Correlation and Regression Lecture PDF
Document Details
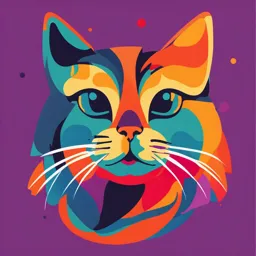
Uploaded by FriendlyEinsteinium
Tags
Summary
This lecture covers correlation and regression analysis. It explores different types of correlation, including positive and negative correlations, and explains how to interpret the results of a correlation analysis.
Full Transcript
Correlation Correlational Research Designs Example Murders Ice Cream Example Murders Ice Cream Or… Murders Ice Cream Correlation Variable X Variable Y Or… Variable X...
Correlation Correlational Research Designs Example Murders Ice Cream Example Murders Ice Cream Or… Murders Ice Cream Correlation Variable X Variable Y Or… Variable X Variable Y Or… Correlation: Variables X & Y Variable X Variable Y Or… Variable X Variable Y Or… Correlation: Variables: X, Y, & Z An unknown Variable Z is responsible for the relationship between Variables X & Y Variable X Variable Y Variable Z Positive Correlation Variable X Variable Y Positive Correlation Variable X Variable Y Negative Correlation Variable X Variable Y Negative Correlation Variable X Variable Y Correlation With Correlation, we may never state that variable X causes a change in variable Y Likewise, we may never state that variable Y causes a change in variable X Correlation With Correlation, we may never infer causation! There is never a cause & effect relationship with correlation Correlation Acceptable terminology for use in a correlation: *Correlation between variables *Relationship between variables *Association between variables Never a difference between variables or a cause and effect relationship between variables Pearson’s r Correlation rcalc = +number, positive correlation rcalc = -number, negative correlation rcalc = +1, a perfect positive correlation rcalc = -1, a perfect negative correlation rcalc = 0, no correlation at all rcalc must be between (-1 to +1) Statistical Assessment of Relationships Pearson’s r – for quantitative dependent variables (interval & ratio) Spearman Rank Order Correlation Coefficient – for ordinal dependent variables Simple Linear Regression A Correlational Design Simple Linear Regression Used for developing an equation that can be used for predicting the values of a specified Dependent Variable (DV). Simple Linear Regression uses one predictor variable and one dependent variable. X Predictor Variable Y Dependent Variable Data with Scatterplot Exam I Exam II Person X Y 1 34 37 2 25 22 3 15 15 4 17 16 Scatterplot with Regression Line Exam I Exam II Person X Y 1 34 37 2 25 22 3 15 15 4 17 16 Simple Linear Regression The Linear Regression Formula is: Y = bX + a Where: b = slope of regression line a = where regression line intercepts the y axis (Y-Intercept) You may “plug-in” any score from the X-axis (X variable) to predict the score on the Y-axis (Y variable) Y = #X ± #