HLST 4200 Sessions 7-12 PDF
Document Details
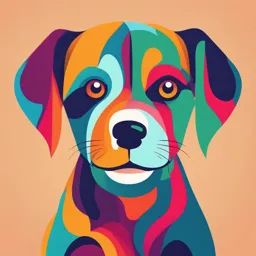
Uploaded by UnforgettableWashington
York University
Tags
Summary
This document provides an overview of various research methods, including literature reviews, systematic reviews, umbrella reviews, realist reviews, mixed systematic reviews, and single-case experiments. It discusses the steps involved in conducting these methods and their applications in various contexts.
Full Transcript
SESSION 7: CHAPTER 5 - Desk Based Research: the process of gathering and researching existing information to answer a research question. Also called secondary research. This research is done via the internet so indirectly - Empirical R...
SESSION 7: CHAPTER 5 - Desk Based Research: the process of gathering and researching existing information to answer a research question. Also called secondary research. This research is done via the internet so indirectly - Empirical Reach: a research where you gather your own data (ex. Through interview or focus groups - Realist synthesis Types of Desk-Based Research: 1. **Literature Reviews:** ○ Purpose: To synthesize existing research on a specific topic, identifying patterns, gaps, and relationships. ○ **Process:** Search Strategy: Develop a systematic approach to locate relevant literature, including databases, keywords, and inclusion/exclusion criteria. Critical Appraisal: Evaluate the quality and relevance of the studies, considering methodologies, findings, and limitations. Synthesis: Integrate findings to provide a coherent narrative or thematic analysis. 2. **Systematic Reviews:** ○ Definition: A rigorous form of literature review that aims to answer a specific research question through comprehensive data collection and analysis. ○ **Characteristics:** Protocol Development: Predefine objectives, criteria, and methods to minimize bias. Comprehensive Search: Include both published and unpublished studies to avoid publication bias. Data Extraction and Analysis: Use standardized forms and, where applicable, statistical methods like meta-analysis. 3. Definition of Umbrella Reviews: An umbrella review is a type of research that looks at multiple existing reviews on a topic to provide a big-picture summary of all the findings. Instead of focusing on individual studies, it gathers information from different systematic reviews and meta-analyses to give a high-level overview of what is already known. Example to Help Remember the Term: Think of an umbrella review like planning a vacation. Instead of looking at individual hotel reviews, flight options, and sightseeing suggestions separately, you read a travel website that summarizes different review sources to give you an overall picture of the best travel choices. The website has already gathered information from various sources, making it easier for you to see the bigger picture without going through each review individually. By visualizing an umbrella covering multiple smaller reviews, you can remember that an umbrella review brings together many different summaries into one comprehensive report. 4. **Realist Reviews:** ○ Focus: To understand the mechanisms through which interventions work (or don't) in particular contexts. ○ **Approach:** Theory-Driven: Develop and refine theories about how interventions achieve outcomes. Context-Mechanism-Outcome (CMO) Configurations: Explore how specific contexts influence the mechanisms and lead to particular outcomes. 5. Mixed Systematic Reviews: Combines both quantitative and qualitative studies to provide a more complete understanding on the topic. 6. Systematic Rapid Reviews: Definition of Systematic Rapid Reviews (in Simple Terms): A systematic rapid review is a type of research that quickly gathers and summarizes existing studies on a topic in a structured and organized way. It follows a systematic process, like a full systematic review, but speeds things up by simplifying certain steps—such as narrowing the search criteria or focusing on fewer sources. This type of review is useful when decisions need to be made quickly, such as in healthcare or policy-making. Example to Help Remember the Term: Imagine you're shopping for a new phone but don’t have much time. Instead of reading hundreds of detailed reviews, you quickly check the top 5 websites that summarize the best options based on key features you care about, like battery life and camera quality. You're still making an informed decision, but much faster than if you reviewed every single phone yourself. By thinking of a systematic rapid review like a fast but organized shopping search, you can remember that it provides reliable information quickly by focusing on the most important sources while maintaining a structured approach. Definition: A rigorous form of literature review that aims to answer a specific research question through comprehensive data collection and analysis. Characteristics: 7. Protocol Development: Predefine objectives, criteria, and methods to minimize bias. 8. Comprehensive Search: Include both published and unpublished studies to avoid publication bias. 9. Data Extraction and Analysis: Use standardized forms and, where applicable, statistical methods like meta-analysis. Literature Reviews: To put together existing research on a specific topic. PROCESS: a. To find out what’s already known about the topic b. To give reader a critical overview of what’s been found c. To integrate findings; find out what’s missing 10. Systematic Review: Purpose: To synthesize existing research on a specific topic, identifying patterns, gaps, and relationships. Process: Search Strategy: Develop a systematic approach to locate relevant literature, including databases, keywords, and inclusion/exclusion criteria. Critical Appraisal: Evaluate the quality and relevance of the studies, considering methodologies, findings, and limitations. Synthesis: Integrate findings to provide a coherent narrative or thematic analysis. 