Lecture on Panel Data and Fixed Effects
Document Details
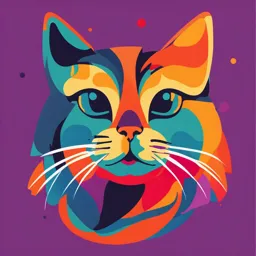
Uploaded by LongLastingGalaxy
Magnus Carlsson
Tags
Summary
This lecture, presented by Magnus Carlsson, explores panel data and fixed effects. It discusses the estimation of causal effects, methods for controlling omitted variables, and the application of models such as random effects and fixed effects approaches. It offers different estimation techniques and provides insights into interpreting the results.
Full Transcript
Panel data and fixed effects Magnus Carlsson Fixed effects, difference-in-differences, and panel data To estimate causal effects, experiments, IV or RD methods are preferred methods ln many applications, however, experiments are impossible and there are simply no good instruments or disco...
Panel data and fixed effects Magnus Carlsson Fixed effects, difference-in-differences, and panel data To estimate causal effects, experiments, IV or RD methods are preferred methods ln many applications, however, experiments are impossible and there are simply no good instruments or discontinuities to exploit An alternative is then methods that allow us to at least control for certain types of omitted variables These methods allow us to control for the unobserved factors that are fixed over time or space Fixed effects, difference-in-differences, and panel data: roadmap 1. Fixed effects and panel data 1. Panel data: random vs fixed effects 2. Fixed effects estimation with panel data 3. Pitfalls 4. Fixed effects estimation with other data structures 2. Difference-in-differences 1. Estimation 2. Pitfalls and sensitivity checks Panel data and fixed effects This lecture deals with panel data and fixed effects estimation Panel data follows outcomes and characteristics of individuals at multiple points in time Typically, the sample of individuals N is relatively large, while the number of time periods T over which these individuals are observed is generally short. Fixed effects refer to one way of analyzing panel data but the fixed effects approach can be applied to other data structures as well This includes family-level data, twin data, etc., without a time dimension The simplest case: running OLS on panel data One can always analyze panel data by just pooling observations over time and running an OLS (and treating all observations as independent): 𝑌𝑖𝑡 = 𝛼 + 𝑋𝑖𝑡 𝛽 + 𝜀𝑖𝑡 𝑖 = 1, … , 𝑁 𝑡 = 1, … , 𝑇, which contains N individuals, which are observed over T periods. The pooled model only provides consistent estimators for 𝛼 and 𝛽 if the zero conditional mean assumption E 𝜀𝑖𝑡 𝑋𝑖1 , … , 𝑋𝑖𝑇 = 0 is satisfied. Violation of this assumption causes the estimators to be biased and inconsistent. The panel data model Consider the basic linear panel data model, where we instead follow individuals over time: 𝑌𝑖𝑡 = 𝛼 + 𝑋𝑖𝑡 𝛽 + 𝜐𝑖𝑡 𝑖 = 1, … , 𝑁 𝑡 = 1, … , 𝑇,. ln panel data models, we assume that the error term 𝜐𝑖𝑡 can be divided into two parts that enter additively, so that: 𝜐𝑖𝑡 = 𝜂𝑖 + 𝜀𝑖𝑡 (1) Note that the 𝜂𝑖 this expression occurs without a time subscript! ln this specification, the error term is thus divided into one part that does not vary over time, 𝜂𝑖 , and one part that does vary over time 𝜀𝑖𝑡 The panel data model What is then 𝜂𝑖 ? It reflects factors that are unobserved by the econometrician and that do not vary over time for the individual This may include factors such as genes, early childhood environment, parental background, certain personality traits, “deep” preferences, etc. The assumptions we make about 𝜂𝑖 determines what type of panel data model to use The panel data model: random effects Panel data models are analyzed as either random effects models or fixed effects models ln the random effect model, we assume that: E 𝜂𝑖 𝑋𝑖1 , … , 𝑋𝑖𝑇 = 0 (2) Here, we assume that the unobserved factors that are fixed over time are independent of the value