Week 8 - Slides PDF
Document Details
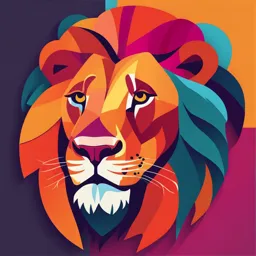
Uploaded by AlluringOnyx4783
Boğaziçi University
2022
Saliha Erman, MA
Tags
Summary
These lecture slides discuss Power & Effect Size in Hypothesis Testing, covering Type I and Type II Errors, significance levels, and power of statistical tests. The contents are adapted from a 2022 lecture at Boğaziçi University.
Full Transcript
Power & Effect Size A Lecture on Type I and Type II Errors and Effect Size in Hypothesis Testing Teaching Assistant: Saliha Erman, MA Source: Adapted from Assoc. Prof. Güneş Ünal, Boğaziçi University, 2022 Hyp...
Power & Effect Size A Lecture on Type I and Type II Errors and Effect Size in Hypothesis Testing Teaching Assistant: Saliha Erman, MA Source: Adapted from Assoc. Prof. Güneş Ünal, Boğaziçi University, 2022 Hypothesis Testing: Correct Decisions and Errors In any hypothesis test there are four possible outcomes, depending on: The True Situation: Either the Null Hypothesis is actually true, or the Alternative Hypothesis is actually true. The Decision: Either not to reject the Null Hypothesis, or to reject the Null Hypothesis and accept the Alternative Hypothesis Basics of this chart:https://www.youtube.com/watch?v=QjF8QP2bf9Q Hypothesis Testing: Correct Decisions and Errors Each cell in this table is a possible outcome of a particular hypothesis test As our decision rule is probabilistic, there are probabilities associated with each of the four outcomes These probabilities are conditional probabilities: They are the probabilities of a decision conditional on the true situation In other words: https://www.youtube.com/watch?v=Hdbbx7DIweQ Hypothesis Testing: Correct Decisions and Errors Two of these probabilities have names in addition to their symbols: The probability of a Type I Error has the symbol α and is called The Significance Level The probability of a Correct Rejection of the Null Hypothesis in favour of the Alternative Hypothesis has the symbol 1-β and is called The Power of the Test (Type II Error is β) Hypothesis Testing: Correct Decisions and Errors Illustration of the power and the significance level of a statistical test, given the null hypothesis (sampling distribution 1) and the alternative hypothesis (sampling distribution 2). Type II Error and the Power of the Test Let us assume that a standardized ability test has scores which are normally distributed with μ = 500 and σ = 100 An investigator is interested in the effects of Computer Assisted Instruction (CAI) on performance testing 25 students. He predicts that CAI will lead to an improvement in performance as measured by the standardized ability test; i.e., HA : μ = 550 From a consideration of the sampling distribution under the Null Hypothesis (with a 5% significance level): Because the hypothesis is directional, the critical value is 1.645. For example, if our observed mean for the 25 participants was 520.4: z = (520.4 – 500) / 20 = 1.02 0 +1.645 z score We would not reject the Null Hypothesis, as this value falls outside the rejection region We would conclude that we were unable to reject the Null Hypothesis - not that we accept the Null Hypothesis The mean score need to fall above 532.9 to be able to reject the null hypothesis. So far, we have been considering the sampling distribution under the null hypothesis. What is the probability of obtaining a Y equal to or greater than 532.9 if the Alternative Hypothesis is true? What is the probability of obtaining a Y equal to or greater than 532.9 if the Alternative Hypothesis is true? The sampling distribution under the Alternative Hypothesis is centred at μ = 550 but otherwise it is identical to the distribution under the null (normal, standard error = 20) z = (532.9 – 550) / 20 = -0.855 p(z ≥ -0.855) = 0.50 + 0.3023 =.8023 (80.23%) The Power of the Test: Effect Size Now let us assume that the sampling distribution under the Alternative hypothesis is centered at μ = 580. What is the probability of obtaining a Y equal to or greater than 532.9 if the Alternative Hypothesis is true? z = (532.9 – 580) / 20 = - 2.355 P(z ≥ -2.355) = 0.991 The Power of the Test: Effect Size The Power of the Test: Effect Size As the effect size increases (in this example from μ = 550 