11. Meta-analyses: Quantitative integration of studies that are similar to each other. Once these studies are combined it can generate new results, new data, and new conclusions 12. Scoping Reviews: Type of research that explores the “likely” size and scope of the research literature. 13. The State of the Art Review: Ways to gather important information before writing a report or thesis. It focus on current matters. Example: an annual review PURPOSE: improving the understanding of a topic 14. Desk-based research: Process of gathering and researching existing information to answer a research question. This is done indirectly via the internet. Conducting a literature review can be simplified into clear steps: 1. Define the Scope: Decide on the specific topic or question you want to explore. This helps keep your research focused and relevant. 2. Search the Literature: Look for existing research related to your topic. Use academic databases and libraries to find books, articles, and papers. 3. Assess Quality: Evaluate the credibility of the sources you've found. Prioritize studies that are well-conducted and published in reputable journals. 4. Synthesize Findings: Combine the information from different sources to see the overall picture. Identify common themes, agreements, or disagreements among the studies. 5. Report Results: Organize your findings into a structured format, such as an essay or report, discussing what you've learned and suggesting areas for further research. Conducting a sytematic review can be simplified into clear steps: Steps to Conduct a Systematic Review: 1. Define the Research Question Clearly state what you want to investigate using a structured format such as PICO (Population, Intervention, Comparison, Outcome) for healthcare topics. Example: Does regular exercise reduce blood pressure in adults compared to no exercise? 2. Develop a Review Protocol Outline a plan that details how the review will be conducted, including search strategies, databases to use, inclusion/exclusion criteria, and how data will be analyzed. The protocol helps ensure transparency and consistency. 3. Search the Literature Conduct a comprehensive search using multiple databases (e.g., PubMed, Scopus, Web of Science). Use well-defined search terms and Boolean operators (e.g., AND, OR, NOT) to refine results. Include gray literature (e.g., reports, conference papers) if relevant. 4. Select Relevant Studies Apply inclusion and exclusion criteria to filter studies based on relevance, publication date, study design, population, and interventions. Two independent reviewers usually assess studies to reduce bias. 5. Assess the Quality of Studies Use standardized tools such as: ○ Cochrane Risk of Bias Tool (for randomized trials) ○ Newcastle-Ottawa Scale (for observational studies) ○ PRISMA Checklist (Preferred Reporting Items for Systematic Reviews and Meta-Analyses) This step ensures that only high-quality studies contribute to the review. 6. Extract Data Create a data extraction form to systematically collect key details from selected studies, such as: ○ Study design ○ Population characteristics ○ Intervention details ○ Outcomes measured This helps in organizing the findings for synthesis. 7. Synthesize and Analyze the Findings If data allows, perform a meta-analysis (statistical pooling of results). If not, conduct a narrative synthesis, summarizing findings descriptively and comparing study results. 8. Interpret the Results Discuss what the findings indicate in relation to your research question. Consider limitations such as publication bias, heterogeneity of studies, and potential confounding factors. 9. Report the Findings Structure the report using a recognized guideline such as PRISMA, including: ○ Introduction (background, research question) ○ Methods (how the review was conducted) ○ Results (findings, tables, charts) ○ Discussion (implications, limitations, recommendations) Present results in a transparent and reproducible manner. 10. Update the Review (if necessary) A systematic review should be updated periodically to include new research and keep the conclusions relevant. Example to Help Remember the Steps: Think of conducting a systematic review like organizing a big event: 1. Decide on the event theme (research question). 2. Make a detailed plan (protocol). 3. Search for vendors and venues (literature search). 4. Shortlist based on budget and style (selection criteria). 5. Check their reviews and credibility (quality assessment). 6. Gather details and prices (data extraction). 7. Compare options and make decisions (synthesis). 8. Analyze the best choice (interpretation). 9. Write a detailed event plan (reporting). 10. Keep checking for updates (reviewing periodically). How to Conduct a Realist Review (Simplified Guide) A realist review is a type of literature review that focuses on understanding how and why an intervention works (or doesn’t work) in different situations. Instead of just asking "Does it work?" like a traditional systematic review, a realist review asks deeper questions like: For whom does it work? In what circumstances does it work? Why does it work (or not work)? Steps to Conduct a Realist Review 1. Define the Scope and Research Question Identify the broad topic and refine it into a focused question using a framework like CIMO (Context, Intervention, Mechanism, Outcome). Example: How does mental health support impact students from diverse backgrounds in university settings? The goal is to uncover how and why interventions work under specific conditions. 2. Develop a Review Protocol Plan the review process by outlining: ○ Objectives of the review ○ Inclusion/exclusion criteria ○ Sources of data (e.g., academic literature, reports, interviews) ○ How data will be analyzed Unlike systematic reviews, a realist review remains flexible to allow new insights to emerge. 