E&O_lecture2_DAGS_2021.pptx
Document Details
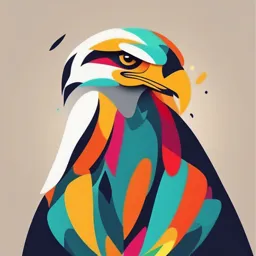
Uploaded by OverjoyedActionPainting
2021
Tags
Full Transcript
Exposure and outcome measurement in epidemiology Class 2 – slide 1 M A S T E R E P I D E M I O LO GY UNIVERSITY OF ANTWERP A N N E L I E S VA N R I E Class 2 Directed acyclic graphs Class 2 Reducing our complex...
Exposure and outcome measurement in epidemiology Class 2 – slide 1 M A S T E R E P I D E M I O LO GY UNIVERSITY OF ANTWERP A N N E L I E S VA N R I E Class 2 Directed acyclic graphs Class 2 Reducing our complex reality 1. Conceptual framework A conceptual framework is an analytical tool used to make conceptual distinctions and organize ideas Class 2 Reducing our complex reality 2. Mathematical model Simply said….the primary purpose of a mathematical model is to reduce the complex reality to a set of parameters and mathematical equations to predict population-level outcomes Class 2 Reducing our complex reality 3. Directed acyclic graphs A representation of causal relations among variables. Used to inform statistical analysis Research question: does watching TV cause obesity? Class 2 Reducing our complex reality The art is to reduce it “just right” Class 2 Directed acyclic graph or DAG A DAG is a directed acyclic graph ◦ Directed: arrows – temporality – causality instead of association ◦ Acyclic: no variable can cause itself ◦ Graph: a graphic representation of our thinking about causality DAGs are used to encode a priori assumptions about relationships between variables. ◦ “A DAG illustrates what is known about the topic, expresses causal assumptions and outlines the statistical implications of these assumptions”. (Sauer & Vanderweele). DAGs push investigators to “think before you start” ◦ At time of study design: which variables to measure ◦ Before starting the analysis: which variables to include in the model Investigators often do not agree on a single representation of a complex clinical or public health question. In that case, they will not agree on a single DAG. Statistical analyses can be undertaken as informed by different DAGs, and the results compared. Class 2 Example OUTCOME EXPOSURE Chronic obstructive Coffee drinking pulmonary disease (COPD) Research question: What is the effect of coffee drinking smoking on COPD in the population of interest? Tendency to use Public health relevance: stimulants Should we promote reduction in coffee drinking to reduce the burden of COPD? Tendency to use stimulants is an unmeasured variable Class 2 Example OUTCOME EXPOSURE X Chronic obstructive Coffee drinking pulmonary disease (COPD) Biological plausibility: Coffee contains molecule X, smoking which causes pulmonary vasoconstriction Tendency to use stimulants Class 2 Marginal and conditional statistical independence Marginal relation between exposure and outcome: no other variables are taken into account ◦ If exposure and outcome are marginally independent, then then information on exposure does not tell you anything about the outcome Conditional relation between exposure and outcome: examination of the relationship between exposure and outcome within levels of a third variable ◦ Conditioning happens when ◦ restriction in study design ◦ matching by study design ◦ loss to follow up during study ◦ stratified analysis ◦ adjusting for covariates in regression modeling ◦ If two variables are conditionally independent, then, within the level of the third variable, information on exposure does not tell you anything about the outcome Class 2 Example Research question: Does coffee drinking affect COPD in the study population? Is there a statistical association between coffee drinking and COPD? Does the marginal distribution of COPD differ across strata of coffee drinking? Does the distribution of COPD differ across strata of coffee drinking conditional on a level of tendency to use stimulants? In other words, when knowing the tendency of use of stimulants, does Tendency to knowledge of coffee drinking matter use with regards to COPD? stimulants Class 2 Components of DAG Arrow between nodes (variables) indicate a causal relationship A missing arrow (absence of arrow) suggests there is a strong believe that there is no causal relationship between the two variables If you are not sure, the conservative choice is to put the arrow Class 2 The Acyclic in DAG Acyclic = no feedback loops - you cannot get back to a variable by following a series of arrows Acyclic: given the concept of causality: if X causes Y, Y cannot cause X Class 2 The directed in DAG This DAG states that: 1. We assume there is a causal relationship between smoking and COPD 2. we are rather “certain” there is no causal relationship between smoking and drinking coffee, i.e. coffee does not make you smoke and smoking does not make Note: classic approach: Tendency to you drink coffee smoking is a use confounder stimulants Class 2 