Druggability PDF
Document Details
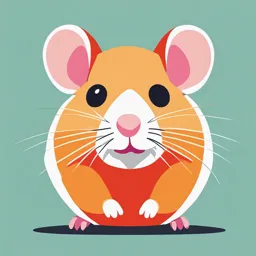
Uploaded by LucrativeYtterbium
Tags
Summary
This document discusses druggability, the ability of a protein target to bind small molecules, and its importance in drug discovery. It examines different methods of assessing druggability and their implications for various drug types.
Full Transcript
So what's this druggability? Definitions of druggability vary, it could be defined simply as the ability of a protein target to bind small molecules, but sometimes it might be more appropriately called ligandability or it could be defined as the likelihood of finding orally bioavailable small molecu...
So what's this druggability? Definitions of druggability vary, it could be defined simply as the ability of a protein target to bind small molecules, but sometimes it might be more appropriately called ligandability or it could be defined as the likelihood of finding orally bioavailable small molecules that bind to the target in the disease-modifying way. So, it includes consideration of pharmacokinetic and pharmacodynamic properties of the compounds, whether the target is related to a disease. This is obviously harder to assess but could be more predictive of drug discovery success in the long term. Whereas identifying whether a protein target just binds small molecules is slightly easier to assess I'd really opt for definition somewhere in the middle: so the ability of a protein to be modulated by a drug-like small molecule, this implies you're somehow modifying the function of that protein but you don't necessarily need to know what disease it's linked. When we talk about orally bioavailable or drug-like small molecules what do we really mean? Lipinski, a medicinal chemist at Pfizer came up with something called the rule-of-five and back in 1997 he basically looked at all of the drugs that were available and what kind of physical chemical properties they had and he observed that molecules were likely to have poor absorption or permeability if they violated more than one of these rules. So ideally the molecular weight of the compound would be below 500, the logP would be below 5, the number of hydrogen-bond acceptors would be less than 10 and the number of hydrogen bond donors would be less than 5. And this is really a rule of thumb that could be used to define whether a molecule is drug like or not. More recently people have gone further, they suggested other descriptors that can be useful like the number of rotatable bonds, the polar surface area of the molecule. A researcher at University of Dundee have come up with a method called quantitative estimate of drug likeness or QED which really builds on the work of Lipinski and provides a more quantitative approach rather than just a yes/no rule. So bearing this in mind, in order for a protein to bind a drug-like small molecule, the protein needs to have a binding site with complementary properties. So it needs to be the appropriate size to accommodate a drug like ligand, it needs to be buried to have sufficient surface area to interact. Not too polar or too hydrophobic either but it needs to have the right balance of properties to complement a drug like ligand. Things like really large exposed polar sites can be considered to be harder to drug than smaller, more buried hydrophobic pockets. This next slide just shows an example of two different proteins with different types of binding sites. The binding site on the left is phosphodiesterase 5. It can be considered to be what we'd call a beautiful binding site. Whereas the CMV protease on the right hand side has a much larger, more exposed polar binding site and it's much harder to find good drug like ligands for this kind of target. Why do we really need to assess druggability? Omics techniques are producing large amounts of data by relating genes or proteins to diseases that we were interested in studying. So there are lots of genome-wide association studies (GWAS) and sequencing studies that have identified mutations linking particular genes to diseases. There are large-scale expression studies comparing disease versus normal tissues; proteomics and metabolomics studies trying to find biomarkers for diseases, and when interpreting all of this data we really want to know which of these proteins that we've identified as being relevant to a disease are going to be the most successful for a drug discovery project. If we consider that maybe only 15% of the human genome might be druggable with a small molecule, what we really need to do is we need to intersect the proteins that are likely to be druggable with those that are likely to be disease-linked and identify the most promising drug targets from within those. It's important to be able to prioritize the targets that we identify and pursue those that are most likely to be amenable to a particular approach. So if a target doesn't bind small molecules we might spend a lot of time doing screening experiments HTS etc and not find any good hits. But even worse, in some respects we might find hits for small molecules in the screen but if we choose a target that doesn't bind drug-like small-molecules this might lead to failure even further down the line, because the compounds that we identified from those screens might have poor pharmacokinetic properties and not have good absorption and really not work on when we get into the clinic. So we really want to assess this as early as possible and it's estimated that around 60% of projects may fail at the lead identification or optimization stages. We have a number of different methods we can use for assessing druggability and these range from precedence-based methods where we already have compounds that are in the clinic or even an approved drugs that bind to that target. These give us the highest level of confidence of druggability and through ligand-based methods where we may have endogenous ligands tool compounds or even good drug like compounds but haven't yet reached the clinic. Then