3. Search the Literature Use academic databases (PubMed, Scopus, Google Scholar) and gray literature (government reports, policy documents). Search broadly to include different contexts and theories. Look for studies that provide explanations (not just results) of how interventions work. 4. Screen and Select Studies Review studies based on relevance to your question rather than rigid inclusion/exclusion criteria. Select papers that offer insights into the context, mechanisms, and outcomes of the intervention. 5. Extract and Organize Data Collect data based on the CIMO framework to understand: ○ Context – What conditions influence the intervention? ○ Intervention – What action is taken? ○ Mechanism – What processes or behaviors make it work? ○ Outcome – What results are observed? Example: A university counseling program might work well for students with social support (context), because it provides coping skills (mechanism), leading to reduced anxiety (outcome). 6. Synthesize Findings (Develop Theories) Identify patterns across studies to understand what works, for whom, and why. Build explanatory models or theories that explain relationships between context, mechanisms, and outcomes. Example: In low-support environments (context), online counseling interventions (intervention) may not provide the emotional connection (mechanism) needed to reduce anxiety (outcome). 7. Refine and Test Theories Check if your theories hold up across different studies and contexts. Adjust your explanations based on new data and stakeholder feedback. 8. Report the Findings Present your findings by explaining: ○ What mechanisms worked (or didn't) in specific contexts ○ Practical recommendations for policymakers or practitioners ○ Areas needing further research Use clear tables or diagrams to show how the intervention works under different conditions. Example to Help Remember the Steps: Think of a realist review like solving a mystery case: 1. You gather clues (different studies). 2. You look at the context (where and when it happened). 3. You identify motives and actions (mechanisms behind the success or failure). 4. You solve the case by understanding why certain factors led to specific results. Advantages of a Realist Review: Helps understand the complexities of real-world interventions. Provides practical insights for policymakers and practitioners. Flexible and adaptable to evolving findings. Challenges of a Realist Review: Requires deep analytical thinking to uncover mechanisms. Can be time-consuming due to the complexity of relationships. May involve subjective interpretation of data. SESSION 8: CHAPTER 6 (P. 135-172) A. Chapter 6: Fixed Designs General Features of Fixed Designs: Emphasis on pre-specification of procedures and control over variables to establish cause-and-effect relationships. Fixed Design (in Simple Terms): Fixed design is a type of research design where the plan is set in advance and remains consistent throughout the study. It is commonly used in quantitative research, where researchers follow a structured approach to collect and analyze data to test a hypothesis. In a fixed design, researchers define all aspects of the study beforehand, such as: The research question The variables being measured The methods for data collection (e.g., surveys, experiments) How data will be analyzed Since everything is planned in advance, fixed designs are useful for making comparisons, finding patterns, and establishing cause-and-effect relationships. Key Characteristics of Fixed Design: 1. Predefined Structure: ○ The study follows a clear, step-by-step plan that does not change once data collection begins. ○ Example: A clinical trial testing a new drug follows a strict protocol from start to finish. 2. Control Over Variables: ○ The researcher controls factors that might influence the results to ensure accuracy. ○ Example: In an experiment, participants are randomly assigned to groups to eliminate bias. 3. Objective Measurement: ○ Data is collected in a standardized way to ensure objectivity and consistency. 4. Reproducibility: ○ Since the design is fixed, other researchers can replicate the study and get similar results. Common Types of Fixed Designs: 1. True Experiments (Randomized Controlled Trials – RCTs): ○ Participants are randomly assigned to groups (e.g., treatment vs. control). ○ Used in healthcare to test new treatments. 2. Quasi-Experiments: ○ Similar to experiments but without random assignment, making them easier to conduct in real-world settings. 3. Surveys: ○ Structured questionnaires with pre-set questions to collect data from large groups. Example to Help Remember Fixed Design: Think of fixed design like a baking recipe. You follow a specific set of instructions (ingredients, steps, baking time), and once you start, you can't change the process. If followed correctly, the result (cake) should turn out the same each time. Advantages of Fixed Design: Provides clear, reliable results. Allows for easy comparison and statistical analysis. Can establish cause-and-effect relationships. Disadvantages of Fixed Design: Less flexibility; can't adjust methods once the study begins. May not fully capture real-world complexity. This chapter delves into fixed research designs, commonly associated with quantitative methods. Key topics include: This first stage seeks to establish – both from discussions with professionals, participants, and others involved and from the empirical data gathered – likely ‘bankers’ for mechanisms that operate in the situation, contexts in which they are likely to operate, and characteristics of the best‐targeted participants. The second fixed‐design phase then incorporates a highly focused survey, experiment, or other fixed‐design study. Even with a preceding exploratory phase, fixed designs should always be piloted. You carry out a mini version of the study before committing yourself to the big one. This is, in part, so you can sort out technical matters to do with methods of data collection - In the true experiment, two or more groups are set up through random allocation of people to the