of the X variables for all time periods Is the random effects assumption realistic? The panel data model: random effects Note that the random effects assumption is similar to the zero conditional mean assumption The assumption says that unobserved, time-invariant, factors such as ability, preferences, parental background are independent of all included X variables For instance, if Y denotes earnings and X schooling, it says that such unobserved factors are independent of the level of schooling But this is exactly what we (often) do not believe and what we want to address! The panel data model: fixed effects In the fixed effect model, we relax the assumption and allow for: E 𝜂𝑖 𝑋𝑖1 , … , 𝑋𝑖𝑇 ≠ 0 (3) Here, we assume that the unobserved factors that are fixed over time are not independent of the value of the X variables for all time periods Without an experiment, this is the more realistic case As we will see, even if we allow for this particular type of break of the zero conditonal mean assumption, the fixed effects model may still give us consistent estimates of the causal effect! The fixed effects model To get more specific, consider the fixed-effect model: 𝑌𝑖𝑡 = 𝛼 + 𝑋𝑖𝑡 𝛽 + 𝜂𝑖 + 𝜀𝑖𝑡 , where 𝑋𝑖𝑡 is a vector of exogenous regressors and 𝜀𝑖𝑡 is independent over time and across individuals. By the fixed effects assumption, we do not rule out correlation between 𝜂𝑖 and 𝑋𝑖𝑡. As an example, 𝜂𝑖 could represent unobserved ability (at least the part of it that does not vary over time) Estimating the fixed effects model ln the specification above, the 𝜂𝑖 is a constant (or “fixed”) for each 𝑖 and therefore looks like a “dummy” variable for each 𝑖! What if we could get rid of the 𝜂𝑖 ? Thus, by including a dummy variable for each 𝑖 in the regression, we could control for 𝜂𝑖 But note that there are as many 𝜂𝑖 parameters as individuals, which could mean thousands of 𝜂𝑖 to estimate! Estimating the fixed effects model Even if we cannot estimate all the 𝜂𝑖 parameters, we can get rid of them using within estimation. We use a trick, where it turns out that including a dummy variable for each 𝑖 is algebraically the same as estimation in deviations from means To implement the trick, we first calculate the individual-specific averages over time, so that: 𝑌𝑖 = 𝑋𝑖 𝛽 + 𝜂𝑖 + 𝜀𝑖 where 1 𝑇 1 𝑇 1 𝑇 1 𝑇 𝑌𝑖 = σ𝑡=1 𝑌𝑖𝑡 𝑋𝑖 = σ𝑡=1 𝑋𝑖𝑡 𝜀𝑖 = σ𝑡=1 𝜀𝑖𝑡 𝜂𝑖 = σ𝑡=1 𝜂𝑖𝑡 𝑇 𝑇 𝑇 𝑇 Estimating the fixed effects model “the trick” Next subtract 𝑌𝑖 from 𝑌𝑖𝑡 : 𝑌𝑖𝑡 − 𝑌𝑖 = 𝑋෨𝑖𝑡 𝛽 + 𝜂𝑖 + 𝜀𝑖𝑡 − 𝑋𝑖 𝛽 − 𝜂𝑖 − 𝜀𝑖 = 𝑋𝑖𝑡 − 𝑋𝑖 𝛽 + (𝜀𝑖𝑡 − 𝜀𝑖 ). Note now that 𝜂𝑖 = 𝜂𝑖 since 𝜂𝑖 is always the same across time periods. This implies that we get the specification 𝑌෨𝑖𝑡 = 𝑋෨𝑖𝑡 𝛽 + 𝜀𝑖𝑡 ǁ 𝑖 = 1, … , 𝑁 t = 1, … , 𝑇 with 𝑌෨𝑖𝑡 = 𝑌𝑖𝑡 − 𝑌𝑖 𝑋෨𝑖𝑡 = 𝑋𝑖𝑡 − 𝑋𝑖 𝜀𝑖𝑡 ǁ = 𝜀𝑖𝑡 − 𝜀𝑖 The within estimator 𝛽መ𝑤𝑖𝑡ℎ𝑖𝑛 is then obtained by applying OLS. ln this specification, we got rid of 𝜂𝑖 , i.e. the fixed effects! Interpreting the fixed effects model Removing 𝜂𝑖 , i.e. the fixed effects, means that we implicitly control for all individual-specific factors—whether observable or unobservable—that are constant over time Thus we have removed a potentially large source of omitted variables bias We can do this even though we may not ever be able to observe or measure these unobserved and time-constant individual-specific factors. We now interpret the estimated effect, 𝛽, as the effect of a within-unit change in treatment. For this reason, the FE estimator is also called the within estimator. Some remarks on the fixed effects estimator The parameters 𝛽 are identified due to (within) variation in 𝑋𝑖𝑡 over time. Estimators for 𝜂𝑖 and 𝛽 are consistent if the asymptotics imply that 𝑇 becomes large. If instead 𝑇 is fixed and 𝑁 goes to infinity, only 𝛽መ𝑤𝑖𝑡ℎ𝑖𝑛 is consistent, but 𝜂𝑖 is not (so called incidental parameters). If 𝑁 is not too large, one could simply include dummy variables for each individual and estimate the original model by