Psychometric Testing PDF
Document Details
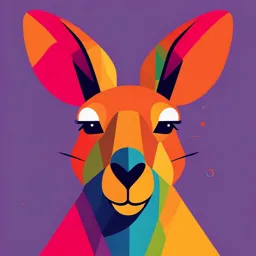
Uploaded by DexterousBoron
Tags
Summary
This document provides a general overview of psychometric testing, including psychological constructs, operationalization, different types of tests, and measurement error. It touches on applications across sciences, particularly in psychology, and discusses various applications from education to business and health. Concepts like validity and reliability of measurements are also briefly introduced.
Full Transcript
Psychometric Testing Focusses on the measurement of psychological constructs through questionnaires and scales. This involves understanding the nature of constructs, ensuring the reliability and validity of measurements, and effectively managing questionnaire data. Psychological Constructs:...
Psychometric Testing Focusses on the measurement of psychological constructs through questionnaires and scales. This involves understanding the nature of constructs, ensuring the reliability and validity of measurements, and effectively managing questionnaire data. Psychological Constructs: 🧠 Definition: Abstract ideas like intelligence, stress, or satisfaction, measured 📏 Operationalisation: Translating constructs into measurable variables (e.g., a indirectly through items/questions. questionnaire on stress levels). ○ Constructs are operationalised through measures. Different operationalisations make it difficult to consolidate findings: Jingle fallacy - Using the same name to denote different things. ○ Both produce narcissism scores but measure completely different things. Jangle fallacy - Using different names to denote the same thing. ○ Leadership psychology makes up new measures that are never used again. Problem with money. Scientific discipline concerned with the construction of psychological measurements. Connects 👁️ Observable phenomena (e.g., item responses) to ✅ theoretical attributes (e.g., life satisfaction)T Theoretical constructs are defined by their domains of observable behaviours. Psychometricians study conceptual and statistical foundations of constructs, the measures that operationalise them and the models used to represent them. Applications across many sciences (e.g., psychology, behavioural genetics, neuroscience, political science, medicine). Tests of typical performance What participants do on a regular basis. Examples: Interests, values, personality traits, political beliefs. Real-world example: “Which Harry Potter house are you in?” Tests of maximal performance What can participants do when exerting maximum effort. Examples: Aptitude tests, exams, IQ tests. Real-world example: Duolingo, Wordle, revision apps. For the most part, the same statistical models are used to evaluate both. Types of Psychometric Tests 🎓 Education Aptitude / ability tests (i.e., standard school tests). Vocational tests. 👩💼 Business Selection (e.g., personality, skills). Development (e.g., interests, leadership). Performance (e.g., well-being, engagement). 🏥 Health Mental health symptoms (e.g., anxiety). Clinical diagnoses (e.g., personality disorders). People make life-changing decisions using psychometric evidence every day. The largest way that psychology touches the outside world. Criteria Lots of important applications, so psychometrics must: ○ Validity: The extent to which the test measures what it pursuits to measure. ○ Reliability: The consistency of the measurement across time and different situations. ○ Interpretability: The clarity with which scores can be understood and used. ○ Relevance: The applicability of the test to specific populations or contexts. ○ Fairness: The ability of the test to differentiate between individuals without bias. Diagrammatic Conventions Square = Observed / measure ○ Collect data on an item. Circle = Latent / unobserved ○ Construct we are estimating. Two-headed arrow = Covariance Single headed arrow = Regression path Representational NOT Actual Measurement. → We measure item responses, not the construct itself. Implicit memory test isn’t actually measuring memory, it’s measuring performance on the task. Measurement Error Random = Unpredictable. Inconsistent values due to something specific to the measurement occasion. Systematic = Predictable. Consistent alteration of the observed score due to something constant about the measurement tool. Social desirability - self-reporting. Any moral valence = likely wrongly reported. Correlations and Covariance Unit of analysis is covariance. Trying to model the covariance amongst 2 variables. Variance = Deviance around the mean of a single variable Covariance = Representation of how two variables change together Correlation = Standardised version of covariance We are trying to explain patterns in the correlation matrix. I.e. among a set of items Pearson Correlation Coefficient (r) measures the strength and direction of the linear relationship between two variables. The coefficient r ranges from -1 to 1: 1: Perfect positive correlation (as one increases, the other increases linearly). 