groups. The experimenter then actively manipulates the situation so that different groups get different treatments. - Single‐case design, as the name suggests, focuses on individuals rather than groups and effectively seeks to use persons as their own control, as they are subjected to different, experimentally manipulated conditions at different times. - Quasi‐experiments lack the random allocation to different conditions found in true experiments. - ‘the ecological fallacy’: when a group of people work on an experiment and results are reported as a group rather than in singles to see what each person as done. This leaves room for danger and error as it creates people to assume about the individuals who participated in the data. - Single‐case experimental designs are an interesting exception to the above rule. Most non‐experimental fixed research also deals with averages and proportions. The relative weakness of fixed designs is that they cannot capture the subtleties and complexities of individual human behaviour. For that you need flexible designs. Or, if you want to capture individual complexities as well as group aggregates (wholeness), then a mixed design (both fixed and flexible design) is a more appropriate route to take. Even single‐case designs are limited to quantitative measures of a single simple behaviour or, at most, to a small number of such behaviours. B. Establishing Trustworthiness: Discussion on validity (internal and external) and reliability, ensuring the research measures what it intends to and can be replicated. Summary of "Establishing Trustworthiness in Fixed-Design Research" Fixed-design research requires establishing trustworthiness, which means ensuring the research is accurate, thorough, and unbiased. This involves several critical aspects, including validity, reliability, and generalizability. 1. Trustworthiness in Fixed-Design Research Trustworthiness is about doing a good, honest job in an open and unbiased way. Findings should not be influenced by personal biases or the desire to prove a specific point. Even with good intentions, trustworthiness depends on clear, well-presented, and logically argued reports that answer key research questions. 2. Validity (Are we measuring what we think we are?) Validity refers to whether the research accurately reflects reality. It asks if the relationships established in the findings are real or influenced by external factors. Threats to Validity: 1. Participant Variability: ○ People's performance can change due to factors like tiredness, mood, or external conditions (e.g., hay fever season). ○ Solution: Control test timing or randomize participants. 2. Participant Bias: ○ Participants may alter their responses to please researchers or rebel against the test. ○ Solution: Ensure anonymous responses and neutral environments. 3. Observer Error: ○ The person recording data might make mistakes due to fatigue or personal biases. ○ Solution: Standardized procedures and training. 4. Observer Bias: ○ Observers might unintentionally influence results based on personal beliefs. ○ Solution: Use double-blind assessments and independent reviewers. Types of Validity: 1. Construct Validity: ○ Does the test measure what it claims to measure? ○ Solution: Use well-established tools and multiple measures (triangulation). 2. Criterion Validity: ○ Does the test predict future performance or real-world outcomes? 3. Internal Validity: ○ Have all possible influences on the findings been controlled? 4. External Validity (Generalizability): ○ Can the results be applied to other populations or settings? 3. Reliability (Are the results consistent over time?) Reliability ensures the study's results would be the same if repeated under similar conditions. Threats to Reliability: 1. Testing Conditions: ○ A participant might score differently on different days due to various factors. ○ Solution: Use multiple testing methods and check consistency. 2. Measurement Tools: ○ Instruments or questions used may not be consistent across applications. ○ Solution: Standardize tests and administer them in controlled settings. 3. Observer Differences: ○ Different researchers might interpret results differently. ○ Solution: Train researchers and cross-verify data. Key Concept: Reliability is essential for validity. A test must be reliable to be valid, but a test can be reliable without being valid. 4. Generalizability (Can the findings apply to other groups or settings?) Generalizability, also known as external validity, measures how well research findings can be applied to different contexts. If research results only apply to the specific participants studied, their usefulness is limited. Factors Affecting Generalizability: 1. Sampling Method: ○ Random sampling improves generalizability. 2. Context Differences: ○ Research done in controlled environments may not reflect real-world conditions. Improving Generalizability: Use diverse samples. Conduct studies in multiple locations/settings. Compare results with other studies. 5. Objectivity (Avoiding Bias) Objectivity means minimizing personal influence or preconceived notions that could distort research outcomes. The goal is to ensure that results reflect reality, not researcher expectations. Solutions to Ensure Objectivity: Use blind or double-blind methods. Document and justify each research step. Seek peer review and feedback. 6. Credibility (How convincing are the findings?) Credibility is established by providing detailed information on research methods, justifying choices, and ensuring findings are well-supported. Ways to Improve Credibility: Transparency about methods and limitations. Clear and logical presentation of data. Comparing findings with other credible studies. 7. Threats to Internal Validity (Key Challenges) The book outlines 12 threats that can compromise internal validity, such as: 1. History: ○ Events outside the research can affect results. ○ Example: A national policy change during a study. 