Impact of Social Media on Attention Span (PDF)
Document Details
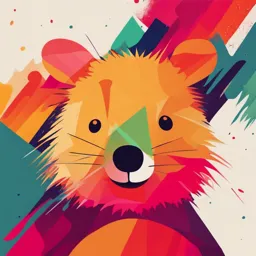
Uploaded by BullishEpiphany5523
Indira Gandhi Delhi Technical University for Women
Akhila Lohidakshan, Diya Gahlot, Harshita Bidhuri
Tags
Summary
This research paper analyzes the impact of using social media platforms on attention spans. The study investigates diverse behavioral parameters from social media activity, including time spent on platforms and user engagement. It aims to develop technologies that can effectively manage attention levels among social media users.
Full Transcript
Impact of Social Media on the Attention Span of Users Akhila Lohidakshan Diya Gahlot Harshita Bidhuri Computer Science Computer Science...
Impact of Social Media on the Attention Span of Users Akhila Lohidakshan Diya Gahlot Harshita Bidhuri Computer Science Computer Science Computer Science Engineering Department Engineering Department Engineering Department Indira Gandhi Delhi Technical Indira Gandhi Delhi Technical Indira Gandhi Delhi Technical University for Women University for Women University for Women New Delhi, India New Delhi, India New Delhi, India [email protected] [email protected] [email protected] Abstract— This research work examines the impact of using by social media has taken control of our attention spans, social media platforms on attention span. The study endeavors to impairing our brains' capacity to concentrate on anything we anticipate users' attention durations by assessing multiple perceive to be less interesting. Ability to focus on a behavioral parameters obtained from social media networks. The single task or piece of information over a comprehensive dataset used for this research incorporated multiple parameters such as time spent on platforms, content engagement metrics, interval of time is termed as attention span. This ability and demographic indicators to forecast users’ attention span. plays a key role in learning, productivity and acing a skill. The data in the dataset was already normalized, and various In a world with so much access to fast pleasure and machine learning models like Linear Regression, Random addictive distractions, short attention spans and lack of Forest, Gradient Boosting, KNN Regressor, and Decision Tree concentration are inevitably the new norms. This shift Regressor were used to predict the desired outcomes. Notably, the could have remarkable consequences, especially in high- Random Forest model demonstrated the highest degree of productivity environment and focus-demanding industries accuracy in predicting the average completion metrics, like academics and professional workspace where rapt functioning to be a surrogate for attention span. Through attention is a necessity for success. hypothesis testing, the study uncovered several important findings, including females tend to have higher attention spans than males; avgDuration and content_views are significant This research leverages a synthetic dataset, meticulously predictors of attention span; and attention spans are higher crafted providing a comprehensive overview of user among users over 30 years old. The research culminates by interactions on social media platform, incorporating 25 emphasizing the significance of these findings for building independent features which comprehensively includes user potential technologies that can trace and possibly improve demographics and content consumption patterns. The attention levels among social media users. objective of this paper is to uncover probable correlations between and attention span. Additionally, our study could Keywords—attention span, social media, machine learning shed light on the effects of attention span across various (ML), random forest, MSE, MAE, R^2. demographics, including gender, urbanization levels (tier 1, tier 2, and tier 3 cities), and global locations, offering a more I. INTRODUCTION comprehensive insight into the factors influencing attention The rapid acceptance of social media platforms over the past span. decade has prompted alterations in the way individuals interact, gather knowledge and spend their past time. The purpose of this research is to contribute to the ongoing Internet communities like Instagram, Facebook, Twitter discourse on social media impacts over cognitive abilities, ,Tiktok , Snapchat etc. have inject ill culture among users by revealing insight-driven patterns that can shape both relying heavily on visual and short-time content, hooking theoretical frameworks and real – world initiatives. These users attentions through short, engaging interactions. The findings would serve a navigational aid to reform policies steady intake of content, fabricated to capture and detain and frame strategies on healthier social media use making attention for short periods, has become a growing concern to sure that these platforms contribute to beneficial cognitive the younger population about the impact caused by social well-being. media over cognitive functions, particularly attention span. Social media’s demand for multitasking overloads cognitive resources, leading to a decreased ability to maintain focus II. LITERATURE REVIEW on single tasks. This literature review will explore how social media affects attention span, particularly among different age groups, the Dopamine in the prefrontal cortex is critical for cognitive addictive nature of these platforms, behavioral changes, and functions such as attention and decision-making. how platforms like Instagram, Facebook, Snapchat etc. Dopamine is a hormone that serves a variety of purposes in influences user’s real life and personality. Kies, S. C. the brain, prominent among them incentive and reward. (2018). “Social media impact on attention span.” Three Every like, follow, or even straightforward notice causes a