Nomenclature: variables X= exposure Y X Y = disease/outcome M = measured variable M U = unmeasured variable U Class 2 Nomenclature: relationships Parent = variable that affects another X Y variable Child = variable that is affected by another variable M Ancestors = all upstream variables Descendants = all downstream variables U Y has two parents (X, U) X and one child (Z) U is a parent of M X has one child (Y) and Y is a child of X Y Z three descendant (U, Z, U Y) U and M are ancestors of Y Z has one parent (Y) and three ancestors M and Y are (X,U, Y) descendants of U Class 2 Nomenclature: paths A path is a sequence of edges starting from X and ending in Y, regardless of the direction of the arrows X Y X Y M M U U Causal, directed Undirected, backdoor path path Class 2 Nomenclature: Causal path An arrow or sequence of arrows constitutes a directed or causal path Any variable along a causal path is an intermediate variable Smoking is an intermediate variable on the causal path from tendency to use stimulants to COPD Tendency to use stimulants Class 2 Nomenclature: collider A collider is a variable that is a common effect of 2 other variables on a given path It is called a collider because 2 arrowheads from the parents “collide” at the child A directed or causal path can never contain a collider Class 2 Constructing a DAG: step 1-3 Step 1: Start with the exposure outcome association Class 2 Constructing a DAG: step 1-3 Step 2: Add variables affecting X and variables affecting Y Tendency to use stimulants Class 2 Constructing a DAG: step 1-3 Step 3: Add variables on the causal pathways Tissue damage Tendency to use stimulants Class 2 Constructing a DAG This DAG states that omitting lung inflammation in an incorrect representation of our current Tissue knowledge of how damage Lung smoking causes COPD inflammation Tendency to use stimulants Class 2 Summary – for now… DAGs are a graphic tool that reduces the complexity of our reality and makes explicit our knowledge/beliefs on the relationships regarding variables relevant to our exposure- outcome research question DAGs are directed to highlight (possible) causal relationships Directed edges (arrows) reflect temporality ~ causality Absence of a directed edge between two variables states that there is no effect of the one variable on the other variable No cyclic relationships, i.e. something cannot cause something else that then again causes the first variable DAGs are used to help to define qualitative statements about statistical (in)dependence between exposure and outcome of interest Class 2 d-separation rules: linking a priori knowledge to statistical analysis 1. X and Y can be d-separated unconditionally (marginally) If X and Y are d-separated, then X and Y are not associated If every path between X and Y is closed, then X and Y are marginally independent Pr (Y=y|X=x) = Pr (Y=y) 2. X and Y can be d-connected and become d- separated by conditioning on other variables Note: These rules will only be correct if the DAG was constructed correctly Class 2 Open and blocked paths A path can be open (unblocked) or closed (blocked) A path that contains a collider is always closed; i.e. blocked by the collider A causal path can never contain a collider Two variables are d-separated if there is no open path between them Two variables are d-connected if there is an open path between them Class 2 Backdoor paths, unblocked paths A backdoor path is an undirected path that goes from the outcome to the exposure (“back from Y to X”) All open backdoor paths are biasing paths. Any backdoor path that is open (unblocked) results in an association between X and Y Backdoor paths can be blocked by conditioning on variables – only need a single block on the path Class 2 Conditioning on a variable on the causal pathway Conditioning on an intermediate between X and Y blocks the path between X and Y One should thus never condition on a variable on the causal pathway between exposure and disease Class 2 Example OUTCOME EXPOSURE Molecul Chronic obstructive Coffee drinking eX pulmonary disease (COPD) smoking Tendency to use stimulants Class 2 DAGs and confounding Statistical concept of a confounder : a variable that is - Associated with the exposure - A risk factor for the outcome - Not on the causal pathway DAG concept of confounding: - There is potential confounding of a potential causal relationship between the exposure and the outcome when a backdoor path is open. - A path contains a variable that is a common cause of exposure and outcome - The path is not blocked Class 2 Conditioning on a confounder variable A confounder is a parent of both exposure and outcome. Conditioning (adjusting) on a confounder blocks (closes) the path, removes C as a source of association between X and Y ,and results in an unbiased (adjusted) association between exposure and outcome Exposure and outcome can be marginally associated but