2. Testing Effects: ○ Repeated testing may improve scores due to familiarity. 3. Instrumentation: ○ Changes in measurement tools over time can affect results. 4. Maturation: ○ Participants naturally change over time, influencing outcomes. 5. Mortality (Dropout Rates): ○ Participants leaving the study can bias results. 6. Compensatory rivalry or the John Henry effect: This is a similar effect, but on the participants themselves: a group in an organization sees itself under threat from a planned change in another part of the organization and improves its performance. Example: John Henry, the steel worker who killed himself through overexertion to prove his superiority to a new steam drill. How to Address These Threats: Use control groups. Random assignment. Longitudinal tracking. 8. Practical Strategies for Ensuring Trustworthiness Triangulation: ○ Use multiple sources and methods to confirm findings. Pilot Testing: ○ Test procedures on a small group before the full study. Audit Trails: ○ Keep detailed records of all research decisions and data. How to Remember These Concepts (Memory Aid): Use the acronym "VRGO" to remember the key elements: V – Validity (Are you measuring what you intend to?) R – Reliability (Are your results consistent?) G – Generalizability (Can your findings apply elsewhere?) O – Objectivity (Are you unbiased?) C. True Experiments: Exploration of randomized controlled trials (RCTs), highlighting random assignment and control groups as gold standards for causal inference. Summary of True Experiments in Fixed-Design Research True experiments are a highly controlled research method designed to test cause-and-effect relationships by randomly assigning participants to different groups. This randomization helps ensure that the groups are comparable, making it easier to determine whether an intervention or treatment is responsible for the observed outcomes. Key Characteristics of True Experiments: 1. Random Allocation: ○ Participants are randomly assigned to groups (e.g., treatment vs. control), reducing biases and ensuring fairness. 2. Control Over Variables: ○ The researcher manipulates one or more independent variables (IVs) while controlling external factors. 3. Comparison Groups: ○ Results from different groups are compared to determine the effect of the intervention. 4. Replicability: ○ True experiments follow a structured process, allowing for replication to confirm findings. Types of True Experimental Designs (Overview from Box 6.5): 1. Two-Group Designs (Most Common) Post-Test-Only Randomized Controlled Trial (RCT): ○ Participants are randomly assigned to either an experimental group (receives treatment) or a control group (no treatment). ○ Outcome is measured after the intervention. Post-Test-Only Two-Treatment Comparison: ○ Participants are randomly assigned to one of two different treatment groups, and results are compared. Pre-Test Post-Test RCT: ○ Participants are randomly assigned to treatment or control groups, and their outcomes are measured before and after the intervention. Pre-Test Post-Test Two-Treatment Comparison: ○ Two experimental groups receive different treatments, and their pre- and post-treatment results are compared. 2. Three-Group or More Designs Builds on the two-group designs by adding more experimental groups to compare multiple treatments, while retaining a control group. 3. Factorial Designs Used when there are two or more independent variables, such as studying the impact of both diet and exercise on weight loss. Participants are assigned to different combinations of the variables (e.g., diet only, exercise only, both, or neither). Helps identify how variables interact with each other. 4. Parametric Designs Focuses on testing different levels of an independent variable (e.g., testing different doses of a drug). Allows researchers to analyze trends and variations in response to varying treatment intensities. 5. Matched Pairs Design Participants are paired based on similarities in a key variable (e.g., age, gender, or baseline performance). Each pair is randomly split into different treatment groups. Helps reduce the impact of individual differences. 6. Repeated Measures Design The same participants are tested under different conditions (e.g., with and without medication). Participants serve as their own controls, making the study more efficient and sensitive to treatment effects. Advantages of True Experimental Designs Strong evidence of causation: ○ Because of randomization and control, results are more reliable in establishing cause-and-effect relationships. Minimizes bias: ○ Random assignment helps eliminate biases and confounding variables. Standardization: ○ Procedures are consistent, making replication easier. Challenges and Limitations of True Experimental Designs Ethical concerns: ○ It may not be ethical to withhold treatment from control groups. Practical difficulties: ○ Conducting experiments in real-world settings can be complex. Cost and time: ○ True experiments can be expensive and time-consuming to conduct. Choosing the Right True Experimental Design (Guidelines from Box 6.6): When selecting a true experimental design, consider the following: Use a Matched Design when: ○ You have a known variable that strongly correlates with the dependent variable. ○ Participants' individual differences might affect results. Use a Repeated Measures Design when: ○ Testing the same participants multiple times is practical. ○ Individual differences might interfere with outcomes. Use a Simple Two-Group Design when: ○ Participants are only exposed to one treatment. ○ Pre-testing could influence results. Use a Before-After Design when: ○ You need to compare participants’ outcomes over time. Use a Factorial Design when: ○ Multiple variables are involved and their interactions are of interest. Use a Parametric Design when: ○ You need to measure the effect of different levels of an intervention. Situations Conducive to Randomized Experiments (Box 6.7): 1. When demand outstrips supply: ○ Randomization may be seen as a fair way to allocate resources. 