s height seems to have a relationship with her mother’s heigth Step 1: ? Plot the height of daughters (y-axis) on the height of mothers (x-axis) Scatterplot: Dr. Mark van Duijn (RUG) Advanced Statistical Analysis 6 Feb 202318 / 31 Introduction B. Exploring relationships C. Illustration Conclusions Exploring relationships Dr. Mark van Duijn (RUG) Advanced Statistical Analysis 6 Feb 202319 / 31 Introduction B. Exploring relationships C. Illustration Conclusions What relationships do we observe? Dr. Mark van Duijn (RUG) Advanced Statistical Analysis 6 Feb 202320 / 31 Introduction B. Exploring relationships C. Illustration Conclusions What relationships do we observe? Dr. Mark van Duijn (RUG) Advanced Statistical Analysis 6 Feb 202321 / 31 Introduction B. Exploring relationships C. Illustration Conclusions What relationships do we observe? Correlation shows the statistical relationship between two variables Pearson correlation coefficient is mostly used: Only sensitive to linear relationships Pearson correlation coeffcient is not good with nonlinear relationships Correlation is NOTcausation!Dr. Mark van Duijn (RUG) Advanced Statistical Analysis 6 Feb 202322 / 31 Introduction B. Exploring relationships C. Illustration Conclusions Back to our example Dr. Mark van Duijn (RUG) Advanced Statistical Analysis 6 Feb 202323 / 31 Introduction B. Exploring relationships C. Illustration Conclusions Back to our illustration Dr. Mark van Duijn (RUG) Advanced Statistical Analysis 6 Feb 202324 / 31 Introduction B. Exploring relationships C. Illustration Conclusions Back to our illustration Dr. Mark van Duijn (RUG) Advanced Statistical Analysis 6 Feb 202325 / 31 Introduction B. Exploring relationships C. Illustration Conclusions Prediction y i = −15 .6 + 1 .103 x 1,i + e i ˆ y i = −15 .6 + 1 .103 x 1,i y i = 1 .010 x 1,i + e i ˆ y i = 1 .010 x 1,i Dr. Mark van Duijn (RUG) Advanced Statistical Analysis 6 Feb 202326 / 31 Introduction B. Exploring relationships C. Illustration Conclusions Prediction Dr. Mark van Duijn (RUG) Advanced Statistical Analysis 6 Feb 202327 / 31 Introduction B. Exploring relationships C. Illustration Conclusions Hypothesis testing Hypothesis: H 0: b 0 = 0 H 1: b 0 ̸ = 0 H 0: b 1 = 0 H 1: b 1 ̸ = 0 Memorize: t 0 .05 = 1 .96 Dr. Mark van Duijn (RUG) Advanced Statistical Analysis 6 Feb 202328 / 31 Introduction B. Exploring relationships C. Illustration Conclusions What did we learn? Recap on some statistics and research methods How to start exploring relationships using scatter plots and correlation matrices How to apply basic regression models Know the difference between prediction and hypothesis testing Dr. Mark van Duijn (RUG) Advanced Statistical Analysis 6 Feb 202329 / 31 Introduction B. Exploring relationships C. Illustration Conclusions Next... Lecture 2: Thursday Feb 9 11-13 OLS + Assumptions + Model fit Computer lab session: Thursday Feb 9 15-17 Deadline Assignment 1: Monday Feb 13 at 9am (upload assignments on Brightspace) Dr. Mark van Duijn (RUG) Advanced Statistical Analysis 6 Feb 202330 / 31 Advanced Statistical Analysis Week 1 Lecture 2: Ordinary Least Squares Dr. Mark van Duijn Department of Economic Geography Department of Demography University of Groningen [email protected] 9 Feb, 2023 Introduction Part I Part II Part III Conclusions Today’s learning objectives Describe the aim of regression models Linear regression (OLS estimation) OLS assumptions Describe different types of independent variables Model fit statistics Dr. Mark van Duijn (RUG) Advanced Statistical Analysis 9 Feb 20232 / 46 Introduction Part I Part II Part III Conclusions Agenda Part I: Linear regression Part II: Various independent variables Part III: Model fit statistics Dr. Mark van Duijn (RUG) Advanced Statistical Analysis 9 Feb 20233 / 46 Introduction Part I Part II Part III Conclusions Linear regression So... What do you know / remember? Dr. Mark van Duijn (RUG) Advanced Statistical Analysis 9 Feb 20234 / 46 Introduction Part I Part II Part III Conclusions Simple linear regression Y=β 0 + β 1X 1+ ϵ (1) Y : dependent variable X 1: independent variable, explanatory variable or regressor β 0: constant parameter that is unknown but can be estimated β 1: parameter of x 1 that is unknown but can be estimated ϵ : error term with specific properties Note in our example: the dependent and independent variable is continuous: Y(daughter’s height) and X 1 (mother’s height) Dr. Mark van Duijn (RUG) Advanced Statistical Analysis 9 Feb 20235 / 46 Introduction Part I Part II Part III Conclusions Population vs sample Dr. Mark van Duijn (RUG) Advanced Statistical Analysis 9 Feb 20236 / 46 Introduction Part I Part II Part III Conclusions Aim of linear regression y = b 0 + b 1x 1 + e (2)Aim? Prediction: Given a new x, predict y Estimation: The effect of an increase of one unit in x on the dependent variable y (hypothesis testing) This simple model is only an approximation to the truth . . . But often helpful to use in discussions on the topic Dr. Mark van Duijn (RUG) Advanced Statistical Analysis 9 Feb 20237 / 46 Introduction Part I Part II Part III Conclusions Aim of linear regression y = b 0 + b 1x 1 + e (2)Aim? Prediction: Given a new x, predict y Estimation: The effect of an increase of one unit in x on the dependent variable y (hypothesis testing) This simple model is only an approximation to the truth . . . But often helpful to use in discussions on the topic Dr. Mark van Duijn (RUG) Advanced Statistical Analysis 9 Feb 20237 / 46 Introduction Part I Part II Part III Conclusions Aim of linear regression y = b 0 + b 1x 1 + e (2)Aim? Prediction: Given a new x, predict y Estimation: The effect of an increase of one unit in x on the dependent variable y (hypothesis testing) This simple model is only an approximation to the truth . . . But often helpful to use in discussions on the topic Dr. Mark van Duijn (RUG) Advanced Statistical Analysis 9 Feb 20237 / 46 Introduction Part I Part II Part III Conclusions Linear regression assumptions Model assumptions Error term has a conditional mean of zero, is independent, is normally distibuted, and have a constant variance Model is correctly specified: Linearity between the Y (dependent variable) and X’s (independent variables) Absence of multicollinearity Dr. Mark van Duijn (RUG) Advanced Statistical Analysis 9 Feb 20238 / 46 Introduction Part I Part II Part III Conclusions OLS assumptions about error term ϵ∼ N(µ, σ 2 ϵ ) (3) with µthe mean and the variance σ2 ϵ Note that for the standard normal distribution the mean equals zero and the variance equal to one 