Ecological Methods Theory Univariate Analysis PDF
Document Details
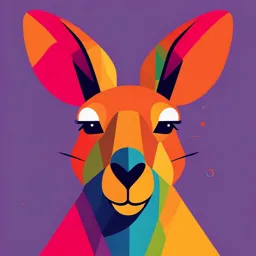
Uploaded by RenownedCuboFuturism
Wageningen University & Research
Tags
Summary
This document appears to be an introduction to ecological methods, covering univariate analysis. Basics of statistics, including standard deviation, are detailed. It also describes different types of statistical tests and distributions, along with relevant formulas and graphs.
Full Transcript
Ecological Methods: Theory WEEK 1: Group differences Day 1: Introduction Learning outcomes: Formulate appropriate null hypotheses Outline the possibilities, limitations and constraints of the different univariate (e.g., t-test,....) and multivariate statistical tests (e.g., PCA....),...
Ecological Methods: Theory WEEK 1: Group differences Day 1: Introduction Learning outcomes: Formulate appropriate null hypotheses Outline the possibilities, limitations and constraints of the different univariate (e.g., t-test,....) and multivariate statistical tests (e.g., PCA....), and able to identify alternative solutions Select the best statistical tool to test the ecological data at hand Analyze ecological data using appropriate statistical procedures Interpret the statistical results in an ecologically meaningful sense Perform both univariate and multivariate analyses Exams: Week 3 -> Univariate analysis Week 4 -> Multivariate analysis Week 5 -> Applied statistics Basics of statistics: A standard deviation (or σ) = measure of how dispersed the data is in relation to the mean Mean and standard deviation stabilize with increasing sample size Between 1 time σ: 68% of the data Between 2 times σ: 95% of the data Between 3 times σ: 95% of the data 3 levels of measurements: Nominal → identifies categories: habitat, sex, color, species Ordinal → identifies an order: Abundant, Frequent, Occasional, Rare Scale (ratio scale): Absolute zero (weight, length, intake, growth….) Subtract, add, multiplication, division Distribution types: Normal distribution symmetric & continuous Lognormal distribution skewed & continuous exponential growth, biomass, concentrations Poisson distribution & skewed not continuous: Negative Binomial discrete, counts, e.g., distribution quadrats Binomial distribution two outcomes: dead/alive present/absent smoking: y/n not continuous, discrete Standard error → indicates how different the population mean is likely to be from a sample mean → Confidence interval Standard deviation vs. confidence interval The standard deviations = mean plus and minus 1 standard deviation (68% of the data fall within this interval) Random + large sample size = SD will not change with increasing N The 95% confidence interval tells you something about how certain you are about the mean → larger N = more certain (confident) about the mean Illustrate a measure of the distribution of your Illustrate whether one mean is significantly differen data set from another mean → Show graph with standard deviation → Show graph with 95% confidence interval or standard error → Impossible to draw safe conclusion based on graphs Stabilizing mean with increasing sample size It is suggested to sample at least 40 waterbucks The mean generally stabilizes with increasing sample size, you become more confident about the mean when you have a larger sample Stabilizing standard deviation with increasing sample size In the beginning, when you have only few samples, your estimated SD might (due to the variation in your sample) be somewhat higher or lower, but after a while, when you increase your sample size, the estimated SD stabilizes Decreasing confidence interval with increasing sample size → Frequency graph How to interpret a frequency graph? Day 2: T-tests T-test = statistical test used to compared the means of two different groups Introduction Hypothesis = Body weight of Grysbok is larger in area B than area A, because of the higher food quality in area B Prediction = Body weight is larger in area B than area A Hypothesis = TESTABLE explanation of an observation → not just an educated guess about what you think will happen → must be testable & well-justified! → Clear direction: larger/smaller, decrease/increase Based on: 1-your observations, 2-what you already know to be true → underlying mechanisms, processes, logic,... Good hypothesis worded like: If I fertilize an area (=X) then the grysbok will have a If …, then…, because … larger body weight (=Y), because of the higher food quality intake. 