0: No correlation (no linear relationship between variables). -1: Perfect negative correlation (as one increases, the other decreases linearly). The diagonal values (1.00) represent the correlation of each variable with itself, which is always r=1. The off-diagonal values represent the correlation between different pairs of variables. lfsat_1 and lfsat_2: Correlation = 0.72 (strong positive correlation). lfsat_4 and lfsat_5: Correlation = 0.72 (strong positive correlation). lfsat_1 and lfsat_4: Correlation = 0.00 (no linear correlation). Two Distinct Groups of Variables: ○ Group 1: lfsat_1, lfsat_2, lfsat_3 (highly correlated among themselves). ○ Group 2: lfsat_4, lfsat_5, lfsat_6 (highly correlated among themselves). ○ The lack of correlation between Group 1 and Group 2 suggests that these two sets of variables may measure different constructs or dimensions. Potential for Factor Analysis: ○ This correlation matrix suggests that a factor analysis or Principal Component Analysis (PCA) might reveal two latent factors underlying these variables. Implications If these are survey items (e.g., life satisfaction measures), the two groups (Group 1 and Group 2) might correspond to different dimensions of life satisfaction. Variables with strong correlations can potentially be collapsed or summarised using techniques like PCA to reduce dimensionality. Variables with no correlation (e.g., lfsat_1 and lfsat_4) do not appear to share a linear relationship. Scale Scores Classical Test Theory Describes scores on any measure as a combination of signal (i.e., true score) and noise (i.e., error): Our test measures some ability or trait, and in the world there is a “true” value of score on this test for each individual. Observed score unlikely to reflect the participants’ true value of construct. CTT Diagram True score = Variance in the score explained by the target construct. ○ E.g., your height. plus + Error = Variance in the score explained by other things (i.e., random or systematic). ○ Ruler measurement is wrong. Observed score = What we actually record in the dataset. Goal of testing is to minimise error in observed scores. Frequentist observation - probability of an event as the proportion of times that the event occurs in a sequence of possibly hypothetical trials. The true score doesn't give your real value of depression, but the score on a certain depression test. Variables are linked by straight arrows that indicate the directions of the causal relationships between them. Scoring in CTT Items summed or averaged (i.e., the mean) to create a score for the target construct. Scores are created by aggregating responses to multiple items. Groups of items measuring the same construct referred to as scales. One or more related scales are administered as a measure, test, or battery. ○ Makes a measure multidimensional. ○ E.g., life satisfaction - work, relationships, social status etc… ○ Might have a life sat work scale, a life sat relationship scale etc… = a battery ○ Single unit, unidimensional, multi-dimensional. Assumption of Classical Test Theory Indicators are equivalent (i.e., all items measure the construct to the same extent) ○ Is this a realistic assumption for psychological tests? Do “I am never dissatisfied with my life” and “I am relatively happy about my life” both measure life satisfaction to the same extent? Evaluating Psychometric Testing Validity = consistency of test results across multiple administrations. Important: Tests can be highly reliable but not at all valid, depending on construct. Tape measure is a reliable measure of stars, not of leadership! Reliability = less ambiguous than validity. Validity to some degree “in the eye of the beholder” - is more of an inference. ○ Depends on what you’re trying to set out to do, Parallel Tests Charles Spearman was the first to note that, under certain assumptions (i.e., tests are truly parallel, each item measures construct to the same extent) correlations between two parallel tests provide an estimate of reliability. E.g., 15% is measurement errors. Parallel tests can come from several sources: ○ Time tests were administered (test-retest). ○ Multiple raters (inter-rater reliability). ○ Items (alternate forms, split-half, internal consistency). Test-Retest Reliability Correlation between tests taken at 2+ points in time (assumed to be equivalent) - one of the strongest tests. How stable is performance on this test. What’s the appropriate time between when measures are taken? ○ We expect memory to change over time so how does this relate? How stable should the construct be if we are to consider it a trait? Inter-Rater Reliability Ask for self-report on personality + others report on personality. Look at the relationship between them. Ask a set of judges to rate a set of