conditionally independent (conditioning on C) Class 2 Example Conditioning on smoking closes the backdoor path from COPD to coffee drinking If this DAG is correct and we find an association between coffee drinking and COPD after conditioning on smoking, then the association between coffee drinking and COPD is unbiased. Tendency to use stimulants Class 2 Conditioning on an indirect (surrogate) measure of a confounder Z Adjusting for an indirect measure will reduce bias Class 2 – slide 18 but some residual X Y confounding may exist if the surrogate is imperfect U M Class 2 Conditioning on a collider A collider is a descendent of both exposure and disease, a shared effect of X and Y Conditioning on a collider opens the path between its parents and induces an association between exposure and outcome In the presence of a collider, exposure and outcome can be marginally independent but conditionally dependent (conditional on conditioning on C) Class 2 Stratification bias is a type of collider bias Research question: do beta blockers result in heart Smoki attacks? ng Any open backdoor (i.e β- Heart biasing) paths? blocker attack Two: Eating 1. Through smoking cookies Death 2. Through eating cookies Path through smoking can be blocked by conditioning on smoking Class 2 Stratification bias = collider bias What if we condition on Smoki death? ng β- Heart blocker attack Eating By stratifying on death (for Death cookies example in a retrospective study excluding people who died), eating cookies becomes a source of confounding Class 2 Another collider example: the M in DAGs Research question: does low education affect a child’s diabetes status? Would you find it logical that we W include mother’s diabetes status as Mother’s a potential confounder in the diabetes statitsical model? status X Y Low Child diabetes education status Class 2 Another collider example: the M in DAGs Research question: does low education Family Mother’s affect a child’s incom genetic diabetes status? The path from X to Y is e diabetes risk closed at the collider W W Mother’s There is thus no open diabetes backdoor path between X status and Y What happens if we condition on the X Y mother’s diabetes Low Child diabetes status (even if the stats education status do not show it is associated with Class 2 exposure or outcome…) The M in DAGs Conditioning on W opens the path and thus Family Mother’s creates an association incom genetic between X and Y e diabetes risk We can solve this by W also conditioning on Mother’s mother’s genetic diabetes status diabetes risk But now you have over- adjusted (where it was not X Y necessary) resulting Low Child diabetes potentially in loss of education status precision of the effect estimate Class 2 Conditioning on a descendant of a collider Even if you condition on a descendant of a collider, you induce an association between a exposure and outcome The farther away the variable is we condition on, the weaker the association will be we induce between outcome and disease Class 2 Importance of ancestors C 4 C1 is a confounder C1 is a also a collider C 3 C 1 Adjustment for C1 results in opening a backdoor path from X to Y via C2, C3 and C4 C 2 X Y Class 2 Importance of ancestors C1 is a confounder C 4 C1 is a also a collider Adjustment for C1 results in opening C 3 C a backdoor path from X to Y via C2, 1 C3 and C4 Adjustment for C1 and C2 or C3 or C4 results in the adjusted (unbiased) association between exposure and C 2 X Y outcome Class 2 Another example of importance of ancestors : the M-plus in a DAG Question of interest is the association between exposure E and outcome D C is a “traditional” confounder for the effect of E on D But C is a also a collider Adjustment for C results in opening a path from A to D and opens backdoor path from E to D Class 2 Another example of the importance of ancestors : the M- plus in a DAG The adjustment for C, thus also requires adjustment for A or B to result in the adjusted association between exposure and outcome Note that if there was no effect of C on E and D, then no adjustment would be the best option as adjusting for C and A or B would be “overadjustment” that could lead to measurement error. Class 2 Summary: conditioning on a variable reverses its status on the path Open Blocked path path M M M M M M M M Class 2 Summary The assessment of the existence of backdoor paths allows you to assess for the presence of bias We focus more on paths than on variables Colliders are path specific. On a given DAG, a variable can be a collider on one path and a mediator or confounder on another path In a DAG, both the parents and “first degree” ancestors of the parents are crucial Class 2 DAG rules: d-separation If X precedes Y temporally, then, without conditioning, there are two possible sources of association between X and Y: 1. The causal path: the path of interest from X to Y 2. The backdoor path(s): common