2 variable types: Independent variable: cause (X) H1 = alternative hypothesis = prediction Dependent variable: effect (Y) H0 = there is no difference between the areas X→Y Equal variances (s² is the same) Unequal variances (s² is different) t-test for unequal variances t-value (threshold value) always associated with a p-value (probability) P = probability of rejecting H0 when H0 is true p = 0.219, thus the probability that the difference is caused by chance is around 22% t-test for equal variances Degrees of freedom Degrees of freedom = number of independent variables that can be estimated in a statistical analysis → d.f. always smaller than N ????? What is the critical t-value? What is the calculated t-value? How to report in scientific paper? Mention used test Statistical parameter value Degrees of freedom P-value 1) Normality test → Shapiro test 2) Variance test → Levene’s test for testing equality of variances 3) t-test → t-test P > 0,05 → var. equal = TRUE (in t-test) → EQUAL variances P < 0,05 → var. equal = FALSE (in t-test)→ UNEQUAL variances One-sided / two-sided testing Type 1 and Type 2 error t-test for paired data Paired data → each grysbok is measured twice, two measurements per individual Power and sample size For example: Error bars overlap so maybe insignificant → t-test stronger than visual inspection = Type 2 error! We say there is no significance but in reality, there is Smaller standard deviation = higher certainty Bigger difference between two means = reach conclusion sooner BUT all depends on sample size Decision: minimum sample size (calculation with GPower): s.d’s increases, then you need a larger sample size!! s.d1 and s.d2 are similar & X1 and X2 are close: difficult to find significant effect Your balance (= method) is not measuring well and accuracy is low (accuracy is low, e.g. 60% = 0.6) → need a larger sample size Use P < 0.0001 & not P < 0.05 to be absolutely sure + use a larger sample size Standard deviation to show variation of all the data around the mean However, testing for differences between two means also depends on the sample size that you have. In those cases where the bar graph is intended to only show the differences among the means, often a bar graph with the SE is depicted or the 95% confidence intervals Indeed, the last bar graph with the 95% confidence intervals suggests that there are significant differences in total weight between the two species (no overlap) In general, Cohen’s d is interpreted as: if d = 0.2, then the effect size is small, values around 0.5 indicate a medium effect size, and if d ≥ 0.8 the effect size is large. How large is the Cohen’s d in your calculation? Using 2000 samples in such a t-test means that the power of your test will be very high, even when the effect sizes reduces to e.g. only 0.2. Still you would be able to detect a significant difference between the number of bites with and without dogs. Such a reduction in effect size can result from two causes: 1) the means become closer, or 2) the standard deviations become larger Day 3: Transformation and non-parametric tests If the data do not follow a normal distribution around the mean these SDs, SEs and 95% CLs are wrong and can not be used Data transformation Normality test → Shapiro test How to choose which transformation? → Always transform the whole variable! + add number to avoid taking log of zero Sometimes impossible to transform → non-parametric test Non-parametric tests (medians) Mann-Whitney U test = test of medians Example: beetle abundance in 2 areas H0 = the two medians are equal Our test variable (U) should be < critical value → there is a statistically significant difference between the medians (Mann Whitney U -test, U=8.5, N1= 8 and N2=7, Pwilcox.test (Pair(massw2,massw3)~1, data=nestlingmass) Calculated: V=47, P critical F-value? So P < 0.05? The mean mass of starlings is significantly different among the 3 countries (Anova, F2,33 = 13.503, P < 0.05 ) Multiple comparison test (= Post-Hoc tests) Power of multiple comparison tests ranked high to low: 1) Least Significant Difference (LSD) A priori Ha! → very low chance type II error 2) Tukey test 3) Student Newman Keuls (NKS) 4) Duncan’s Multiple Range test 5) (Holm-)Bonferroni p/N° combinations 6) Scheffé’s test (very conservative) Differences between all these tests? Example Tukey test Tukey test was made as