Observation Oriented Modeling PDF - Teaching & Research
Document Details
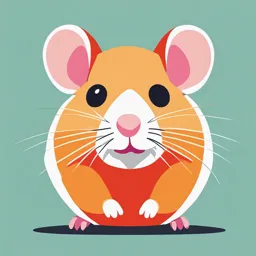
Uploaded by JamesWGrice_OSU
Oklahoma State University
2014
James W. Grice
Tags
Summary
This document, published in 2014, by James W. Grice, presents Observation Oriented Modeling (OOM) as an alternative to traditional statistical methods in research. It focuses on teaching OOM to students in the life sciences. The document explains OOM, emphasizing its focus on assessing the accuracy of judgments based on observations. The author discusses the shift away from aggregate statistics toward integrated models.
Full Transcript
COMPREHENSIVE PSYCHOLOGY 2014, Volume 3, Article 3 ISSN 2165-2228 DOI 10.2466/05.08.IT.3.3 © James W. Grice 2014 NOTICE Attribution-NonCommercial- NoDerivs CC-BY-NC-ND Received October 14, 2013 Accepted January 23, 2014 Published Febru...
COMPREHENSIVE PSYCHOLOGY 2014, Volume 3, Article 3 ISSN 2165-2228 DOI 10.2466/05.08.IT.3.3 © James W. Grice 2014 NOTICE Attribution-NonCommercial- NoDerivs CC-BY-NC-ND Received October 14, 2013 Accepted January 23, 2014 Published February, 2014 Re-licensed December 9, 2015 This Open Access article originally appeared in Innovative Teach- ing, published by Ammons Scientific LTD. It is reproduced in the following pages in that form. CITATION Innovative Teaching was sold to SAGE Publishing Inc., and will not Grice, J. W. (2014) Observation be published after December 31, 2015. Oriented Modeling: preparing students for research in the 21st century. Comprehensive Psychology, With their permission, the authors are hereby issued a new Creative 3, 3. Commons license for the article to be included in Comprehensive Psychology. In this manner, the article can continue to be accessed and cited in an active Open Access journal, operated now by SAGE Publishing Inc. This article should be cited as a part of Comprehensive Psychology in the format listed in the side bar of this cover page. The original DOI has not changed. Ammons Scientific www.AmSci.com INNOVATIVE Observation Oriented Modeling: Preparing students for TEACHING research in the 21st century1 2014, Volume 3, Article 3 ISSN 2165-2236 James W. Grice DOI 10.2466/05.08.IT.3.3 Oklahoma State University © James W. Grice 2014 Attribution-NonCommercial- NoDerivs CC-BY-NC-ND Abstract Observation Oriented Modeling is an alternative to traditional methods of data conceptualization and analysis that challenges researchers to develop inte- grated, explanatory models of patterns of observations. The focus of research Received October 14, 2013 is thus shifted away from aggregate statistics, such as means, variances, and Accepted January 23, 2014 correlations, and is instead directed toward assessing the accuracy of judgments Published February, 2014 based on the observations in hand. In this paper a number of example data sets will be used to demonstrate how Observation Oriented Modeling can be taught to undergraduate and graduate students. While the examples are drawn from psychology, the method of contrasting Observation Oriented Modeling with traditional methods of research design and statistical analysis can easily be adapted to examples from other sciences. CITATION Grice, J. W. (2014) Observation Oriented Modeling: prepar- Overview ing students for research in Observation Oriented Modeling (Grice, 2011) is a novel and intuitive approach for both con- the 21st century. Innovative ceptualizing and analyzing data in the social and biological sciences. Imagine a scientist, for Teaching, 3, 3. instance, studying a mother and child interacting in a laboratory. Countless observations can be made regarding the mother and child in this artificial setting. Physical characteristics such as height and weight can readily be measured, behaviors such as talking and touching can be directly observed, and qualities such as parenting style or child temperament may be inferred from other observable behaviors. The goal of the observation-oriented scientist is to construct an explanatory model for the observations being made, and this goal is equivalent to seeking the causal structure of the natural system under investigation. In other words, the observation oriented scientist assumes that the persons, physical features, behaviors, and qualities directly or indirectly observed are organized or oriented toward one another in consistent and knowable ways because of nature's causal structure. Of course no scientist is a passive observer or tabula rasa; therefore, observations are structured and ordered the way they are in part because of the scientist's judgments. Plac- ing toys or magazines in the room, for instance, may affect the mother-child interaction and the subsequent observations. Decisions must also be made regarding which physical features or behaviors are to be observed and how they are to be recorded, and methods must be derived for assessing qualities that are not directly observable. To insure that his or her model captures the causal structure of nature accurately, then, the observation-oriented scientist must also be oriented toward the many assumptions and decisions that go into making observations. As an example, consider the commonplace strategy among person- ality psychologists of summing responses from multiple-item inventories to obtain trait scores. This procedure assumes that personality traits are structured as continuous quan- tities and can be measured as such, even though no scientific evidence exists for this latter assumption (Michell, 2011). For the observation-oriented scientist this is an untenable posi- tion. Either evidence must be gathered to support the continuity claim or the various mod- els of traits must be altered to provide a more accurate view of reality. The latter approach would require personality psychologists to return to the question “What is a personality trait?” and to devise novel methods of observation in conjunction with their new models. To accomplish the goal of constructing a model that accurately captures the causal structure of nature, the observation-oriented scientist must furthermore steer away Ammons Scientific 1 Address correspondence to James W. Grice, Ph.D., Department of Psychology, 116 North Murray, Oklahoma www.AmmonsScientific.com State University, Stillwater, OK 74078, or e-mail ([email protected]). Teaching OOM / J. W. Grice from the modern paradigm of statistical modeling. This We begin with an explanation of the impetus behind the paradigm is perhaps epitomized by structural equa- development of Observation Oriented Modeling. tion and path diagrams that connect constructs and variables in networks of associations. The variables in Why Observation Oriented Modeling? these diagrams are often assumed to represent continu- The majority of students today are taught that psycho- ous quantities and their analysis is consequently based logical research can be subdivided into three areas: sta- on aggregate statistics, such as means, variances, and tistical analysis, research design, and measurement. covariances. Their efficacy is moreover almost uni- As revealed in a recent review of doctoral programs in versally assessed via the estimation of abstract popu- the United States by Aiken, West, and Millsap (2008), lation parameters in the assumption-laden context of students receive more training in statistics than either null hypothesis significance testing (i.e., via p values). research design or measurement, although Aiken, et al. In contrast, the observation-oriented scientist must be are quick to point out that training in all three areas is willing to get beyond these methods and re-orient his inadequate. They also imply in their review that insuffi- or her attention to the observations being made and cient training – coupled with declining numbers of quanti- to the individuals being studied. Rather than compute tative psychologists – will have a decidedly negative effect aggregate statistics and estimate population parame- on the overall quality of future research in psychology. It ters, patterns of observations are instead examined in could in fact be argued that the predicted decline in qual- light of a causal model using analysis techniques that ity has already occurred, as recent scholarly critiques have are relatively free of assumptions and yield results that highlighted a general lack of understanding among mod- are both transparent and interpretable at the level of the ern researchers with regard to fundamental issues of sta- individuals in the study. The overall analysis objective tistics (particularly the p value; Gigerenzer, 2004; Lambdin, is thus to assess the accuracy of a model as an explana- 2012), research design (Lebel & Peters, 2011), and mea- tion of patterns of observations. surement (Michell, 2008, 2011). Highly publicized cri- As a novel approach for conceptualizing and ana- tiques have moreover spotlighted psychologists' general lyzing data, the use of Observation Oriented Model- neglect of fundamental principles of scientific investiga- ing requires something of a Gestalt shift on behalf of the tion, particularly the importance of exact replication and scientist trained in contemporary experimental statis- the value of critical discourse among colleagues (Lehrer, tics. The purpose of this paper is to facilitate this shift by 2010; Alcock, 2011; Yong, 2012; Abbott, 2013). providing instructional information and class projects to While these recent critical appraisals may seem to teach Observation Oriented Modeling to graduate and support Aiken, et al.'s call for more quantitative train- advanced undergraduate students in the life sciences. The ing, other scholars have drawn attention to a deeper set information and projects presented