2. When an innovation is introduced gradually: ○ Allows randomization by selecting which group gets the intervention first. 3. When experimental units are isolated: ○ If different locations or populations are independent, randomization is easier. 4. When decision-makers are unsure about solutions: ○ Random allocation provides an objective way to test solutions. 5. When a tie occurs (borderline cases): ○ Randomly selecting participants from a pool of equally eligible candidates. 6. When individuals express no preference for alternatives: ○ If participants are indifferent to treatment options, they can be randomly assigned. How to Remember True Experimental Designs (Memory Aid) Think of true experiments like baking a cake with a new recipe: 1. Random Allocation (Choosing Ingredients): ○ Randomly select ingredients (participants) without bias. 2. Control Over Variables (Exact Measurements): ○ Carefully control how much of each ingredient is used. 3. Comparison Groups (Taste Testing): ○ Give some people the new recipe (treatment) and others the old one (control). 4. Analysis (Checking the Taste): ○ Compare the results to see if the new recipe works better. By remembering that true experiments are like following a recipe, it becomes easier to recall their structure and purpose. Conclusion: True experiments are a powerful way to test hypotheses and determine causal relationships. By carefully choosing the right experimental design, researchers can minimize biases, ensure validity, and produce reliable, generalizable results. Quasi-Experiments: Examination of designs lacking random assignment, such as non-equivalent control group designs and time-series designs, and strategies to mitigate associated validity threats. Summary of Quasi-Experiments in Fixed-Design Research Quasi-experiments are research designs that attempt to establish cause-and-effect relationships, like true experiments, but without random assignment to treatment and control groups. They are widely used in real-world settings where randomization is impractical or unethical. Key Characteristics of Quasi-Experiments 1. No Random Assignment: ○ Groups are formed based on pre-existing characteristics (e.g., classroom groups, workplace teams). 2. Comparison of Groups: ○ There is usually a treatment group and a comparison (control) group, but group differences may already exist. 3. Flexibility in Design: ○ More adaptable to real-world settings, but interpretation is more complex due to potential confounding variables. 4. Threats to Validity: ○ Since groups are not randomly assigned, the study is more vulnerable to biases (e.g., selection bias, maturation). Types of Quasi-Experimental Designs (Overview from Box 6.8) 1. Pre-Experimental Designs (To Be Avoided) These designs are considered weak because they lack control and provide limited evidence for causal relationships. 1. Single-Group Post-Test-Only: ○ Participants receive the intervention, and their outcomes are measured after. ○ Problem: No pre-test, so it’s unclear if changes are due to the intervention or other factors. ○ Example: A new teaching method is introduced, and student performance is measured afterward without knowing their previous level. 2. Post-Test-Only Non-Equivalent Groups: ○ Two pre-existing groups (one receiving treatment, the other not) are compared after the intervention. ○ Problem: Differences could exist between groups before treatment, leading to biased results. 3. Pre-Test Post-Test Single-Group Design: ○ A single group is measured before and after treatment. ○ Problem: Changes could be due to factors like maturation or historical events rather than the intervention itself. 2. Quasi-Experimental Designs to Consider These designs offer stronger validity and are commonly used when randomization is not feasible. 1. Pre-Test Post-Test Non-Equivalent Groups Design: ○ Two or more groups are tested before and after treatment without random assignment. ○ Strength: Comparing pre-test scores allows for some control over initial differences. ○ Weakness: Differences between groups might still exist. 2. Interrupted Time-Series Design: ○ A single group is measured repeatedly before and after the intervention to track changes over time. ○ Strength: Trends can be analyzed, helping identify whether changes are due to the intervention. ○ Weakness: External factors may influence results over time. 3. Regression-Discontinuity Design: ○ Participants are assigned to groups based on a cutoff score from a pre-test (e.g., low scorers receive intervention, high scorers do not). ○ Strength: Provides strong causal inference without randomization. ○ Weakness: Requires precise cutoff criteria and large sample sizes. Why Use Quasi-Experiments? While quasi-experiments are sometimes seen as a "second-best" option compared to true experiments, they have several advantages, including: Ethical feasibility: ○ Suitable when random assignment is not possible (e.g., in educational or medical settings). Practicality: ○ They allow studying interventions in real-world conditions. Flexibility: ○ Easier to implement in existing environments such as schools or workplaces. However, researchers must carefully consider threats to validity, as the absence of randomization can lead to selection bias, making it difficult to determine if the treatment truly caused the observed effect. Threats to Validity in Quasi-Experiments Since quasi-experiments lack randomization, several threats to validity must be considered: 1. Selection Bias: ○ Differences between groups may exist before the intervention, affecting outcomes. ○ Solution: Use pre-tests to assess baseline equivalence. 2. History Effects: ○ Events occurring during the study (e.g., policy changes) might influence results. ○ Solution: Compare results across multiple time periods. 3. Maturation Effects: ○ Participants naturally change over time, which can affect results. ○ Solution: Use control groups for comparison. 