an addition to ANOVA, developed to do after ANOVA Groups have to be the same length for the ANOVA test T = (q) x √(within variance/N) = 4.3 (threshold) H0 = there is no difference in body weight between the two countries If outcome > threshold → ? q → q-table for number of samples and d.f. = 3.82 within variance → SS/df = MS (see Anova table, 12.66) N = number of samples at each location (= 12) = Anova table CONCLUSION: More than 2 groups? → 5 phases Day 5: Two Way Anova Univariate test = there is only 1 dependent variable, but there can be multiple independent variable? Anova = Analysis of Variances 2-factor Anova Total variance = variance within + variance between Total MS = MS within + MS between (=MS sex + MS season + MS interaction) In F-table for P < 0.01 → critical F-value = 8.5310 (what does this mean?) Multiple R-squared vs. Adjusted R-squared? Lineair model (LM) GLM = General Lineair Model Model → procedure calculates expected values (= mean) R² = proportion of explained variance = relative measure of fit of the model or spread data around the mean R² adjusted → corrects for df (best parameter?) 0 ≤ R² adjusted ≤ 1 Multiple comparison tests: Least significant Difference (LSD) (N equal!) A priori Ha! Tukey-test Student Newman Keuls (SNK) Duncan’s Multiple Range test Scheffé’s test What is an interaction? → Does the effect of the one variable (sex) depends on the other variable (season)? NO INTERACTION YES INTERACTION Multiple comparison (more than 1 factor) Test for significant interaction → make a new variable with unique codes for each factor combination, and carry out a Multiple Comparison Test on those new groups Factorial design More than 2 factors Anova: Starling mass: sex, country, season Spp. richness,: vegetation type, temperature, rainfall Food intake: vegetation type, grass biomass, time of day Etc.... More than 1 factor clustered bargraph/boxplot → each factor different color Solution → full factorial design or nested design (?) → You need data of all combinations! And ideally with equal N Powerful: you can separate all individual and interactive effects Residuals and normality Normality test for each unique combination of: Sex (N = 2) Country (N = 4) Season (N = 2) = 2 x 4 x 2 = 16 classes → normality test for all 16 classes… → Increased chance of a Type I error Solution = analysis of normality of the residuals = one test for all classes General approach The partial η2 = relative measure of the proportion of variation that can be explained by a certain variable. The partial η2 & the power of the test increases with an increase in the difference between the means (i.e. a larger gap between the means), and decreases with an increase in the variation of the data (such as an increase in SD). ↑ difference between means = ↑ power & partial η2 ↑ variation of data (↑ in SD)= ↓ power & partial η2 The larger the sample size, the more likely significant effects will be found. So: statistical significance (and the power) depends on the sample size and on the difference between the means. The partial η2 is independent of sample size. The p-value is the probability that you falsely reject your null-hypothesis (there is some criticism about this formulation, and some scientists do not agree, see these references: link 1, link 2, link 3, link 4, or link 5, but within the framework of the course, we will maintain this definition). p-values can be misused, especially for larger sample sizes. You should also study and report the effect sizes. Another solution to decrease the effect of large sample sizes is to use “bootstrapping”, i.e. taking random smaller samples and use these p-values and effect sizes. Interpretation p-values → Type I error, i.e., the probability that you falsely reject your null hypothesis The power of a test = probability that a test correctly rejects the null hypothesis. Power = 0.86 → if you repeat the experiment 100 times, 86 times you would correctly reject the null hypothesis. Commonly used → calculate the effect size for each independent variable Effect size = proportion of variation in the dependent variable that is explained by each of the independent variables Partial eta squared = proportion of variation that is explained by the model Difference R² and partial η² → can be calculated for each of the variables in the model separately Partial eta squared is better than normal eta squared → it corrects for multiple independent