hypotheses were to be tested as part of the investigation. brief surge of euphoria, which reinforces the brain's need for The first theory was that attention spans are unrelated to the this pleasure. Immediate and instant gratification provided quantity of social media profiles. According to the second XXX-X-XXXX-XXXX-X/XX/$XX.00 ©20XX IEEE theory, there is no difference in attention span between Social media audiences can greatly influence an account’s frequent users of episodic and non-episodic social media content and even pressure users to maintain a certain image, accounts. According to the third hypothesis, individuals who which is typically an elevated version of themselves. In favor mobile over PC forms of social media have similar some cases, content is deliberately exaggerated to gain a attention spans. desired reaction – or a reaction in general! Impact on Attention Span among different Age Groups: Real life v/s social media persona: Young Generation-The impact of social media on young In recent years, social media has impacted the way in which users has become a major concern as their attention span is users communicate with their friends, family, colleagues, as getting affected by it. A meta-analysis by Ouyang et al. well as how they communicate with people, they have never (2021) found that adolescents and young adults are met offline. People generally have different personality particularly susceptible to these effects due to their in real-life as on virtual life. One can be a completely developmental stage and high engagement levels. Extensive different person. Individuals may present a more idealized use of social media may also lead short temperedness and version of themselves online, which can impact their self- rage in young generation. Somehow it is also stopping kids esteem and real-life interactions. The pressure to maintain from creative thinking. this online persona can lead to changes in how individuals Constructivist educational ideas are emphasized as perceive themselves and are perceived by others. beneficial factors that might improve students' ability to think critically and creatively in math classes. (Sanders, S. Presence and perception: (2016)) As people we as a whole want to be acknowledged and to be Older Generation-It has been studied less but it is becoming approved and perceived by others. When people post on a significant area of interest. Many older adults have social media, they expect to get appreciated by everyone, smartphones these days and they are slowly getting interest get likes and positive comments but if they don’t get likes in it as it is becoming their source of passing time in their and comments as expected they get disappointed. daily lives. Extended periods of screen time, particularly in As indicated by insights uncovered by Young Minds and sedentary positions, can contribute to physical health issues The Children's Society, practically half (47%) of those such as eye strain, neck and back pain, and disrupted sleep matured 11-25 have encountered undermining, scaring, or in patterns. For elderly individuals who may already be prone any case frightful messages by means of social media, email to these health concerns, excessive social media use can or text. Almost a third (32%) have had individual, private, exacerbate these issues. or humiliating data shared publically without their assent by menaces. Two of every five (39%) have felt like they have Addiction and Behavioral Changes: been tormented internet (Harrigian et. al., 2020). Social media platforms have been designed in such a way that no one can resist it and it becomes an addiction day by day. Addiction of social media allows a person to III. METHODOLOGY procrastinate in his/her daily life. The endless scrolling of Research Design and Approach: posts or videos on social platforms has become a loop. This research adopts quantitative approach leveraging a Social media has affected users’ sleep patterns, increased cross-sectional survey to assess the correlation between procrastinating, decreased face to face meetups or social media usage and attention span. The analysis interactions. encompasses Exploratory data analysis (EDA) various Menon, D. (2022). “Factors influencing Instagram Reels statistical models like Linear Regression, Random Forest, usage behaviors.” This study highlighted seven reasons why Gradient Boosting, KNN Regressor, and Decision Tree people use Reels, including socially rewarding self- Regressor are to forecast attention span based on social promotion, amusement, escapism, surveillance, novelty, media metrics. documentation, and trendiness. The following key conclusions were made: Narcissists used Reels more Data Collection Methods and sources: frequently; socially rewarding self-promotion and The dataset obtained from source, Kaggle comprises 26 entertainment predicted video creation and engagement; features related to demographics and social media usage escapist users consumed and engaged more, and such as user_Id, gender, following_rate, avg_Completion, entertainment seekers actively participated in Reels. etc. Data was collected from secondary (existing research datasets) sources, leveraging diverse inspection. Social Media and Loneliness: A user may feel lonely by getting influenced by people Modelling Techniques: posting on social platforms. While these platforms can offer 1.Linear Regression: Linear regression is also a type of online friends and connections, they may also make users machine-learning algorithm more specifically a supervised feel isolated encouraging only online interactions and machine-learning algorithm that learns from the labelled decreasing the quality of real-life relations. High social datasets and maps the data points to the most optimized media use has been associated with