we can use structure-based methods if we have a crystal structure available for the protein and then finally we can try to use sequence based methods to really analyze those proteins where we don't have any other information Precedence-based assessment: if a proteins is the target of a proved small-molecule drug this gives us a pretty high degree of confidence and druggability. Ut also gives us some other information, so targeting this protein may be relatively safe. But there are some caveats: it's not an absolute guarantee of success for a different disease or a different “product profile”, so for example the existing drug could be an antagonist and you require an agonist, it could be a systemic drug but you need central nervous system penetration for the disease that you're interested in. Some drugs have shorter or longer half-lifes, or side effects and the acceptability of side effects should be underestimated as well. For example, a cancer drug might have quite a lot of adverse effects that wouldn't be acceptable for something that is supposed to be used for less severe indications. The ChEMBL database extract scientific information from the literature and organize it into a structured form. From full text of papers of the Journal of medicinal chemistry and other medical journals, are extracted information about the compounds that have been synthesized in those papers, the assays that have been performed on their compounds which could be protein targets or it could be things like cell based assays. Quantitative endpoint are extracted such as Ki values, but also functional assay measurements, and toxicity measurements. These data are organized into a structured way so people can query for particular targets or compounds that they're interested in. On top of this, medicinal chemistry and bioactivity data are also annotated around approved drugs and clinical candidates. At ChEMBL we try to identify the type of molecule whether it's a small molecule or antibody, indications on what that drugs is used for and the molecular targets of the drug. Using this information people can derive insights and tools for drug discovery, so really the data within ChEMBL tracks the full discovery process and so the medicinal chemistry literature data that we extract really covers the discovery stages of the drug discovery process and through lead discovery by lead optimization and then we aim to track clinical candidates and drugs to cover the clinical stages of the drug discovery process. And we try to assign molecular targets to approved drugs. I mentioned this is not trivial. In many cases, it's not a black and white thing. Quite often, there are different rules for how you can assign drug targets. So, for example, you could choose to use binding affinity measurements and really annotate a target if the drug is known to bind with high potency. You could consider compound selectivity; so, if the drug is non-selective and binds to all members of a protein family, for example, then you'd include all members of the family as the drug target. You can try to consider the approved indication. Should you only include a target if you have evidence that it's responsible for the efficacy in that particular disease? And then you could also consider expression data. Which targets are expressed in the particular tissue that's relevant to the disease that the drug's used for? Maybe you can narrow down the choice of targets further. And then, dealing with things like protein complexes. A lot of drugs bind to protein complexes rather than individual proteins. Do you include only the binding subunits where the drug actually interacts? Or do you include all subunits that make up that complex? And these are just a couple of examples to illustrate the complexity of actually assigning drug targets. Tiotropium is a drug that's approved for COPD, and it's a non-selective muscarinic antagonist. There are five members of this protein family, so it binds to all five family members. But it's actually a topical agent that's inhaled, and it's believed that the M3 receptor is the one with the highest expression in the lung and has been linked to contraction of smooth muscle in bronchoconstriction. So, based on the fact that we know it's approved for COPD, then we could try to annotate more specifically the muscarinic M3 receptor as being the key efficacy target there. Another example: benzodiazepine drugs. These are approved for various indications, including insomnia. They are well known to be positive allosteric modulators of GABA receptors and GABA(A) receptors, heteropentameric complexes, and they have different combinations of alpha, beta, and gamma subunits. There are six known different alpha subunits, three beta subunits, and three gamma subunits. We also know that binding of GABA, which is the endogenous ligand to the receptor, occurs between the alpha and beta subunits, and that benzodiazepines bind between the alpha and gamma subunits but only in some of the specific alpha subunits. So, they don't bind alpha six-containing receptors. How do we represent this kind of target? We could just include all of the possible subunits. We could try to further narrow down the classification. For example, it's been shown that the alpha 1 subunit is particularly important for insomnia, so we could try to further narrow down the specificity. So, we do try to tackle this in ChEMBL. We've assigned manually curated efficacy targets for all of the FDA-approved drugs and also anti-malarials. We try to annotate targets with which we know the drug directly interacts, the molecular targets, not the downstream or pathway targets. And we try to limit these targets to those that we know are responsible for the efficacy in the approved indication. So, we're not including targets that could be linked to adverse effects or other indications for which the drug is not yet approved, and we're not assigning targets just purely on the basis of pharmacology data. Although if you're interested in that, you can