Lecture 4 - Stats Power and t Tests PDF
Document Details
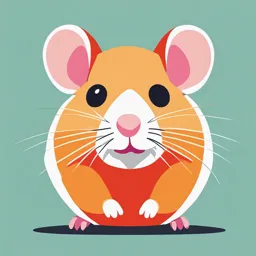
Uploaded by PrizeKineticArt7826
King's College London
2024
Dr Oliver Pain
Tags
Summary
This lecture covers statistical power and t-tests, including the relationship between sample size, effect size, and statistical power. It also discusses the types of t-tests and their assumptions.
Full Transcript
IoPPN October 2024 Dr Oliver Pain Introduction to Statistics SGDP Centre 4. Statistical Power and the t-test Where we left off in the Statistical Inference lecture… We use samples to understand populations Estimates may vary due to sam...
IoPPN October 2024 Dr Oliver Pain Introduction to Statistics SGDP Centre 4. Statistical Power and the t-test Where we left off in the Statistical Inference lecture… We use samples to understand populations Estimates may vary due to sampling variation Larger samples help give more precise estimates The error is quantified using the standard error and confidence intervals We can assess statistical significance of difference between values using z-scores and p-values Recap Null hypothesis (H0): there is no effect/difference. Alternative hypothesis (H1): there is an effect/difference. The p-value is the probability that the result would be observed if the null hypothesis is true One sample z-test example: What is the probability that average IQ in a classroom (N=36) is > 105? SE = 2.5 = 15/sqrt(36) = SD/sqrt(N) z-score = 2 = (105-100)/2.5 = (samp_mean – pop_mean)/SE p-value = 0.023 Test results meeting the reality Look at the bigger picture. Statistical test results may be wrong. False positive (“Type I error”): the null hypothesis H0 is in fact true, but we reject it in favour of our alternative hypothesis H1. The rate is called alpha (α) “Positive” indicates the test result is positive (H0 rejected, H1 supported) “False” means that the test result does not fit the reality A major goal of hypothesis testing is to control these errors Example: In security screening, there are no dangerous items but the system alarms. False negative (“Type II error”): our alternative hypothesis H1 is in fact true, but we don’t reject the null hypothesis H0. The rate is called beta (β) Example: In security screening, there are dangerous items, but the system does not alarm. Minimising β = maximizing (1 – β), which is statistical power. Test results meeting the reality Test result Rejects H0 Doesn’t reject H0 True positive rate + False negative Reality H1 is rate = 1 true 1-β β False positive rate + True negative H0 is rate = 1 true α 1-α Test result Power = true positive rate = sensitivity = Pr (reject H0 | H1 is true) = 1 – β Rejects H0 Doesn’t reject “under the condition” H0 “given” “if” Reality H1 is True true positive False negative H0 is False true positive True negative Statistical power The likelihood we’ll detect an effect if there is in fact an effect Power = Pr (reject H0 | H1 is true) = true positive rate = 1 – β = sensitivity Consequences of low power: High false-negatives (β), that is, we risk missing effects that really are there The conventional target (again, arbitrary) is to have a minimum of 80% power to detect the effect we’re interested in Power is a property of a statistical test, not of a sample or of a study What does power depend on ? Four factors: While holding the other 3 factors Statistical test (z test/t test, constant: one/two-sided) One-sided tests have more Sample size (n) power than two-sided. Effect size (d) Larger samples -> higher Significance threshold (α), type-I power Larger effect sizes -> higher error How do we use power in research? power In the planning phase, we calculate what Higher α -> higher power (lower β) sample size is needed to achieve a predefined power. We can also calculate how much power is Caution: it is possible to reduce α and β achieved based the four factors above. simultaneously by increasing the sample size. Sampling distribution of z Statistic under H0 Two-sided vs. one-sided tests Use one sample z-test as an example Null hypothesis (H0): group mean = a given value. Alternative hypothesis (H1): Two-sided: group mean ≠ a given value One-sided: group mean > a given value (or 0.8 sample size and effect size With a smaller effect size, a larger sample size is needed to reach 80% power With a smaller sample size, only power < 0.8 larger effect sizes reach significance threshold with 80% power Caution: Without fixing power and alpha, i.e., without applying any threshold for statistical significance, effect sizes are independent of the sample size Further reading: Using Effect Size—or Why the P Value Is Not Enough Publication Bias in Psychology Two bad consequences of low power 1. Missing effects that are really there Running underpowered studies wastes resources: they’re not fit for purpose 2. Overestimating effect sizes Low power makes it less likely to for small effect sizes to pass the significance threshold Publication bias makes non-significant results less likely to publish These two reasons result in overall inflated effect sizes in published literature Further reading: Ioannidis, J. P. A. (2008) The t-test We’ve been talking about one-sample z-tests up until now, but hardly anyone uses these in practice The z-test assumes (among other things) that we know the true population standard deviation (SD) Not a realistic assumption Have to make a slight adjustment to the sampling distribution to use an estimate of the population SD (next slide) Deal with small sample sizes (e.g., N