below are likely most of issues that must first be addressed. Joseph Rychlak suitable for an existing course on experimental statistics or (1985, 1988) argued nearly thirty years ago that psychol- research methods, and the example analyses can be taught ogists have a tendency to permit their methods to drive alongside traditional methods of data analysis. In fact, the their metaphysics (their general view of human nature), example analyses below compare and contrast traditional whereas in proper scientific investigation methods must methods with Observation Oriented Modeling. be devised according to a suitable metaphysics. David As will be made plain below as well, however, a basic Bakan (1967) referred to this error more forcefully as knowledge of the different philosophical schools of “methodolatry” – the irrational belief that nature should thought (e.g., positivism, realism, idealism) is required conform to research methods rather than the other way of the instructor. Accordingly, important concepts and round. In the domain of statistics, David Freedman, ideas that must be taught to students are discussed gen- author of one of the most highly regarded textbooks erally, and they are also specifically presented before on statistics (Freedman, Pisani, & Purves, 2007), argued each example project. The projects themselves are based for a return to simpler statistical methods with greater on genuine or contrived studies and are designed to emphasis placed on sound theorizing and sleuth-like demonstrate: (1) the application of key concepts; (2) the detective work (1991; see also Mason, 1991). Psychol- development of integrated, causal models; and (3) how ogists' tendency to idolize complex statistical methods data can be analyzed with the Observation Oriented at the expense of sound reasoning was more recently Modeling (OOM) software.2 The examples are drawn dubbed “statisticism” by James Lamiell (2013), which fits from the psychological literature but students from well with other descriptions of modern statistical analy- other life sciences (e.g., biology, sociology, education) sis as “sorcery” (Lambdin, 2012), “mindless” (Gigeren- should find them accessible and meaningful as well. zer, 2004), and “inevitable and useless” (Toomela, 2010). With regard to measurement, Joel Michell has argued 2 The OOM software can be downloaded for free at http://www.id persuasively that psychologists have, since the early iogrid.com/OOM. Additional information regarding the software and the Observation Oriented Modeling book (Grice, 2011). can also be 1900s, avoided the fundamental issue of measurement; found at this website. namely, the establishment of additive units of measure Innovative Teaching 2 2014, Volume 3, Article 3 Teaching OOM / J. W. Grice for the psychological attributes they study. The conse- is regarded as his or her score on a psychometrically quent being, “There is no evidence that the attributes sound intelligence test. The circularity of this alleged that psychometricians aspire to measure (such as abili- solution is obvious and unfortunate, but it is also neces- ties, attitudes and personality traits) are quantitative” sary if the things themselves—as they truly are—cannot (Michell, 2011, p. 245). If Michell's assessment is correct, be known to us on some level. then psychologists have been using and advocating In contrast, moderate realism posits that things have methods that often presume continuous quantities (e.g., essences (or natures) and that these natures are indeed ANOVA, SEM, IRT, etc.) where no evidence exists that knowable. Knowledge of essences may at times be intu- the qualities or attributes they study are in fact continu- itive or even confused, but it is nonetheless a power of ous. Again, this is an instance of psychologists allowing the human intellect that makes science possible (Wallace, their methods to drive their metaphysics. 1996; Dougherty, 2013). In the simplest terms, imagine a The premise underlying the development of Obser- scientist who wishes to study trees. Presupposed in said vation Oriented Modeling and the OOM software is that study is the capability of discerning trees from shrubs, the arguments of methodolatry and statisticism are essen- various grasses, and ferns, to name a few plants, not tially correct; and they are correct because psychology has to mention the millions of animals and other objects of built its research methods foundation primarily upon the nature that are not trees. Students can walk around cam- tenets of philosophical positivism, which is a viewpoint pus and examine one hundred different trees without steeped in phenomenalism and long ago abandoned by ever once confusing a tree for a shrub or a tree for a per- philosophers due to its many additional inadequacies (see son. Their ability to abstract from the countless individ- Costa & Shimp, 2011). What is currently needed, then, is ual, unique objects the universal essence “tree” is what a way of thinking about research that does not necessar- makes this exercise possible. Now, a student may encoun- ily entail training in contemporary statistical methods ter a shrub, such as a crape myrtle, and wonder if it is a or psychometric theory, but instead emphasizes model small tree; but this uncertainty in no way removes the stu- building and model evaluation from the perspective of dent's certainty about all of the other trees encountered. philosophical realism. Observation Oriented Modeling is With the crape myrtle, the student investigates further, one such alternative in which students are challenged to moving from what is well known into a part of the world confront human nature and the qualities people possess in which his or her knowledge is less certain. Through while building causal models that emphasize the integra- critical thought, careful investigation, and research the tion of structures and processes rather than the construc- student may come to realize the crape myrtle is best clas- tion and analysis of networks of variables in a structural sified as a shrub because it normally has multiple stems. equation or path diagram. In moderate realism essences, or the “whatness” of things, are distinguished from their various qualities A General Change in Perspective (or accidents). Whereas trees and persons are integrated As mentioned above learning about Observation Ori- wholes existing of themselves, qualities exist within trees ented Modeling involves something of a Gestalt shift or and persons, e.g., some trees are evergreens whereas oth- change in perspective that can be described as a move ers lose their leaves in winter, and some people have blue from phenomenalism to moderate realism. Phenom- eyes whereas others have brown eyes. Empirical psychol- enalism is a catchall term that encompasses important ogy often busies itself with the study of qualities, includ- aspects of positivism and the philosophies of Descartes, ing determining whether or not a given quality, like intelli- Hume, Kant, and others. The most important common gence, is structured continuously and measurable as such. feature of these philosophies is that the essences of It is therefore critical to ask “what is” types of questions. If things cannot be known; in other words, as Kant would intelligence is not in fact a continuous quality, for instance, say, the “things-in-themselves” cannot be known. When then all current tests of intelligence are misguided. While discussing nature through phenomenalism, human- this statement may be dramatic, the premises of moderate ity will always be limited to the appearances of things realism require students to ask such difficult questions to as they are represented in consciousness. This attitude, push their knowledge further and further toward confor- advocated by Stanovich (2007, pp. 35–52) in his popu- mity with the ways things are truly arranged in nature. lar book How to Think Straight about Psychology, remark- A useful class exercise in light of this discussion is to ably prevents psychologists from asking “what is” types require students to answer the question, “What is hand- of questions. Questions such as “What is intelligence?” edness, and how do I observe (or measure) it?” Hand- are regarded as misguided and scientifically useless edness is an excellent choice for this question because because psychologists work with numeric representa- it is something all students understand and experience tions of concepts that can be manipulated and analyzed in their daily lives. Students may indeed offer an initial for their consistency and coherence. Operational defini- simple answer based on their experience, such as “hand- tions are furthermore put forth as the solution to “what edness is the dominant hand a person uses to write is” types of questions so that a person's intelligence, e.g., with, and it can be observed as ‘left,’ ‘ambidextrous.’ Innovative Teaching 3 2014, Volume 3, Article 3 Teaching OOM / J. W. Grice TABLE 1 Components of Phenomenalism and Moderate Realism Page Phenomenalism Moderate Realism 4 Hume's Causation Aristotle's Causality (Four Causes) 7 Means, Variances, Covariances Patterns of Observations 7 Aggregates Individuals 10 Effect Sizes Accuracy 10 Estimating Population Parameters Generalizing via Theory & Replication 10 Assumption-Laden p-values Randomization & Permutation Tests 16 Assumed Continuous Quantities Discrete or Continuous Quantities 16 Variables Entities and Qualities 16 Operational Definitions Natures of Things and their Qualities 22 Statistical Replication Systematic Model Building & Testing Note Page numbers refer to the sections in the manuscript in which the concept will be discussed or demonstrated via example analyses. or ‘right.’” Further inquiry, however, reveals that while low level in terms of atoms, or at the level of the chem- some people write exclusively with one hand, they may ical compounds, Adenine, Cytosine, Guanine, and Thy- brush their teeth, swing a ping-pong paddle, or throw a mine (ACGT). Speaking generically, a cause is that which ball with the other hand. Of course, a person who writes is necessary for an effect, and in this sense ACGT are the with the left hand may also swing a baseball bat or use necessary material causes of DNA. Of course DNA is per- a broom like a right-handed person. What if a person haps known best – or at least most readily recognizable – who writes with the left hand kicks a ball with the right through its double-helix structure. It is primarily the dis- foot? Does this matter in developing an understand- covery of this structure for which Watson and Crick were ing of handedness? Students should also be encour- awarded the Nobel Prize in 1962. Speaking of structure, aged to search for handedness questionnaires that are pattern, or shape incorporates Aristotle's formal cause in freely available on the Internet and to discuss the vari- the explanation of DNA. In a deeper sense, referred to as ous strengths and weaknesses of operational definitions. substantial form, formal cause may also refer to the “what- The goal of asking “what is” types of questions is to ness” of the thing, or the essence of the thing that makes it begin the move from the phenomenalism inherent in to be what it is rather than something else. modern research practices to a position of moderate real- Efficient cause will most likely be familiar to stu- ism. Table 1 presents a list of additional concepts that can dents in the form of the classic S R or S O R be compared and contrasted when making this shift in models or in the common variable-based models found perspective. The concepts are not presented in order of in published papers, like the one shown here: importance, and they will in turn be discussed in subse- quent sections of this paper. As indicated by the integers in Table 1 some concepts will be discussed and demon- strated together. From Causation to Causality This model presents the efficient cause (personal hap- To begin the move from phenomenalism to moderate piness) as a source of change or production that precedes realism, students must be taught to think more richly the effect (subjective happiness) in time. Although not rel- about causality. This will entail a review of Aristotle's four evant to material or formal cause, time is therefore criti- causes (material, formal, efficient, and final) discussed in cal when invoking efficient cause. Time is also relevant, his Physics (Book II, Chapter 3) and Metaphysics (particu- but not necessary, to understanding final cause, which larly Books I–VII). Although students should always be refers to a thing's end. When theorizing about persons, encouraged to read primary sources, a number of second- final cause can refer to the goals or purposes that explain ary sources may be referenced (e.g., Rychlak, 1988; Wal- behavior. For instance, a person enrolls in a psychology lace, 1996; Falcon, 2012). Fundamentally, students should class to learn more about himself or herself, or enrolls in understand that Aristotle sought to explain nature; con- a Ph.D. program with the design of becoming a professor sequently, when asking “what is” and “why” types of in the future. Final cause also entails natural end states questions the material, formal, efficient, and final causes as well that are important in explaining processes. For will be employed. For instance, a response to the question instance, the human body maintains a fairly steady over- “What is deoxyribonucleic acid (DNA)?” will refer to the all temperature. When factors in the environment warm matter of which it is made. The response may be at a very the body (efficient cause), such as when a Caucasian per- Innovative Teaching 4 2014, Volume 3, Article 3 Teaching OOM / J. W. Grice son sits in direct sunlight to deepen his tan (final cause), lock Holmes or Father Brown, requiring skills of careful the body will change in ways to release the added heat observation, patience, right reasoning, and intuition to (e.g., perspiration will increase; efficient cause), but it will solve the mysteries of nature (cf., Freedman, 1991). not release too much heat in moving toward its balanced A simple mental exercise that will help students realize point (final cause). Another example involves growth; for the distinction between Aristotle's causality and Hume's instance, red oaks normally grow to approximately 90 feet causation is to consider a scientist who develops a new whereas pinon pines normally grow to approximately 30 type of fertilizer for corn. The scientist works with a com- feet in height. These homoeostatic points or natural end puter program and with a molecular model kit to build states towards which things move or change are therefore models of the chemical compounds that will comprise the also entailed in Aristotle's final cause (Wallace, 1996). fertilizer. She then goes to the laboratory and manufactures Biochemical pathway models are perhaps the simplest the compounds and places a quantity of the fertilizer in a and most effective models to use when introducing stu- container. She writes instructions on the container detail- dents to Aristotle's four causes (e.g., an image of the Krebs ing how the fertilizer is to be added to the soil to insure cycle can be used: http://en.wikipedia.org/wiki/Citric_ maximum plant growth and health. Next, consider a stu- acid_cycle). The atoms in the model are the material causes, dent working in the scientist's laboratory. Does the stu- the structures of the different molecules in the model are the dent need to know the molecular formulas for the com- formal causes, the arrows in the model showing how com- pounds to test the fertilizer's efficacy on samples of corn pounds influence each other or change their patterns are crops? The answer is clearly, “no.” The student need only the efficient causes, and the rhythmic, stable nature of the follow the instructions on the container to deliver the fer- structures and processes in the model (viz., it is in a normal tilizer in appropriate proportions to the samples. The stu- “holding pattern”) is the final cause. A simplified model of dent does not need to know the formal and material causal an atom can also be discussed. The protons, neutrons, and structure of the compounds. As the scientist begins work- electrons are the material cause, the electrons moving about ing in her laboratory the causes exist intentionally in her in time in their orbits are efficient causes. Students can also mind. Once the fertilizer is created the causes inhere within imagine energy being used to change the orbits of the elec- it. The point is that causes are something more than sim- trons as an efficient cause. Formal cause is the “whatness” ple Humean associations of sense impressions in the scien- of the atom, it's pattern or configuration of matter that tist's mind, they are instead “wrapped up” in the fertilizer's makes it to be a particular atom. Lastly, the structures and nature. This is what was meant above in stating that causes processes in the atom are in balance. Again, students can are bound to the natures of the things themselves. imagine adding or subtracting energy to change the orbits Biochemical models, atomic models, computer models of the electrons, and in normal circumstances the electrons of the mind, and similar types of models used to under- will settle into a stable holding pattern (final cause). stand the structures and processes of nature are referred to Once students appreciate the rich explanations of generally as analogical models (Haig, 2013); and when such nature afforded by the four causes, they can apply them models are developed to be faithful representations of to psychological theories. A critical point must be made, specific natural systems (usually in visual form) they are however, either prior to or during this transition. The referred to as iconic models (Harré, 1976). In Observation point is this: causes are bound to the natures of the things Oriented Modeling, these visual or diagrammatic models themselves. An unfortunate turn in history is that psy- are referred to as integrated models to highlight the meta- chologists adopted a positivistic view of causation popu- physical position that nature is integrated and intelligible larly found in the writings of J. S. Mill and David Hume. (i.e., explainable; see Doroughty, 2013). Perhaps the most In this view, cause is reduced to efficient cause only, and challenging aspect of Observation Oriented Modeling, moreover causes are regarded as consistent associations and the most challenging prospect for future psychologists formed in the mind. In other words, the causal struc- as well, is the development of integrated (iconic) models. ture of nature itself cannot be known; rather, the consis- Examples can be found in Grice (2011) and Grice, Barrett, tent associations of sense impressions are all that can be Schlimgen, and Abramson (2012), and students should be known, and these consistently associated impressions encouraged to develop such models on their own or as are regarded as causation. This metaphysical standpoint part of a common class exercise. It is most effective, how- not only reduces Aristotle's causality to Hume's causa- ever, to build a model for which actual observations (data) tion, but it is highly subjective (viz., it fits with phenom- are available or can be obtained by the students. Students enalism) as well and serves as the impetus for viewing can collect