to μ = 580) the power of the test increases (i.e., the probability of a correct rejection of H0) and the probability of a Type II Error decreases In other words: As the effect size increases, 1-β increases As the effect size increases, β decreases Another way to reduce the overlap between the distributions is to reduce the size of the standard error (σY) As the sample size increases, the estimates (Y values) of the parameter (μ) become more and more accurate The power of the test will increase as the standard error decreases (all else being equal) In a real study, one usually has rather little control over the effect size, but clearly one does have control over the sample size The Power of the Test: Effect Size Self exercise: With reference to the CAI example, imagine that the investigator had decided to test 100 students (n = 100 rather than 25) after they had received CAI. How does power change? Overall, as shown with the graph on the right: As sample size increases, the standard error of the mean decreases, and the distribution becomes narrower. As the distribution becomes narrower, even though the means are the same, the power to detect the effect increases. The Power of the Test: Effect Size ! The difference between a statistically significant result and a non-significant result may be just the size of the sample. Even a small difference has a high probability (power) of producing a statistically significant result if the sample size is large enough Hypothesis Testing Recap Teaching Assistant: Saliha Erman, MA Source: Adapted from Assoc. Prof. Güneş Ünal, Boğaziçi University, 2022 Hypothesis Testing - Begin with a Null and Alternative Hypothesis - Set the alpha level. - Perform the appropriate statistical test. - Calculate the p-value from the test statistic. - Compare the p-value to the alpha level and decide if results due to chance (not statistically significant) or unlikely due to chance (statistically significant). Alternatively, find the critical and the observed test statistic and make a comparisiona for making a decision (to reject or not to reject). Sampling Distributions A population of scores >> reflects the frequency of occurence of every score in the distribution. SO Frequency distributions are also called probability distributions. Remember: Given mean and standard deviation of a normal distribution >> you can make probability statements about the likelihood of selecting a score from a specified area of the distribution. Sampling Distributions In hypothesis testing, you are usually interested in the MEANS rather than individual scores. SO If you want to make probability statement about a randomly selected mean falling within a specified area under the normal curve you need a NORMAL DISTRIBUTION OF MEANS. When were we interested in the individual scores: i.e. What is the probability of selecting a score of less than 20, given that the mean of the population is 26 and the standard deviation 4. Give an example for a one-sample z-test (when are interested in means) i.e. The mean IQ of the 23 third graders in Mrs. Smith’s class is 108.6. Are the children in Mrs. Smith’s class representative of the general population with respect to IQ? (Given that population mean = 100 & population SD = 15) (inferential test) Their commonality is that we use the z-table for both! REMEMBER: 3 different types of questions that are solved using the same probability distribution based on the z-table: Probability question based Probability question based Inference question based on sample (one on a single datapoint on sample mean: What is sample z-test): The mean IQ of the 42 (without a sample): What is the probability of observing students from a class is 94. Is this class the probability of randomly a mean IQ of 94 or less representative of the general population? (µ selecting an individual with based on 42 randomly = 100; σ = 15) an IQ of 94 or less from the selected individuals from the general population? (µ = 100; general population? (µ = -2.59 σ = 15) 100; σ = 15) Null Hypothesis: H0: The class is representative of the population (there is no difference) Alternative Hypothesis: H1 or Ha: The class is not representative of the general population (there is a difference) -0.40 -2.59 The area