Psychometrics Podcast PDF
Document Details
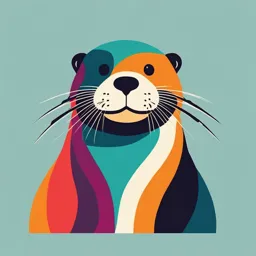
Uploaded by MagicalWoodland4124
National University of Singapore
Tags
Summary
This podcast, presented as a PDF, introduces the concepts of classical psychometrics, including reliability and validity. It covers various aspects of measurement theory, describes different measurement models (like reflective latent variable modeling), and provides examples of how to quantify psychological constructs. The document explains concepts like construct validity, criterion validity, convergent validity, and discriminant validity, as well as different approaches to modeling (like factor and item response theory).
Full Transcript
Classical Psychometrics & Reliability Psychometrics Construct Validity Construct validity is the extent to which a measure (or test) actually measures/tests what it claims, or purports, to be measuring There are two subjective ways of assessing construct validity Face Validity, which asks if...
Classical Psychometrics & Reliability Psychometrics Construct Validity Construct validity is the extent to which a measure (or test) actually measures/tests what it claims, or purports, to be measuring There are two subjective ways of assessing construct validity Face Validity, which asks if the measure looks like it is measuring what it is supposed to measure Content Validity, which asks if the measure covers all aspects of the construct In addition, we can also use empirical ways of assessing construct validity Criterion Validity asks if the measure correlates with the relevant behaviour In Concurrent Criterion Validity, the variables are all measured at the same time, and checked if they correlate with the relevant behaviour In Predictive Criterion Validity, the variables are measured at different times, and checked if they correlate with the relevant behaviour Convergent Validity asks if the measure correlates with other measures of the same construct Discriminant Validity asks if the measure does not correlate with measures of other constructs Classical Test Theory The Classical Test Theory (CTT) is a framework for understanding test scores, usually sum scores It is based on the idea that a test score (often a sum score) is the sum of a true score and an error score A test is a measure used to quantify a psychological construct In CTT, this is often a sum of items A true score is defined as an expectation of the test score Meaning, the average score a person would get if we would repeat the test in the exact same setting an infinite number of times The true score is not known, and therefore the error is also not known The true score is not necessarily a valid measure of the construct Any bias associated with the test will affect the true score Reliability We do not know the true score, and therefore we cannot know reliability. The best we can try to do is to estimate reliability Estimating Reliability: Parallel Tests (Alternate Form) It is hard to come up with a completely new parallel / alternate test Instead, CTT focused on several ways of estimating reliability using the same test In Test-Retest Reliability, the same test is administered twice to the same participants The same test administered at a later time point is considered the parallel test But, there might be learning effects, developmental changes, and/or the errors might be correlated In Split-Half Reliability, the test is split in two (e.g. even items and odd items) One half can be treated as a parallel test for the other half Correlation between the two halves is adjusted for the halves being shorter than the entire test (Spearman-Brown formula) But, the two halves should be as similar as possible Estimating Reliability: Internal Consistency Another common way of estimating reliability is by assessing internal consistency Here, all items of the test are considered parallel tests Internal consistency is assessed by the extent to which items of a test correlate with each other This is done by investigating the correlation matrix of the items The most common measure of internal consistency is Cronbach’s Alpha, which gives the reliability given the assumptions that All items are completely exchangeable (tau-equivalent) The variance of and the correlation between all items is the same Example: Modern Psychometrics: Latent Variables & Networks Reflective Latent Variable Modelling In CTT, we typically focus on the test scores only, the true score is an expectation and is not the construct of interest CTT also does not distinguish between binary tests (exams) and continuous tests (surveys) In modern psychometrics, we instead focus on latent variable modelling A latent variable represents the construct of interest, and we assume that it causes the observed variables We can model the relationship between the latent variable and the observed variables We can also model the relationship between latent variables This allows for much more flexibility in modelling and more accurate representation of the construct of interest Example: This is a reflective measurement model, as the observed variable is reflected in the model To model a latent variable, for statistical reasons, we need at least three observed variables. The assumptions we make when modelling a latent variable are Local Independence, where the latent variable (factor) explains all correlations between the observed variables (indicators) Unidimentionality, where only one latent variable (factor) underlies the observed variables (indicators) Factor Analysis & Item Response Theory Two separate frameworks have been developed for modelling reflective latent variables Factor Analysis, for continuous indicators in surveys/questionnaires Item Response Theory, for binary (yes/no) indicators in tests (e.g. exams) These frameworks differ from CTT in that Analysis is at the item level Items are not interchangeable Biases in items/tests can be modelled and accounted for Benefits of Latent Variable Modelling Allows for testing if a latent common cause is plausible (e.g. unidimensionality) Provides more accurate estimates of the latent variables Allows hypothesis testing at the latent level without the need for test scores Provides better reliability estimates such as McDonald’s Omega Essentially, IRT lets you model the probability of getting a correct response on an item, for any given ability level Structural Equation Modelling (SEM) Structural Equation Modelling (SEM) allows for also modelling (causal) relationships between latent variables Factor analysis is a special case of SEM Formative constructs do not cause the indicators, but is defined by the indicators For example, SES is defined by income, occupation, years of education, rather than causing them Formative constructs do not need to be unidimensional Formative constructs are usually composite scores (e.g. sum scores) and are defined rather than estimated However, there are measures for estimating an optimal composite score Principle Component Analysis (PCA) aims to find the linear combination of variables that explains the most variance, used as a way to reduce the number of variables to explain the most variance Multiple-Indicator, Multiple Cause Model (MIMC) models combine reflective and formative indicators The Network Perspective The network perspective sees psychological constructs as networks of interacting components Based on this idea, Network Psychometrics concerns estimating network structures from data Similar to EFA, but with a focus on the unique interactions between observed variables, rather than the latent variables Network model estimation methods have been developed for cross-sectional and longitudinal studies