targets, compare similarity: Get friends to rate the personality of a family member Get zookeepers to rate the subjective well-being of an animal We can determine how consistent raters are across: Their individual estimates (i.e., across targets) How reliable is the average estimate based on the judges’ ratings (i.e., across raters) Alternate Forms and Split-Half Reliability Correlation between two variants of a test: Same items in different order (randomise the stimuli). Tests with similar, but not identical content (e.g., tests with fixed number of numerical problems). Assumption: If the tests are perfectly reliable, they should correlate perfectly (they won’t, estimate = reliability). Split-half reliability: Split test into equal halves, score them up and correlate the halves. How much does items set 1 correlate with item set 2. Algorithms will do it for every potential combination of these items. Internal Consistency Extent to which items correlate with each other within a scale. How much does any given item relate to all the other items in the test? Most common assessment of reliability: Easy and cheap, all at one time-point with one set of items. Calculated through some form of average covariance / average (variance + covariance) Multiple ways to estimate: Cronbach’s Alpha = Assumes all items equivalent measures of construct in the same way. ○ Cut off = 0.7 McDonald’s Omega = Does not, allows for items to relate to the construct in different ways. Answers to these questions, if similar, should be consistent and similar among individuals. Validity Validity refers to the degree to which evidence and theory support the interpretations of test scores for proposed uses of tests. “A test is valid for measuring an attribute if (a) the attribute exists and (b) variations in the attribute causally produce variation in the measurement outcomes” (Borsboom et al., 2004) “The goal of psychometric development is to generate a psychometric that accurately measures the intended construct, as precisely as possible, and that uses of the psychometric are appropriate for the given purpose, population, and context.” (Hughes, 2018) Content / Construct validity ○ A test should contain only content relevant to the intended construct. ○ It should measure what it was intended to measure. ○ “Easy” for questionnaire items, hard for tasks / implicit measures. ○ E.g., “I enjoy directing others” - Leadership questionnaire Face validity ○ i.e., for those taking the test, does the test “appear to” measure what it was designed to measure? ○ Less relevant for questionnaire items + more relevant for implicit tasks. E.g., measuring impulsivity using a balloon blowing up task. All measurement in a test occurs between the participant reading the item and selecting a response. Everything we have discussed so far is analysed after measurement. ○ Post-hoc. Need to assess content / construct validity during data generating process (i.e. when completing the questionnaire). ○ How does one judge the item, what is their process for coming to an answer. Can do this using qualitative think-aloud-protocol interviews: ○ Participants complete a questionnaire, select response options and verbalise reason / self-construal / opinion. Example of a think-aloud-protocol output: Provide justification for why they were selecting a response. ○ “Tell me why you ‘strongly agree’ with that?” ○ Can understand that participants share the desired definition of constructs. Idiosyncratic, very time consuming, very expensive. Structural Validity Many constructs are multi-dimensional i.e. they have multiple underlying components ○ e.g. Narcissism = Grandiosity + Vulnerability + Antagonism. If I am going to group these items into multiple scales, how do I do it? Goal is to assess whether the the items ‘fit’ this structure Then assess stability of structure across samples / time / groups. Most commonly assessed using exploratory / confirmatory factor analysis. Relationships with Other Constructs Once sure about structure of test, might want to consider against other measures: ⬆️ Convergent: Measure should have high correlations with other measures of the same construct. ⬇️ Discriminant: Measure should have low correlations with measures of different constructs Nomological net: Measures should have expected relations (positive/negative) correlations with other constructs. Also, some measures should vary depending on manipulations (e.g., a measure of “stress” should be higher when about to take an exam). ○ E.g., conscientiousness positively correlates with educational attainment, income etc. Reliability: relation of true score to observed score. Validity: correlations with other measures play a key role. Low reliability: correlations between observed variables are attenuated and underestimated. Reliability is thus the ceiling for validity: tests cannot correlate with each other more than they correlate with