Lecture 5 – Perceiving and Recognizing Objects PDF
Document Details
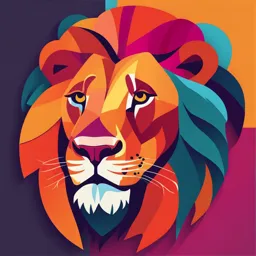
Uploaded by GlamorousComplex9991
York University
2024
Tags
Summary
This document is a lecture about object recognition processes, including the visual pathway and receptive fields in the brain. The lecture notes cover various aspects of object recognition, such as the roles of different brain areas and computational methods in object recognition. The document uses numerous figures and diagrams to illustrate the concepts.
Full Transcript
Lecture 5 Perceiving and Recognizing Objects © 2024 Oxford University Press 2 Perceiving and Recognizing Objects 2 4.1 From Simple Lines and Edges to Properties...
Lecture 5 Perceiving and Recognizing Objects © 2024 Oxford University Press 2 Perceiving and Recognizing Objects 2 4.1 From Simple Lines and Edges to Properties of Objects 4.2 What and Where (How) Pathways 4.3 The Problems of Perceiving and Recognizing Objects 4.4 Mid-Level Vision 4.5 Object Recognition © 2024 Oxford University Press 3 4.1 From Simple Lines and Edges to Properties of Objects 3 How do we recognize objects? – Retinal ganglion cells and LGN = Spots – Primary visual cortex = Bars How do spots and bars get turned into objects and surfaces? – Clearly our brains are doing something pretty sophisticated beyond V1. © 2024 Oxford University Press 4 4.1 From Simple Lines and Edges to Properties of Objects 4 Objects in the brain – Extrastriate cortex: The region of cortex bordering the primary visual cortex and containing multiple areas involved in visual processing. V2, V3, V4, Inferotemporal Cortex, etc. © 2024 Oxford University Press 5 4.1 From Simple Lines and Edges to Properties of Objects 5 Objects in the brain (continued) – After extrastriate cortex, processing of object information is split into a “what” pathway and a “where / how” pathway. “Where / how” pathway is concerned with the locations and shapes of objects but not their names or functions. “What” pathway is concerned with the names and functions of objects regardless of location. © 2024 Oxford University Press FIGURE 4.2 The main visual areas of the macaque monkey cortex © 2024 Oxford University Press FIGURE 4.3 A “wiring” diagram for the monkey visual system © 2024 Oxford University Press FIGURE 4.4 The main visual areas of the human cortex © 2024 Oxford University Press 9 4.1 From Simple Lines and Edges to Properties of Objects 9 The receptive fields of extrastriate cells are more sophisticated than those in striate cortex. They respond to visual properties important for perceiving objects. – For instance, “boundary ownership.” For a given boundary, which side is part of the object and which side is part of the background? © 2024 Oxford University Press FIGURE 4.5 Edges and the receptive field © 2024 Oxford University Press 11 4.1 From Simple Lines and Edges to Properties of Objects 11 The same visual input occurs in (a), (b) and (c) and a V1 neuron would respond the same to all three. A V2 neuron might respond more to (b) than (c) because the black edge is owned by the square in (b) but not in (c). © 2024 Oxford University Press 12 4.2 What and Where Pathways 12 Inferotemporal (IT) cortex: Part of the cerebral cortex in the lower portion of the temporal lobe, important for object recognition. – Part of the “what” pathway Lesion, in neuropsychology: 1.(n.) A region of damaged brain. 2.(v.) To destroy a section of the brain. © 2024 Oxford University Press 13 4.2 What and Where Pathways 13 When IT cortex is lesioned, it leads to agnosias. – Agnosia: Failure to recognize objects in spite of the ability to see them. © 2024 Oxford University Press 14 4.2 What and Where Pathways 14 Receptive field properties of IT neurons – Very large—some cover half the visual field – Don’t respond well to spots or lines – Do respond well to stimuli such as hands, faces, or objects © 2024 Oxford University Press 15 4.2 What and Where Pathways 15 Grandmother cells – Could a single neuron be responsible for recognizing your grandmother? – (not really) – Quiroga et al. (2005) identified a cell that responds specifically to Jennifer Aniston. © 2024 Oxford University Press FIGURE 4.6 A Jennifer Aniston cell © 2024 