data through an individual, approved project the search for causes as largely a statistical exercise, as or through a common class project. Existing data can be with mediation or structural equation modeling, or still provided by the instructor, requested from colleagues, worse solely as “equation surgery” (Pearl, 2009, p. 417). obtained from open sources, or taken from printed mate- From the perspective of moderate realism, however, the rial such as textbooks or instructional manuals. Integrated, search for causes is not necessarily statistical or math- iconic models are not widely used in psychology, hence ematical and is instead similar to the sleuthing of Sher- the goal is to build such a model for the data. Innovative Teaching 5 2014, Volume 3, Article 3 Teaching OOM / J. W. Grice An Exercise in Causal Modeling with delay values (estimated r = –.20).” Again, compare Zealure Holcomb's (1997) Real Data workbook is an in these statements carefully to the ways students will natu- stance of an accessible resource for students that re- rally think about the problem. When thinking about opti- ports numerous studies with actual data. One example mists and the time it takes them to report to the doctor the (p. 123) reports data for 135 women who visited a hos- students' natural focus is on the persons in the study, which is pital seeking evaluation for self-identified symptoms of proper for discussing causes that firstly inhere in the women breast cancer, such as a lump or discharge (see Lauver themselves, not in the variables and variable labels (e.g., & Youngran, 1995 for the original article). Among oth- “optimism”) constructed by the researchers. This statement er things, the goal of the study was to identify the rela- is of course in line with philosophical realism, and the capa- tionship between optimism and any delay in seeking bility of creating consistency between how students will the evaluation. The authors expected a negative linear think naturally about the world and how they will analyze relationship between the optimism and delay values as their observations is one of the main advantages of Obser- shown in the following variable-based model: vation Oriented Modeling and the OOM software. By focusing on the persons in the study, the integrated whole under consideration by the students is any given woman detecting an anomaly in her breast. The inte- grated model (a type of iconic model) therefore begins with a person, as shown in Fig. 1. In this case, a simple A negative relationship was expected partly because stick figure is drawn to represent an individual woman optimism has purportedly been shown to correlate posi- in the study. Every integrated model must begin with tively with better future planning and with positive atti- a judgment about the integrated whole or the system tudes of acceptance (Lauver & Youngran, 1995, p. 203). under investigation, whether it be a person, a baseball The optimism scores ranged from 0 to 4 and were team, another type of animal, an atom, a bio-chemical computed from responses to the original Life Orienta- process, etc. As shown in Fig. 1, a mind-body distinction tion Test, an 8-item self-report questionnaire. The women is made by denoting that the woman senses the lump in responded to statements such as “In uncertain times I her breast, presumably by using her hands and fingers. usually expect the best” and “I’m always optimistic about In her mind an abstraction is made on the basis of what is my future” using a 5-point rating scale. The numerical reported by the senses. This abstraction is denoted in the ratings on the scale were then averaged. The delay val- figure by the circle enclosing the word “lump” and a pic- ues were also reported by the women and ranged from ture of the lump. How abstraction occurs and whether 1 to 2,538 days (i.e., days between initial detection of the or not it includes a visual component is not spelled out symptom and the visit to the hospital). Over half of the by the model in its current form. The woman must, how- delay values were less than 21 days, and several extreme ever, know that her fingers have detected an internal cases were noted, which will be discussed below. lump rather than, for instance, an external skin tag. Students should be encouraged to write down their With the lump identified, the model next incorporates own thoughts and questions about the posited link optimism, and the question is raised, “What is optimism?” between optimism and delay in visiting a doctor. The On the one hand, it may be considered a quality inhering following types of questions may emerge: in the woman and may therefore be subject to observation “Are optimistic women necessarily better planners, by another person. On the other hand, the actual observa- and why isn't planning in the model?” tions relevant to optimism in the study are self-report. It “Once an optimistic woman has identified what she is more appropriate, therefore, to represent optimism as thinks is a cancerous lump, would she necessarily feel a an abstraction made by the woman. Similar to “this is a sense of panic and want to immediately see a doctor, or lump,” the abstraction is “this (myself) is a pessimist.” The would she be less worried?” problem with this part of the model, however, is that it “Wouldn't an optimistic woman actually be more does not fit the optimism observations which are numbers likely to judge the lump as non-cancerous and therefore computed by averaging values attached to a scale for judg- delay a visit to the doctor?” ments about the self (e.g., “I’m always optimistic about The last question would in fact lead to an opposite my future” rated on a scale with values from 0 to 4). With expected correlation, but the more general and important scores ranging from 0 to 4, is it presumed that optimism is point is that the students' questions will likely not fit any a continuum? Moreover, is it presumed that the woman variable-based model and Pearson correlation analysis. somehow erects a crude “optimism ruler” in her mind Why? Because to be perfectly consistent, models like the upon which she demarcates herself? How would such a one above only permit statements about variables, such as process actually occur? By contrast, why cannot one state- “Relatively high values on the optimism scale are expected ment, such as “Generally speaking, I consider myself to be to be associated with lower reported delay values,” or an optimist,” be used and code it as “Yes” or “No?” Why “Optimism scores are expected to be negatively correlated use a 5-point scale (0 to 4) rather than a 9-point scale? Does Innovative Teaching 6 2014, Volume 3, Article 3 Teaching OOM / J. W. Grice Lump Visit Optimistic Could be Doctor if then Cancerous Lump Lump is Pessimistic Probably Not else then Ignore Cancerous (P)articipant P Visits Doctor or Woman senses lump P Does Not Visit Doctor in her breast Fig. 1. Integrated model for optimism as a cause of planning a visit to the doctor. averaging items somehow improve the scores? Students than the simple variable-based model above. Asking stu- can be encouraged to ask additional questions about the dents to construct an integrated model will require them questionnaire in an attempt to determine what the opti- to think about the structures and processes under inves- mism values actually mean in the context of the nature of tigation; in other words, to think in terms of causality optimism itself. They will quickly realize that theoretical, rather than simply causation. not statistical, answers are required, and they will learn that Finally, outside of the woman's mind in the model, making relevant observations about optimism requires a whether or not she visited the doctor is observed. richer theoretical understanding of exactly what it is as a Again, to be truer to the observations in hand, the quality ostensibly inhering in persons. model needs to be adjusted so that the number of days Proceeding with the example, nonetheless, and pre- between detecting the anomaly and the first visit to suming that optimism is an abstraction drawn from the the doctor is the “output” from the internal features of sensory and intellectual “data” of one's self, it is logi- the model. Whether the structures and processes are cally related to judgments about the lump discovered in directly observable or not, the observations acquired the breast. If the woman forms an abstraction of herself must reflect some commitment to them, which is partly as optimistic, then she will judge the lump as cancerous; why this overall approach is referred to as Observation whereas if she forms an abstraction of herself as pessi- Oriented Modeling. mistic, then she will judge the lump as not cancerous. A judgment is distinguished from an abstraction by repre- From Statistics and Aggregates to Patterns and senting the former as a regular pentagon (see Fig. 1), and Individuals again exactly how the woman makes a judgment is not As Fig. 1 shows, the proper level of reasoning about spelled out by the model. Once either judgment is made, causes and effects is at the level of the individual women then it acts as an efficient cause (arrows in the model) for in the breast cancer study. If the causal model is accurate, a judgment regarding whether a visit to the doctor is war- then a woman who self-identifies as an optimist should ranted or the lump can be ignored. It can be seen in the not delay in seeking an evaluation from a doctor, and a model, however, that the lump is judged as “could be” or woman who self-identifies as a pessimist should delay “is probably not” cancerous. Are these the expected judg- seeking an evaluation. The actual data from this study ments? Would it be an important