Econometrics Lecture 10: Panel Data (PDF)
Document Details
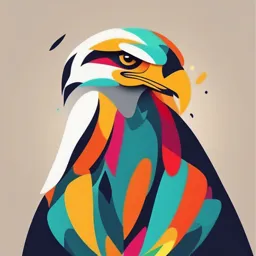
Uploaded by JollyMoldavite4497
Universitat Pompeu Fabra
2024
Tags
Summary
This document provides a lecture on the topic of panel data in econometrics. It covers the fundamental concepts and various approaches to analyzing panel datasets. It discusses pooled cross-section data, fixed effects, and time-demeaned models.
Full Transcript
Lecture 10: An Introduction to Panel Data 25117 - Econometrics Universitat Pompeu Fabra November 20th, 2024 What we learned in the last lesson - Instrumental variables regression is a way to estimate causal coefficients when one or more regressors are correlat...
Lecture 10: An Introduction to Panel Data 25117 - Econometrics Universitat Pompeu Fabra November 20th, 2024 What we learned in the last lesson - Instrumental variables regression is a way to estimate causal coefficients when one or more regressors are correlated with the error term. - Endogenous variables are correlated with the error term in the equation of interest; exogenous variables are uncorrelated with this error term. - For an instrument to be valid, it must be (1) correlated with the included endogenous variable and (2) exogenous. - IV regression requires at least as many instruments as included endogenous variables. - The TSLS estimator has two stages. - First, the included endogenous variables are regressed against the included exogenous variables and the instruments. - Second, the dependent variable is regressed against the included exogenous variables and the predicted values of the included endogenous variables from the first-stage regression(s). - Weak instruments (instruments that are nearly uncorrelated with the included endogenous variables) make the TSLS estimator biased and TSLS confidence intervals and hypothesis tests unreliable. - If an instrument is not exogenous, the TSLS estimator is inconsistent. Starting with Pooled Data From Pooled to Panel Data Fixed Effects Models References 2 / 54 Starting with Pooled Data Starting with Pooled Data From Pooled to Panel Data Fixed Effects Models References 3 / 54 Cross-Section So far we have been mostly focused on cross-sectional data — a type of data collected at a single point in time or over a very short period, capturing information from multiple subjects or entities at that specific moment. In other words, it provides a snapshot or a “cross-section” of a population or a phenomenon at a particular point in time. Starting with Pooled Data From Pooled to Panel Data Fixed Effects Models References 4 / 54 Pooled Cross-Section A natural extension of the cross-sectional data is the pooled cross-sectional data — where cross-sectional data is gathered at multiple points in time and where each cross-sectional study is independent of the others. In other words, different samples of subjects or entities are observed during each data collection period. Starting with Pooled Data From Pooled to Panel Data Fixed Effects Models References 5 / 54 Pooled Cross-Section Many surveys, randomly sampling individuals, families, or firms are repeated at regular time intervals (e.g., CPS in the U.S.) Pooled together =⇒ independently pooled cross section Pros - Statistical inference is the same as for Cons cross-sectional methods - Larger sample size (i.e., lower standard - Populations have different distribution errors) across time periods, thus they are not - Enables us to estimate trends conditional identically distributed on explanatory variables. → we can allow the intercept to differ - Enables us to estimate how the effect of across periods! (how?) one factor has changed over time (e.g., how did the gender wage gap change?) Starting with Pooled Data From Pooled to Panel Data Fixed Effects Models References 6 / 54 Fertility over time Starting with Pooled Data From Pooled to Panel Data Fixed Effects Models References 7 / 54 Fertility over time Starting with Pooled Data From Pooled to Panel Data Fixed Effects Models References 8 / 54 Fertility over time Starting with Pooled Data From Pooled to Panel Data Fixed Effects Models References 9 / 54 Fertility over time Starting with Pooled Data From Pooled to Panel Data Fixed Effects Models References 10 / 54 Fertility over time Starting with Pooled Data From Pooled to Panel Data