find that information within the chemical database for each drug. We tried to annotate the type, whether it's a small molecule or antibody, the action types, or whether it functions as an agonist, antagonist, or inhibitor. And then also, where we have information, we can annotate the binding site or subunit within the protein complex where the drug actually interacts. So, we have a number of different ways of handling targets within ChEMBL. This is just showing the molecular targets that we have within the database. Some drugs do interact with proteins, but others actually interact with DNA or with a ribosome. Some drugs actually target small molecules, like antitoxins for metal toxins. And then, even within protein targets, we have drugs that act on single proteins, like phosphodiesterase V. We have drugs that act on specific protein complexes, so for example, integrin alpha 4 beta 7. We have drugs that act on less well-defined protein complexes, like the benzodiazepine receptor example that I gave earlier. We have non-selective drugs that might act on all members of a protein family. For example, muscarinic antagonists. And there are a few drugs that actually disrupt protein-protein interactions as well. So, we're able to model these different types of targets. And if you want to find the list of approved drug targets, you can go to the "Browse Drug Targets" tab within the ChEMBL interface and download all the targets in a tab-separated file and do further analysis with them. We're also trying to include clinical candidate information. So, if the targets have compounds that have already reached the clinic in phase one, two, or three trials, this also provides good evidence that the target is druggable. And particularly, if a candidate has reached quite late phases, then it's likely to have shown a degree of safety and a good pharmacokinetic profile as well. So, we can get additional information from those cases. But a lot of the databases that provide comprehensive clinical candidate information are commercial or expensive. There are some resources available, but it's harder to find all of the information relating to the targets. So, clinicaltrials.gov is a freely accessible database of clinical trials, and it's required to register all US trials within the database, and they have information about the compounds that are tested, the indications, the phases of development, so what stage the trial is at, and you can search this or download data. But it doesn't contain target information in a large number of cases. Similarly, people often apply for a United States Adopted Name for a compound when it reaches around phase two of clinical development, sometimes earlier or later. So, if we track applications for these USAN names, then we can get a good idea of the compounds that are within clinical development. But as I mentioned, it's quite difficult to then identify the targets for all these clinical compounds. So, this usually requires searching of published literature. Sometimes pharmaceutical company pipeline documents contain information, and other web resources and there's a useful paper that was published by GSK quite recently and which lists and the targets that are in clinical development but it doesn't provide links back to the actual drugs their target notes and those proteins. I try to use bioactivity data such as that contained within ChEMBL to identify potential targets for compound. Although targets that the compound is most active against this is no guarantee that they're really the targets responsible for the efficacy for the indication. The United States adopted names that I mentioned has rules for assigning stems within the names and this can give you some clues as to the mechanism of action of the compound so names that end in panel for example here receptor antagonists names ending in Kirin of renin inhibitors so sometimes this can help with target assignment Here are a number of public resources that exist that capture pharmacology data so Ken wall I've mentioned already pubchem bioassay is a large database based in the US and which has a lot of high-throughput screening information; binding DB is another database who curates information from the published literature particularly around binding affinity measurements and then the guide to pharmacology is also a good resource and for identifying and pharmacology data for key drug target families and the existence of a compound that binds with high affinity to a protein and implies that it's trickable but it's also important to consider whether the compounds that you see are actually drug like and so it could be that there's a small molecule that binds to the target but it doesn't really obey Lipinski's rule of five it's not a very drug like compound it to be too large or polar and we can also consider things like selectivity issues so all the compounds that bind to a target nonselective then it could be harder to see that target. So this just gives an indication of how the bioactivity data is organized within ChEMBL so you can search for protein targets and then go to a report card page you see a summary of information about that protein target and then you have these little graphs that you can click on to go through to a bio activity data being where you see all the individual binding measurements for example that have been recorded with links back to the publications that they came from Even f you don't have medicinal chemistry compounds and for a given target even knowledge of the endogenous ligand or substrate for protein could be useful in assessing druggability so for a protein is known to bind a small molecule within the body there's no reason you might not be able to find a good drug-like small molecule as well, and you can identify databases that have ligands information and try to use these to identifyadditional proteins that might have small molecule binding sites. Again, guide to pharmacology has quite a lot of endogenous ligand information. You can also use crystal