1 The error term has a conditional mean of zero: E(µ ) = 0 2 Homoscedasticity (constant error variance: σ2 ϵ ) 3 Uncorrelated errors (autocorrelation) - over time / across space 4 Normally distributed errors ( N(− ,− )) Dr. Mark van Duijn (RUG) Advanced Statistical Analysis 9 Feb 20239 / 46 Introduction Part I Part II Part III Conclusions Violation of OLS assumptions and its consequences ϵ∼ N(µ, σ 2 ϵ ) (4) 1 The error term has a conditional mean of zero: E(µ ) = 0 2 Homoscedasticity (constant error variance: σ2 ϵ ) 3 Uncorrelated errors (autocorrelation) - over time / across space 4 Normally distributed errors ( N(− ,− )) Test your skills: Consistency? - Correctness of coefficients (aka BLUE) Efficiency? - Correctness of standard errors Chapter 7 in Mehmetoglu & Jakobsen (2017): Applied Statistics using STATA - A Guide for the Social Sciences Dr. Mark van Duijn (RUG) Advanced Statistical Analysis 9 Feb 202310 / 46 Introduction Part I Part II Part III Conclusions Intercept and slope Dr. Mark van Duijn (RUG) Advanced Statistical Analysis 9 Feb 202311 / 46 Introduction Part I Part II Part III Conclusions Prediction and error term y 1 = ˆ y 1 + e 1 = b 0 + b 1x 1,1 + e 1 y 2 = ˆ y 2 + e 2 = b 0 + b 2x 1,2 + e 2 . . . y n = ˆ y n + e n = b 0 + b 1x 1,n + e n Test your skills: What would b0, b1, and e be if daughters would always end up with the same height as their mothers? Dr. Mark van Duijn (RUG) Advanced Statistical Analysis 9 Feb 202312 / 46 Introduction Part I Part II Part III Conclusions Simple method to obtain parameters Remember: Our data : ( x 1,1 , y 1), ( x 1,2 , y 2), ( x 1,n , y n) ( x 1,i , y i) Parameters to be estimated: b 0, b 1 How to choose b 0 and b 1? Ordinary Least Squares (OLS) Choose b 0 and b 1 that minimizes the sum of all squared residuals (RSS or SSR) Residual sum of squares: ( e 1)2 + ( e 2)2 + . . . + (e n )2 = P n i =1 ( e i) 2 Reminder: y i = ˆ y i + e i → e i = y i − ˆ y i → e i = y i − (b 0 + b 1x 1,i ) Dr. Mark van Duijn (RUG) Advanced Statistical Analysis 9 Feb 202313 / 46 Introduction Part I Part II Part III Conclusions Simple method to obtain parameters Remember: Our data : ( x 1,1 , y 1), ( x 1,2 , y 2), ( x 1,n , y n) ( x 1,i , y i) Parameters to be estimated: b 0, b 1 How to choose b 0 and b 1? Ordinary Least Squares (OLS) Choose b 0 and b 1 that minimizes the sum of all squared residuals (RSS or SSR) Residual sum of squares: ( e 1)2 + ( e 2)2 + . . . + (e n )2 = P n i =1 ( e i) 2 Reminder: y i = ˆ y i + e i → e i = y i − ˆ y i → e i = y i − (b 0 + b 1x 1,i ) Dr. Mark van Duijn (RUG) Advanced Statistical Analysis 9 Feb 202313 / 46 Introduction Part I Part II Part III Conclusions Simple method to obtain parameters Remember: Our data : ( x 1,1 , y 1), ( x 1,2 , y 2), ( x 1,n , y n) ( x 1,i , y i) Parameters to be estimated: b 0, b 1 How to choose b 0 and b 1? Ordinary Least Squares (OLS) Choose b 0 and b 1 that minimizes the sum of all squared residuals (RSS or SSR) Residual sum of squares: ( e 1)2 + ( e 2)2 + . . . + (e n )2 = P n i =1 ( e i) 2 Reminder: y i = ˆ y i + e i → e i = y i − ˆ y i → e i = y i − (b 0 + b 1x 1,i ) Dr. Mark van Duijn (RUG) Advanced Statistical Analysis 9 Feb 202313 / 46 Introduction Part I Part II Part III Conclusions Back to our example Dr. Mark van Duijn (RUG) Advanced Statistical Analysis 9 Feb 202314 / 46 Introduction Part I Part II Part III Conclusions Back to our example Dr. Mark van Duijn (RUG) Advanced Statistical Analysis 9 Feb 202315 / 46 Introduction Part I Part II Part III Conclusions Back to our example Dr. Mark van Duijn (RUG) Advanced Statistical Analysis 9 Feb 202316 / 46 Introduction Part I Part II Part III Conclusions Prediction y i = −15 .6 + 1 .103 x 1,i + e i ˆ y i = −15 .6 + 1 .103 x 1,i y i = 1 .010 x 1,i + e i ˆ y i = 1 .010 x 1,i Dr. Mark van Duijn (RUG) Advanced Statistical Analysis 9 Feb 202317 / 46 Introduction Part I Part II Part III Conclusions Hypothesis testing Hypothesis: H 0: b 0 = 0 H 1: b 0 ̸ = 0 H 0: b 1 = 0 H 1: b 1 ̸ = 0 Memorize: t 0 .05 = 1 .96 Dr. Mark van Duijn (RUG) Advanced Statistical Analysis 9 Feb 202318 / 46 Introduction Part I Part II Part III Conclusions Critical t-values for various significance levels Dr. Mark van Duijn (RUG) Advanced Statistical Analysis 9 Feb 202319 / 46 Introduction Part I Part II Part III Conclusions Hypothesis testing Dr. Mark van Duijn (RUG) Advanced Statistical Analysis 9 Feb 202320 / 46 Introduction Part I Part II Part III Conclusions Multiple linear regression In principle the same. . . yi = b 0 + b 1X 1,i + b 2X 2,i + b 3X 3,i + ... +b kX k,i + ϵ i (5)Matrix notation for OLS: y= bX +ϵ (6) b = ˆ β = ( x′ x )− 1 x ′ y (7)Back to our example: Daughter’s height does not only depend on mother’s heigth, but also on father’s height Dr. Mark van Duijn (RUG) Advanced Statistical Analysis 9 Feb 202321 / 46 Introduction Part I Part II Part III Conclusions Comparison Dr. Mark van Duijn (RUG) Advanced Statistical Analysis 9 Feb 202322 / 46 Introduction Part I Part II Part III Conclusions Different types of variables Continuous, categorical, binary, . . . Independent variable Dependent variable What types of variables do you remember? Can you give an example for that type of variable? Dr. Mark van Duijn (RUG) Advanced Statistical Analysis 9 Feb 202323 / 46 Introduction Part I Part II Part III Conclusions Different types of variables Continuous, categorical, binary, . . . Independent variable Dependent variable Survey is extended and we gathered extra data in EU For now, we ignore the father’s height New variable: Daughter is Dutch or non-Dutch Binary independent variable: recode to a dummy (0/1) variable D=1 if daughter is Dutch; D=0 if daughter is non-Dutch Dr. Mark van Duijn (RUG) Advanced Statistical Analysis 9 Feb 202324 / 46 Introduction Part I Part II Part III Conclusions Multiple regression Dr. Mark van Duijn (RUG) Advanced Statistical Analysis 9 Feb 202325 / 46 Introduction Part I Part II Part III Conclusions Coding dummy variables non-Dutch = 0 Dutch = 1 Estimation: y i = b 0 + b 1x 1,i + b 2D i+ e i For non-Dutch: y i = b 0 + b 1x 1,i + b 20+ e i For Dutch: y i = b 0 + b 1x 1,i + b 21+ e i Dr. Mark van Duijn (RUG) Advanced Statistical Analysis 9 Feb 202326 / 46 Introduction Part I Part II Part III Conclusions Multiple regression Dr. Mark van Duijn (RUG) Advanced Statistical Analysis 9 Feb 202327 / 46 Introduction Part I Part II Part III Conclusions Hypothesis testing and interpretation Significance of the dummy variable (Dutch=1): H 0: b 2 = 0 H 1: b 2 ̸ = 0 Interpretation: The effect of being Dutch relative to being non-Dutch on