ancestors of X and Y, which introduce bias If the DAG is correctly constructed, and X and Y are d-separated by a set of variables S (no open backdoor paths), then X and Y will be conditionally independent, given S d-separation thus separates the upstream effects (causes of X) from the downstream effects (effect of X on Y). I.e. Conditioning on the upstream effects (S) or “holding S constant” renders the downstream effects (effects of X an Y) independent of the upstream effects. I.e. d-separation results in conditional independencies If, after conditioning on S, there is evidence of an association between X and Y, then there must be a causal association between X and Y Class 2 DAGs and their use 1. Identify selection bias 2. Identify the minimal set of covariates to be measured 3. Assess mediation 4. …. Class 2 DAG and identification of selection bias Selection bias occurs when the risk for the outcome in the study population is different from the risk in the target population This can happen: ◦ by design: study participants are not representative for the domain - healthy-worker bias, volunteer bias,… ◦ Due to loss to follow up Evaluation of selection bias in a DAG is done by representing the “selection” as a variable and drawing edges to visualize whether the selection is associated with the exposure, outcome or their causes (whether shared or not) Class 2 DAG and identification of selection bias RCT of the effect of drug A on outcome Y S represents loss to follow up C (alcohol abuse) is not associated with exposure A given randomization into the RCT ‘Conditioning” on LTFU opens a backdoor path from Y to A trough C Thus, conditional on S, we expect exposure and outcome to be associated even if exposure does not affect the outcome So even for an RCT, it is useful to draw a DAG ! Class 2 Which variables to measure and include in the analysis: minimally sufficient set S A sufficient set S is a set of variables that, when conditioned on, closes all backdoor paths between X and Y A sufficient set is minimally sufficient if removing any variable from S results in open backdoor paths Investigators often want to adjust for more variables than what is minimally sufficient. This can be problematic because this can ◦ reduce precision ◦ increases cost of the study ◦ result in bias ◦ For example, controlling for a larger set that now contains a collider Class 2 DAG and Mediation Facial Inflammator Incident injury y response temporomandib ular disorder Research question: what is the effect of facial injury on incident temporomanidibular disorder not mediated through inflammatory response? i.e. what is the direct causal effect of facial injury on temporomandibular disorder? Class 2 DAG and Mediation Facial Inflammator Incident injury y response temporomandib ular disorder If this DAG is correct, then adjusting for inflammatory response will allow one to assess the direct causal effect of facial injury on temporomandibular disorder Class 2 DAG and Mediation If the DAG includes knowledge on the subject, it becomes more complex… Inflammator Incident Facial y response temporomandib injury To estimate the direct ular disorder effect of facial injury on temporomandibular disorder, the analysis has gende to include gender as a r confounder Psychologi cal distress Class 2 DAG and Mediation Inflammator Incident Facial y response temporomandib injury ular disorder What if we then stratify gende by inflammatory r response, i..e include Psychologi inflammatory response as cal distress a mediator? Class 2 DAG and Mediation Inflammator Incident Facial y response temporomandib injury Because inflammatory ular disorder response was a collider, this opens a backdoor path from TMD to facial injury through psychosocial distress gende Psychologi r cal distress Class 2 What does this example teach us 1. For the same exposure-outcome association, inclusion of a variable can increase bias in one analysis and reduce bias in another analysis. 2. To decide which variables one wants to measure during a study, one needs to decide a priori what the specific aims of the study are and the corresponding hypotheses and planned analyses 3. Conditioning on mediators, common practice in epidemiology, can have unintended consequences Class 2 DAG and selection of variables to include in the statistical regression model DAGs indicate which paths are confounding the association between X and Y (backdoor paths) and as such, identifies which variables should be included in the regression model to estimate the statistically independent (unbiased) effect of exposure on outcome. DAGS are non-parametric. They make no assumption about the functional form of the relationship between variables. The form of the model could be linear, U shaped,… Class 2 Constructing a DAG = the hard work 1. Identify your important health outcome and a modifiable exposure 2. Draw the causal path(s) from exposure