increased feelings of linear functions. which can be used for prediction on new loneliness and social isolation, especially among younger datasets. adults. It has increased virtual connections and people have decreased socializing in real-life, decreased the quality time spent together in real-life. 2.Random Forest: Random Forest algorithm is a powerful IV. RESULT tree learning technique in Machine Learning. It works by This study aimed to find the relationship between social creating several Decision Trees during the training phase. media usage and user’s attention span, putting forward that Each tree is constructed using a random subset of the data increased time spent on social media negatively impacts set to measure a random subset of features in each partition. one’s attention span. This randomness introduces variability among individual trees, reducing the risk of overfitting and improving overall Table 1: Model Performance Metrics prediction performance. MODEL MAE MSE R^2 3.Gradient Boosting: Gradient Boosting is a machine learning algorithm, used for both classification and Linear 0.1286 0.0286 0.2505 regression problems. It works on the principle that many Regression weak learners (eg: shallow trees) can together make a more accurate predictor. Gradient boosting works by building simpler (weak) prediction models sequentially where each Random Forest 0.0643 0.0083 0.7834 model tries to predict the error left over by the previous Gradient 0.0685 0.0088 0.7684 model. Boosting KNN 0.0751 0.0110 0.7106 4.KNN Regressor: KNN regression is a non-parametric Regressor method used for predicting continuous values. The core idea Decision Tree 0.0893 0.0163 0.5728 is to predict the target value for a new data point by averaging the target values of the K nearest neighbors in the feature space. The distance between data points is typically measured using Euclidean distance, although other distance Figure-1: Comparison of Model Performance Matrix metrics can be used. 5.Decision Tree Regressor: It is a tree-structured classifier with three types of nodes. The Root Node is the initial node which represents the entire sample and may get split further into further nodes. The Interior Nodes represent the features of a data set and the branches represent the decision rules. Finally, the Leaf Nodes represent the outcome. This algorithm is very useful for solving decision-related problems. Justification of Methods: Suitability: Surveys furnish quantitative data necessary for model training and analysis. The above-mentioned training is selected to manage different types of data correlations and accuracy in prediction. Limitations and Potential Biases: Fig-1 includes a bar graph comparing the MAE, MSE and Limitations: R^2 scores of each model to visually represent the 1. Self-reported data: responses may be vulnerable to performance difference. memory biases and social accessibility biases, potentially impacting the precision of the findings. Figure-2: Gender-wise prediction 2. Cross-Sectional Design: Hinders ability to understand cause – and –effect relationship due to static data (data collected at a single point in time). Potential Biases: Sampling Bias: The sample may not cover broader population ceasing generalizability factor in findings. Platform-Specific Biases: Difference in sustained focus in various social media platforms can bring inaccuracies resulting in possible model biases. Fig-2 shows gender wise prediction and clearly shows that Interpretation- avgDuration is the most important feature for females have more attention span than males. Males are finding out avgCompletion with a core of 0.434. It shows found more addicted towards social media. that the average duration of videos watched has a major impact on the completion rate. Figure-3: Age group prediction 2. avgTimeSpent: 0.379951 Interpretation- avgTimeSpent is also important with a score of 0.380. It shows that average time spent on watching videos also play a major role in determining the completion rate. 3. content_views: 0.063582 Interpretation- It contributes to the model but is less important than the avgDuration and avgTimeSpent. 4. weekdays_trails_watched_per_day: 0.021766 Interpretation- It has a small but a notable impact on avgCompletion,suggesting that weekday video consumption has slight influence on completion rate. Features such as tier,gender,num_of_comments and Fig-3 shows age wise prediction of attention span among following_avg_age have very less impact on the model, users. It includes: suggesting that they may be less relevant for predicting avgCompletion. ‘1’ - less than 18 years old Table 2: Pearson Correlation Coefficients ‘2’ - 18-24 years old Features Correlation P-value ‘3’ - 24-30 years old Value following_rate 0.00199 0.164 ‘4’ - greater than 30 years old avgTimeSpent 0.00164 0.252 It clearly shows that the age group above 30 years old has number_of_words_per_action 0.00544 0.00014 more attention span as compared to the other age groups. avgDuration 0.50040 0.0 weekdays_trails_watched_per_day 0.09952 0.0 Figure-4: Feature Importance from Random Forest Model weekends_trails_watched_per_day 0.11373 0.0 emoji_count_per_action 0.00677 2.23e-06 creations 0.00673 2.55e-06 content_views 012058 0.0 We have performed the hypothesis testing between features to check the relationship between the features and avgCompletion. According to Table-2 it is observed that NEGATIVE RELATIONSHIP: avgDuration has a strong negative correlation with avgCompletion indicating that longer videos might be associated with lower completion rates. In Random Forest model, feature importance is used to determine how much each feature contributes to predicting POSITIVE RELATIONSHIP: Features like the target variable (avgCompletion). Here’s a detailed weekdays_trails_watched_per_day, interpretation