Oxford University Press 17 4.2 What and Where Pathways 17 Object recognition is fast! – Studies indicate that object recognition happens in as little as 150 ms. – This is such a short time that there cannot be a lot of feedback from higher brain areas. Feed-forward process: A process that carries out a computation (e.g., object recognition) one neural step after another, without the need for feedback from a later stage to an earlier stage. © 2024 Oxford University Press 18 4.2 What and Where Pathways 18 Reverse-hierarchy theory Hochstein & Ahissar (2002) proposed that feed-forward processes give initial, crude information about objects by activating high-level parts of visual cortex. More detailed information becomes available when activation flows back down the hierarchy to lower visual areas where the detailed information is preserved. © 2024 Oxford University Press FIGURE 4.9 The problem of object recognition © 2024 Oxford University Press 20 4.3 The Problems of Perceiving and Recognizing Objects 20 The problem of object recognition – The pictures were just a bunch of pixels on a screen, but in each case you perceived an elephant. – How did you recognize all four images as depicting an elephant? – How does your visual system move from points of light, like pixels, to whole entities in the world, like elephants? © 2024 Oxford University Press FIGURE 4.10 Local elements, global elephants © 2024 Oxford University Press 22 4.4 Mid-Level Vision 22 Mid-level vision: A loosely defined stage of visual processing that comes after basic features have been extracted from the image (low-level vision) and before object recognition and scene understanding (high-level vision). – Involves the perception of edges and surfaces – Determines which regions of an image should be grouped together into objects © 2024 Oxford University Press 23 4.4 Mid-Level Vision 23 Finding edges – How do you find the edges of objects? – Cells in primary visual cortex have small receptive fields. – How do you know which edges go together and which ones don’t? © 2024 Oxford University Press 24 4.4 Mid-Level Vision 24 Computer-based edge detectors are not as good as humans. – Sometimes computers don’t find edges that humans see easily. © 2024 Oxford University Press FIGURE 4.12 Seeing invisible contours © 2024 Oxford University Press 26 4.4 Mid-Level Vision 26 Illusory contour: A contour that is perceived even though nothing changes from one side of the contour to the other. © 2024 Oxford University Press FIGURE 4.13 Illusory contours © 2024 Oxford University Press 28 4.4 Mid-Level Vision 28 Do you see a white arrow sitting on top of some circles? There is no arrow! Just some “Pac-Men” and disconnected lines. © 2024 Oxford University Press FIGURE 4.14 The making of illusory contours © 2024 Oxford University Press 30 4.4 Mid-Level Vision 30 Rules of evidence – Gestalt: In German, “form” or “whole.” – Gestalt psychology: “The whole is greater than the sum of its parts.” Opposed to other schools of thought, such as structuralism, that emphasize the basic elements of perception – Gestalt grouping rules: A set of rules that describe when elements in an image will appear to group together. © 2024 Oxford University Press 31 4.4 Mid-Level Vision 31 Rules of evidence (continued) – Good continuation: A Gestalt grouping rule stating that two elements will tend to group together if they lie on the same contour. © 2024 Oxford University Press FIGURE 4.16 The principle of good continuation © 2024 Oxford University Press FIGURE 4.17 Good continuation isn’t everything © 2024 Oxford University Press FIGURE 4.18 How “sensible” are these rules? © 2024 Oxford University Press 35 4.4 Mid-Level Vision 35 Rules of evidence (continued) – Some contours in an image will group because of good continuation. – Can you find the shape embedded within the field of lines in (a) on the next slide? © 2024 Oxford University Press FIGURE 4.15 Contour completion © 2024 Oxford University Press 37 4.4 Mid-Level Vision 37 Texture segmentation and grouping – Texture segmentation: Carving an image into regions of common texture properties. – Texture grouping depends on the statistics of textures in one region versus another. © 2024 Oxford University