themselves. Test manuals (should) contain all information needed to assess reliability and validity: Papers describing new tests and papers investigating existing measures in different groups, languages, contexts, etc. Assessment Psychological Assessment European Journal of Psychological Assessment Organisational Research Methods Personality journals Papers describing new ways to establish reliability, validity, etc found in: Behaviour Research Methods Psychometrika Multivariate Behavioural Research Other Scoring Techniques Unit Weighted Scores Mean score from the set of items assumes all contribute equally. Equivalent to multiplying each observation by 1 before summing. Do both items contribute the same to life satisfaction? Weighted Scores Weighted scores created by multiplying each observation by unique weight before averaging / summing. Allows each item to contribute in a unique way. More realistic representation of psychometric items? How to Identify the Weights Dimension reduction models reveal relationships between observed variables/items and composites/underlying dimensions (i.e., aggregations of multiple items). Two most common in psychology: Principal components analysis (PCA) and Factor analysis (FA) PCA = items –> component FA = latent variable (factor) –> items Important: Can also use these techniques to assign items to scales. Questionnaire Data Handling 1. Data Cleaning: ○ Handle missing responses. ○ Recoding items for consistency (e.g., reverse coding). 2. Reliability: ○ Internal Consistency: Ensuring all items in a scale measure the same construct (e.g., Cronbach’s Alpha). 3. Validity: ○ Ensuring the questionnaire accurately measures the construct it is intended to. Reverse Coding: Some items are phrased negatively (e.g., "I feel dissatisfied with my life"). Responses to these items need to be reversed for consistency in scoring. Working with Questionnaire Data Handling questionnaire data involves several steps to ensure accuracy and meaningful analysis: Data Wrangling: Cleaning and organising data, which may include renaming variables for clarity, recoding responses into numerical formats, and handling missing data. Reverse Coding: Adjusting items where higher scores indicate lower levels of the construct to ensure consistency in scoring. Scale Scoring: Aggregating item responses to compute overall scores for each construct measured. Given a dataset where responses are recorded as text (e.g., "Strongly Agree," "Agree"), these need to be converted to numerical values (e.g., 5 for "Strongly Agree" to 1 for "Strongly Disagree") to facilitate analysis. Practical Application Consider a study assessing stress reduction techniques. Participants complete a 6-item stress questionnaire before and after an intervention. Each item is rated on a 5-point Likert scale. Steps: 1. Data Importation: Read the dataset into a statistical software environment. 2. Variable Renaming: Assign concise, meaningful names to variables (e.g., t1_q1 for Time 1, Question 1). 3. Recoding Responses: Convert textual responses to numerical values for analysis. 4. Reverse Coding: For items where higher scores indicate lower stress, reverse the coding to align with the overall scoring direction. 5. Calculating Scale Scores: Sum the item scores to obtain an overall stress score for each participant at each time point. 6. Analysis: Compare pre- and post-intervention stress scores to evaluate the effectiveness of the intervention. Principal Component Analysis (PCA) Is a statistical technique used to reduce the dimensionality of data by transforming a large set of variables into a smaller one that still contains most of the information in the original set. Purpose of PCA: ○ PCA aims to identify uncorrelated components (principal components) that capture the maximum variance in the data. ○ It simplifies complex datasets by reducing the number of variables while retaining essential information. Dimension reduction rationale: 1. Theory Testing - number and nature of dimensions that best describe a theoretical construct. 2. Test construction - which items are the best measures of my constructs? 3. Pragmatic - multicollinearity issues/too many variables = high standard error. Pull out multicollinearity to stabilise model. When to Use PCA: ○ To address multicollinearity issues in regression models. ○ For data visualisation and noise reduction. ○ Reduce dimensionality = make 10 constructs into 3. ○ To identify underlying structures in the data.. ○ To maximise variance explained in our observed data. ○ Not necessarily assuming that relationships in data is caused by latent factors. PCA identifies components (linear combinations of observed variables), but these components are not latent constructs. Goal is explaining as much of the total variance in a data set as possible. ○ Starts with original data. ○ Calculates covariances (correlations) between variables. ○ Applies a procedure called eigendecomposition to calculate a set of linear composites of the original variables. Factorisation is e.g., 12 - 6 + 2 or 3 + 4. Performing PCA: 1. Standardise the data if variables are on different scales. 