under the standard normal curve from negative infinity to -2.59 is The area under the.0048; and from positive infinity to +2.59 is standard normal curve from The area under the negative infinity to -0.4 is standard normal curve from.0048 (Since this is a two sided test, we take negative infinity to -2.59 is the p value as their total, which is.0096). The 0.345. Hence, the probability critical z-values for a two sided test is -1.96 of observing a score of 94.004. Hence, the probability and +1.96. Since.0096 is below the alpha or less based on random of observing a mean of 94 or less based on a random level of.05 (and the z score of -2.59 is selection is.345 (34.5%) sample of 42 is.004. (0.4%) lower than -1.96), we reject the null hypothesis. The class is not representative of the population. Sampling Distributions The Mean of the Sampling Distribution: Adding up all the sample means and divide by the number of means. The mean of the sampling distribution is exactly the same as the mean of the population SO µ= Sampling Distributions The Standard Deviation of the Sampling Distribution (σ y ) STANDARD ERROR OF THE MEAN Notice: It is SMALLER than the standard deviation of the original population. SO The variability of the sampling distribution is determined by: - The variability of the population distribution - The size of the samples used to establish the sampling distribution Sampling Distributions How N Affects the Standard Error of the Mean Standard error becomes SMALLER as N becomes LARGER As the sample size increases, the sampling distribution will approach a normal distribution (central limit theorem) How large should N be before the sampling distribution is normal? - Standard answer is when N = 30 BUT If the population is NORMAL >> ANY size N lead to a normally distributed sampling distribution If the population is VERY UNNORMAL >> N > 30 may be needed. One Sample t-Test Teaching Assistant: Saliha Erman, MA Source: Adapted from Assoc. Prof. Güneş Ünal, Boğaziçi University, 2022 The Standard Normal Distribution We already know that: and accordingly: Standard error What if σY and therefore σY is not known? >> Then, it must be estimated from sample data. Student’s t Distribution While working with small samples, there would be considerable error in the estimates of the parameters based on the sample information. The sample standard deviation (ŝ) is used as an estimate of the population standard deviation (σ) and the t-distribution is generated. This formula calculates the estimated number of standard errors that the sample mean is from µ Who is ‘the student’?: you can watch this. W. S. Gosset >> an Oxford graduate, an employee of the Guinness Brewery in Dublin >> spent the academic year 1906-1907 @UCL, London working with the well-famous Karl Pearson to come up with the t distribution (Pearson founded the world's first university statistics department at UCL at 1911) >> Gosset published under the pen name ‘Student’ (Guinness would not let him publish under his real name). REMEMBER WHAT ŝ is: Estimating the Population Variance The sample variance (s2, SS divided by N) is a biased estimate of the population variance (σ2, sigma-squared). The unbiased estimate of the population variance is the estimated population variance (ŝ2 , SS divided by N-1). Σ (Y - Y) 2 Σ (Y - µ) 2 Σ (Y - Y) 2 s2 = σ2 = ŝ2 = n N n-1 (biased) (unbiased) Bessel’s correction N-1 is known as the degrees of freedom. To learn more about it, you can watch this. Student’s t Distribution p that a sample mean will be a certain number of actual standard errors away from µY is not as same as the p that a sample mean will be a certain number of estimated standard errors away from µY (the mean of the sampling distribution). >> Sampling distribution in this case is NOT a normal distribution but approximates to a theoretical distribution called t distribution. Unlike a normal distribution, the shape of t distribution is influenced by the number of degrees of freedom (which is itself influenced by sample size) → (n-1) When the estimate is based on very few samples (so that the estimate of SD is particularly uncertain) we have a distribution which is far more spread out than the normal. When the number of samples is very large, the