difference if the woman were obtained, however, under the phenomenalism (see judged the lump as “most certainly cancerous” or “abso- Table 1) inherent in the variable-based model shown lutely not” cancerous? Would such judgments be an effect above. Some ambiguity or difficulty must therefore be of optimism or some other judgment about oneself, such expected when attempting to analyze the data with the as fatalism? Students may also ponder if, as suggested OOM software (Grice, 2011). Nonetheless, students will above and contrary to the study authors' expectations, learn important lessons when they attempt to analyze the the pessimistic woman should actually be the one who data from the breast cancer study.3 judges the lump as cancerous and who rushes to make an appointment with her doctor; after all, bad things like 3 All of data files in both SPSS and OOM formats can be found on the Observation Oriented Modeling website: http://www.idiogrid. cancer are expected to happen. All of this is left open to com/OOM/InnovativeTeaching. In addition, instructional videos debate for the students, because the point is that the inte- can be found on the website for the examples discussed in this pa- grated model in Fig. 1 is much more rich and complex per. These videos demonstrate how the analyses are conducted and interpreted using the OOM software. Innovative Teaching 7 2014, Volume 3, Article 3 Teaching OOM / J. W. Grice An Initial Exercise in OOM Data Analysis goal is to build a model for explaining how the optimism In the variable-based model, optimism and delay were and delay observations are to be ordered to one another. assumed to be continuous quantities, hence a Pearson's The groupings into units here is entirely ad hoc, whereas in correlation was computed and reported by the study au- original research how the observations are ordered will be thors. The correlation was significant (r = –.18, p <.05, two- determined by an integrated model. tailed). It is also important to point out that the authors With the observations grouped into their respective transformed the delay responses due to radical skewness units, the next goal for the students is to devise a way and a number of salient outliers. The p value of course in- of ordering one set of observations to the other, and the dicates that, assuming the null hypothesis is true, and a simplest and most powerful way to introduce them to host of other assumptions have been met (including ran- this idea is through the Pattern Analysis – Crossed Obser- dom sampling, bivariate normality, and homoscedastici- vations procedure of the OOM software. By crossing the ty), the probability of obtaining results as extreme or more two orderings a matrix is created, as shown in Fig. 3. extreme than –.18 or.18 is less than.05. The conclusion to The question now is, if the optimism observations rep- be drawn in the standard null hypothesis significance test- resent the cause, and the delay observations represent ing procedure is therefore that the two assumed continu- the effect, how are the effect observations ordered to (or ous variables are linearly related in the population. The conformed to) the cause observations? In simpler terms, observed correlation serves as the estimated magnitude of what is the expected cause-effect pattern? Here the stu- the linear association, and at –.18 is rather anemic. dents are working visually rather than with equations, In the OOM software, students must first define the although simple equations could be used to determine a units of observation for the optimism and delay values. predicted pattern for discrete or continuous values. Fig- Rather than refer to optimism and delay as variables, ure 3a shows a pattern the students might suggest. The in OOM they are referred to as “orderings” to reflect the units are matched, in order, on a one-to-one basis with understanding that nature is integrated and ordered in par- units ostensibly representing high optimism matched ticular ways. The integrated model is supposed to reflect with units representing short delays in choosing to visit this ordering, both in terms of how the optimism and delay the doctor. Every woman in the study is expected to be observations are ordered or structured within themselves found in one of the grey squares denoted in the pattern. and how they are ordered to one another. As derived by The students can be further pressed to explain exactly the study authors, the optimism observations are ordered why the observations (all of the women) should fall into 33 units (0, 0.125, 0.250, 0.375,… 3.875, 4.00). Recall exactly in the grey units of the pattern. They may wish these values were computed by averaging responses on a to build some imprecision or “wiggle room” into the 0–4 scale to eight items on the Life Orientation Test ques- expected pattern, like that shown in Fig. 3b, or they may tionnaire. A simpler less precise ordering should be used to opt for a more radical dichotomizing as shown in Fig. make the task of building and testing a pattern, as described 3c. As debate ensues, students should be encouraged to below, more manageable for students. Twenty units work return to the integrated model, which will again require well, with scores ordered as 0–0.20, 0.21–0.40, 0.41–0.60,… them to take greater responsibility in thinking clearly 3.81–4.0. The delay observations can similarly be ordered and deeply about optimism itself (what it is, and how it into 20 units as shown in Fig. 2. The task here is similar must be observed) and exactly how it affects a decision to creating a grouped frequency histogram, but the overall to seek evaluation for an anomaly in the breast. Fig. 2. Delay and optimism observations grouped into 20 units. Each asterisk indicates two persons, and observed frequencies are reported in square brackets. Innovative Teaching 8 2014, Volume 3, Article 3 Teaching OOM / J. W. Grice Fig. 3. Expected patterns (darkened cells) for optimism and delay orderings (a–c), and actual observations for 135 women (d). Using the imprecise pattern in Fig. 3b, the OOM Classification Results software has an option for showing the observations Pairs of Observations Classified According to in the figure as well. As can be seen in Fig. 3d, only the Defined Pattern(s) about one quarter of the observations fit the expected Classifiable Pairs of Observations : 135 pattern. With such a simple and elegant visual tool the Correct Classifications : 33 students immediately realize using only an “eye test” Percent Correct Classifications : 24.44 that the prediction is a bust. Of the 135 women, only Randomization Results 33 observations (24.44%) were located in the expected Observed Percent Correct Classified : 24.44 crossed units. Without any equations, without any p Number of Randomized Trials : 1000 values, without any statistical assumptions, and with- Minimum Random Percent Correct : 13.33 out any attempt to conjure an imaginary population, Maximum Random Percent Correct : 34.07 Values >= Observed Percent Correct : 355 the students are also here reminded of the importance Model c-value : 0.35 of accuracy in science. The current cause-effect pattern is clearly inaccurate, and since the pattern is derived The Percent Correct Classification (PCC) index, as from the integrated model developed by the students, noted, is only 24.44%, and this is the primary numeric the integrated model is deemed inaccurate as well. This result to be considered. The PCC index is computed is the primary question to be answered in Observation or derived for most all of the analyses available in the Oriented Modeling: Is the model accurate? As an inten- OOM software, and students should be encouraged to tional representation of the causes and effects inhering look for it first in the program's output. As can also be in the women in the current example study, the model seen, a probability statistic can be requested and used is largely a failure. in an entirely secondary role. This probability statistic is Beyond the simple and elegant graph, the OOM referred to as a chance value, or c-value, in OOM in order software provides summary information regarding the to avoid confusion with the p value commonly encoun- accuracy of the model. tered in null hypothesis significance testing. The c-value Innovative Teaching 9 2014, Volume 3, Article 3 Teaching OOM / J. W. Grice is in most instances in OOM derived from a randomiza- the OOM software students can explicitly identify the tion test, which is becoming more widely recognized 17 women whose observations fit the expected pattern. by quantitative experts as superior to traditional p val- The readily interpretable PCC index can also be com- ues (see Manly, 2006; Howell, 2007, Chapter 18) despite pared to the correlation value in this example to remind being lauded as early as 1969 (Winch & Campbell). students that “statistical significance” does not indicate The c-value for the breast cancer data is computed practical, clinical, or theoretical significance (Thompson, by randomly pairing the optimism observations with 2002), nor does statistical significance indicate that even the delay observations. Specifically, the optimism val- a simple majority of observations fit an hypothesized ues for the 135 women are randomly shuffled. The pre- model. Lastly, students can contrast this rich exercise dicted pattern is then applied to the randomly paired of thinking about what the scores on the Life Orienta- optimism and delay observations and the PCC index tion Test really mean and how they are causally related computed. If this PCC index equals or exceeds the PCC to observations of delay, with the relatively sterile exer- index for the actual data (here, 24.44), then randomized