structures in the protein databank so if you go to PDB for example you can look for all the crystal structures that contain small molecule ligands and that implies those proteins are druggable. It's useful to consider the type of endogenous ligand so if the ligands are peptide or protein for example like proteases and it's likely to be harder to drib these type of targets then if the endogenous ligand is itself drug like. Let me just show the guide to pharmacology resource. The targets within this database are organized by different protein families, you can click to go through to individual targets within these families and then you can view ligands information for that target. So they have a wide range of information around typical tool compounds endogenous ligands and also drug like compounds, and approved drugs in some cases, together with binding affinity data and links back to publications. Even when you don't have any ligand-based information, you may be able to use structurebased methods to try to assess the drug ability of a protein. These methods really rely on identifying cavities within crystal structures and then assessing these cavities to try to predict whether they have appropriate properties to find drug-like small molecules. So typically, what's done is to take a test set of data from the Protein Data Bank where you have co-crystal complexes with drug-like ligands bound to them. Then, the properties of these and the cavities where the drug-like ligands are bound are assessed. You can calculate lots of different descriptors like volume, surface area, polarity of this area, degree of burial, etc. And then train an algorithm to distinguish the properties of those cavities that do have drug-like ligands bound from other cavities that don't have drug-like ligands. And then you can apply these rules to new proteins. So if you have a novel crystal structure which has been solved, you can try to identify all of the cavities within that protein and assess them to see whether the properties suggest it could be a drug-like binding site or not. There are lots and lots of different algorithms that have been developed to do this kind of thing. There's just one example that I've chosen because it has a nice web interface and where you can actually interact with the predictions. So, DoGSiteScorer was trained on a dataset of around a thousand targets that had been manually assigned to one of these categories: either being druggable, being difficult to drug, or being undruggable. They used a support vector machine approach to build a model for whether targets were druggable or not, and you can go to this web server and try out the algorithm. All you need to do is select a PDB structure that you're interested in analyzing and you paste that in, and then you get back this nice and kind of graphical display. So, down the left-hand side, they show all the different cavities or binding sites that they've identified within the protein structure, and some of the properties of this site. Then each of these sites is assigned a druggability score. The ones shown in green at the top here are predicted to be druggable, those at the bottom in red are predicted to be undruggable, and then you really get back some nice information. So, on the right-hand side, you can see a picture of the protein structure with the cavity highlighted. So, it's not sort of a black box method in so much as if you see that site P0 is predicted to be druggable, you can then see where on the crystal structure that is, and really that could be very valuable information later on for things like structure-based drug design. Ligand-based methods are great because they give you a reason behind grave confidence and drug ability, but they can't really tell us anything about novel targets. Structure-based assessments, similarly, give us something really concrete to work with: a pocket within a crystal structure. But if we don't have a crystal structure available for our protein of interest, then we can't really apply those methods. So, we really need additional methods to help us to prioritize the remainder of the proteins within the human genome or indeed within other genomes, such as those of parasites. So, there may be many druggable proteins within these genomes that haven't really been investigated previously. And we can use homology in some cases. So, we can do a BLAST search and identify whether a sequence is similar to a known drug target. But again, this is really only telling us about past success and won't really take us into identifying novel families. There are some of the sequence-based methods that can potentially go a little bit further. So, with the earliest definitions of the druggable genome, for example, the paper in 2002 by Hopkins and Greene relied on identifying the drug-binding domains within known drug targets and then identifying other proteins containing those binding domains. You could use this list to do sequence similarity or domain analysis of other proteins and try to identify additional proteins that have one of these known druggable domains within them. This takes us a little bit further than just using sequence similarity alone, but again, it's still based on past success. People are trying to come up with more general methods for defining the features of a good drug target, and machine learning methods can be used here. Since these are generally based on amino acid sequence only, they can be applied to whole genomes. They don't need any other information to be available like structures or ligands. The basis of these methods is to calculate a large number of different descriptors from the amino acid sequences. So, this can be things like amino acid composition, length, hydrophobicity, the presence of certain features like transmembrane domains, signal peptides, and glycosylation sites. Then, taking into consideration things like predicted secondary structure, domain composition, or subcellular localization - things that can be predicted based on