2. Compute the covariance or correlation matrix. 3. Calculate eigenvalues and eigenvectors to determine principal components. 4. Decide the number of components to retain based on criteria like the Kaiser criterion (eigenvalues > 1) or scree plot analysis. Interpreting PCA Output: ○ Loadings: Correlation coefficients between original variables and principal components. ○ Scores: The representation of original data in the new component space. ○ Eigenvalues/Explained Variance: Indicates how much variance each principal component captures. What does it do? Repackages the variance from the correlation matrix into a set of components. Each item contains 1 variance, take as much variance as possible + put it into one variable. Take as much covariance as possible + cram it into the first component. ○ This component can't be correlated with the next component, how much variation can we pull out + put it in. Components = orthogonal (i.e.,uncorrelated) linear combinations of the original variables 1st component is the linear combination that accounts for the most possible variance. 2nd accounts for second-largest after the variance accounted for by the first is removed. 3rd…etc… Each component accounts for as much remaining variance as possible. 🤞 If variables are very closely related (large correlations), then we can represent them by fewer composites. We can represent these using only 2 or 3 components. Capturing information in less. 🤟 If variables are not very closely related (small correlations), then we will need more composites to adequately represent them. 🤲 In the extreme, if variables are entirely uncorrelated, we will need as many components as there were variables in original correlation matrix. Eigendecomposition Components are formed using an eigen-decomposition of the correlation matrix Eigen-decomposition is a transformation of the correlation matrix to re-express it in terms of eigenvalues and eigenvectors Eigenvalues are a measure of the size of the variance packaged into a component: Larger eigenvalues mean that the component accounts for a large proportion of the variance = the more variance packaged into it. Visually (previous slide) eigenvalues are the length of the line. Eigenvectors provide information on the relationship of each variable to each component: Visually, eigenvectors provide the direction of the line. Where the line points through a cloud of observations. Where the variable is positioned along the long. There is one eigenvector and one eigenvalue for each component. Each eigenvector is contributing to the eigenvalue + has a position on the eigenvector. Eigenvalues and Eigenvectors Eigenvectors are sets of weights (one weight per variable in the original correlation matrix). Matrix represents how each item relates to each component. ○ e.g., if we had 5 variables each eigenvector would contain 5 weights ○ Larger weights mean a variable makes a bigger contribution to the component. First line = Eigenvalues (if we multiply these together we can reproduce our correlation matrix). W11 = relationship between this item + this component, e.g., sleep W21 = e.g., BMI ALWAYS get the same number of components as variables + the eigenvector shows these weights. It’s the extent to which they are more or less correlated that affects the size of the components. Really strongly correlated = first components would be very big. Less so = roughly all at the same time. Want them to be as big as possible + we keep them. Might first decide to do an unconstrained PCA but look at output + make decisions of what component we might want to keep. Eigenvalues and Variance We can use the eigen() function to conduct an eigen-decomposition for our health items. Eigenvalues 1st component carrying the weight, doing the work, so if we could only save one then we would choose this. When you add all eigenvalues together, will be how many variables in our data set. Redistributing the variance from “1, 1, 1, 1, 1, 1” - Eigenvalues always sum to the number of values in our dataset. Eigenvectors How much each item relates to each component. Things along the y axis = eigenvector Column = each item, how much eigenvalue relates to component, defined by the eigenvector. 