daughter’s height is . . . b 2 What happens if the coding is the other way around? Dutch=0 and non-Dutch=1 − b 2 Other results do not depend on coding Two categories (Dutch, non-Dutch) →One dummy variable with one reference category Dr. Mark van Duijn (RUG) Advanced Statistical Analysis 9 Feb 202328 / 46 Introduction Part I Part II Part III Conclusions Hypothesis testing and interpretation Significance of the dummy variable (Dutch=1): H 0: b 2 = 0 H 1: b 2 ̸ = 0 Interpretation: The effect of being Dutch relative to being non-Dutch on daughter’s height is . . . b 2 What happens if the coding is the other way around? Dutch=0 and non-Dutch=1 − b 2 Other results do not depend on coding Two categories (Dutch, non-Dutch) →One dummy variable with one reference category Dr. Mark van Duijn (RUG) Advanced Statistical Analysis 9 Feb 202328 / 46 Introduction Part I Part II Part III Conclusions Hypothesis testing and interpretation Significance of the dummy variable (Dutch=1): H 0: b 2 = 0 H 1: b 2 ̸ = 0 Interpretation: The effect of being Dutch relative to being non-Dutch on daughter’s height is . . . b 2 What happens if the coding is the other way around? Dutch=0 and non-Dutch=1 − b 2 Other results do not depend on coding Two categories (Dutch, non-Dutch) →One dummy variable with one reference category Dr. Mark van Duijn (RUG) Advanced Statistical Analysis 9 Feb 202328 / 46 Introduction Part I Part II Part III Conclusions Hypothesis testing and interpretation Significance of the dummy variable (Dutch=1): H 0: b 2 = 0 H 1: b 2 ̸ = 0 Interpretation: The effect of being Dutch relative to being non-Dutch on daughter’s height is . . . b 2 What happens if the coding is the other way around? Dutch=0 and non-Dutch=1 − b 2 Other results do not depend on coding Two categories (Dutch, non-Dutch) →One dummy variable with one reference category Dr. Mark van Duijn (RUG) Advanced Statistical Analysis 9 Feb 202328 / 46 Introduction Part I Part II Part III Conclusions Categorical (independent) variables Survey question: What is your country of birth? 1 France 2 Italy 3 Netherlands How do we use a categorical (independent) variable in regression analysis? Dr. Mark van Duijn (RUG) Advanced Statistical Analysis 9 Feb 202329 / 46 Introduction Part I Part II Part III Conclusions Transform categorical (independent) variables to binary dummy variables Next: Pick a reference category (Netherlands) Create dummy variables: D 1: France D 2: Italy y i = b 0 + b 1x 1,i + b 2D 1+ b 3D 2+ e i Dr. Mark van Duijn (RUG) Advanced Statistical Analysis 9 Feb 202330 / 46 Introduction Part I Part II Part III Conclusions Categorical and binary (independent) variables Next: Pick a reference category (Netherlands) Create dummy variables: D 1: France D 2: Italy y i = b 0 + b 1x 1,i + b 2D 1+ b 3D 2+ e i Without further investigation, always transform categorical (independent) variables to dummy variables (0/1) Dr. Mark van Duijn (RUG) Advanced Statistical Analysis 9 Feb 202331 / 46 Introduction Part I Part II Part III Conclusions Different types of variables Next weeks: Continuous, categorical, binary, . . . Independent variable Dependent variable Dr. Mark van Duijn (RUG) Advanced Statistical Analysis 9 Feb 202332 / 46 Introduction Part I Part II Part III Conclusions Focus on model fit statistics Dr. Mark van Duijn (RUG) Advanced Statistical Analysis 9 Feb 202333 / 46 Introduction Part I Part II Part III Conclusions Do you recognize the following distributions? Dr. Mark van Duijn (RUG) Advanced Statistical Analysis 9 Feb 202334 / 46 Introduction Part I Part II Part III Conclusions Do you recognize the following distributions? Dr. Mark van Duijn (RUG) Advanced Statistical Analysis 9 Feb 202335 / 46 Introduction Part I Part II Part III Conclusions Statistics: Distributions Z and t distributions Dr. Mark van Duijn (RUG) Advanced Statistical Analysis 9 Feb 202336 / 46 Introduction Part I Part II Part III Conclusions Statistics: Distributions F distribution Dr. Mark van Duijn (RUG) Advanced Statistical Analysis 9 Feb 202337 / 46 Introduction Part I Part II Part III Conclusions Statistics: Distributions Chi square distribution Dr. Mark van Duijn (RUG) Advanced Statistical Analysis 9 Feb 202338 / 46 Introduction Part I Part II Part III Conclusions Model performance linear regression H 0 : b 1 = b 2 = b k = 0 Joint significance of the model using F-test: F[k − 1,n − k] = R 2 / (k − 1) (1 −R2 )/ (n − k) (8) F [k − 1,n − k] = (1 − SSR SST ) / (k − 1) ( SSR SST ) / (n − k) (9)Critical F-value? Model F-value higher or lower than the critical F-value? Dr. Mark van Duijn (RUG) Advanced Statistical Analysis 9 Feb 202339 / 46 Introduction Part I Part II Part III Conclusions Critical F-values (95% significance level) Dr. Mark van Duijn (RUG) Advanced Statistical Analysis 9 Feb 202340 / 46 Introduction Part I Part II Part III Conclusions Model performance linear regression Or . . . considering the variation explained by the model: R2 = P i( ˆ y i − ¯ y )2 P i( y i − ¯ y )2 = 1 −SSR SST (10)0 < R2 < 1 In general, R squared increases as number (k) of independent variables increases R2 adj = 1 −n − 1 n − k(1 −R2 ) (11) 0 < R2 adj < 1 Adjusted R squared accounts for the number (k) of independent variables Dr. Mark van Duijn (RUG) Advanced Statistical Analysis 9 Feb 202341 / 46 Introduction Part I Part II Part III Conclusions Focus on model fit statistics df1? df2? Critical F-value? Dr. Mark van Duijn (RUG) Advanced Statistical Analysis 9 Feb 202342 / 46 Introduction Part I Part II Part III Conclusions Another example: Model performance Dr. Mark van Duijn (RUG) Advanced Statistical Analysis 9 Feb 202343 / 46 Introduction Part I Part II Part III Conclusions Another example: Model performance Specification (1): R2 adj = 0 .369: 36.9% of the variance in the dependent variable is explained by the independent variables Specification (4) R2 adj = 0 .898: 89.8% of the variance in the dependent variable is explained by the independent variables Spec. (1): F value = 3389 and F criticalvalue (30 ,208274) = ∼1.45 F-value is higher than F-critical value so reject or not reject H0? Spec. (4): F value = 44537 and F criticalvalue (703 ,207601) = ∼1.1 F-value is higher than F-critical value so . . . Dr. Mark van Duijn (RUG) Advanced Statistical Analysis 9 Feb 202344 / 46 Introduction Part I Part II Part III Conclusions What did we learn? Describe the aim of regression models Linear regression (OLS estimation) OLS assumptions Describe different types of independent variables Model fit statistics Dr. Mark van Duijn (RUG) Advanced Statistical Analysis 9 Feb 202345 / 46 Introduction Part I Part II Part III Conclusions Next week... Lecture 3: Monday Feb. 13 11-13 Don’t forget the deadline for the assignment: Monday 9am Dr. Mark van Duijn (RUG) Advanced Statistical Analysis 9 Feb 202346 / 46 Advanced Statistical Analysis Week 2 - Lecture 3: Power of OLS and its limitations Dr. Mark van Duijn Department of Economic Geography Department of DemographyUniversity of Groningen [email protected] 13 Feb, 2023 Introduction Part I Part III Conclusions Agenda Part I: Transformations and interpretation Part II: Data and the power of OLS Schafer & Graham (2002) - Missing Data: Our View of the State of the Art Mehmetoglu & Jakobsen (2022) - Chapter 4,5,7 Dr. Mark van Duijn (RUG) Advanced Statistical Analysis 13 Feb 20232 / 39 Introduction Part I Part III Conclusions Transformations and interpretation Linear: y= b 0 + b 1x 1 + . . . +e When do we want to transform the variables? No normal distribution. Create a histogram. Transformation can help to obtain normal distribution Check the model fit before and after to say something about the transformation Log-linear: ln(y ) = b 0 + b 1x 1 + . . . +e Log-log: ln(y ) = b 0 + b 1ln (x 1) + . . .+e Dr. Mark van Duijn (RUG) Advanced Statistical Analysis 13 Feb 20233 / 39 Introduction Part I Part III Conclusions Transformations and interpretation Linear: y= b 0 + b 1x 1 + . . . +e When do we want to transform the variables? No normal distribution. Create a histogram. Transformation can help to obtain normal distribution Check the model fit before and after to say something about the transformation Log-linear: ln(y ) = b 0 + b 1x 1 + . . . +e Log-log: ln(y ) = b 0 + b 1ln (x 1) + . . .+e Dr. Mark van Duijn (RUG) Advanced Statistical Analysis 13 Feb 20233 / 39 Introduction Part I Part III Conclusions Transformations and interpretation Linear: y= b 0 + b 1x 1 + . . . +e When do we want to transform the variables? No normal distribution. Create a histogram. Transformation can help to obtain normal distribution Check the model fit before and after to say something about the transformation Log-linear: ln(y ) = b 0 + b 1x 1 + . . . +e Log-log: ln(y ) = b 0 + b 1ln (x 1) + . . .+e Dr. Mark van Duijn (RUG) Advanced Statistical Analysis 13 Feb 20233 / 39 Introduction Part I Part III Conclusions Transformations and interpretation Linear: y= b 0 + b 1x + . . . +e b 1 : level-effect +1 x increases y with b 1 Log-linear: ln(y ) = b 0 + b 1x + . . . +e b 1 : growth rate +1 x increases y with exp( b 1) times or +1 x increases y with (( exp( b 1) − 1) ∗100) % Log-log: ln(y ) = b 0 + b 1ln (x ) + . . .+e b 1 : elasticity +1% in x, increases y with ( b 1)% Assuming that x is a continuous variable! Dr. Mark van Duijn (RUG) Advanced Statistical Analysis 13 Feb 20234 / 39 Introduction Part I Part III Conclusions Data Cross-sectional: Observe multiple economic agents or events once Time-series: Observe one economic agent or event over multiple time periods Panel: Observe multiple economic agents or events over multiple time periods Dr. Mark van Duijn (RUG) Advanced Statistical Analysis 13 Feb 20235 / 39 Introduction Part I Part III Conclusions Data issues Show you the power of linear regression: Perfect data, perfect predictability, perfect linear relationships Introduce errors in data: Measurement error Introduce errors in data: Exclude important variables Introduce errors in data: Missing values Dr. Mark van Duijn (RUG) Advanced Statistical Analysis 13 Feb 20236 / 39 Introduction Part I Part III Conclusions Example Start with an everyday example to explain the price of groceries Imagine you do grocery shopping You have the following information on: Total price of all items Some of the bought items (and quantities) Multiple observations Dr. Mark van Duijn (RUG) Advanced Statistical Analysis 13 Feb 20237 / 39 Introduction Part I Part III Conclusions Linear regression We then can reveal: The implicit prices for each item More specifically, the willingness to pay for each item How to do this?: Regress the (total) price of the heterogeneous goods on the quantities of the attributes Example on how this works: Dr. Mark van Duijn (RUG) Advanced Statistical Analysis 13 Feb 20238 / 39 Introduction Part I Part III Conclusions Example data Dr. Mark van Duijn (RUG) Advanced Statistical Analysis 13 Feb 20239 / 39 Introduction Part I Part III Conclusions Linear regression price =β 0+ β 1∗ apples +β 2∗ pears +β 3∗ milk +β 4∗ bread +β 5∗ chocolate +ϵ Dr. Mark van Duijn (RUG) Advanced Statistical Analysis 13 Feb 202310 / 39 Introduction Part I Part III Conclusions Linear regression price =β 0+ β 1∗ apples +β 2∗ pears +β 3∗ milk +β 4∗ bread +β 5∗ chocolate +ϵ Dr. Mark van Duijn (RUG) Advanced Statistical Analysis 13 Feb 202311 / 39 Introduction Part I Part III Conclusions Linear regression with perfect information price =β 0+ β 1∗ apples +β 2∗ pears +β 3∗ milk +β 4∗ bread +β 5∗ chocolate +ϵ Dr. Mark van Duijn (RUG) Advanced Statistical Analysis 13 Feb 202312 / 39 Introduction Part I Part III Conclusions Measurement error In surveys there are always some errors. What are the sources of these errors? Respondents do not honestly answer the question (age, income, . . . ) Why do we care about measurement error? Can distort observed relationships and makes multivariate techniques less powerful Can result in biased coefficients, but always results in efficiency losses (read: larger standard errors →lower t-values →higher p-values) 3 possible scenarios: Measurement error in dependent variable Measurement error in independent variable Measurement error in both dependent and independent variable Dr. Mark van Duijn (RUG) Advanced Statistical Analysis 13 Feb 202313 / 39 Introduction Part I Part III Conclusions Measurement error Measurement error in dependent variable If the error in Y is random (and thus uncorrelated with X), it does not bias the estimates of the coefficients The error in Y estimates the relationship less precisely: larger estimated standard errors Measurement error in independent variable The error in X always biases the coefficients towards zero Underestimate positive coefficients and overestimate negative coefficients Known as attenuation bias Dr. Mark van Duijn (RUG) Advanced Statistical Analysis 13 Feb 202314 / 39 Introduction Part I Part III Conclusions Measurement error Solutions? It may not be a problem: if your results are still acceptable, it only makes your results more ’conservative’ Use instrumental variables (2SLS): Find a ’strong’ instrument which is correlated with your X and uncorrelated with the error term Use an