Fixed Effects Models References 11 / 54 Fertility over time Starting with Pooled Data From Pooled to Panel Data Fixed Effects Models References 12 / 54 Fertility over time Starting with Pooled Data From Pooled to Panel Data Fixed Effects Models References 13 / 54 Fertility over time From Lecture 7 — We can use year-specific intercepts by including year-specific dummies Remember from Lecture 7 — the base year here is...? Sharp decline in the number of kids per woman in the 1980s which is not explained by changes in main demographics Starting with Pooled Data From Pooled to Panel Data Fixed Effects Models References 14 / 54 Gender Gap As in Lecture 7, we can allow for different intercepts and slopes gradients by interacting a dummy and a continuous variable Example: - Pooled 2 cross-sectional datasets (1978 and 1985) - Data on wages, educational attainment, and gender - Questions: - Did the gender wage gap change from 1978 to 1985? - Did the return to education change from 1978 to 1985? ln(wage) = β0 + β1 11985 + β2 Educ + β3 (Educ × 11985 ) + β4 Female + β5 (Female × 11985 ) +β6 Experience + β7 Experience2 + β8 Union + u Starting with Pooled Data From Pooled to Panel Data Fixed Effects Models References 15 / 54 Gender Gap Starting with Pooled Data From Pooled to Panel Data Fixed Effects Models References 16 / 54 Testing Differences in Pooled regressions When all variables are interacted with the time dummies, the regression specification is equivalent to estimating separate regressions — one for each year. As in Lecture 7, one can test whether the population regression lines differ across two different years by running a simple Chow test. First, run the fully interacted model (year1 serves as the base period) Yi = β0 + β1 X1i + · · · + βk Xki + βk +1 1year2 + βk +2 (1year2 × X1i ) + · · · + β2k +1 (1year2 × Xki ) + ui Then test βk +1 = βk+2 = · · · = β2k +1 = 0 We can also test whether the intercepts differ between the 2 groups (H0 : βk+1 = 0) or whether the slopes differ (H0 : βk+2 = · · · = β2k +1 = 0) Starting with Pooled Data From Pooled to Panel Data Fixed Effects Models References 17 / 54 From Pooled to Panel Data Starting with Pooled Data From Pooled to Panel Data Fixed Effects Models References 18 / 54 What is a panel dataset? A panel dataset (also called longitudinal data) contains observations on multiple entities (e.g., individuals, states, companies, etc.), observed at two or more points in time (e.g., days, months, years, decades, etc.). For example, - Data on 420 California school districts in 1999 and again in 2000, for 840 observations total. - Data on 50 U.S. states, each state is observed in 3 years, for a total of 150 observations. - Data on 1000 individuals in four different months, for 4000 observations total. Panel data with k regressors: {X1,it , X2,it ,..., Xk,it , Yit } where i = 1,... , n and t = 1,... , T When all k + 1 variables are observed for all units i at all points in time t (i.e., no missing observations), we say the panel is balanced. Starting with Pooled Data From Pooled to Panel Data Fixed Effects Models References 19 / 54 Panel Data Panel data is also known as longitudinal data or cross-sectional time-series data It involves observations of multiple entities over multiple time periods. We say a panel is balanced if all entities are observed in each time period, and there are no missing observations. We will focus on the case where N >> T Starting with Pooled Data From Pooled to Panel Data Fixed Effects Models References 20 / 54 Why are panel data useful? With panel data, we can control for factors that: - Vary across units but not over time (in the sample period) ←− (we will focus on that case first) - Vary over time (in the sample period) but not across units These ‘black boxes’ could contain omitted variables, which are typically unobserved or unmeasured — and therefore cannot be included in the regression using multiple regression. For example, - Average individual ability or preferences - Cultural attitudes - First geography (distances, topography, etc.) components - Aggregate trends Here’s the key idea: - If an omitted variable does not change over time or across units, then any changes in Y over time and across units cannot be caused by the omitted variable. Starting with Pooled Data From Pooled to Panel