to outcome 3. Identify from the literature which other factors cause the outcome 4. Review literature about variables that can be related to the outcome 5. Brainstorm about possible missing variables related to the outcomes 6. Review literature about variables related to the exposure 7. Brainstorm about possible missing variables related to exposure 8. Any missing common ancestors, i.e. common causes of two variables in the DAG? 9. Any associations between the common ancestor added and variables already in the DAG? Constructing the DAG pushes you to think about your research question – before you start Use DAGityy online - https://www.youtube.com/watch?v=pJhU4fimHBQ& Class 2 Constructing a DAG = the hard work Reality is complex. Simplification is often a necessary step in science; DAGs are useful in that they make these often unstated simplifications explicit so that their implications can be evaluated. Similar to all models, a DAG is a simplifying tool rather than as a comprehensive representation of reality To be plausible, a DAG must be based on a priori knowledge of the exposure- outcome association. This must be based on subject matter expertise, most often obtained from across a range of scientific disciplines to fully evaluate the a priori knowledge related to a research question. Drawing a DAG before data collection and again before analysis ensures that any underlying assumptions guiding the research question are made explicit. Class 2 Algorithm for identifying confounders 1. Erase all directed edges originating form the exposure (including edge to Y) 2. Identify all unblocked backdoor paths (paths back to X) 3. Define S, your sufficient set of variables you need to adjust for confounding. S may be the smallest set or the set that is most feasible to measure 4. Draw an edge to connect all pairs of variables with a child in S or a child with a descendant in S 5. Identify any new unblocked backdoor paths 6. Update S 7. Explore choices and consider how these affect S Class 2 Tools DAGityy online - https://www.youtube.com/watch?v=pJhU4fimHBQ& Can be used to make the graphic construction and identify S The R package ‘dagitty’, that can be used to: - evaluate whether a DAG is consistent with the dataset it is intended to represent - enumerate ‘statistically equivalent’ but causally different DAGs - identify exposure outcome adjustment sets that are valid for causally different but statistically equivalent DAGs. Example: social epidemiology Research question: what is the effect of neighborhood violence on the risk of incident cardiovascular disease? Incident Neighborho cardiovascula od violence r disease Example : social epidemiology Research question: what is the effect of neighborhood violence on the risk of incident cardiovascular income Physical diseases? activity What do we know? Incident Neighborho cardiovascula od violence r disease Any important ancestors? Example : social epidemiology Research question: what Race/ ethnicity is the effect of neighborhood violence on the risk of incident income Physical activity cardiovascular diseases? Incident Any important Neighborho cardiovascula od violence ancestors? r disease Example : social epidemiology Research question: what is Race/ the effect of neighborhood ethnicity violence on the risk of incident cardiovascular income Physical diseases? activity What are the paths between exposure Incident Neighborho cardiovascula and outcome? od violence r disease Example : social epidemiology Race/ What are the paths ethnicity between exposure and outcome? income Physical activity Two causal paths - The direct path from Incident Neighborho violence to CVD od violence cardiovascula - The path mediated by r disease physical activity Three backdoor (biasing) paths Example : social epidemiology Are the mediating paths? Race/ ethnicity Are there confounding income Physical activity (backdoor) paths? Are there colliders? Incident Neighborho cardiovascula od violence r disease Example : social epidemiology Race/ Physical activity is a ethnicity mediator of the direct effect of violence on income Physical activity CVD Income, race/ethnicity and physical activity are Neighborho Incident cardiovascula potential confounders (at od violence r disease least on 1 path) Physical activity is a collider (at least on 1 path) Example : social epidemiology Race/ What is the minimally ethnicity sufficient set S? income Physical activity 1. Delete all arrows leaving exposure Incident Neighborho cardiovascula od violence r disease Example : social epidemiology Race/ What is the minimally ethnicity sufficient set S? income Physical activity 1. Delete all arrows leaving exposure Incident Neighborho cardiovascula 2. Identify the od violence r disease unblocked backdoor paths from X to Y Example : social epidemiology Race/ What is the minimally ethnicity sufficient set S? income Physical activity 2. Identify the