error-in-variables model: if we know, or can estimate, the amount of error in X, one can ’fix-up’ the estimates (eivreg in STATA) Dr. Mark van Duijn (RUG) Advanced Statistical Analysis 13 Feb 202315 / 39 Introduction Part I Part III Conclusions Linear regression with measurement error price =β 0+ β 1∗ apples +β 2∗ pears +β 3∗ milk +β 4∗ bread +β 5∗ chocolate +ϵ Dr. Mark van Duijn (RUG) Advanced Statistical Analysis 13 Feb 202316 / 39 Introduction Part I Part III Conclusions Linear regression price =β 0+ β 1∗ apples +β 2∗ pears +β 3∗ milk +β 4∗ bread +β 5∗ chocolate +ϵ Estimated price Real price Apples β 1 ¿ 0.254 ¿ 0.25 Pears β 2 ¿ 0.362 ¿ 0.35 Milk β 3 ¿ 1.196 ¿ 1.20 Bread β 4 ¿ 0.942 ¿ 0.99 Chocolat β 5 ¿ 2.493 ¿ 2.50 n=500 What happens if ndecreases? What happens if we do not observe quantities of chocolat? Dr. Mark van Duijn (RUG) Advanced Statistical Analysis 13 Feb 202317 / 39 Introduction Part I Part III Conclusions Missing observations and omitted variable bias Fewer observations lead to less precise estimates! Omitted variables can lead to inconsistent (biased) results! Dr. Mark van Duijn (RUG) Advanced Statistical Analysis 13 Feb 202318 / 39 Introduction Part I Part III Conclusions Missing observations and omitted variable bias Fewer observations lead to less precise estimates! Omitted variables can lead to inconsistent (biased) results! Dr. Mark van Duijn (RUG) Advanced Statistical Analysis 13 Feb 202318 / 39 Introduction Part I Part III Conclusions Missing values in variables Some of the information is not available for a respondent in dependent or independent variables? Important question: Is it systematic or random? What is its impact? Reduces sample size available for analysis (see previous example) Can distort results (bias and inefficiency) Types of missing data: Missings completely at random (MCAR) Missings not at random (MNAR) Missings at random (MAR) Dr. Mark van Duijn (RUG) Advanced Statistical Analysis 13 Feb 202319 / 39 Introduction Part I Part III Conclusions Missing values in variables Some of the information is not available for a respondent in dependent or independent variables? Important question: Is it systematic or random? What is its impact? Reduces sample size available for analysis (see previous example) Can distort results (bias and inefficiency) Types of missing data: Missings completely at random (MCAR) Missings not at random (MNAR) Missings at random (MAR) Dr. Mark van Duijn (RUG) Advanced Statistical Analysis 13 Feb 202319 / 39 Introduction Part I Part III Conclusions Missing values in variables Some of the information is not available for a respondent in dependent or independent variables? Important question: Is it systematic or random? What is its impact? Reduces sample size available for analysis (see previous example) Can distort results (bias and inefficiency) Types of missing data: Missings completely at random (MCAR) Missings not at random (MNAR) Missings at random (MAR) Dr. Mark van Duijn (RUG) Advanced Statistical Analysis 13 Feb 202319 / 39 Introduction Part I Part III Conclusions Types of missing data Missing completely at random (MCAR) Probability that an observation is missing is unrelated to the value or another variable Does not lead to bias in regression analysis. Missings are ignorable Dr. Mark van Duijn (RUG) Advanced Statistical Analysis 13 Feb 202320 / 39 Introduction Part I Part III Conclusions Types of missing data Missing not at random (MNAR) Probability that an observation is missing is related to the value or another variable For example, high income people do not fill in the question about income Does lead to bias in regression analysis. Missings are non-ignorable Dr. Mark van Duijn (RUG) Advanced Statistical Analysis 13 Feb 202321 / 39 Introduction Part I Part III Conclusions Types of missing data Missing at random (MAR) Probability that an observation is missing is only related to another variable For example, depressed people have a lower income in general and may be less inclined to report their income Does lead to bias in regression analysis if control variables are missing. Missings are non-ignorable Dr. Mark van Duijn (RUG) Advanced Statistical Analysis 13 Feb 202322 / 39 Introduction Part I Part III Conclusions Reporting on missing data Survey How many respondents did participate in the survey? Response rate Where did you survey the respondents? and/or Where do the respondents live? Which variables are affected? Frequency of not responding to each of the variables involved Show this in a table: ”Descriptive statistics” Dr. Mark van Duijn (RUG) Advanced Statistical Analysis 13 Feb 202323 / 39 Introduction Part I Part III Conclusions Missing data How to deal with missing data 1 Determine the type of missing data 2 Determine the extent of missing data 3 Diagnose the randomness of the missing data 4 Select the appropriate method for solution Dr. Mark van Duijn (RUG) Advanced Statistical Analysis 13 Feb 202324 / 39 Introduction Part I Part III Conclusions Solutions Data reduction Missing category Carry over value Imputation Mean imputation Regression mean imputation Multiple imputation Other: weighting Dr. Mark van Duijn (RUG) Advanced Statistical Analysis 13 Feb 202325 / 39 Introduction Part I Part III Conclusions Data reduction Listwise deletion (default) Each case that has a missing value for any variable in the analysis is dropped from the analysis Reduced sample size; reduces statistical power Unbiased results if data are MCAR Unbiased results if data are MAR when controlled for variables that affect missingness Dr. Mark van Duijn (RUG) Advanced Statistical Analysis 13 Feb 202326 / 39 Introduction Part I Part III Conclusions Extra category Add an additional category Only works for missings in categorical variables or with recoding of continuous variable Does not reduce sample size Can bias results as very different cases can be grouped Dr. Mark van Duijn (RUG) Advanced Statistical Analysis 13 Feb 202327 / 39 Introduction Part I Part III Conclusions Carry over value Carry last entry forward Missing item at t=4, t=5, t=6 takes on the value from t3 Only works with longitudinal data Dr. Mark van Duijn (RUG) Advanced Statistical Analysis 13 Feb 202328 / 39 Introduction Part I Part III Conclusions Imputation Simple mean Only possible for continuous variables Dr. Mark van Duijn (RUG) Advanced Statistical Analysis 13 Feb 202329 / 39 Introduction Part I Part III Conclusions Imputation Regression mean Dr. Mark van Duijn (RUG) Advanced Statistical Analysis 13 Feb 202330 / 39 Introduction Part I Part III Conclusions