variables in a model p-value depends on the sample size → larger sample size → smaller p-value → rejection null hypothesis Common perception is now that p-values should be reported together with their effect sizes → reader can decide Effect size → contains information about the magnitude of the difference between two groups (such as e.g., in a t-test) or the relationship between two variables Measures to quantify effect size → partial η2 and Cohen’s d Cohen’s d using the standard deviation partial η2 providing a measure of the variance explained by a variable WEEK 2: Trend analyses Relationship with two or more variables Day 6: Chi square & correlation Introduction Why do we need replicates? → to cover the variation → t-test to test whether the mean differs Different kind of data → 7 birds in Finland & 7 birds in the Netherlands → 14 different birds instead of two measurements per bird for NL and Finland (previous lectures) Nominal variable → there is no order, just classes (black, brown) Ordinal variable → there is an order (small, large) Null hypotheses: No difference between two groups (week 1) No relationship with two or more variables (week 2) Type of data: Distribution Scale - Ordinal - Nominal Chi-square test Chi-square test (X²) → compare observed vs. expected value (ratios) → for group differences and trend analysis based on Chi-square distribution How does it work? Works for counts (frequencies) = exam question? No percentages or fractions? → transform to counts! Remember for test!!!! H0 = observed = expected values Degrees of freedom = (#rows-1)(#colums-1) Expected ratio = 3:1 = 75%/25% H0 = observed = expected values Compare outcome with Chi-square table: If X² > than critical value, then p < 0.05 → rejection H0 If X² < than critical value, then p > 0.05 → no rejection H0, there is no difference between two countries → observed = expected Transformation to counts, how does it work? Degrees of freedom: df = (#r – 1)(#c – 1) = (2 – 1)(4 – 1) = 3 → → So the ratio’s for Veluwe and Finland are DIFFERENT! Summary Chi-square test: Different questions can lead to different expectations Think carefully about your expected values (how to calculate?) Always observations against expectations: do the data match expected ratio? Null hypothesis: observations and expectations are the same Rule of thumb: each expected value > 5 Correlation Correlation = relation between two variables Null hypothesis = there is no relationship Trend analysis → not looking at difference between groups but relationship between variables!! When are we testing for a causal relationship? → experimental setup, only change 1 condition Causation: X changes because of Y Correlation: similar trend but Y does not necessarily cause X? Correlation does NOT imply causation Example of doctors and the plague → area with a lot of infected people have the most doctors BUT doctors are not causing spread of the plaque, they are travelling to areas where most people are infected to help the people Spurious correlation = occurs when two variables appear causally related to one another but are not For example: divorce rate and consumption of margarine follow same trend but are NOT related Correlation coefficient r = measure for relation between two variables Between -1 (negative correlation) and 1 (positive correlation) Correlation coefficient r = 0, no relation Correlation coefficient r ≠ 0, there is a relation but is it significant? Significance of correlation: 0 < r < 0.3 → weak correlation 0.3 < r < 0.6 → moderate correlation 0.6 < r < 1 → strong correlation Pearson correlation coefficient rp Spearman rank correlation coefficient rs Parametric test Non-parametric test Normally distributed data Can always be used: also on not-normal Linear relationship between variables distributed data Only transform one variable when one does For relationship between ranks not follow a normal distribution df = total number of pairs (aantal blokjes in df = total number of pairs (aantal blokjes in tabel) of observations - 2 tabel) of observations - 2 sr = Standard error of r p > 0.05 → no significant correlation Tied data: not a problem (e.g. two times 11) Day 7: Regression I (lineair) Basics of regression Regression = relationship between 2 or more variables → (Multiple) independent variable(s) affect 1 dependent variable (not more than 1) Causality is assumed, not tested! Difference with correlation? → only 2 variables