cise of checking statistical assumptions and interpreting data performed just as well as the actual data in the con- the magnitude of the observed correlation coefficient text of the model, which is not a desirable occurrence. according to some arbitrary convention (e.g., “according This process of randomizing the data and comparing to Cohen's conventions, a correlation of –.18 represents PCC indices is repeated a set number of times (1000 a small effect size”). trials in the output above) and the results tallied. The c-value is the proportion of instances in which the PCC From Effect Sizes, p-values, and Parameters to index from the randomized data equaled or exceeded Accuracy, c-values, and Chance the PCC index for the actual data. As with the tradi- From the perspective of Observation Oriented Model- tional p value, then, a low value is desirable, although ing, the word “effect” has been hijacked by phenom- no rational person would put forth or adopt a univer- enalism (see Table 1), or more specifically by statisti- sal convention to determine “significance” (e.g., c <.05). cism (Lamiell, 2013). Because psychology has primarily The c-value is entirely secondary, whereas the inte- adopted statistics, particularly null hypothesis signifi- grated model and PCC index are primary. The c-value cance testing, as its main approach to working with in the shown output for the breast cancer data is.35, quantities (discrete or continuous), the word “effect” indicating that for 355 of the 1000 trials, a PCC index of has taken on an esoteric meaning not generally under- 24.44 or higher could be achieved by simply randomly stood by scientists, philosophers, or the general pub- shuffling the data. Not only is the PCC value of 24.44 lic. The word and its derivatives are of course power- unimpressive, but it is fairly ordinary or usual as well. ful and influential, as can be seen in statements such as: Lastly, in the move from aggregates to persons, stu- “The effect of the SAD light in combating depression dents can be asked to compare their interpretation of the was significant,” “The effectiveness of the psychoana- PCC index to the Pearson's correlation coefficient. Recall lytic treatment was negligible,” “The bystander effect is the correlation between optimism and delay for the breast reliable,” “This effect has been observed across numer- cancer data was –.18. Students can be challenged to ous studies,” and “The size of the effect was rather interpret the correlation coefficient in a way that can be large.” These statements convey a sense of importance, applied to the 135 women or to any given woman in the certitude, and scientific understanding, even though no study. The correlation can be squared as well to convert details of the studies from which they may have come it to a proportional statistic. Again, students can be chal- are provided. The meaning of effect in modern psychol- lenged to explain how accounting for 3% of the variance ogy, however, typically has very little to do with certi- in one variable by the second variable applies to the 135 tude and is instead almost always embedded in a prob- women or any given woman in the study. The point of abilistic or statistical point of view. asking students to interpret the correlation in this way An introductory class exercise regarding the mean- is of course to remind them that their interpretation ing of the word “effect” requires students to write must be confined to the variables; i.e., the variances and about any event in their lives and then explain why covariances of the variables. There is simply no clear the event happened. Students may respond: “My car way to cross the bridge from the aggregate statistic (the wouldn't start today because the battery was dead,” Pearson's r) to the scores, or observations, themselves in “I felt sick last week because I contracted the flu from a way that conveys the meaningfulness of the result for my brother,” “My girlfriend and I broke up because these women. By contrast, the PCC index is tied directly we discovered we have little in common.” Normally, to the observations and how they are ordered, both in the students' statements will attribute a cause to each terms of their units and in terms of the expected pat- event and will express an understanding of nature that tern. Whether or not any given woman's observations seems to run deep while also transcending the particu- are consistent with the integrated model and pattern lar events of their lives. Such thoughts express the way analysis can be determined. Indeed, using options in things truly are…the way nature is. Cars with dead Innovative Teaching 10 2014, Volume 3, Article 3 Teaching OOM / J. W. Grice batteries don't start, people who contract the influ- popular conventions and in the expected direction (i.e., enza virus grow ill, and uncommon people cannot the mean for the Therapy group will be greater than the stay romantically tied for life. But are these statements mean for the Control group); yet, when examined at the true? Do they really express the way nature is? Can- level of the individuals, most anorexic women would not an old car without electronics be physically pushed actually keep their existing weight or lose weight, con- and started by popping the clutch? Aren't some people trary to prediction. The altered data are reported in immune to certain strains of influenza? With respect Table 2, and the exercise to be performed by students to romantic relationships, is it not also true that some- is to analyze the data using the traditional null hypoth- times “opposites attract?” Clearly, gaining an under- esis significance testing approach and the OOM soft- standing of nature will require more than the students’ ware. day-to-day informal thoughts of causes and effects. Weight is a continuous quantity, and the observa- Still, each question raised implies that an answer might tions are independent both between and within groups. be found, thus bringing students to the doorstep of sci- An independent samples t test is therefore suitable for ence; for as Aristotle understood well, the business of these data, and the hypotheses can be written in three- science is causal explanation. valued logic form (Harris, 1997), With a statement such as “The effect of the SAD light in combating depression was significant,” the psy- H 0 : µ therapy = µ control chologist is also intending to express a causal relation; H A1 : µ therapy > µ control < − predicted namely, the SAD light causes a reduction in depression. Such statements are the “stuff” of science, but in psy- H A2 : µ therapy < µ control. chology these causal-sounding expressions are almost invariably statistical expressions. While students may The μ's of course represent population weights in naturally pair effects to their causes in the class exercise pounds. Students will find that the Therapy group described above, in psychology the opposite of “effect” gained an average of 1.35 pounds (SD = 3.72) compared is not typically “cause” but rather “null statistical dif- to the Control group who lost an average of 1.23 pounds ference.” In other words, an effect is said to be found (SD = 3.25), thus supporting the predicted difference when the null hypothesis has been rejected; in other the (95%CI = 0.77 ≤ μT – μC ≤ 4.38) and efficacy of the thera- words, an effect is a statistically significant finding. The py. The t test is statistically significant (t58 = 2.86, p =.006), magnitude of the effect or the size of the effect moreover at the.05 level, and the magnitude of the effect (d = 0.74, often refers to the average difference between groups or η2 = 0.12) is nearly large according to Cohen's widely the proportion of variance shared by two variables. A used conventions (d = 0.80 is a large effect). Examina- useful exercise to drive home these points is to again tion of the data also reveals no salient outliers, but the rely on published data, contrived data, or data from distributions appear to be somewhat bimodal, particu- workbooks or textbooks. These data can be used “as is” larly for the therapy group, when examined with his- or manipulated in a way to shed light on what is meant tograms. Boxplots, however, will show only moderate by a statistical effect. skewness. Students should be required to list and evaluate or An Exercise on Effect Sizes in the OOM Software discuss the various assumptions of the independent In his chapter on t tests, David C. Howell (2007) reports an samples t test: (1) random sampling or random assign- example data set of weight gain (or loss) for anorexic girls ment to groups, (2) continuous dependent variable, (3) who received therapy for their eating disorder and those null hypothesis is true, (4) p ≤.05 is a reasonable cut- who did not receive therapy. The outcome variable is the point for statistical significance, (5) normal population amount of weight gain, in pounds, from the beginning distributions, (6) observations are independent both to the end of the treatment or therapy. In the parlance of within and between groups, and (7) homogeneity of modern research design, the grouping variable (Therapy population variances. Many of these assumptions of vs Control) is the independent variable and cause, while course underlie the validity of the observed p value, a weight gain is the dependent variable and effect. Using a probability the students should be expected to under- standard variable-based model, the relationship between stand completely. An excellent resource for checking the two variables can be shown as follows: students' understanding of the p value is Oakes' (1986; see also, Gigerenzer, 2004) 6-item quiz. In the context of discussing the p value students should also be