the sequence. And then, by defining which descriptors are enriched in known drug targets versus targets that are not known to be druggable, we can really come up with a model that will help us to predict whether a protein is druggable or not based on which of these descriptors and it satisfies. I've covered really the methods that we can use to assess small molecule drug ability of a protein, and this has been historically the approach that has been most desirable for pharmaceutical companies. But based on our best estimates, the overlap between small molecule drug-able proteins and disease-modifying proteins may be relatively small, as I showed at the beginning. Therefore, we might need to consider other approaches to target proteins that don't necessarily have small molecule binding sites. So, these could be inhibiting protein-protein interactions or using protein therapeutic or monoclonal antibody approaches. Or even other approaches like siRNA and monoclonal antibodies, in particular, are becoming increasingly important in drug discovery. The ability to target a protein with a protein therapeutic drug, such as a monoclonal antibody, largely just depends on the extracellular location of the protein. So, the protein needs to be secreted or plasma membrane-bound; otherwise, it won't be accessible to a large molecule like an antibody. And we can determine this in a number of ways. So, if we know there are no protein therapeutic drugs already available for the target, we can use that information. But we can also use annotation, experimental evidence, and content within resources like UniProt and the Gene Ontology, which really tell us which proteins are already known to be membrane-bound or found in plasma or secreted. And then, these resources also have predictions as well. So, proteins that are predicted to have transmembrane domains, predicted to have signal peptides, which suggests they could be secreted, and there are also subcellular localization prediction algorithms that can be used. So, if we use a resource like UniProt, which is shown at the bottom here, for example, we can get quite a lot of information about the cellular localization of the protein. Moving on to and trying to target protein-protein interactions. So, even if we don't have a small molecule binding site on a protein, it's almost certainly going to participate in proteinprotein interactions of some sort. So, if we're able to modulate this interaction, that could be a highly effective means of modulating the target. However, protein-protein interaction surfaces are often large, flat surfaces, so they're not really beautiful binding sites. Targeting these large, flat surfaces is quite difficult, particularly if we want an oral small molecule drug. You're likely to end up with something that's a peptide mimetic. But research has shown that these surfaces may still have hot spots, so sort of sub-pockets within the larger surface that contribute most to the binding affinity. And it may be possible, if you know where these hot spots are, to design smaller inhibitors that specifically target these areas of the surface. And there are lots of algorithms that are being developed to predict such hot spots and try to assess the drug ability of protein-protein interaction sites. So, just trying to put all of this together, this is kind of my view of the druggable genome based on data that we have currently within Kimball. I wouldn't take the numbers here too literally because it really depends on exactly what data sets you include and what criteria are used, and you can come up with slightly different values. But it's really to give you an idea of the kind of scale of the druggable genome. So, if we consider just the targets of approved drugs, and I filtered out some of the really nonspecific drugs that bind large numbers of molecules, we have around 400 proteins that are known to be targeted by small molecule drugs that are already FDA-approved. And we have around a hundred targets for protein therapeutics, including things like recombinant proteins and antibodies. There are a small number of targets that overlap, that have both small molecule drugs and protein drugs. If we try to expand this out to include clinical candidates as well, there may be in excess of 300 to 350 proteins that have protein therapeutic drugs in development or already approved. And then for small molecules, we don't get a comprehensive set of clinical candidates within the ChEMBL database, but there's probably in excess of 1,000 to 1,200 that may have small molecules within the clinic. If we then expand this out further, so targets that have drug-like small molecules within the chemical database but where those molecules aren't already in the clinic, we can expand this out to around 2,000 different protein targets. And then, if we also include some of these predictive drug ability methods including domain similarity and structural methods, for example, we can expand this out to maybe 3,000 or more proteins for viable therapeutics. If we include all extracellular targets based on annotations within things like UniProt and Gene Ontology, this could be between around 2,500 and 4,000 different proteins, depending on whether you take into account the more predictive methods like signal peptide identification or just those ones that have concrete experimental evidence at the moment. And if we combine all this, because there's quite a significant overlap between extracellular proteins and small molecule targets, maybe around 5,000 proteins in total that could be targeted by one of these two approaches. So, this is around a quarter of the human genome. So, knowing this information, knowing which proteins are likely to be druggable or not, how can we really apply this information? It's quite critical to use this for things like target prioritization. So, if you're doing biological experiments or looking at biological data that links targets to particular diseases of interest, you have a large number of potential