estimate s varies hardly at all from , and the corresponding t distribution is very close to normal. Student’s t Distribution The density of the t distribution for different degrees of freedom, together with that of the normal. Note that the t distribution is symmetric around 0, just like the normal distribution. Student’s t Distribution Student’s t Distribution In general the symbol ν is used to represent the degrees of freedom. A particular t value always has a df associated with it:t(df) For a one-sample case, df = N - 1. Notice that we're talking about a new distribution here (or family of distributions, the t-distributions). This also means that we won't be using the z table. Instead we'll have to use a different table, the t distribution table. Student’s t Distribution In general, the normal and t distributions are similar when N > 40. In fact, when the sample size is theoretically infinite, the t distribution and the z distribution are identical. But until N = 40; it is easier to say that there is an effect according to the values in the z-table. E.G., a sample of size of n = 12 (df = 11). For a 95 percent confidence interval (two-sided test) the critical t-value (or t-score) is 2.201. Recall that the Z score for the same level of confidence is 1.96. SO If you compute t from the data but look it up on a table computed from z, the probability of rejecting a true H0 (Type I error) would be larger >> This is something you’d like to avoid! In one sample t-test, make sure you are using the t-table, not the z-table. z Distribution vs. t Distribution The population standard deviation (sigma) is not known and must be The population standard deviation estimated (sigma) is known Watch this for a basic difference. Watch this to see examples from both. Example General recommendation: Adults exercise at least twice a week. A sample of 35-to-40-year-olds >> N = 30 individuals mean: 1.84 standard deviation estimate: 1.68 Test the viability of the hypothesis that µ = 2 using a one-sample t test. 1) Define your null and alternative hypotheses: H0: µ = 2 (the mean number of workouts undertaken by the population of 35-to-40-year-olds is two per week) H1: µ ≠ 2 (the mean number of workouts undertaken by the population of 35-to-40-year-olds is NOT two per week) 2) Set your alpha level: α =.05 3) Define your test: Whether a one-sided or two-sided test you will use: A non-directional test 4) Degrees of freedom: N - 1 = 30 – 1 = 29 Example Find the critical values of t from the table of critical values of t distribution: For an alpha level of.05, non-directional test, and N – 1 = 29 degrees of freedom, the critical values of t (from Appendix D in book) are ± 2.045. One sample t test: Reject or fail to reject the null hypothesis: Because -.52 does not exceed the negative critical value of – 2.045, we fail to reject the null hypothesis that the mean number of workouts undertaken by the population of 35-to-40-year-olds is two per week. Example Lets solve it with the confidence intervals approach. First find the critical t’s: For an alpha level of.05, non-directional test, and N – 1 = 29 degrees of freedom, the critical values of t (from Appendix D in book) are ± 2.045. ŝY = (ŝ / √N) = 1.68 / √30 = 0.31 df = N – 1 = 30 – 1 = 29 95% confidence Y ± (t x (ŝ / √N)) interval = 1.84 – (2.045)(0.31) to 1.84 + (2.045)(0.31) = 1.21 to 2.47 → The population mean (2.00) is within this interval, therefore we fail to reject the null hypothesis (i.e., there is not effect, the sample comes from the population, there is no difference). → at alpha = 0.05. Another example for Confidence Intervals Example: An investigator administered a reading test to a sample of 30 students and found a mean of 83.00 with a standard deviation estimate of 17.35. Calculate the 95% and 99% confidence intervals. (Remember: CI’s are calculated around the sample mean!) ŝY = (ŝ / √N) = 17.35 / √30 = 3.17 df = N – 1 = 30 – 1 = 29 95% confidence Y ± (t x (ŝ / √N)) → A population mean that interval = 83.00 – (2.045)(3.17) to 83.00 + (2.045)(3.17) is outside of this interval means rejecting the null = 76.52 to 89.48 hypothesis. (alpha =.05) 99% confidence Y ± (t x (ŝ / √N)) → A population mean that interval = 83.00 – (2.756)(3.17) to 83.00 + (2.756)(3.17) is outside of this interval = 74.26 to 91.94 means rejecting the null hypothesis. (alpha =.01) Sample Size and t Distribution Remember: a sample of size of n = 12 (df = 11). For a 95 percent confidence interval (two-sided test) the critical t-value (or t-score) is 2.201. Moreover, If n increases, say to 26 (df = 25), then the critical t-score becomes 2.060. >> Let’s check how does this influence confidence interval: ̂ Y ± (t x s Confidence interval around the sample mean gets smaller as N √N Smaller t Larger N gets bigger t Table cum. prob t.50 t.75 t.80 t.85 t.90 t.95 t.975 t.99 t.995 t.999 t.9995 one-tail 0.50 0.25 0.20 0.15 0.10 0.05 0.025 0.01 0.005 0.001 0.0005 two-tails 1.00 0.50 0.40 0.30 0.20 0.10 0.05 0.02 0.01 0.002 0.001 df 1 0.000 1.000 1.376 1.963 3.078 6.314 12.71 31.82 63.66 318.31 636.62 2 0.000 0.816 1.061 1.386 1.886 2.920 4.303 6.965 9.925 22.327 31.599 3 0.000 0.765 0.978 1.250 1.638 2.353 3.182 4.541 5.841 10.215 12.924 4 0.000 0.741 0.941 1.190 1.533 2.132 2.776 3.747 4.604 7.173 8.610 5 0.000 0.727 0.920 1.156 1.476 2.015 2.571 3.365 4.032 5.893 6.869 6 0.000 0.718 0.906 1.134 1.440 1.943 2.447 3.143 3.707 5.208 5.959 7 0.000 0.711 0.896 1.119 1.415 1.895 2.365 2.998 3.499 4.785 5.408 8 0.000 0.706 0.889 1.108 1.397 1.860 2.306 2.896 3.355 4.501 5.041 9 0.000 0.703 0.883 1.100 1.383 1.833 2.262 2.821 3.250 4.297 4.781 10 0.000 0.700 0.879 1.093 1.372 1.812 2.228 2.764 3.169 4.144 4.587 11 0.000 0.697 0.876 1.088 1.363 1.796 2.201 2.718 3.106 4.025 4.437 12 0.000 0.695 0.873 1.083 1.356 1.782 2.179 2.681 3.055 3.930 4.318 13 0.000 0.694 0.870 1.079 1.350 1.771 2.160 2.650 3.012 3.852 4.221 14 0.000 0.692 0.868 1.076 1.345 1.761 2.145 2.624 2.977 3.787 4.140 15 0.000 0.691 0.866 1.074 1.341 1.753 2.131 2.602 2.947 3.733 4.073 16 0.000 0.690 0.865 1.071 1.337 1.746 2.120 2.583 2.921 3.686 4.015 17 0.000 0.689 0.863 1.069 1.333 1.740 2.110 2.567 2.898 3.646 3.965 18 0.000 0.688 0.862 1.067 1.330 1.734 2.101 2.552 2.878 3.610 3.922 19 0.000 0.688 0.861 1.066 1.328 1.729 2.093 2.539 2.861 3.579 3.883 20 0.000 0.687 0.860 1.064 1.325 1.725 2.086 2.528 2.845 3.552 3.850 21 0.000 0.686 0.859 1.063 1.323 1.721 2.080 2.518 2.831 3.527 3.819 22 0.000 0.686 0.858 1.061 1.321 1.717 2.074 2.508 2.819 3.505 3.792 23 0.000 0.685 0.858 1.060 1.319 1.714 2.069 2.500 2.807 3.485 3.768 24 0.000 0.685 0.857 1.059 1.318 1.711 2.064 2.492 2.797 3.467 3.745 25 0.000 0.684 0.856 1.058 1.316 1.708 2.060 2.485 2.787 3.450 3.725 26 0.000 0.684 0.856 1.058 1.315 1.706 2.056 2.479 2.779 3.435 3.707 27 0.000 0.684 0.855 1.057 1.314 1.703 2.052 2.473 2.771 3.421 3.690 28 0.000 0.683 0.855 1.056 1.313 1.701 2.048 2.467 2.763 3.408 3.674 29 0.000 0.683 0.854 1.055 1.311 1.699 2.045 2.462 2.756 3.396 3.659 30 0.000 0.683 0.854 1.055 1.310 1.697 2.042 2.457 2.750 3.385 3.646 40 0.000 0.681 0.851 1.050 1.303 1.684 2.021 2.423 2.704 3.307 3.551 60 0.000 0.679 0.848 1.045 1.296 1.671 2.000 2.390 2.660 3.232 3.460 80 0.000 0.678 0.846 1.043 1.292 1.664 1.990 2.374 2.639 3.195 3.416 100 0.000 0.677 0.845 1.042 1.290 1.660 1.984 2.364 2.626 3.174 3.390 1000 0.000 0.675 0.842 1.037 1.282 1.646 1.962 2.330 2.581 3.098 3.300 z 0.000 0.674 0.842 1.036 1.282 1.645 1.960 2.326 2.576 3.090 3.291 0% 50% 60% 70% 80% 90% 95% 98% 99% 99.8% 99.9% Confidence Level Sample Size and t-Distribution and Confidence Intervals Similarly, confidence interval around the sample mean gets bigger as N gets smaller. >> In this case, the larger interval for a desired level of confidence is required to account for the additional uncertainty introduced by the small sample. (remember the power & N relationship). Confidence intervals are not only used for making statistical decisions in hypothesis testing but also for understanding an estimate in an "interval" format. For example, being 95% confident that the mean value lies somewhere between 7.5 and 9.5. Sample Size and t-Distribution and Confidence Intervals Example: A new model vehicle tested for safety. Because it requires a crash test (and the budget to crash new vehicles is small), a small sample would be used for a safety rating. Test is performed on a random sample of five of the new model cars from the manufacturer. The mean safety rating of the 5 cars is 8, with an estimated standard deviation of.94 (0=unsafet 10=safe). 