Example: How environmental variables affect one particular species Strength of relation: Less variability, P 0.05 → datapoints are spread Shape of relation: Lineair Polynomial Parabolic … Direction of relation: Positive or negative effect Large of small effect (steepness of slope) Regression model → Based on locations that have been measured Linear model → Simplest regression model Y = dependent variable X = independent variable Residuals = unexplained variation Minimize residuals!! (datapoints close to the line) Residuals → normally distributed Normality test to check If not → transform dependent variable Then calculate residuals again b0 and b1 estimated based on observations Using the least-square method → sum (observed-expected)² over all observations → minimise sum Calculations Sum of squares (SS) to minimalize residuals Variation explained by regression → Regression SS Remaining variation → Residual SS ANOVA calculation Regression line always passes through turning point (= mean of x, mean of y) In regression, we compare the slope of our data with a zero slope (x = 0, y = mean) Red = Remaining variation (Residual SS) Blue = Total variation (Total SS) Explained variation (Regression SS) = Total SS - Residual SS F-ratio and ANOVA output for regression 𝑚𝑒𝑎𝑛 𝑜𝑓 𝑠𝑞𝑢𝑎𝑟𝑒𝑠 (𝑀𝑆) 𝑅𝑒𝑔𝑟𝑒𝑠𝑠𝑖𝑜𝑛 𝐹= 𝑚𝑒𝑎𝑛 𝑜𝑓 𝑠𝑞𝑢𝑎𝑟𝑒𝑠 (𝑀𝑆) 𝑅𝑒𝑠𝑖𝑑𝑢𝑎𝑙𝑠 Significant regression: MSreg > MSres Non-significant regression: MSreg < MSres Large value for F = low P value Low value for F = high P value Regression model: Null hypothesis = no effect of independent variable on dependent variable = Regression coefficient b1 = zero → slope is horizontal Using t- or F-value to test Fraction of variation explained: adjusted R square Example: relation between size Daphnia and predator density Null hypothesis = there is no effect of predator density on size Null hypothesis = intercept equals 0 (b0 = 0) → graph goes through source Density of predators has a positive effect on the size of daphnia (linear regression, df=11, t=13.386, p 2, then Random factor = significant Normality & equal variances → assumed, not tested because too complex Advantage of including random factors: Makes the analysis more sensitive for the main experimental factors N young Used a lot in research, sites often differ from each other Site as random factor → not independent (?) Latin square design Is the factor fixed or random? Research-driven factor = fixed Question to ask yourself: If experiment repeated, would you use exactly the same setup? (similar sites/ similar river distance, etc)? Yes → fixed factor No, does not matter → random factor Multiple comparison tests NEVER carry out a posthoc test on: Random factors → do not compare them Interaction with a random factor → Because you have no testable hypothesis Single factor: P ≤ 0.05 (significant interaction) → use EMMeans (?) Make new variable with unique codes for each factor combination Carry out multiple comparison test on those new groups LMM: fixed + random effects How could you improve the study of the owls? → include prey density (regression) Combine regression & ANOVA in mixed model AIC is much lower in mixed model → anova(mixmodel1, mixmodel2) Fixed effects Random effects Anova Factor Random factor Regression Covariate Random factor Model General Linear Model Linear Mixed Model All Linear Mixed Models: Model 1: Anova → Fixed factor + Random factor Model 2: Regression → Covariate (fixed) + Random factor Model 2: Combination Anova & Regression → Fixed factor + Covariate factor + Random factor Pseudo Replication = similar conditions → data is not independent (plots too close to each other) Solution: add site as random factor in LMM (linear mixed model) Other examples: same family, same fish tank,... Solutions for pseudoreplication: Solution 1: Independent sampling (test) → Design Solution 2: Work with the means (e.g., tank) → Analysis Solution 3: (G)LMM + random factor → Analysis Solution 4: Repeated measures → Analysis Repeated measures in LMM Examlpe: one owl individual gets measured multiple times, owl number 1, owl number 2,... Solution → use ID as random factor Repeated measurements → Mixed model (e.g. LMM) ~ Random intercept model In lectures/assignments (=this course): → Random term for correction (e.g. pseudoreplicates) In papers/“real studies” (=advice for thesis): → Random term only if >7 classes (e.g. 8 sites), → else: Fixed factor Overview EMMeans = estimated marginal means