chal- lenged to define the populations under consideration. Are they all women? Are they women from certain ethnic groups or regions? Are they women of certain Howell's original data were altered here so that the ages? Are they women now living, or do the popula- computed effect size would be medium according to tions include women from the past and future? The Innovative Teaching 11 2014, Volume 3, Article 3 Teaching OOM / J. W. Grice TABLE 2 Weight Gain Data, Example Data, and Randomized Data For Control and Anorexia Therapy Groups Control Group Therapy Group Weight Gain Clear One Clear Two Random Weight Gain Clear One Clear Two Random −7.5 −7.5 −7.5 3.8 −3.2 3.2 −7.0 −4.1 −7.0 −7.0 −7.0 −7.0 −3.1 3.1 −7.4 −4.5 −5.7 −5.7 −5.7 0.0 −2.5 2.5 4.0 0.0 −5.1 −5.1 −5.1 6.2 −2.4 2.4 2.4 6.4 −4.5 −4.5 −4.5 7.0 −2.2 6.2 −6.2 −5.1 −4.1 −4.1 −4.1 −0.2 −2.0 5.0 5.0 3.2 −3.8 −3.8 −3.8 3.0 −2.0 7.0 −7.0 −5.7 −3.8 −3.8 −3.8 −0.3 −2.0 4.0 4.0 −7.5 −3.7 −3.7 −3.7 5.5 −1.9 5.0 5.0 2.2 −3.4 −3.4 −3.4 −3.8 −1.9 2.0 2.0 3.4 −3.1 −3.1 −3.1 2.4 −1.9 2.0 2.0 5.5 −3.0 −3.0 −3.0 5.9 −1.8 3.8 3.8 2.0 −2.5 −2.5 −2.5 4.1 −1.4 2.2 2.2 −3.0 −2.2 −2.2 −2.2 4.8 −1.3 2.2 2.2 2.0 −1.8 −1.8 −1.8 6.1 −0.2 3.0 3.0 4.0 0.3 −0.3 −0.3 −3.7 −0.1 4.1 4.1 2.0 0.8 −0.3 −0.3 −2.2 1.7 2.0 2.0 −2.5 0.2 −0.2 −0.2 0.0 2.3 3.4 3.4 0.0 0.2 0.0 0.0 0.0 4.1 4.1 4.1 −1.8 0.5 0.0 0.0 6.1 4.1 6.1 −6.1 7.2 1.0 0.0 0.0 7.5 4.2 4.2 4.2 −0.3 1.0 0.0 0.0 4.2 4.8 4.8 4.8 −3.1 1.1 0.0 0.0 3.1 5.1 5.1 5.1 −3.4 1.3 0.0 0.0 4.1 5.5 5.5 5.5 −0.8 2.0 0.0 0.0 2.2 5.5 5.5 5.5 0.0 2.1 0.0 0.0 −0.5 5.9 5.9 5.9 0.0 3.5 0.0 −6.0 5.0 6.1 6.1 −6.1 0.0 3.6 −0.5 −0.5 −1.0 6.4 6.4 −6.4 0.0 3.9 −0.8 −0.8 2.5 7.5 7.5 −7.5 5.0 2.9 −1.0 −1.0 −3.8 7.2 7.2 −7.2 5.1 point of this challenge is to remind students that psy- Xtherapy − Xcontrol 1.35 − ( −1.23 ) chologists are almost always working with arbitrarily d= = = 0.74. 2 Spooled 12.117 defined, imaginary populations. The population means are therefore almost always non-empirical; i.e., they are The formula incorporates aggregate statistics and values that cannot be obtained in actuality. The essen- consequently has no direct bearing on any one of the tial lesson to be learned from discussing assumptions women in the study or on any pair of women from the and populations is that null hypothesis significance two groups. As has been made abundantly clear above, testing is an extremely abstract, mathematical-statisti- however, causes and their effects inhere in the things cal routine in which the primary goal is to pursue (esti- or entities under investigation – in this instance, the mate) population parameters that rarely have any basis women in the two groups. Students should be chal- in lived reality. lenged to relate the value of d to the women in the Turning to effect size, by declaring the t test “sta- study. Another popular index of effect size is η2, which tistically significant” and rejecting the null hypothesis, the students should compute and again attempt to the conclusion is that the population means differ by relate to the women in the study: η2 = t2/(t2 + df) = 2.862/ some magnitude. Cohen's d represents a standardized (2.862 + 58) = 0.12. This task will prove even more diffi- estimate of that magnitude based on the sample means cult as the interpretation of η2 is one based on shared and pooled standard deviation. For the current data the overlap between variables. The value of 0.12 indicates value is computed as, that the independent and dependent variables share Innovative Teaching 12 2014, Volume 3, Article 3 Teaching OOM / J. W. Grice 12% of their variance. Conveying what this effect size 2, showing clear patterns. Consider 60 weight observa- index means for the actual effectiveness of the ther- tions, for instance, in which all of the women in therapy apy for the individual women in the study is impos- gain two or more pounds while all women in the con- sible, which is why psychologists eschew the problem trol group lose weight or maintain their current weight by using Cohen's arbitrary conventions to describe their (column labeled “Clear One” in Table 2). The resulting effects as small, medium, or large. multigram is shown in Fig. 4. As can be seen, a multi- By contrast, analyzing the same data in the OOM gram graphs the two frequency distributions side by software will remind students that causes inhere in the side, and in this extreme example the two distributions persons in the study, and that any discussion of effects do not overlap at all. A clear pattern is present in which must be relevant to these persons. The analysis begins the weight values can be separated into two groups. The by first defining the units of observation. The women Percent Correct Classification (PCC) index for these data are observed to be in one of two groups: the Therapy is 100%, and the pattern clearly supports the effective- group or the Control group. These two units of obser- ness of the therapy. Every woman in the therapy group vation represent the cause, or they at least carry infor- gained weight, and moreover gained more weight than mation about the causal forces effecting weight gain or every woman in the control group. loss (in Observation Oriented Modeling, an integrated As another extreme example, consider data (“Clear model must eventually be worked out to truly under- Two” in Table 2) that generate the multigram in Fig. 5. stand the causes). The changes in weight are continuous Again, the 8-unit weight observations can be classified quantities representing a change in this quality (heavi- into the 2-unit group orderings with remarkable accu- ness) over time. The values in Table 2 range from –7.5 racy (PCC = 96.67%). The pattern in the multigram shows to 7.5 pounds for the two groups of women, and most that: (1) all of the women who gained 2 or more pounds of the women have unique values. In OOM, continuous were in the Therapy group; (2) all who gained up to 1.9 quantities must often be grouped so that each unit (val- pounds or lost as much as 6 pounds were in the Control ue) is represented by more than one observation. Be- group; and (3) most of the women who lost 6.1 or more cause a population parameter is not being estimated, pounds were in the Therapy group, contrary to expecta- the notion of statistical power is meaningless in OOM. tion. Based on the relative frequencies both within the 8 Observations can consequently be grouped without fear weight units and the 2 group units, the algorithm deter- of losing power. While modern methodologists, for in- mined that all of the observations should have fit this stance, advise strongly against dichotomizing data (Mac- pattern and were therefore classified as such. As can be Callum, Zhang, Preacher, & Rucker, 2002; Irwin & Mc- seen in Fig. 5, then, two observations (viz., two Control Clelland, 2003), such alterations are welcome in OOM if women who lost 6.1 or more pounds) were inconsis- they advance the goal of identifying clear and meaning- tent with this overall pattern and were hence counted ful patterns in the observations. The weights for the two groups of women were thus organized into 8 units (–8.0 to –6.1, –6.0 to –4.1,… 4.0 to 5.9, 6.0 to 8.1). Students can be encouraged to try different groupings after the initial analysis and explore how they affect the results, as will be shown below. The Build/Test Model option in the OOM software is then used to attempt to bring the 8-unit weight ordered observations into conformity with the 2-unit group ordered observations using binary Procrustes rota- tion, a procedure that does not rely on means or covari- ances but is instead based on the observations them- selves (see Grice, 2011, Chapter 3). The analysis is ad hoc compared to the a priori pattern defining methods used with the breast cancer data above. Specifically, the algo- rithm examines the relative magnitudes of frequencies of observations and determines how every observation should be classified based on the predominant pattern in the data. Each observation is then evaluated according to this pattern and judged as either correctly or incorrectly classified. The results can be presented in a simple graph referred to as a “multigram.” To help students interpret Fig. 4. Multigram of clear pattern example. This pattern matches multigrams, instructors should prepare at least two con- expectation with the individuals in the Therapy group gaining trived sets of observations, like those reported in Table weight. Innovative Teaching 13 2014, Volume 3, Article 3 Teaching OOM / J. W. Grice OOM software, making certain the students randomize a large number of the 60 values across both groups. In this way, some weights for the women in the Therapy group will be given to women in the Control group and vice versa. Once the weights are randomly shuffled, the students run the binary Procrustes rotation and examine the multigram and PCC index. Fig. 6 shows an exam- ple multigram from the randomized data (labeled “Ran- dom”) in Table 2. The clear pattern in Fig. 4 has now been lost, and the PCC index for the randomized data, 61.67, is much smaller than the PCC index for the “Clear One” data (100%). The randomization test in OOM sim- ply repeats this process a set number of times (e.g., 1000 trials) and determines the proportion of trials in which the PCC index equals or exceeds the original value. This proportion is the chance value, or c-value, and for the data in Figs. 4 and 5 it is less than.001, indicating that not one time in 1000 trials did the randomized data yield Fig. 5. Multigram of clear pattern example. This pattern does PCC indices as high as 100 or 96.67, respectively. A low not match expectation perfectly, as nine women in the Ther- c-value indicates that randomized versions