4. Regression to the Mean: ○ Extreme pre-test scores may naturally return to average values over time. ○ Solution: Consider using repeated measures to track trends. Choosing the Right Quasi-Experimental Design (Box 6.9–6.11 Recommendations) 1. Use Pre-Test Post-Test Non-Equivalent Groups When: Random assignment is not possible. A baseline comparison is needed to detect changes. 2. Use Interrupted Time-Series When: The intervention effect needs to be tracked over time. You have access to multiple data points before and after intervention. 3. Use Regression-Discontinuity When: A clear cutoff exists for treatment allocation. You want to establish causal relationships without randomization. Common Scenarios Where Quasi-Experiments Are Used (Real-Life Applications) 1. Education: ○ Evaluating a new teaching method by comparing different schools. 2. Healthcare: ○ Studying the impact of a public health campaign in different cities. 3. Social Policy: ○ Assessing the effect of a new government policy across different regions. Strengths and Limitations of Quasi-Experiments Strengths: More practical and applicable to real-world problems. Ethical and feasible in complex environments. Allows for analysis of long-term trends. Limitations: Higher risk of bias due to lack of randomization. Difficult to control for external factors influencing results. Requires careful interpretation of findings. How to Remember Quasi-Experiments (Memory Aid) Think of quasi-experiments like observing a classroom with two different teaching styles: 1. Without Random Assignment: ○ You cannot randomly choose which students get which teacher. 2. Comparing Outcomes: ○ You still compare their test scores before and after the new teaching style is introduced. 3. Potential Biases: ○ Some students may already be better at studying than others, which could affect the results. By thinking of quasi-experiments as studying groups where randomness is not possible but comparisons are still made, you can easily remember their purpose and challenges. Conclusion: Quasi-experiments are a valuable research tool when randomization is not feasible, offering flexibility and practical applications. However, researchers must take extra steps to account for potential biases and threats to validity to ensure the credibility of their findings. Single-Case Experiments: Focus on intensive study of individual cases over time, utilizing designs like ABAB to assess intervention effects. Summary of Single-Case Experiments in Fixed-Design Research Single-case experiments (also known as small-N, single-subject, or applied behavior analysis designs) focus on studying an individual or a small number of participants to determine the effects of an intervention. This approach, pioneered by B.F. Skinner, emphasizes careful observation and repeated measurement over time, often without relying on statistical analysis. Key Characteristics of Single-Case Experiments: 1. Focus on Individual Cases: ○ The experiment is conducted on a single subject (e.g., a person, class, or organization), making it highly detailed and personalized. 2. Phased Interventions: ○ Observations are made before, during, and after the intervention to assess its effectiveness. 3. Baseline Stability: ○ Establishing a stable baseline before introducing the intervention is crucial for meaningful interpretation. 4. Visual Analysis of Data: ○ Data is often analyzed visually (by "eyeballing" graphs), although statistical tests can now supplement interpretation. 5. Replicability: ○ Typically repeated with a small number of participants to establish the reliability of findings. Types of Single-Case Designs (Overview from Box 6.12): 1. A–B Designs (Basic Design) Structure: ○ Phase A: Baseline (pre-intervention observations) ○ Phase B: Treatment (observations during intervention) Purpose: ○ To compare pre- and post-treatment results. Weaknesses: ○ Vulnerable to validity threats such as history or maturation effects. Example: ○ Monitoring a student’s attention span before and after introducing a new teaching method. 2. A–B–A Designs (Reversal Design) Structure: ○ Phase A: Baseline ○ Phase B: Treatment ○ Phase A: Return to baseline (removal of intervention) Purpose: ○ To determine if changes revert after treatment withdrawal, strengthening causal inference. Weaknesses: ○ Ethical concerns about withdrawing an effective intervention. Example: ○ Testing a new medication, then stopping it to see if symptoms return. 3. A–B–A–B Designs (Repetition of Treatment) Structure: ○ Phase A: Baseline ○ Phase B: Treatment ○ Phase A: Return to baseline ○ Phase B: Reintroduction of treatment Purpose: ○ Provides stronger evidence of cause and effect by demonstrating treatment effectiveness twice. Example: ○ Evaluating the impact of a behavioral intervention, then reintroducing it for confirmation. 4. Multiple-Baseline Designs Used when withdrawing treatment is impractical or unethical. It involves introducing the intervention at different times across: 1. Settings: ○ Example: Observing behavior at home, school, and daycare, introducing intervention at different times in each. 2. Behaviors: ○ Example: Tracking different disruptive behaviors (e.g., shouting, fidgeting) and applying treatment to one behavior at a time. 3. Participants: ○ Example: Implementing the intervention for different students at staggered intervals. Strength: Stronger evidence of treatment effect without withdrawal. 5. Changing Criterion Designs Structure: ○ A gradually increasing or decreasing performance criterion is set for the participant. Purpose: ○ To test whether the participant’s behavior changes progressively with the new standards. Example: ○ Gradually reducing the number of cigarettes smoked per day over weeks. 