1 column = 1 vector The sum of the eigenvalues will equal the number of variables in the data set: The covariance of an item with itself is 1 (think the diagonal in a correlation matrix). Adding these up = total variance. A full eigendecomposition accounts for all variance distributed across eigenvalues. So the sum of the eigenvalues must = 6 for our example. sum(eigen_res$values) If we want to know the variance accounted for a given component: p = number of items Calculates the percentage/proportion of each variance contained in each eigenvalue. Together, eigenvalues capture 100% of this variance. Coefficients are called component loadings for interpretation. Not weights but influenced by them. How Many Components to Keep? The relation of eigenvalues to variance is useful to us in order to understand how many components we should retain in our analysis. Eigen-decomposition repackages the variance but does not reduce our dimensions. Dimension reduction comes from keeping only the largest components: ○ Assume the others can be dropped with little loss of information Simple cutoff = 1. If a component contains less variance than a single item does then it’s worthless + there's no point of including it. Our decisions on how many components to keep can be guided by several methods: Set a amount of variance you wish to account for Scree plot Minimum average partial test (MAP) Parallel analysis Each component accounts for some proportion of the variance in our original data. Kaison criteria - eigenvalue > 1 The simplest method we can use to select a number of components is simply to state a minimum variance we wish to account for. We then select the number of components above this value. Scree Plot Based on plotting the eigenvalues: ○ Remember our eigenvalues are representing variance. Looking for a sudden change of slope. Assumed to potentially reflect the point at which components become substantively unimportant. ○ As the slope flattens, each subsequent component is not explaining much additional variance. Where the line bends = the kink = take everything above that. eigenvalues0.3: Weak but meaningful. >0.4: Stronger relationship. >0.6: Very strong relationship Salient loadings indicate which items contribute significantly to each factor and help assign items to specific factors. Finding the Range of Communalities We Need Communality (h2) is the proportion of variance in an item explained by all the extracted factors combined. To find the range: ○ Look at the communalities (h2) in the output of your factor analysis. ○ These values are typically listed in a column titled "Communalities" or calculated as the sum of squared loadings for each item across all factors. Interpreting the Range: h2≈0.4 or higher: The item is well explained by the factors. h2) on multiple factors, suggesting overextraction as the factors are not well-defined. What to Do Next: Run parallel analysis to confirm the number of factors/components that exceed the random eigenvalue threshold. Reassess factor retention using the scree plot and ensure all retained factors are interpretable. Consider using model fit indices (e.g., RMSEA, AIC, BIC) to evaluate whether the solution improves with fewer factors. Signs of Under Extraction Underextraction means you’ve retained too few factors, resulting in a loss of meaningful structure in the data. Signs in the Solution: 1. High Residual Correlations: ○ Residual correlations (differences between observed and reproduced correlations) remain large, suggesting the retained factors don’t fully explain the data. 2. Low Total Variance Explained: ○ The cumulative variance explained by retained factors is too low. ○ Ideally, cumulative variance should be >70% in PCA, or sufficient to reflect meaningful latent constructs in EFA. 3. High Communalities Not Explained by Fewer Factors: ○ If communalities (h2) for items are still high after underextracting, it suggests that additional factors are needed to capture the shared variance. 4. Interpretable Factors Missing: ○ Key constructs expected based on theory or prior research are absent in the retained solution. What to Do Next: Reassess the scree plot for the "elbow" where eigenvalues level off. Conduct a parallel analysis or minimum average partial (MAP) test to evaluate the optimal number of factors. Extract additional factors and check whether interpretability and fit improve. A good solution strikes a balance, retaining enough factors to explain the variance without overfitting noise. Signs in the Solution: 1. Eigenvalues and SS Loadings: ○ Retained factors have eigenvalues >1 (in PCA). ○ SS loadings in EFA are sufficiently large to indicate meaningful contributions to explained variance. 2. Sufficient Items Per Factor: ○ Each factor has at least 3-4 items for reliability with strong salient loadings (∣loading∣>0.3), indicating stable and well-defined factors. 3. High Total Variance Explained: ○ Retained factors explain a large proportion of the variance (>70% in PCA). ○ In EFA, ensure the total variance explained reflects the complexity of the data. 4. Clear Interpretability: ○ Factors are conceptually meaningful, and loadings align well with theoretical expectations. ○ Minimal cross-loadings between factors. 5. Low Residual Correlations: ○ Residual correlations between observed and reproduced matrices are close to 0, indicating good model fit. What to Look For: Low residual correlations (