Press FIGURE 4.20 Regions defined by texture © 2024 Oxford University Press 39 4.4 Mid-Level Vision 39 Gestalt grouping rules – Similarity: Similar looking items tend to group. – Proximity: Items that are near each other tend to group. © 2024 Oxford University Press FIGURE 4.22 Similarity and proximity © 2024 Oxford University Press 41 4.4 Mid-Level Vision 41 Camouflage – Animals exploit Gestalt grouping principles to group into their surroundings. – Sometimes camouflage is used to confuse the observer. © 2024 Oxford University Press FIGURE 4.23 Camouflage © 2024 Oxford University Press 43 4.4 Mid-Level Vision 43 Ambiguity and perceptual “committees” – A metaphor for how perception works Committees must integrate conflicting opinions and reach a consensus. – Many different and sometimes competing principles are involved in perception. – Perception results from the consensus that emerges. © 2024 Oxford University Press 44 4.4 Mid-Level Vision 44 Committee rules: Honor physics and avoid accidents – Ambiguous figure: A visual stimulus that gives rise to two or more interpretations of its identity or structure. Perceptual committees tend to obey the laws of physics. © 2024 Oxford University Press FIGURE 4.24 The Necker cube © 2024 Oxford University Press 46 4.4 Mid-Level Vision 46 Committee rules: Honor physics and avoid accidents (continued) – Accidental viewpoint: A viewing position that produces some regularity in the visual image that is not present in the world. Perceptual committees assume viewpoints are not accidental. © 2024 Oxford University Press FIGURE 4.26 Accidental viewpoint © 2024 Oxford University Press FIGURE 4.27 Accidental tourist © 2024 Oxford University Press 49 4.4 Mid-Level Vision 49 Figure and ground – Figure-ground assignment: The process of determining that some regions of an image belong to a foreground object (figure) and other regions are part of the background (ground). © 2024 Oxford University Press FIGURE 4.29 The ambiguous Rubin vase/face figure © 2024 Oxford University Press 51 4.4 Mid-Level Vision 51 Gestalt figure-ground assignment principles – Surroundedness: The surrounding region is likely to be ground. – Size: The smaller region is likely to be figure. – Symmetry: A symmetrical region tends to be seen as figure. © 2024 Oxford University Press FIGURE 4.30 Parallel contours © 2024 Oxford University Press 53 4.4 Mid-Level Vision 53 Gestalt figure-ground assignment principles (continued) – Parallelism: Regions with parallel contours tend to be seen as figure. – Relative motion: If one region moves in front of another, then the closer region is figure. © 2024 Oxford University Press 54 4.4 Mid-Level Vision 54 Dealing with occlusion – Relatability: The degree to which two line segments appear to be part of the same contour. © 2024 Oxford University Press FIGURE 4.31 Relatability © 2024 Oxford University Press 56 4.4 Mid-Level Vision 56 Nonaccidental feature: A feature of an object that is not dependent on the exact (or accidental) viewing position of the observer. – T junctions: Indicate occlusion. Top of T is in front and stem of T is in back. – Y junctions: Indicate corners facing the observer. – Arrow junctions: Indicate corners facing away from the observer. © 2024 Oxford University Press FIGURE 4.32 Line junctions are nonaccidental features © 2024 Oxford University Press 58 4.4 Mid-Level Vision 58 Parts and wholes – Global superiority effect: The properties of the whole object take precedence over the properties of parts of the object. © 2024 Oxford University Press FIGURE 4.33 The global superiority effect © 2024 Oxford University Press FIGURE 4.34 Finding parts from object boundaries © 2024 Oxford University Press 61 4.4 Mid-Level Vision 61 Summarizing middle vision Five principles of middle vision: 1. Bring together that which should be brought together 2. Split asunder that which should be split asunder 3. Use what you know 4. Avoid accidents 5. Seek consensus and avoid ambiguity © 2024 Oxford University Press 62 4.4 Mid-Level Vision 62 From metaphor to formal model – “Perceptual committees” is a metaphor, but there are formal, mathematical models that can be used. – The Bayesian approach: A formal, mathematical system