6. Multiple-Treatment Designs Structure: ○ Several interventions (B, C, etc.) are applied sequentially to assess their relative effects. Purpose: ○ To compare multiple treatments or interventions without reverting to baseline conditions. Example: ○ Comparing different therapy approaches for reducing anxiety. Advantages of Single-Case Experiments 1. Customization: ○ Tailored to the individual or specific setting. 2. Practical and Cost-Effective: ○ Requires fewer participants and resources. 3. Ethical Feasibility: ○ Suitable when traditional randomization isn't possible. 4. Flexibility: ○ Designs can be modified based on emerging data. Limitations of Single-Case Experiments 1. Limited Generalizability: ○ Findings may not apply to a broader population. 2. Reliance on Visual Inspection: ○ Eyeballing results can introduce subjectivity and inconsistency. 3. Baseline Challenges: ○ Establishing a stable baseline can be difficult in dynamic environments. 4. Carry-Over Effects: ○ If treatment effects persist, it may be challenging to revert to baseline. When to Use Single-Case Experiments Behavioral Interventions: ○ Applied behavior analysis (ABA) in therapy or special education. Clinical Trials: ○ Testing new medical treatments on individuals with unique conditions. Workplace Studies: ○ Evaluating the impact of policy changes within an organization. Threats to Validity in Single-Case Experiments 1. History Effects: ○ Other events occurring during the study may influence results. ○ Solution: Use multiple baselines to compare changes. 2. Maturation Effects: ○ Natural changes over time can affect outcomes. ○ Solution: Conduct repeated measurements over time. 3. Observer Bias: ○ The researcher may unintentionally influence outcomes. ○ Solution: Use independent observers for data collection. 4. Regression to the Mean: ○ Extreme initial scores may naturally return to average levels. ○ Solution: Use repeated observations for accuracy. How to Remember Single-Case Experiments (Memory Aid) Think of single-case experiments like testing a new study technique on yourself: 1. A-B Design (Before & After): ○ Try a new study method for a week and see if your grades improve. 2. A-B-A Design (Reversal): ○ Stop using the method and see if grades drop back. 3. A-B-A-B Design (Repetition): ○ Use the technique again to confirm improvements. 4. Multiple Baseline Design: ○ Apply the method to different subjects (e.g., essays vs. quizzes) at different times. Conclusion: Single-case experiments offer a powerful and flexible approach for testing interventions at an individual level. While they may not provide the broad generalizability of larger experimental designs, they are highly valuable in applied settings where individualized assessment and intervention are critical. By combining visual inspection with modern statistical methods, researchers can ensure their findings are both reliable and valid SESSION 9: CHAPTER 11 This chapter provides a comprehensive guide to designing and implementing surveys and questionnaires: Designing Surveys: Steps for planning, including defining objectives, selecting the target population, and choosing the mode of administration (e.g., online, face-to-face). Carrying Out a Sample Survey: Detailed procedures for sampling, emphasizing the importance of representative samples and various sampling techniques (probability and non-probability sampling). Designing and Using Questionnaires: Best practices for question formulation, including clarity, neutrality, and avoiding leading or double-barreled questions. Discussion on question types (open vs. closed) and scaling methods (Likert scales). Diaries: Use of participant diaries as a data collection tool, advantages of capturing real-time data, and considerations for implementation. Sampling in Surveys: In-depth look at sampling methods, including stratified, cluster, and systematic sampling, and their applicability. SESSION 11: CHAPTER 12 This chapter explores qualitative data collection methods through interviews and focus groups: Types and Styles of Interviews: Comparison of structured, semi-structured, and unstructured interviews, and guidance on selecting the appropriate format based on research objectives. Advantages and Disadvantages of Interviews: Discussion on the depth of data obtained versus potential biases and resource intensiveness. General Advice for Interviewers: Tips on building rapport, active listening, probing techniques, and maintaining neutrality. Content of the Interview: Guidance on developing interview guides, sequencing questions, and ensuring coverage of key topics. Carrying Out Different Types of Interviews: Practical considerations for various interview settings, including telephone and online interviews. Focus Groups: Insights into group dynamics, facilitation techniques, and the benefits of interactive discussions for data richness. Dealing with Interview Data: Strategies for transcription, coding, and analysis to derive meaningful insights. Skills in Interviewing: Emphasis on developing interpersonal skills, cultural sensitivity, and adaptability. SESSION 12: CHAPTER 18 This chapter focuses on methods for analyzing qualitative data: Types of Qualitative Analysis: Overview of approaches like thematic analysis, narrative analysis, and discourse analysis. Using the Computer for Qualitative Data Analysis: Introduction to software tools (e.g., NVivo, Atlas.ti) that assist in organizing and coding qualitative data. Dealing with the Quantity of Qualitative Data: Techniques for managing large volumes of data, including data reduction and summarization. Thematic Coding Analysis: Step-by-step guide to coding data, identifying themes, and building theoretical frameworks. Data Analysis in Grounded Theory Studies: Explanation of grounded theory methodology, including open, axial, and selective coding processes. Alternative Approaches to Qualitative Analysis: Discussion of phenomenological analysis, content analysis, and other methodologies. Integrating Qualitative and Quantitative Data in Mixed Designs: Strategies for combining data types to enrich findings and provide comprehensive insights.