Data Fixed Effects Models References 21 / 54 Example of a panel data set You want to study the impact of alcohol taxes on traffic death. - Observational unit: U.S. state (n = 48), yearly (T = 7) between 1982 and 1988 - Balanced panel, so total observations = 7 × 48 = 336 - Variables: - Traffic fatality rate ( traffic deaths in that state in that year, per 10,000 state residents) - Tax on a case of beer - Other (legal driving age, drunk driving laws, etc.) Consider the simple following specification: Fatalitiesi,t = β0 + β1 BeerTaxi,t + ui,t where t is a specific year, so we are just looking at individual cross-sections... Starting with Pooled Data From Pooled to Panel Data Fixed Effects Models References 22 / 54 Traffic Fatalities vs. Beer Tax — 1982 Starting with Pooled Data From Pooled to Panel Data Fixed Effects Models References 23 / 54 Traffic Fatalities vs. Beer Tax — 1983 Starting with Pooled Data From Pooled to Panel Data Fixed Effects Models References 24 / 54 Traffic Fatalities vs. Beer Tax — 1984 Starting with Pooled Data From Pooled to Panel Data Fixed Effects Models References 25 / 54 Traffic Fatalities vs. Beer Tax — 1985 Starting with Pooled Data From Pooled to Panel Data Fixed Effects Models References 26 / 54 Traffic Fatalities vs. Beer Tax — 1986 Starting with Pooled Data From Pooled to Panel Data Fixed Effects Models References 27 / 54 Traffic Fatalities vs. Beer Tax — 1987 Starting with Pooled Data From Pooled to Panel Data Fixed Effects Models References 28 / 54 Traffic Fatalities vs. Beer Tax — 1988 Starting with Pooled Data From Pooled to Panel Data Fixed Effects Models References 29 / 54 Traffic Fatalities vs. Beer Tax – First Differences Simple analysis of individual cross sections return weird results... Higher alcohol taxes, more traffic deaths? Arguably, omitted factors could cause omitted variable bias (which ones?) Now consider the simple panel specification: Fatalitiesi,t = β0 + δ0 11988 + β1 BeerTaxi,t + β2 Zi + ui,t where t ∈ {1982, 1988}. If Zi is not observed, we could run in an OVB issue. But taking the first difference eliminates the value of Zi ! Fatalitiesi,1988 − Fatalitiesi,1982 = δ0 + β1 (BeerTaxi,1988 − BeerTaxi,1982 ) + (ui,1988 − ui,1982 ) This “difference” equation can be estimated by OLS, even though Zi is unobserved! Starting with Pooled Data From Pooled to Panel Data Fixed Effects Models References 30 / 54 Traffic Fatalities vs. Beer Tax – First Differences Starting with Pooled Data From Pooled to Panel Data Fixed Effects Models References 31 / 54 First Differences when T > 2 We can also use differencing with more than two time periods: Fatalitiesi,t = β0 + δ1 11983 + δ2 11984 + · · · + δ6 11988 + β1 BeerTaxi,t + β2 Zi + ui,t That is, Fatalitiesi,1982 = β0 + β1 BeerTaxi,1982 + β2 Zi + ui,1982 Fatalitiesi,1983 = β0 + δ1 11983 + β1 BeerTaxi,1983 + β2 Zi + ui,1983 Fatalitiesi,1984 = β0 + δ2 11984 + β1 BeerTaxi,1984 + β2 Zi + ui,1984 ··· Fatalitiesi,1988 = β0 + δ6 11988 + β1 BeerTaxi,1988 + β2 Zi + ui,1988 We do so by taking differences between adjacent time periods: ∆Fatalitiesi,t = δ1 + ∆δ2 11984 + · · · + ∆δ6 11988 + β1 ∆BeerTaxi,t + ∆ui,t Starting with Pooled Data From Pooled to Panel Data Fixed Effects Models References 32 / 54 Limits of First-Differences - The main limitation of using FD compared to other methods is probably that we lose the initial period (there are N(T − 1) observations) - Because FD builds on ensuing observations, missing data can be very problematic (imagine if we only observe some i once every other year) - Typically, the variation in the differenced independent variable is much smaller than the variation in the original independent variable. Thus, imprecise estimates could happen from the FD estimator. - Serial correlation (more on that later) - First differencing can enlarge classical errors-in-variable (i.e., measurement error) bias Starting with Pooled Data From Pooled to Panel Data Fixed Effects Models References 33 / 54 Fixed Effects Models Starting with Pooled Data From Pooled to Panel Data Fixed Effects Models References 34 / 54 Fixed Effects Regressions The general FE model reads Fatalitiesi,t = β0 + β1 BeerTaxi,t + β2 Zi + ui,t For example, in the case of California, FatalitiesCA,t = β0 + β1 BeerTaxCA,t + β2 ZCA + uCA,t = (β0 + β2 ZCA ) + β1 BeerTaxCA,t + uCA,t = αCA + β1 BeerTaxCA,t + uCA,t Where αCA is the intercept for California, and β1 is the slope. The intercept is unique to CA, but the slope is the same in all the states: parallel lines. Starting with Pooled Data From Pooled to Panel Data Fixed Effects Models References 37 / 54 Fixed Effects Regressions Two equivalent ways of writing the same specification: Yi,t = β0 + β1 Xi,t + γ1 Di |i=1 +γ2 Di |i=2 + · · · + γn Di |i=n +ui,t where Di |i=n = 1 if i = n (i.e., state #n). And, Yi,t = αi + β1 Xi,t + ui,t Starting with Pooled Data From Pooled to Panel Data Fixed Effects Models References 38 / 54 Fixed Effects Regressions Including n extra (fixed effect) dummies can be computationally intensive when n is large. What regression softwares typically do is to (time-)demean (within transformation) the specification: T T 1X 1X Ȳi = αi + β1 Xi,t + ui,t T T t=1 t=1 Which yields to T T 1X 1X Yi,t − Ȳi = β1 (Xi,t − Xi,t ) + (ui,t − ui,t ) T T t=1 t=1 which can be estimated by simple OLS, that is Ỹi,t = β1 X̃i,t + ũi,t and β1 isolates the impact of changes in X within a unit, ignoring the constant, unit-specific factors influencing both X and Y. Starting with Pooled Data From Pooled to Panel Data Fixed Effects Models References 39 / 54 Fixed Effects Regressions Starting with Pooled Data From Pooled to Panel Data Fixed Effects Models References 40 / 54 Fixed Effects Regressions By analogy, we can also include time FEs (i.e., they are the same across unit but vary in time). What omitted variable could be captured by time FEs in the traffic fatalities vs. beer taxes case? The population line for, say, 1985 reads Fatalitiesi,1985 = β0 + β1 BeerTaxi,1985 + β2 Z1985 + ui,1985 = (β0 + β2 Z1985 ) + β1 BeerTaxi,1985 + ui,1985 = λ1985 + β1 BeerTaxi,1985 + ui,1985 Where λ1985 is the intercept for 1985, and β1 is the slope. The dummies notation holds, as in the preceding example with unit FEs. Starting with Pooled Data From Pooled to Panel Data Fixed Effects Models References 41 / 54 Fixed Effects Regressions Including T extra (fixed effect) dummies can be computationally intensive when T is large. As before, we can transform the panel model specification into a simple OLS one by demeaning: n n 1X 1X Ȳt = λt + β1 Xi,t + ui,t n n i=1 i=1 Which yields to n n 1X 1X Yi,t − Ȳt = β1 (Xi,t − Xi,t ) + (ui,t − ui,t ) n n i=1 i=1 which can be estimated by simple OLS, that is Y̌i,t = β1 X̌i,t + ǔi,t and β1 captures the effect of changes in X within a specific time period, accounting for shared temporal factors that affect both X and Y at the same time. Starting with Pooled Data From Pooled to Panel Data Fixed Effects Models References 42 / 54 Raw Panel Data Starting with Pooled Data From Pooled to Panel Data Fixed Effects Models References 43 / 54 Accounting for αi Starting with Pooled Data From Pooled to Panel Data Fixed Effects Models References 44 / 54 Accounting for λt Starting with Pooled Data From Pooled to Panel Data Fixed Effects Models References 45 / 54 Both unit and time fixed effects Starting with Pooled Data From Pooled to Panel Data Fixed Effects Models References 46 / 54 Both unit and time fixed effects Starting with Pooled Data From Pooled to Panel Data Fixed Effects Models References 47 / 54 The Fixed Effects Simple Regression Assumptions Consider the following regression model Yit = αi + β1 Xi,t + ui,t 1 E(ui | Xi1 , Xi2 ,... , XiT , αi , λt ) = 0 2 (Xi1 , Xi2 ,... , XiT , ui1 , ui2 ,... , uiT ) are i.i.d. draws from their joint distribution 3 Large outliers are unlikely: (Xit , ui,t ) have fourth moments. 4 There is no perfect multicollinearity (multiple X ’s) Assumption 1 means that the error term cannot be correlated with any present, past, or future value of X (no omitted lagged effects, no feedback loop!) Assumption 2 means that variables are independent across units but makes no such restriction within a unit. Assumption 3 and 4 did not change (see Lecture 7). Starting with Pooled Data From Pooled to Panel Data Fixed Effects Models References 48 / 54 Serial Correlation - Following up on Assumption 2, when ui,t is correlated over time within a unit, i.e., when cov (ui,t , ui,t+j ) ̸= 0 we say that ui,t is autocorrelated, or serially correlated. - If entities are sampled by simple random sampling, then (ui1 , ui2 ,... , uiT ) is independent of - But in many panel data