unblocked backdoor paths from X to Y Neighborho Incident cardiovascula od violence 3. Block the backdoor r disease paths Example : social epidemiology Race/ What is the minimally ethnicity sufficient set S? income Physical activity Conditioning on income is not Neighborho Incident sufficient as it leaves cardiovascula od violence r disease a backdoor path through race/ ethnicity open Example : social epidemiology Race/ What is the minimally ethnicity sufficient set S? income Physical activity The combination of income and Incident race/ethnicity is a Neighborho cardiovascula od violence minimally sufficient r disease set of variables Example : social epidemiology Race/ Is income and ethnicity Race/ethnicity the only possible minimally income Physical sufficient set S? activity Which of the two Incident possible sets S would Neighborho cardiovascula od violence you choose to r disease measure? - Income and race/ethnicity - Income and physical activity? Example : social epidemiology Race/ Wat if our DAG was ethnicity wrong? What if you forgot about income Physical important ancestors? activity Incident Neighborho cardiovascula od violence r disease District zoning law Access to healthy food Example : social epidemiology Race/ Does this change our ethnicity definition of S? income Physical activity Incident Neighborho cardiovascula od violence r disease District zoning law Access to healthy food Example : social epidemiology Race/ Does this change our ethnicity definition of S? income Physical activity Only conditioning on income is still not sufficient Neighborho Incident cardiovascula od violence r disease District zoning law Access to healthy food Example : social epidemiology Race/ ethnicity Conditioning on income and race/ethnicity is income Physical also not sufficient (and activity neither is conditioning on income and physical Incident activity) Neighborho cardiovascula od violence r disease District zoning law Access to healthy food What does this example teach us? Invest in the right study design Know your topic well Do not work in isolation and consult the experts Otherwise you end up by regretting not measuring a variable (at minimal cost) and have to include in your limitations : “our lack of knowledge of access to healthy food could have resulted in a biased estimate of the association between neighborhood violence and incident CVD” Example : social epidemiology What if we forgot an Race/ important ancestor of ethnicity the outcome? occupatio n income Physical activity Incident Neighborho cardiovascula od violence r disease Example : social epidemiology What if we forgot an Race/ important ancestor of ethnicity the outcome? occupatio n income Physical Research question: activity direct effect of violence on CVD Incident What happens Neighborho cardiovascula when we condition od violence r disease on income? Example : social epidemiology Research question: Race/ direct effect of violence ethnicity on CVD occupatio n Conditioning on income Physical activity income does not close all backdoor paths Neighborho Incident cardiovascula What does od violence r disease stratifying on the mediator do? Example : social epidemiology Research question: Race/ direct effect of violence ethnicity on CVD occupatio n Conditioning on income Physical activity income does not close all backdoor paths Neighborho Incident cardiovascula What does od violence r disease stratifying on the mediator do? What does this example teach us? Carefully consider the ancestors of both exposure and outcome One more thing Now that we know which variables we should measure with minimal error, should we be concerned about who we enroll into the study? Urban Family history of residence CVD Study participati on Incident Neighborho cardiovascula od violence r disease One more thing What happens if we condition on study participation? Urban Family history of residence CVD Study participati on Incident Neighborho cardiovascula od violence r disease One more thing If people living close to the academic study site and those who have a relative with a family history of CVD are more likely to participate, we create selection bias Urban Family history of residence CVD Study participati on Incident Neighborho cardiovascula od violence r disease References Brian Sauer, Tyler J. VanderWeele. Use of Directed Acyclic Graphs Scott Vennon – YouTube video’s Kenneth Rothman, Sander Greenland and Timothy Lash. Modern epidemiology. Chapter 12 Textor et al. Robust causal inference using directed acyclic graphs: the R package ‘dagitty’. International Journal of Epidemiology, 2017 Akinkugbe et al. Directed Acyclic Graphs for Oral Disease Research. Journal of Dental Research 2016, Vol. 95(8) 853–859 Fleischer and Diez Roux. Using directed acyclic graphs to guide analyses of neighborhood health effects: an introduction. J Epidemiol Community Health 2008; 62:842-846 Class 2 – slide 25