CHS 729 Module 2 Review: Tests and Interpretations
Document Details
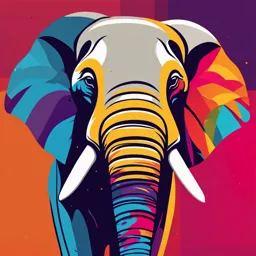
Uploaded by LargeCapacityAntigorite4770
Tags
Summary
The document reviews key concepts in statistical testing, including Null Hypothesis Significance Testing (NHST), effect sizes, and statistical power. It contrasts Fisher's and Neyman-Pearson approaches. This document emphasizes understanding the limitations and best practices when interpreting statistical results, focusing on the nuances of p-values and the importance of considering effect sizes alongside statistical significance.
Full Transcript
Module 2: Assess -- Tests and Interpretations ============================================= Foundations to Assess --------------------- - We moved from **general inference theory and related distributions** to **testing approaches and interpretation**. - Key focus areas: 1. Understa...
Module 2: Assess -- Tests and Interpretations ============================================= Foundations to Assess --------------------- - We moved from **general inference theory and related distributions** to **testing approaches and interpretation**. - Key focus areas: 1. Understanding **Null Hypothesis Significance Testing (NHST)**. 2. Evaluating **effect sizes** and **power** in statistical tests. 3. Recognizing **errors and limitations** in hypothesis testing. Fisher's Approach: P-Values as Evidence --------------------------------------- - **Ronald Fisher (1890-1962)** introduced **p-values** as a measure of how well observed data align with the **null hypothesis (H~0~)**. - **Key idea**: A small p-value suggests evidence against **(H~0~)**, but does not prove it false. - **Common misconceptions** - A **p-value is NOT** the probability that **(H~0~)** is true. - A **p-value does NOT** indicate effect size or practical importance. - Fisher treated p-values as **continuous measures of evidence**, not strict decision rules. Neyman-Pearson Approach: Decision-Making & Error Control -------------------------------------------------------- - Jerzy Neyman & Egon Pearson developed a decision-making framework emphasizing: - **Type I error (α)**: False positive (rejecting a true **(H~0~)**). - **Type II error (β)**: False negative (failing to reject a false **(H~0~)**). - **Power (1 - β)**: The probability of detecting a true effect. - **Key Differences from Fisher**: - Neyman-Pearson requires a **firm decision**: **reject or accept** **(H~0~)**. - Emphasizes **long-term error control** through repeated testing. NHST in Practice: Strengths & Pitfalls -------------------------------------- - **Strengths** - Provides a **standardized** way to test hypotheses. - Helps quantify **uncertainty** in research. - **Pitfalls** - **Over-reliance on p-values** (without considering effect sizes). - **Misinterpretation** of \"non-significance\" as **evidence of no effect**. - **Publication bias**---favoring \"significant\" results over null findings. - **Best Practices**: - Use **confidence intervals** alongside p-values. - Report **effect sizes** to show practical importance. - Consider **Bayesian approaches** for better uncertainty estimation. Effect Size & Power: What NHST Misses ------------------------------------- - **Effect Size**: The **magnitude** of an association (e.g., Cohen's d, correlation coefficients). - **Power Analysis**: Ensures a study has a large enough **sample size** to detect meaningful effects. - **Key rule**: Power should be **≥80%** to minimize Type II errors. - **Why this matters**: A **non-significant p-value** might mean a **true effect exists but is too small to detect** with the given sample size. One-Sided vs. Two-Sided Tests ----------------------------- - **Two-sided test**: Tests for an effect in **either direction** (e.g., does a drug increase OR decrease blood pressure?). - **One-sided test**: Tests for an effect in a **specific direction** (e.g., does a drug only increase blood pressure?). - **Most NHST tests are two-sided unless a strong rationale exists for a directional hypothesis**. Choosing the Right Approach --------------------------- Fisher's Approach Neyman-Pearson Approach -------------------------------- ------------------------------------------------ Flexible, exploratory research Decision-making, industrial testing p-values as evidence Accept/reject framework with **error control** Best for one-time studies Best for **repeated experiments** Takeaways --------- - **Statistical significance ≠ Scientific significance**---always consider effect sizes. - **NHST is a tool, not a final answer**---interpret results in **context**. - **Errors and power matter**---low power can hide real effects. - **Misuse of NHST leads to bad science**---avoid mechanical \"p \< 0.05\" thinking.