that combines information about the current stimulus with prior knowledge about the world. © 2024 Oxford University Press FIGURE 4.35 Bayesian perception © 2024 Oxford University Press 64 4.5 Object Recognition 64 Moving from V1 to IT in the what pathway, neurons respond to more and more complex stimuli. – By area V4, cells are interested in stimuli such as fans, spirals, and pinwheels. – It is difficult to know exactly what V4 neurons like, but it is something more complicated than spots or bars of light. © 2024 Oxford University Press FIGURE 4.36 Response of V4 cells to different shapes © 2024 Oxford University Press FIGURE 4.37 Occluded shapes © 2024 Oxford University Press 67 4.5 Object Recognition 67 Functional imaging can help us identify brain regions that respond best to certain stimuli. Subtraction method: Comparing brain activity measured in two conditions: one with and one without the mental process of interest. The difference between the images may show the brain regions specifically activated by that mental process. © 2024 Oxford University Press 68 4.5 Object Recognition 68 Functional imaging can help us identify brain regions that respond best to certain stimuli. Decoding method: Take fMRI scans of a participant looking at many images from various known categories. Train a computer model to recognize brain activity from each category. Then test the computer model to see if it can identify an untrained image based on what it has learned. © 2024 Oxford University Press FIGURE 4.39 Object-decoding methods in functional magnetic resonance imaging (fMRI) © 2024 Oxford University Press 70 4.5 Object Recognition 70 The pandemonium model – Oliver Selfridge’s (1959) simple model of letter recognition. – Perceptual committee made up of “demons.” Demons loosely represent neurons. Each level is like a different brain area. © 2024 Oxford University Press FIGURE 4.40 Selfridge’s pandemonium model of letter recognition © 2024 Oxford University Press 72 4.5 Object Recognition 72 Templates versus structural descriptions – Template theory: The proposal that the visual system recognizes objects by matching the neural representation of the image with a stored representation of the same “shape” in the brain. – Structural description: A description of an object in terms of its parts and the relationships between those parts. © 2024 Oxford University Press FIGURE 4.41 Templates © 2024 Oxford University Press FIGURE 4.42 Cow templates © 2024 Oxford University Press 75 4.5 Object Recognition 75 Recognition-by-components – Biederman’s model of object recognition: Holds that objects are recognized by the identities and relationships of their component parts. – Geons: The “geometric ions” out of which objects are built. © 2024 Oxford University Press FIGURE 4.43 Building objects from geons © 2024 Oxford University Press 77 4.5 Object Recognition 77 Deep neural network (DNN) A more modern version of Selfridge’s Pandemonium proposal. Multi-level neural networks that can be trained to recognize objects. Many instances of an object are shown to the network, with feedback. Over time, the network can recognize new instances of the object that it has never been trained on. © 2024 Oxford University Press FIGURE 4.45 A deep neural network recognizes a cow © 2024 Oxford University Press 79 4.5 Object Recognition 79 Multiple recognition committees? – Perhaps there are several object recognition processes, depending on the category level. Entry-level category: For an object, the label that comes to mind most quickly when we identify the object. Subordinate-level category: A more specific term for an object. Superordinate-level category: A more general term for an object. © 2024 Oxford University Press 80 4.5 Object Recognition 80 Faces: An illustrative special case – Face recognition seems to be special and different from object recognition. – Holistic processing: Processing based on an analysis of the entire object or scene and not on adding together a set of smaller parts or features. – Prosopagnosia: An inability to recognize faces (“face blindness”). © 2024 Oxford University Press FIGURE 4.47 Faces © 2024 Oxford University Press FIGURE 4.48 That is obvious © 2024 Oxford University Press