Imputation Multiple imputation 1 Imputation multiple times 2 Analysis each imputation 3 Pooling of results Dr. Mark van Duijn (RUG) Advanced Statistical Analysis 13 Feb 202331 / 39 Introduction Part I Part III Conclusions Weighting Weighting to let sample distribution of key variables match the (assumed) known population distribution Simple example: survey response rate is 100 % of women, but only 50% of men Inverse weighting solution: every male response is given a weight of 2 However, in practice much more difficult! The more key variables, the more difficult to create weights (gender, income, ethnicity, . . . ) Dr. Mark van Duijn (RUG) Advanced Statistical Analysis 13 Feb 202332 / 39 Introduction Part I Part III Conclusions Solutions Data reduction Missing category Carry over value Imputation Other: weighting None of the proposed methods is ideal, most rely on strong assumptions More complete data →Better results, less bias Important for any scientific paper: Report how you deal with missing data and discuss possible bias due to missing data Dr. Mark van Duijn (RUG) Advanced Statistical Analysis 13 Feb 202333 / 39 Introduction Part I Part III Conclusions Data issues There are quite some data issues that you might encounter. Some examples: Sampling errors Measurement errors Omitted variables Non-response . . . and many more (see, e.g., https://www.statisticshowto.datasciencecentral.com/what-is-bias/) Bias often leads to consistency issues and always leads to efficiency issues! Dr. Mark van Duijn (RUG) Advanced Statistical Analysis 13 Feb 202334 / 39 Introduction Part I Part III Conclusions Diagnostics Dr. Mark van Duijn (RUG) Advanced Statistical Analysis 13 Feb 202335 / 39 Introduction Part I Part III Conclusions Obligatory article to study Schafer & Graham (2002). Missing Data: Our View of the State of the Art. Psychological Methods , 7(2), 147-177.Dr. Mark van Duijn (RUG) Advanced Statistical Analysis 13 Feb 202336 / 39 Introduction Part I Part III Conclusions What did we learn? Solutions to data issues are all about: Does my data still represent the population? Consistency andefficiency !Dr. Mark van Duijn (RUG) Advanced Statistical Analysis 13 Feb 202337 / 39 Introduction Part I Part III Conclusions What did we learn? Make judgements on when to transform variables (and when not) Describe the causes and consequences of measurement error Describe and identify the problems and impact of missing variables and missing values Describe and apply solutions to missing values Dr. Mark van Duijn (RUG) Advanced Statistical Analysis 13 Feb 202338 / 39 Introduction Part I Part III Conclusions Next Lecture 4: Feb 16 at 11h00-13h00 Computer lab sessions: Thursday Feb 16 at 15h00-17h00 Prepare Mehmetoglu & Jakobsen (2022) - Chapter 6 Dr. Mark van Duijn (RUG) Advanced Statistical Analysis 13 Feb 202339 / 39 Advanced Statistical Analysis Week 2 Lecture 4 Dr. Mark van Duijn Department of Economic Geography Department of DemographyUniversity of Groningen [email protected] 16 Feb, 2023 Introduction Interpretation Functional form Collinearity issues Conclusions Last lecture OLS properties and assumptions Interpretation and model fit Data transformation Data problems: Measurement error, omitted variables and missing values Schafer & Graham (2002) - Missing Data: Our View of the State of the Art Mehmetoglu & Jakobsen (2017) - Chapter 7 Dr. Mark van Duijn (RUG) Advanced Statistical Analysis 16 Feb 20232 / 28 Introduction Interpretation Functional form Collinearity issues Conclusions Agenda Part I: Interpretation Part II: Functional form Part III: (Multi)collinearity Part IV: Room for Q&A Finalizing OLS Mehmetoglu & Jakobsen (2017) - Chapter 7,15.3-15.6 Dr. Mark van Duijn (RUG) Advanced Statistical Analysis 16 Feb 20233 / 28 Introduction Interpretation Functional form Collinearity issues Conclusions Intercept and slope If x increases by 1, y increases with . . . Dr. Mark van Duijn (RUG) Advanced Statistical Analysis 16 Feb 20234 / 28 Introduction Interpretation Functional form Collinearity issues Conclusions Transformations Continuous, categorical, binary, . . . Independent variable Dependent variable(income) Continuous: X can be any number (age) Categorical: C=1 or 2, or 3, or 4, etc. (high school, college-degree, university-degree, doctor-degree) Binary/Dummy: D=0 or D=1 (male or female) Which type of variable is easiest to transform? And do you know why? Remember, that data transformation is notunethical data manipulation! Dr. Mark van Duijn (RUG) Advanced Statistical Analysis 16 Feb 20235 / 28 Introduction Interpretation Functional form Collinearity issues Conclusions Different types of variables Continuous, categorical, binary, . . . Independent variable Dependent variable (income) Continuous: X can be any number (age) Categorical: C=0 or 1, or 2, or 3, or 4, etc. (none=0, high school=1, college-degree=2, university-degree=3, doctor-degree=4) Binary/Dummy: D=0 or D=1 (male or female) inc i= b 0 + b 1x 1,i + b 2C i+ b 3D i+ e i If C increases by 1, y increases with . . . If D=1=female, y increases with . . . compared to D=0=male Dr. Mark van Duijn (RUG) Advanced Statistical Analysis 16 Feb 20236 / 28 Introduction Interpretation Functional form Collinearity issues Conclusions Dummy variables If D=1=female, y increases with . . . compared to D=0=male Or, the effect of being a women compared to being a man is . . . Dr. Mark van Duijn (RUG) Advanced Statistical Analysis 16 Feb 20237 / 28 Introduction Interpretation Functional form Collinearity issues Conclusions Transformation + Interpretation What changes if we take the natural logarithm of income? Continuous: X can be any number (age) Categorical: C=0 or 1, or 2, or 3, or 4, etc. (none=0, high school=1, college-degree=2, university-degree=3, doctor-degree=4) Binary/Dummy: D=0 or D=1 (male or female) ln (inc i) = b 0 + b 1x 1,i + b 2C i+ b 3D i+ e i If C increases by 1, y increases with . . . If D=1=female, y increases with . . . compared to D=0=male Dr. Mark van Duijn (RUG) Advanced Statistical Analysis 16 Feb 20238 / 28 Introduction Interpretation Functional form Collinearity issues Conclusions Example of a ln-transformation Dr. Mark van Duijn (RUG) Advanced Statistical Analysis 16 Feb 20239 / 28 Introduction Interpretation Functional form Collinearity issues Conclusions Transformations and interpretation Linear: y= b 0 + b 1x + . . . +e b 1 : level-effect +1 x increases y with b 1 Log-linear: ln(y ) = b 0 + b 1x + . . . +e b 1 : growth rate +1 x increases y with exp( b 1) times or +1 x increases y with (( exp( b 1) − 1) ∗100) % Log-log: ln(y ) = b 0 + b 1ln (x ) + . . .