99% confidence interval is decided to be used: The t-score for 99 percent and df = 4 is found in the t-distribution table to be 4.604. Sample Size and t-Distribution and Confidence Intervals Example (continue): ̂ s Y ± (t x ) = 8 ± (4.604 x (.94 / √5)) √N = 8 ± 1.94 CI from 6.06 to 9.94 This result means that the consumer safety group can say that it is 99 percent confident the safety rating of the new model is between 6.06 and 9.94. To get a smaller confidence interval at the 99 percent confidence level, more cars will have to be crash tested. For instance, if they test 20 cars (df = 19) and still find the mean of 8: ̂ Y ± (t x s ) = 8 ± (2.861 x (.94 / √20)) √N = 8 ± 0.60 CI from 7.40 to 8.60 z Distribution vs. t Distribution The population standard deviation (sigma) is not known and must be The population standard deviation estimated (sigma) is known Watch this for a basic difference. Watch this to see examples from both. EXERCISE QUESTION 1 The scores on a standardized test are normally distributed with a mean of 100 and a standard deviation of 15. What is the probability of selecting a test score higher than 120? QUESTION 1 The scores on a standardized test are normally distributed with a mean of 100 and a standard deviation of 15. What is the probability of selecting a test score higher than 120? QUESTION 2 A factory claims that the average weight of a bag of chips is 500 grams. A random sample of 40 bags has an average weight of 495 grams, with a known population standard deviation of 10 grams. At the 0.05 significance level, is there evidence to reject the factory's claim? QUESTION 2 A factory claims that the average weight of a bag of chips is 500 grams. A random sample of 40 bags has an average weight of 495 grams, with a known population standard deviation of 10 grams. At the 0.05 significance level, is there evidence to reject the factory's claim? QUESTION 2 A factory claims that the average weight of a bag of chips is 500 grams. A random sample of 40 bags has an average weight of 495 grams, with a known population standard deviation of 10 grams. At the 0.05 significance level, is there evidence to reject the factory's claim? QUESTION 2 QUESTION 2 QUESTION 3 A researcher wants to test if the average height of a particular plant species differs from 15 cm. A sample of 10 plants has an average height of 14.5 cm with an unbiased sample standard deviation of 1.2 cm. At the 0.01 significance level, does the data provide evidence to reject the null hypothesis? QUESTION 3 A researcher wants to test if the average height of a particular plant species differs from 15 cm. A sample of 10 plants has an average height of 14.5 cm with an unbiased sample standard deviation of 1.2 cm. At the 0.01 significance level, does the data provide evidence to reject the null hypothesis? QUESTION 3 A researcher wants to test if the average height of a particular plant species differs from 15 cm. A sample of 10 plants has an average height of 14.5 cm with an unbiased sample standard deviation of 1.2 cm. At the 0.01 significance level, does the data provide evidence to reject the null hypothesis? ^ → Unbiased sample SD = estimated population SD QUESTION 3 Two-Samples Test: Between-Subjects t-Test (Independent Samples t-Test) Teaching Assistant: Saliha Erman, MA Source: Adapted from Assoc. Prof. Güneş Ünal, Boğaziçi University, 2022 Recap Between-Subjects or Independent Groups t-Test The independent groups t test can be applied to either True Experiments or Quasi Experiments This test is typically used to analyze the relationship between two variables (stated more clearly, to analyze the effect of x on y) under the following conditions: 1.The dependent variable is quantitative in nature and is measured on a level that at least approximates interval characteristics. 2.The independent variable is between-subjects in nature (it can be either qualitative or quantitative), but divides participants into two groups. 3.The independent variable has two, and only two, levels. Between-Subjects or Independent Groups t-Test Example 1: A study was conducted to investigate whether noise exposure affects task performance. 