of the obser- apy group lost weight. These nine women were classified as correct by the ad hoc algorithm used in the analysis because vations do not readily produce a pattern as clear or dis- they were distinct from the other observations. criminating as the one obtained for the actual data. It informs the students of the distinctiveness or unusual- as misclassified by the algorithm even though they were ness of the observed PCC index and accompanying pat- in the Control group and lost relatively large amounts of tern, and it does so without any assumptions. Recall weight. This example thus demonstrates that the algo- from above the list of assumptions necessary for the rithm works on the pattern of observations (data), not accuracy of the p value for the independent samples t on the researcher's predicted pattern of results as in the test (e.g., normality of population distributions, homo- breast cancer study above. Nine women in the Therapy geneous population variances, independence of obser- group lost 6.1 or more pounds, which is entirely con- vations). None of these assumptions are required for the trary to expectation; yet, the algorithm counted them randomization test (Manly, 2006), and students will con- as correctly classified. Only two women in the Control sequently find the c-value to be concrete, simple, and group lost this much weight, and all of the other women intuitive in comparison to the traditional p value. in the Therapy group lost more weight than all of the Turning students to the OOM results for the actual women in the Control group. Based on the clear sepa- weight data in Table 2, the multigram in Fig. 7 reveals that ration between groups of observations, the algorithm a single discrimination point between the two frequency determined that all women who lost 6.1 pounds or more distributions cannot be made. Nonetheless, a pattern of should be classified as belonging to the Therapy group, bimodal, non-overlapping units is evident in the mul- which unfortunately contradicts expectation. The anal- tigram, permitting a large number of the observations ysis is entirely ad hoc, however, making such outcomes to be correctly and distinctively classified, PCC = 86.67, possible. A high PCC index in the Build/Test Model analy- c-value <.001. The OOM results are as follows: sis therefore only indicates that the observations formed a clear pattern. It does not mean that the pattern fits the Classification Results scientist's expectation. The multigram must be examined Conforming (Effect) Observations Classified to – preferably in light of an integrated model – to decide Target (Cause) Observations whether the pattern is consistent with expectation or Classifiable Observations : 60 theory, and students will quickly realize that interpret- Ambiguous Classifications : 0 Correct Classifications : 52 ing the effects (and causes) embodied in a multigram is Percent Correct Classifications : 86.67 clearly different from computing and interpreting statis- Randomization Results tical effects embodied in d and η2. Observed Percent Correct The extreme multigrams and their accompanying Classifications : 86.67 data in Table 2 can also be used to help students under- Number of Randomized Trials : 1000 stand the chance value. Specifically, each student can Minimum Random Percent Correct : 28.33 be asked to shuffle randomly the weight change val- Maximum Random Percent Correct : 78.33 Values >= Observed Percent Correct : 0 ues (“Clear One” in Table 2) that generated the mul- Model c-value :less than (1/1000); tigram in Fig. 4. Values can simply be changed in the that is, < 0.001 Innovative Teaching 14 2014, Volume 3, Article 3 Teaching OOM / J. W. Grice Fig. 6. Multigram of randomized data with no clear pattern. Fig. 7. Multigram of weight gain data for Control and Therapy The OOM software has detected a pattern that classifies groups. This pattern partly matches expectation, but 11 women an overwhelming majority of the women correctly based in the anorexia Therapy group lost weight and were classified on the observations themselves…but what is the mean- correctly by the ad hoc algorithm because of their distinctiveness. ing of the result? Because population parameters are not estimated, the three-valued logic hypotheses above are Effect size indices accompanying results for most not relevant. Without an integrated model to guide their modern statistical analyses are understood to be aggre- thinking, students must examine the multigram in Fig. 7 gate-based or variable-based values that are difficult and interpret the pattern. As can be seen, it appears the to interpret without the aid of established conven- effect of the therapy is dichotomous. Sixteen women have tions, like Cohen's conventions. As such, they are not lost weight, which is a terrible outcome given they are effects that are logically tied to the causes that inhere anorexic, while 14 women have gained weight, which is in the people in the study. Effect sizes are statistical, a positive outcome. The Control group as well appears to aggregate summaries of the data, and their magnitudes be made up of two groups, those who lost approximately have no meaning for any given individual in the study. 1 to 8 pounds and those who gained approximately 1 to Through this particular class exercise, students realize 4 pounds. Cohen's d and η2 offered no information about that OOM analyses are not based on aggregate statis- these patterns, and to speak of d as indicating a “typi- tics. Not a single mean, median, or standard deviation cal” standardized difference between women in the two has been invoked. The output across analyses is also groups is recognized as misleading. A majority of women highly similar, allowing students to focus on the pri- in the Therapy group actually lost weight, contradicting mary information; viz., the patterns and PCC indices. the t test conclusion supportive of the efficacy of the ther- This streamlining is possible because OOM reduces apy – a disaster for the anorexia therapy. If the weight observations are represented as two units (–0.1 to –8.0, weight loss; 0.1 to 8.0, weight gain), then the meaning of the results for the women in the study becomes even more clear. As can be seen in the multigram in Fig. 8, the two groups of women appear highly similar with respect to the 2-unit weight observations; and again, a small majority of women in the anorexia therapy group actually lost weight. These 16 women in the Therapy group were therefore classified correctly by the algorithm, a result entirely opposite of expectation. This analysis also shows, however, no ability to discriminate clearly between the women in terms of simple gain or loss in weight, as indicated by a PCC index of 51.67 and c-value of 1. In every Fig. 8. Multigram of weight gain data for Control and Ther- instance, randomized versions of the data yielded a PCC apy groups. Weight gain has been ordered into two units, and index of 51.67 or higher, indicating that the pattern in Fig. 8, the pattern is opposite of expectation with a slight majority although opposite of expectation, is not at all distinct. of women in the anorexia Therapy group having lost weight. Innovative Teaching 15 2014, Volume 3, Article 3 Teaching OOM / J. W. Grice all data to a common binary form, referred to as deep An Exercise in “Measurement” structure, upon which the analyses are based. More- Following modern psychometric theory, students are over, the goal in OOM is not to estimate abstract popu- instructed at the beginning of this exercise that Need lation parameters, but to accurately classify the obser- for Speed is a continuous latent variable that cannot be vations based on some a priori or ad hoc pattern (ideally, measured without error. More or less time can be spent of course, an integrated model would be available for on discussing the modern conceptualization of latent explaining the pattern). Accuracy is therefore the key variables (e.g., see Bollen, 2002; Borsboom, 2008), but judgment, and the PCC index is readily understood by the pragmatic point is that multiple items must be con- students, other scientists, and laypersons as well. The structed from which scores (values) can be obtained. c-value in OOM is also free of assumptions and rela- These scores are expected to vary from person to per- tively concrete compared to the traditional p value in son for two reasons: (1) variation due to true individ- null hypothesis significance testing, and is based on a ual differences in Need for Speed, and (2) variation due firm foundation of reasoning dating to at least the 1960s to unknown factors (errors). The latter errors are often (Winch & Campbell, 1969). Nonetheless, the inference undifferentiated and expected to be normally distrib- to cause and effect is only aided by a high PCC index uted and independent. This is of course the simple clas- and low c-value. The basis and force of such an infer- sical true score model of psychometrics. ence ultimately rest upon the structures and processes Eight items are written to include in the question- in an integrated model. naire, as shown in Fig. 9. Data are obtained from rat- ings on a Likert-type scale, i.e., arbitrarily numbered From Continuities and Variables to Entities with values from 0 (highly disagree) to 6 (highly agree). and Their Qualities The fictitious data for this exercise are reported in the With regard to measurement, one of the three domains Appendix. Students next conduct a standard item anal- of quantitative training reviewed by Aiken, et al. (2008), ysis, examining the inter-item correlations, corrected students of psychology are universally taught to mem- item-total correlations, and Cronbach's α. The results orize a