OLS. This provides the within estimators and 𝜂Ƹ 𝑖 in a single step. An alternative way to “kill” the fixed effects: the first-differences estimator Instead of the within estimation procedure, one could also use first- differences over time: 𝑌𝑖𝑡 − 𝑌𝑖𝑡−1 = 𝑋𝑖𝑡 𝛽 + 𝜂𝑖 + 𝜀𝑖𝑡 − 𝑋𝑖𝑡−1 𝛽 − 𝜂𝑖 − 𝜀𝑖𝑡−1 = 𝑋𝑖𝑡 − 𝑋𝑖𝑡−1 𝛽 + (𝜀𝑖𝑡 − 𝜀𝑖𝑡−1 ) 𝑡 = 2…,𝑇 or Δ𝑌𝑖𝑡 = Δ𝑋𝑖𝑡 𝛽 + Δ𝜀𝑖𝑡 , where taking first-differences eliminates 𝜂𝑖 from the model. If we perform OLS we obtain the first-difference estimator 𝛽መ𝑓𝑑𝑖𝑓𝑓. What additional assumptions are needed for the FE or first-difference model? So far we only addressed the assumptions about 𝜂𝑖. But what about 𝜀𝑖𝑡 , i.e. unobserved factors that are allowed to vary over time? For both the first-differences and within estimator to provide consistent estimates, we now need the regressors to be strictly exogenous: 𝐸 𝜀𝑖𝑡 𝑋𝑖1 , … , 𝑋𝑖𝑇 , 𝜂𝑖 = 0 𝑖 = 1…,𝑁 𝑡 = 1…,𝑇 The strict exogeneity assumption The strict exogeneity assumption is a version of the zero conditional mean assumption lt says that the part of the error term that is allowed to vary over time, 𝜀𝑖𝑡 , must be unrelated to the value of the treatment indicator or other control variables in any time period It would typically fail, if there is some time-specific unobserved shock that affect both the outcome and our X variable of interest What looks like an effect of X on Y may then simply reflect the influence of this shock The fixed effects model: Angrist and Pischke In the book (MHE), the authors use potential outcomes notation and use the example of the effect of unionship on wages Let 𝑌𝑖𝑡 equal the (log) earnings of worker 𝑖 at time 𝑡 and let 𝐷𝑖𝑡 denote his union status. The observed 𝑌𝑖𝑡 is either 𝑌0𝑖𝑡 or 𝑌1𝑖𝑡 , depending on union status. Suppose further that: 𝐸(𝑌0𝑡 |𝐴𝑖 ; 𝑋𝑖𝑡 , 𝑡, 𝐷𝑖𝑡 ) = 𝐸(𝑌0𝑡 |𝐴𝑖 ; 𝑋𝑖𝑡 , 𝑡) (4) The fixed effects model: Angrist and Pischke The expression above says that the potential outcome as untreated is independent of actual treatment status, conditional on unobserved worker ability, 𝐴𝑖 , and other observed covariates 𝑋𝑖𝑡 , and time 𝑡 ln other words, union status is as good as randomly assigned conditional on these factors If this is true, we would be able to get a consistent estimate of 𝐷𝑖𝑡 if we could control for, or somehow account for 𝐴𝑖. With a fixed effects model, we can accomplish this, as long as the unobserved factors is constant over time. Example: Freeman (1984), returns to union membership Freeman (1984) estimates the effect of union membership on wages Ideally, we would like to observe each individual’s potential outcome with and without union membership ln general, could we get at the potential outcomes by just observing the wages of members and non-members? If not, maybe the unobserved differences between members and non-members are constant over time? “Whatever makes us special is timeless” (Angrist and Kreuger 1998) Example: Freeman (1984), returns to union membership Example: Freeman (1984), returns to union membership Why are Freeman’s fixed effects estimates smaller than his cross- sectional estimates? Two explanations: 1. The fixed effect estimates are closer to the “true” causal effect of union membership. This would suggest that the effect was overestimated in the cross-sectional estimates 2. There are measurement errors in the union status variable. Unfortunately, the role of measurement errors normally becomes exaggerated in the fixed-effects model. The measurement error problem brings us to the potential pitfalls of the fixed effects method. Pitfalls of the fixed effects approach The measurement error problem The intuition for the measurement errors problem is that fixed effects models restricts the variation in the Xs to within individuals This also means that the fraction of the variation that reflects measurement errors may increase It can be shown that the downward bias that results from “classical” measurement error