+e b 1 : elasticity +1% in x, increases y with ( b 1)% Assuming that x is a continuous variable! Dr. Mark van Duijn (RUG) Advanced Statistical Analysis 16 Feb 202310 / 28 Introduction Interpretation Functional form Collinearity issues Conclusions Functional form Dr. Mark van Duijn (RUG) Advanced Statistical Analysis 16 Feb 202311 / 28 Introduction Interpretation Functional form Collinearity issues Conclusions Functional form Functional form refers to the form of a relationship between a dependent variable and regressors So, what to do when we do not observe a linear relationship between y and x? Dr. Mark van Duijn (RUG) Advanced Statistical Analysis 16 Feb 202312 / 28 Introduction Interpretation Functional form Collinearity issues Conclusions Last example How to get from line 1 to line 2? What kind of transformation is needed? Dr. Mark van Duijn (RUG) Advanced Statistical Analysis 16 Feb 202313 / 28 Introduction Interpretation Functional form Collinearity issues Conclusions Last example Polynomials y = b 0 + b 1x + b 2x 2 + e If we plot this formula it looks much like LINE 2 First derivative gives us the interpretation (if x increases with 1, y increases with . . . ) ∂ y ∂ x = . . . ∂ y ∂ x = b 1 + b 22 x Note: Slope is a linear line increasing in x Dr. Mark van Duijn (RUG) Advanced Statistical Analysis 16 Feb 202314 / 28 Introduction Interpretation Functional form Collinearity issues Conclusions Last example Polynomials y = b 0 + b 1x + b 2x 2 + e If we plot this formula it looks much like LINE 2 First derivative gives us the interpretation (if x increases with 1, y increases with . . . ) ∂ y ∂ x = . . . ∂ y ∂ x = b 1 + b 22 x Note: Slope is a linear line increasing in x Dr. Mark van Duijn (RUG) Advanced Statistical Analysis 16 Feb 202314 / 28 Introduction Interpretation Functional form Collinearity issues Conclusions Remember: Multivariate analysis y= b 0 + b 1x 1 + b 2x 2 + ... +ϵ (1)Corrects for associations between two (or more) independent variables Coefficients measures the effect of each independent variable in the presence of other independent variables Example: Income and level of education affect the transition to home-ownership But what if the associations are too strong? Example: Length and weight of babies Dr. Mark van Duijn (RUG) Advanced Statistical Analysis 16 Feb 202315 / 28 Introduction Interpretation Functional form Collinearity issues Conclusions What is (multi)collinearity Strong association between two (or more) variables: one variable (almost) perfectly predicts the other Example of perfect multicollinearity: Suppose: y= β 0 + β 1x 1 + β 2x 2 + ϵwith x 1 = 2 x 2 + 4 Problem: No unique solution to the least squares minimization problem In other words: β 1 measures the effect of x 1 on y, holding x 2 constant. However, . . . Variables ’take over’ each others’ effects: unclear what causes what High correlation (above 0.9? or 0.8??) Dr. Mark van Duijn (RUG) Advanced Statistical Analysis 16 Feb 202316 / 28 Introduction Interpretation Functional form Collinearity issues Conclusions Detect (multi)collinearity Rule of thumb: Sample correlation >0.8 is evidence of severe collinearity However, if the collinear relationship involves more than 2 independent variables, you may not detect it using simple correlations Look at Variance Inflation Factors (VIFs): 1 Regress each independent variable, ( x k ), on all the other independent variables 2 Collect the R squared of each of the regressions: ( R2 k ) 3 Compute the VIF: VIF(x k ) = 1 (1 −R2 j ) 4 Rule of thumb: VIF(x k ) > 5 is evidence of severe multicollinearity Dr. Mark van Duijn (RUG) Advanced Statistical Analysis 16 Feb 202317 / 28 Introduction Interpretation Functional form Collinearity issues Conclusions Solution for perfect (multi)collinearity Delete one of the (irrelevant) variables from the model For categorical variables: leave out one whole variable OR a category; rearrange variables or categories Usually, this is not very difficult Dr. Mark van Duijn (RUG) Advanced Statistical Analysis 16 Feb 202318 / 28 Introduction Interpretation Functional form Collinearity issues Conclusions Solution for imperfect (multi)collinearity Usually, this is more difficult Do nothing: face the consequences Delete one of the (irrelevant) variables from the model For categorical variables: leave out one whole variable OR a category; rearrange variables or categories Transform one of the variables - only if it is consistent with theory and common sense Principal component analysis Dr. Mark van Duijn (RUG) Advanced Statistical Analysis 16 Feb 202319 / 28 Introduction Interpretation Functional form Collinearity issues Conclusions Example Dependent variable: realising the wish to become a homeowner (categories: did not move, moved to owner-occupied market, moved to rental market) Theoretical reasons to expect an effect of: Absolute local house price Ratio of average local house price to average local rent Correlation matrix: Both variables are collinear (apparently too little regional variation in average rents) Solution? Dr. Mark van Duijn (RUG) Advanced Statistical Analysis 16 Feb 202320 / 28 Introduction Interpretation Functional form Collinearity issues Conclusions Choice that was made in the paper Present the model with price-to-rent ratio only (theoretical reason) Discuss absolute local house prices in the text and report the existence of collinearity Dr. Mark van Duijn (RUG) Advanced Statistical Analysis 16 Feb 202321 / 28 Introduction Interpretation Functional form Collinearity issues Conclusions Last example: xand x2 Very strong association. . . but no perfect collinearity Neat way of relaxing the assumption of linear effect Compute in STATA: gen agesq = age*age Include age and agesq as independent variables (remember our discussion on polynomials in week 1) Remember how to interpret the coefficients (increase of one unit in X . . . ) Dr. Mark van Duijn (RUG) Advanced Statistical Analysis 16 Feb 202322 / 28 Introduction Interpretation Functional form Collinearity issues Conclusions Other example Dr. Mark van Duijn (RUG) Advanced Statistical Analysis 16 Feb 202323 / 28 Introduction Interpretation Functional form Collinearity issues Conclusions General lessons: Always. . . Explore your data carefully Look at your data Run frequencies, crosstabs, correlations, . . . Build your models carefully Start with few variables, then add more and see what changes in the results Only add variables that make sense! Preferably, derive them from theory and/or literature It is never a bad