15 participants in the experimental group solved a standard sudoku task while being exposed to 40 decibels of noise, whereas 20 participants in the control group solved the same sudoku task in a quiet environment without any noise exposure. The time (in seconds) it took for participants to complete the task was recorded for each individual. DV (Dependent Variable): Task completion time (quantitative, ratio) IV (Independent Variable): Noise exposure (qualitative, nominal) Level 1: Noise exposure (40 decibels) Level 2: No noise exposure Between-Subjects or Independent Groups t-Test The Null Hypothesis: The Alternative Hypothesis: H1 :μ1 ≠ μ2 (Two-sided test) H0 :μ1 = μ2 H1 :μ1 > μ2 (One-sided test) No difference between the two means. There is a difference between the two means. If the direction of the difference is specifies (either bigger or smaller) it is a one sided alternative hypothesis. Population Distributions Population Distributions Under Null Hypothesis Under Alternative Hypothesis Both have the same mean, same Under the alternative hypothesis variance and same normality. though, they have different means! Thus, under H0 both samples have been Therefore they come from two different drawn from the same population of population distributions with two scores. different means. Example: Example: The sampling distribution for the difference between two means (Between Subjects t-Test) In Independent samples t-test, there two conditions, two samples, and Standard error two populations that there samples of the difference come from. Therefore, the sampling distribution is based on the the differences between the means of samples that Where two population were drawn from the two means are equal populations. The sampling distribution for the mean (One Sample t-Test) In one sample t-test, there is only one Standard error condition, one sample and one of the mean population that the sample comes from. Therefore, the sampling distribution is based on the means of samples that were drawn from that one population. More clearly, the sampling distribution for the difference between two means (in independent samples t-test) comes from this method: You randomly select numerous samples from each population (just like how we do it one in one sample t-test), you calculate their means, and you calculate the differences between these means for each pair. When we average all of the sample mean differences, the result will equal the difference between the population means. And the distribution of this list will result in this sampling distribution of difference between the means Standard Error of the Difference and the t-Test Formula in Independent Samples t-Test Standard Error of the Difference The variance sum law states that the variance of a sum or difference between two independent random variables is equal to the sum of their respective variances. When population parameter (population std. dev.) is known: Standard Error of the Mean Standard Error of the Difference (one sample t-test) (two sample between subjects t-test σ Y = Standard error of the difference n1: Sample size for group 1 n2: Sample size for group 2 Population variance How we came to that formula? for group 1 Population variance Note that the standard error of the mean for group 2 difference formula is actually like this, based on the variance sum law: But due to homogeneity of variances assumption, we assume that under the null hypothesis, the variances of the two populations are the same. Then, the new formula for the std. error of the difference is this: If take the square of this formula, we get: If the take the square root of the parenthetical expression, we get: Formula for Independent Samples z-Test THEN; When we know the population std. deviation, based on the sampling distribution for differences between the means: of mean differences Under the null hypothesis, the difference between two population means is zero: H0 :μ1 - μ2 = 0 The standard error of the difference (std. dev. of the sampling distribution of mean differences) We can conduct between subjects Z-test with this formula. Formula for Intependent Samples t-Test However, you would rarely know σ in a two-sample case. So you need to estimate it, and have estimated standard error of the difference in the denominator. (and