is greater in fixed effects models than in OLS The downward bias gets stronger, the stronger the correlation is between the x-variables in different periods Pitfalls of the fixed effects approach Impossible to estimate the effect of time-invariant regressors. Why? Because the deviation from the individual-specific mean will always be zero for such a variable We can therefore not estimate the effect of time-invariant factors such as gender, ethnicity, education (at least as an adult), etc. With a random effects specification we can estimate the time-invariant factors, but the problem is that the underlying assumptions of the RE-model are unrealistic in most applications Pitfalls of the fixed effects approach The effect is only identified for those who actually change treatment status The ones who do not change treatment status do not contribute to the estimates, since we are relying on within variation. Problem is that the sample who actually changes status in the causal variable of interest may be selective This may make it difficult to compare the OLS estimates with the FE estimates, since OLS exploits all observations Pitfalls of the fixed effects approach Violation of the strict exogeneity assumption ln many applications, the strict exogeneity assumption may be criticized. Selection into treatment may be based on unobserved factors that do vary over time, such as shocks, which would violate the strict exogeneity assumption. Thus, we have: 𝐸 𝜀𝑖𝑡 𝑋𝑖1 , … , 𝑋𝑖𝑇 , 𝜂𝑖 ≠ 0 (7) Fixed effects without a time dimension: exploiting family data such as siblings and twins The fixed effects approach does not require a time dimension! As long as important unobserved variables are shared by some group of individuals, they can be cancelled out using a fixed effects approach: Examples: 1. Twins: identical twins (monozygotic) twins share genetics and family background 2. Siblings: shares some genetics and family background Example 1 of fixed effects with twins: The effects of birth weight over the life cycle (Bharadwaj, Lundborg, Rooth (2015). What is the effect of early childhood health over the life cycle? The role of birth weight over the life cycle is examined in order to answer questions about the persistence of health inequalities at birth. With detailed register data on income, welfare payments etc, the extent to which lower birth weight children ever catch up to their heavier counterparts is examined. Example 1 of fixed effects with twins: Note that identifying the effect of birth weight is normally very difficult If one compares the birth weight of babies born in different families, there is a concern that those with low birth weight differ in unobservable ways from those with high birth weight For instance, those with low birth weight are more likely to be born in poorer families or in less healthy families To the extent that such factors are unobserved, it is likely that the birth weight coefficient partly or fully simply pick up such unobserved background factors We thus have an omitted variables problem (or “endogeneity” problem) Example 1 of fixed effects with twins: ln order to deal with omitted variables, data on the birth weight of almost all twins born in Sweden between 1926-1958 is exploited. These data are linked to register data on incomes and education from 1968 and onwards. Empirical specification: 𝑌𝑖𝑗𝑡 = 𝛽𝑡 𝐵𝑊𝑖𝑗 + 𝜂𝑗 + 𝜀𝑖𝑗𝑡 , where 𝑌𝑖𝑗𝑡 is log income of twin 𝑖 in twin pair 𝑗 at time 𝑡, 𝛽𝑡 is the coefficient of the birth weight variable 𝐵𝑊𝑖𝑗 , 𝜂𝑗 are twin-pair-specific unobserved factors, and 𝜀𝑖𝑗𝑡 is unobserved factors of twin 𝑖 Example 1 of fixed effects with twins: ln this specification, it is (partly) possible to deal with the omitted variables problem The reason is that the fixed effect 𝜂𝑖 , will account for the influence of genetics, the background and behaviors of the parents, and any environmental problems shared by