applications, ui,t is serially correlated (uj1 , uj2 ,... , ujT )... −→ Clustering/Moulton’s problem (1986) Starting with Pooled Data From Pooled to Panel Data Fixed Effects Models References 49 / 54 Clustered SEs The usual OLS standard errors (both homoskedasticity-only and heteroskedasticity-robust) will in general be wrong because they assume that uit is serially uncorrelated. In practice, they often understate the true sampling uncertainty: if uit are correlated over time, you don’t have as much information (as much random variation) as you would if uit were uncorrelated! To see this, consider the panel the panel (time-)demeaned regression estimator Pn PT Pn PT 1 Pn PT i=1 t=1 (Xit − X̄i )(Yit − Ȳi ) i=1 t=1 X̃it Ỹit nT i=1 t=1 X̃it ũit β̂1 = Pn PT = Pn PT = β1 + Pn PT 2 1 i=1 t=1 (Xit − X̄i ) 2 i=1 t=1 X̃it nT i=1 t=1 X̃it2 This completely mirrors the basic OLS estimator equation!!! Starting with Pooled Data From Pooled to Panel Data Fixed Effects Models References 50 / 54 Clustered SEs Rearranging the terms, q P q P q P 1 n 1 T 1 n √ n i=1 T t=1 X̃it ũit n i=1 ηi nT (β̂1 − β1 ) = Pn PT = 1 nT i=1 t=1 X̃it2 Q̂X̃ Because ηi is i.i.d across units (by Assumption 2), of mean 0 (by Assumption 1), with finite variance (by Assumption 3), then (same reasoning as in Lecture 3!) q P 1 n √ i=1 ηi n σ2 η nT (β̂1 − β1 ) = ∼ N 0, 2 as n grows large Q̂X̃ QX̃ Now, note that r T ! T 1X 1 X 1 h i ση2 = var X̃it ũit = var X̃it ũit = var X̃i1 ũi1 + · · · + X̃iT ũiT T T T t=1 t=1 Starting with Pooled Data From Pooled to Panel Data Fixed Effects Models References 51 / 54 Clustered SEs Remember that var (X + Y ) = var (X ) + var (Y ) + 2cov (X , Y )... therefore 1h ση2 = var (X̃i1 ũi1 ) + · · · + var (X̃iT ũiT ) + T i 2cov (X̃i1 ũi1 , X̃i2 ũi2 ) + · · · + 2cov (X̃i,T −1 ũi,T −1 , X̃iT ũiT ) The heteroskedasticity-robust variance formula seen so far misses all the (auto)covariances in the final part the equation... If there is a serial correlation, the usual heteroskedasticity-robust variance estimator is inconsistent! Clustered SEs for panel data are the logical extension of heteroskedastic (HR) SEs for cross-section. In cross-section regression, HR SEs are valid whether or not there is HR. In panel data regression, clustered SEs are valid whether or not there is HR and/or serial correlation. To conclude, the HR-robust clustered SEs reads v u 1 sη̂2 u SE(β̂1 ) = t nT Q̂ 2 X̃ Starting with Pooled Data From Pooled to Panel Data Fixed Effects Models References 52 / 54 Final Remarks on Clustered SEs We can cluster SEs by unit of observation (e.g., serial correlation within states), but also across units (e.g., regions above states) if we suspect potential correlation in observations within these higher-level clusters. However, asymptotic inference (as n grows large) supposes we have a large number of clusters... This is not always the case. When there are too few clusters and serial correlation, standard errors might be biased! In the Traffic Fatalities vs. Beer Tax example, is 48 (states) a number of clusters that is large enough? What should be done when there are too few clusters? This is out of the scope of this (introductory) lecture! Starting with Pooled Data From Pooled to Panel Data Fixed Effects Models References 53 / 54 Material I – Textbooks: - Introduction to Econometrics, 4th Edition, Global Edition, by Stock and Watson – Chapters 10 - 13 - Introductory Econometrics, 5th Edition, A Modern Approach, by Jeff. Wooldridge – Chapters 13-14. - Causal Inference, The Mixtape, by Scott Cunningham – Chapter 7 – Papers: - Ashenfelter, O., & Krueger, A. (1994). Estimates of the economic return to schooling from a new sample of twins. The American economic review, 1157-1173. - Ashenfelter, O., & Rouse, C. (1998). Income, schooling, and ability: Evidence from a new sample of identical twins. The Quarterly Journal of Economics, 113(1), 253-284. - Bertrand, M., Duflo, E., & Mullainathan, S. (2004). How much should we trust differences-in-differences estimates?. The Quarterly journal of economics, 119(1), 249-275. - Tanaka, S. (2014). Does abolishing user fees lead to improved health status? Evidence from post-apartheid South Africa. American economic Journal: economic policy, 6(3), 282-312. Starting with Pooled Data From Pooled to Panel Data Fixed Effects Models References 54 / 54