of the feature importances provided: weekends_trails_watched_per_day and content_views have positive correlations with avgCompletion, suggesting higher 1. avgDuration: 0.433896 engagement and viewing activity may be associated with better completion rates. While the research’s outcomes validate previous V. DISCUSSION understanding and findings, they also potentially provide The results of this research furnish a comprehension of how new perspective. The robust predictive power of machine attention span is impacted due to social media consumption learning models like Random Forest and Gradient Boosting, using Artificial Intelligence and Machine Learning models. as demonstrated in the studies, produced outcome that The finding unveils insights that align with existing attention span impact prediction has correlation with knowledge in this field, and in some case, expand upon behavioural factors echoes previous research. However, this existing literature in this field. These findings in relation to study goes further by pointing out the importance of specific research objectives have been discussed below and features within these models, leveraging detailed interpreted along with the comparison based on previous understanding of how various aspects of social media usage studies, citing limitations and providing potential directions is detrimental to attention span. for future research. One deviation with the existing literature that this study The key objective of this research was to forecast and trace offers is that the relatively lower importance of the impact of social media on attention span using different followers_avg_age and following_avg_age in forecasting machine learning models. The results, as shown above, attention span. While some previous researches have illustrates that out of all the trained models, Random Forest notably pointed the significance of age-related factors, our model outperformed others, attaining the least MSE findings cite the direct usage metrics to have more (0.0083) and MAE (0.0643), and the highest R^2 score pronounced effect on attention span. (0.7834). This implies that the model is reliable in predicting the impact on attention span using social media The inferences of these findings provide contributions to usage features provided in the dataset. both academic theories and practical applications. The implications of avgDuration and avgTimeSpent as crucial In relation to feature importance, avgDuration and indicators in forecasting attention span emphasize the avgTimeSpent were consistently the most pivotal features importance of more sophisticated approach towards harmful across both the Random Forest and Gradient Boosting effects of social media usage on cognitive functions. For models. For every single second increase in the average example, these findings could provide way to invent duration of videos watched, the avgCompletion metric innovative methods to cease the prolonged usage of social experiences a decrease of 0.00129 units (linear regression media leading to conservation or even improvement of coefficient), indicating a potentially inverse relationship cognitive function like attention among users. between video length and viewer engagement. This suggests that as videos become progressively longer, viewers may be From an academic point of view, this research offers less likely to complete them, ultimately leading to reduced contribution to growing body of knowledge on cognitive completion rates and lower overall viewer satisfaction. This impacts of digital media. The machine Learning models aligns with the existing literature evidence which cites time usage have serve as valuable tools for future research in this spent on social media platforms as critical factor for low field, furnishing more precise and forecasting insights. attention spans in individuals. The comparatively lesser important factors were age_group and gender suggesting Notwithstanding its contributions, this study has its that the behavioural indicators are more contributing to the limitations. First limitation is the dependence on self- predictions than demographic factors. reported data for some aspects, may be resulting in biases or inaccuracies. Secondly, although the machine learning Gender-wise analysis revealed that females generally have a models are reliable but there may be possibilities of higher attention span compared to males, who were found to overfitting, especially with sophisticated models like be more addicted to social media.These implications can be Random Forest and Gradient Boosting. Future research can evidently witnessed in the predictive Machine Learning expand on this by utilizing larger and heterogenous datasets models showing that males get engaged more frequently and as input, as well as exploring and incorporating deep for longer durations with social media platforms, possibly learning approaches. leading to reduced attention spans. This inference aligns with studies that furnish males are more prone to excessive Another potential area for future research is exploration of use of social media. Also, findings also reveal that older correlation between type of social media content consumed age groups might have slightly higher completion rates. or emotional tone of interaction with attention span. Moreover, long-term studies could shed more light on the The hypothesis testing results provide further insights. dynamic changes in social media usage patterns and their Pertaining to statistics, significant correlations were found enduring consequences for cognitive performance. between attention span and several other features, including avgDuration, content_views, and emoji_count_per_action. Strikingly, correlation between avgDuration and attention VI. CONCLUSION span is negative (r = -0.5004, p