twins, etc. To see this clearly, we can take the difference between twins within twin pairs (where the other twin is 𝑖 ′ ): 𝑌𝑖𝑗𝑡 − 𝑌𝑖 ′ 𝑗𝑡 = 𝛽𝑡 𝐵𝑊𝑖𝑗 − 𝐵𝑊𝑖 ′ 𝑗 + 𝜂𝑗 − 𝜂𝑗 + (𝜀𝑖𝑗𝑡 −𝜀𝑖 ′𝑗𝑡 ) = 𝛽𝑡 𝐵𝑊𝑖𝑗 − 𝐵𝑊𝑖 ′ 𝑗 + (𝜀𝑖𝑗𝑡 −𝜀𝑖 ′ 𝑗𝑡 ) Example 1 of fixed effects with twins: The key identifying assumption needed in order to give 𝛽𝑡 a causal interpretation is that 𝐵𝑊𝑖𝑗 − 𝐵𝑊𝑖 ′ 𝑗 is uncorrelated with 𝜀𝑖𝑗𝑡 − 𝜀𝑖 ′ 𝑗𝑡 Is this a strict assumption? Maybe, if we for instance think that parental investments in their kids is a function of birth weight. Or if differences in birth weight are related to differences in cognition. If parents try to compensate for the lower birth weight of one twin, the estimated effect is the effect of birth weight that remains despite parents’ best attempts to compensate Example 1 of fixed effects with twins: results Example 2: Critical periods in the development of cognitive skills and health (van den berg, Lundborg 2014) Very difficult to study the causal effect of poor circumstances during childhood on later life outcomes Periods during childhood in which poor conditions have particularly bad consequences for development are called “critical periods” Some famous evidence comes from studies Romanian orphans, who were rescued from incredibly bad conditions by adoption parents from Western countries These results may reflect self-selection, however, if adoption parents picked out the least “damaged” children from the orphanages Example 2: Critical periods in the development of cognitive skills and health (van den berg et al (2014) Van den Berg et al. (2014) exploit data on immigrant brothers who migrated to Sweden from different countries The study exploits that the brothers migrated from more or less poor conditions to a richer country The brothers entered Sweden at the same point in time, calendar-wise, but at different development stages (ages) By comparing the outcomes of brothers who entered at the same time (fixed effects) but at different stages, we may be able to identify “critical periods” in the development height Critical periods in the development of height at age 18: results Example 2: Critical periods in the development height By imposing brother fixed effects, it is possible to difference out everything unobserved at the family level This includes factors such as reasons for migrating, parental background, language, which all may be important omitted variables Brother fixed effects also address the self-selection of the families who migrate, since the unobserved selection factors are at the family level! The results show larger effects if migrating from poorer countries, as one would expect Summary fixed effects A fixed effects estimator allows us to control for certain types of omitted variables The method allows us to control for the unobserved factors that are fixed over time (time-invariant) or space The method cannot account for the influence of unobserved factors that vary over time (or space) A useful application of fixed effects estimators is on siblings or twin data Appendix: Panel data and fixed effects Comparing the first-differences and the within estimators If 𝑇 = 2, the within estimator and first-difference estimator are the same. To see this note that the within model computes individual means: 1 𝑋𝑖 = (𝑋𝑖1 + 𝑋𝑖2 ) (5) 2 And, in mean deviation form, we have: 1 1 1 ෨ 𝑋𝑖2 = 𝑋𝑖2 − 𝑋𝑖 = 𝑋𝑖2 − 𝑋𝑖1 + 𝑋𝑖2 = 𝑋𝑖2 − 𝑋𝑖1 , (6) 2 2 2 which will always be half the first-difference. So effectively the same model. Comparing the first-differences and the within estimators If 𝑈𝑖𝑡 is uncorrelated over time, the within estimator is more efficient than the first-difference estimator. If the 𝑈𝑖𝑡 are serially correlated, the first-difference estimator is more efficient. If strict exogeneity is violated, the first-difference estimator and the within estimator become both inconsistent and have different probability limits.