targets and you want to know which to pursue. Using the druggability information can be really valuable. For example, if you know that the target already has an approved drug, this can suggest a drug repurposing opportunity. Targets that have clinical candidates, for example, would be really highly druggable, and therefore, there's a high chance of success if you pursue that kind of approach. If you know that the target binds a small molecule, again, it might be much easier to start a drug discovery program around this target, already having chemical matter that you can use as a start. Then, if you know the protein is extracellular and you think a monoclonal antibody approach could be suitable for that indication, then this would really allow you to try to pursue those targets. And again, just members of known druggable families or proteins that contain domains that have been known to be drugged in the past can help us to identify potentially novel targets that could be tractable with smalmolecule approaches. This is a publication from Nature a couple of years ago, and the authors studied a large number of subjects, a lot of whom suffered from rheumatoid arthritis, and tried to identify genetic variations within these patients that were linked to the incidence of rheumatoid arthritis. So, they identified 101 different risk loci within the genomes of those subjects that were linked to rheumatoid arthritis. Then, when they looked at where these loci actually are and mapped them to genes, there were around 98 different candidate genes that they identified. Many of these loci were actually novel and hadn't been identified in previous studies. So, you have these 98 genes, where do you want to go next? This is really where the drug target information can come in. The authors looked at whether any of the candidate proteins that they identified were targets for existing drugs, both approved and experimental, and they found that 27 of these proteins were already targeted by approved drugs for rheumatoid arthritis. So, they've kinetically confirmed the genetic associations with known drug to information, but also, as well as those targets, they notified some additional proteins that had drugs on the market, with those drugs were for other medications, not for rheumatoid arthritis. So, these are potential drug repurposing opportunities. For example, cdk4 and cdk6 were identified among the candidate genes, and Palbociclib is a compound that's been recently approved for breast cancer and actually targets these two cyclin-dependent kinases.This could be a potential drug repurposing opportunity and that could be quite a quick win in terms of really using the output from this genetic study. So, you can say that understanding the mechanism of action and targets of existing drugs is quite crucial in exploiting this biological data that's being produced. Even if we didn't have any drugs or clinical candidates already available for a particular target, then knowledge of drugability information can still be used to prioritize which proteins might be suitable for further follow-up. Particularly, for example, if you have compounds already available, you might be able to test those proteins in a disease model and see if there is any kind of impact on the disease that you're looking at. You can do one large-scale analysis, so you can try to assemble across all different diseases that you might be interested in and all the biological evidence that you have that links a target to a disease. This could be things like expression data, text mining information, genetic associations, and also knowledge of clinical projects that are already being carried out, perhaps by other organizations. You can assemble this information in order of confidence in biology. Just the fact that proteins are expressed in a particular tissue doesn't give you very strong evidence of disease linkage, whereas something like a very statistically significant genetic association or the presence of a project in clinical development would give you much more confidence. Then you can overlay the drugability information onto that, increasing confidence in chemistry if you like, from the drugability prediction methods, the ligand-based methods where you have chemical tools available for target clinical compounds and finally approved drugs. By combining all this information together, you can really see each one of these dots would represent a potential protein or target you can try to identify areas of space and for your particular disease that you might want to look at. So up here we have reasonably high confidence linking the target to a disease and we already have drugs or clinical candidates available so those could be potential repurposing opportunities. On the left-hand side, you have relatively weak information linking the target to the disease but you do have chemical tools available so these approaches might be quite high-risk but they are testable. So you have a compound you could potentially use in the disease model and see if there is any effect on the right hand side we have a high linkage to a disease we don't have any clinical compounds already but we do have chemical tools so again these are lower risk opportunities and they are again testable. So this might be an area you would want to pursue quite quickly and then moving down further if we don't have any chemical tools available but the target is predicted to be druggable and there's good evidence linking it to a disease this is an opportunity to try to identify some novel chemistry for these targets and which we predict would have a good degree of success because the target is druggable and then right down at the bottom you have strong linkage between the target and the disease but the target's not predicted to be druggable so this is where a novel bi-therapeutic approach might be used. So this is just to give you an idea of really how you can use this drugability information, knowledge of the drugable genome to help further drug discovery.