thing to run 20+ regressions and see how robust your results are to small changes (adding/removing variables, adding/removing groups, . . . ) Dr. Mark van Duijn (RUG) Advanced Statistical Analysis 16 Feb 202324 / 28 Introduction Interpretation Functional form Collinearity issues Conclusions Data management Where to save the data? Open source vs. Non-disclosure agreements What is a ’safe’ environment? When to delete data? How to deal with data in research? Data quality + ethics: What am I allowed to do with the raw data obtained? Report on data management: How do you go from raw data to data used for analysis (in main text + details in the Appendix) Syntax files: Transparancy + reproducability of analyses (add to the Appendix) Dr. Mark van Duijn (RUG) Advanced Statistical Analysis 16 Feb 202325 / 28 Introduction Interpretation Functional form Collinearity issues Conclusions Any remaining questions about OLS? Any remaining questions about OLS? Estimation technique? Tranforming data vs. data manipulation? Ethical considerations? Model building? Interpretation coefficients (of various types of independent (x) variables)? Model fit statistics? . . . We move to discrete models (e.g. logistic regression) on Monday! Dr. Mark van Duijn (RUG) Advanced Statistical Analysis 16 Feb 202326 / 28 Introduction Interpretation Functional form Collinearity issues Conclusions What did we learn? Make judgements on when to transform variables (and when not) Being able to interpret estimated coefficients of different kind of independent variables Exploring non-linear relationships between y and x using OLS Identify and deal with (multi)collinearity How to deal with data in a responsible way Dr. Mark van Duijn (RUG) Advanced Statistical Analysis 16 Feb 202327 / 28 Introduction Interpretation Functional form Collinearity issues Conclusions Next Computer lab sessions: Today 15h00-17h00 Lecture: Next Monday from 11h00-13h00 Prepare Mehmetoglu & Jakobsen (2022) - Chapter 8 DeMaris (1995) - Tutorial on logistic regression Dr. Mark van Duijn (RUG) Advanced Statistical Analysis 16 Feb 202328 / 28 Advanced Statistical Analysis Week 3 - Lecture 5 Dr. Mark van Duijn Department of Economic Geography Department of DemographyUniversity of Groningen [email protected] 20 Feb, 2023 Introduction Part I Part II Conclusions Announcement National Student Survey! Important to promote your Master! Dr. Mark van Duijn (RUG) Advanced Statistical Analysis 20 Feb 20232 / 27 Introduction Part I Part II Conclusions Agenda Part I: Discrete choice models: Logistic regression / Logit Part II: DeMaris (1995) Dr. Mark van Duijn (RUG) Advanced Statistical Analysis 20 Feb 20233 / 27 Introduction Part I Part II Conclusions Logistic regression So... What do you know / remember? Dr. Mark van Duijn (RUG) Advanced Statistical Analysis 20 Feb 20234 / 27 Introduction Part I Part II Conclusions Discrete choice models Discrete choice models: These type of models describe (decision makers’) choices among two or more discrete alternatives (can also be applied to events) In other words, the dependent variable is not a continuous variable but a limited dependent variable (or discrete variable)Binary choice (0/1) Multinomial choice . . . Decision makers: people, households, firms, etc. Alternatives: competing products or any other options or items over which choices must be made Background literature:Ch.1-2-3 Train (2009) Dr. Mark van Duijn (RUG) Advanced Statistical Analysis 20 Feb 20235 / 27 Introduction Part I Part II Conclusions Discrete choice models Discrete choice models: These type of models describe (decision makers’) choices among two or more discrete alternatives (can also be applied to events) In other words, the dependent variable is not a continuous variable but a limited dependent variable (or discrete variable)Binary choice (0/1) Multinomial choice . . . Decision makers: people, households, firms, etc. Alternatives: competing products or any other options or items over which choices must be made Background literature:Ch.1-2-3 Train (2009) Dr. Mark van Duijn (RUG) Advanced Statistical Analysis 20 Feb 20235 / 27 Introduction Part I Part II Conclusions Discrete choice models Discrete choice models: These type of models describe (decision makers’) choices among two or more discrete alternatives (can also be applied to events) In other words, the dependent variable is not a continuous variable but a limited dependent variable (or discrete variable)Binary choice (0/1) Multinomial choice . . . Decision makers: people, households, firms, etc. Alternatives: competing products or any other options or items over which choices must be made Background literature:Ch.1-2-3 Train (2009) Dr. Mark van Duijn (RUG) Advanced Statistical Analysis 20 Feb 20235 / 27 Introduction Part I Part II Conclusions Discrete choice models Discrete choice models: These type of models describe (decision makers’) choices among two or more discrete alternatives (can also be applied to events) In other words, the dependent variable is not a continuous variable but a limited dependent variable (or discrete variable)Binary choice (0/1) Multinomial choice . . . Decision makers: people, households, firms, etc. Alternatives: competing products or any other options or items over which choices must be made Background literature:Ch.1-2-3 Train (2009) Dr. Mark van Duijn (RUG) Advanced Statistical Analysis 20 Feb 20235 / 27 Introduction Part I Part II Conclusions Discrete choice models When to use discrete choice models: ”In general, the researcher needs to consider the goals of the research and the capabilities of alternative methods when deciding whether to apply a discrete choice model” (Train, 2009, p.14)Train’s Ch. 1, 2 and 3 is no exam material but the following sheets are. . . Dr. Mark van Duijn (RUG) Advanced Statistical Analysis 20 Feb 20236 / 27 Introduction Part I Part II Conclusions The choice set Characteristics that should be met to use discrete choice models: Alternatives must be mutually exclusiveThe choice must be exhaustiveThe number of alternatives must be finiteThe first and second criteria can nearly always be met if they are violated! For example, suppose 2 alternatives are labeled A and B. Both A and B can be chosen. . . Solution? Individual can choose neither. . . Solution? Important assumption: Choice is based on utility maximization behavior! Dr. Mark van Duijn (RUG) Advanced Statistical Analysis 20 Feb 20237 / 27 Introduction Part I Part II Conclusions The choice set Characteristics that should be met to use discrete choice models: Alternatives must