use the t-test, instead of the z-test) Estimated standard error of the difference (estimated std. dev. of the sampling distribution of mean differences) Independent Samples t-Test: Estimated Standard Error of the Difference When you don’t know the σ in a two-sample case, you need to estimate it using the two samples’ standard deviations. Information from both samples are “averaged” to provide a pooled estimate (ŝ 2pooled) of the parameter value (σ2) >> Weighted mean of variance estimates (weighted by the respected df’s): (df1)(ŝ 12) + (df2)(ŝ 22) ŝ 2pooled = dfTOTAL To find the estimated std. error of the difference, make a substitution in the formula (replace σ, with s^pooled) → replace a known parameter with its estimate. 1st Way of Applying the T-test Formula: if s^pooled (or sample s^’s) are known: Remember the two sample z-test formula? (Slide 12) ^ µ1 - µ2 under H0: µ1 = µ2 If your H0 is different than that, put the value accordingly. ^ Estimated standard error of the difference = ŝ pooled 2nd Way of Applying the Formula: if sum of squares of the two samples are known Therefore, the final formula in the second method is: Applying Independent Samples t-Test Assumptions of the Independent Groups t-test The samples are independently and randomly selected from their respective populations. The scores in each population are normally distributed. Dependent variable at least interval level of measure. The scores in the two populations have equal variances: the assumption of homogeneity of variance. Assumptions of the Independent Groups t-test Under certain conditions, independent samples t-test is robust to violations of the normality and homogeneity of variance: >> If the sample sizes in each group > 40 >> test is robust to departures from normality assumption. >> If the sample sizes are equal >> test is robust to violations of the homogeneity of variance assumption. >> in Jamovi: Equal variance assumed / Equal variance not assumed Levene Test evaluates a null hypothesis of equal variances in the populations against an alternative hypothesis of unequal variances in the populations. >> If the test is significant: Variances are not homogenous. Using Independent Samples t-Test dftotal= df1 + df2 – 2 dfe = 5 -1 = 4 dfn = 15 -1 = 14 dftotal = 4 + 14 = 18 -6 is the difference between the sample means. (WHY -6 but not +6) Because we constructed our alternative hypothesis as such. dftotal= df1 + df2 – 2 dfe = 5 -1 = 4 dfn = 15 -1 = 14 dftotal = 4 + 14 = 18 Confidence Intervals in Independent Samples t-Test The confidence interval fromula for a two sided test: Estimated Standard deviation of the sampling It is calculated distribution of the difference around the between two means (a.k.a CI for population sample mean estimated standard error of mean difference differences the difference) Note that when it is two sided, you would have two ends: a lower point and an upper point of the confidence interval. When it is one sided, you would either calculate a lower or an upper end, according to the direction of the hypothesis. The opposide end would be infinity. Now lets assume that the previous question was a two-sided test, and calculate the two-sided confidence intervals for it. Estimated standard deviation of the sampling distribution of the mean difference (a.k.a estimated standard error of the difference) is 4.42, as we found in the previous slide. In independent samples t-test, we construct our confidence interval around the sample mean difference, to refer to (compare with) the difference at the population level under null hypothesis. (In this case, the sample mean difference is -6.) Next, we should check whether the interval includes population mean difference under the null hypothesis (which is 0). If it includes 0 → there is no difference → we fail to reject the null hypothesis. If it doesn’t include 0 → there is either a positive or negative difference → we reject the null hypothesis. Remember CI formula in one sample t-test: In one sampe t-test: Instead of sample mean differences, you have single sample mean; Instead of std. error of the mean difference, you have standard error of the mean. Exercise 1 changes Exercise 2 changes You can calculate the CIs on your own as an exercise