Climate Change Summary PDF
Document Details
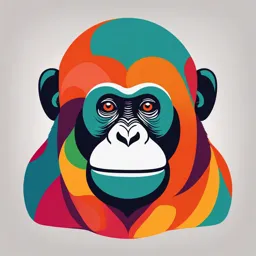
Uploaded by HappierIris
Tags
Summary
This document provides a summary of climate change observations, data, and models. It covers topics like weather observations, remote sensing, and future developments. The text also touches upon the roles of weather models and climate models in short- and long-term predictions, respectively.
Full Transcript
Lec 2 Introduction to Weather Observations: Weather observations have evolved from traditional knowledge (e.g., lunar cycles like the Lunda calendar) to systematic, instrumental measurements. Early instruments measured basic variables such as temperature and pressure. Over time, g...
Lec 2 Introduction to Weather Observations: Weather observations have evolved from traditional knowledge (e.g., lunar cycles like the Lunda calendar) to systematic, instrumental measurements. Early instruments measured basic variables such as temperature and pressure. Over time, global observation networks were developed, integrating surface and upper-air measurements. Current Observation Networks: Surface Network: 1023 stations globally. Upper Air Network: 171 stations with uneven coverage, especially in parts of Africa where data is sparse. Challenges: ○ Many stations have stopped reporting. ○ A mix of automatic weather stations, weather balloons, ship observations, ocean buoys, and aircraft data are integrated using the Global Telecommunications System (GTS). Remote Sensing Development: Satellite Observations began in 1976 using electromagnetic radiation to measure: ○ Visible light (0.4-0.7 microns) ○ Infrared (3-14 microns) ○ Microwaves (5-500mm) Active Remote Sensing (RADAR and LIDAR) is used to measure: ○ Rainfall and sea surface height (with RADAR). ○ Aerosols and atmospheric winds (with LIDAR). Satellite Orbits: Different orbits (e.g., polar vs. geostationary) provide trade-offs between spatial and temporal coverage. Data Challenges: Observations are heterogeneous: varying protocols, temporal and spatial coverage. The biggest challenges include: ○ Uncertainty in measurement types, temporal gaps, and changing protocols. ○ Data merging is crucial to create long-term, consistent records. Future Developments in Observations: Data Assimilation integrates observations with 4D models (latitude, longitude, height, and time) to improve weather predictions. Machine Learning (ML) can handle large-scale data, optimizing forecasts, but needs careful attention to data quality and uncertainty. Critical Role of Data Pipelining: Managing data flow for real-time use is key for accurate predictions and climate forecasting. 1. Monsoon Overview: ○ Monsoon is a mixture of heavy and steady rain. ○ The Indian Monsoon plays a crucial role in South Asia’s climate, bringing vital rainfall during the summer months. ○ This rain is vital for agricultural, economic, and cultural activities in the region. 2. Wet and Dry Belts: ○ Wet Belt and Dry Belt curves are important in understanding how clouds form, especially in relation to the Indian Monsoon. These belts define areas of high and low moisture content and precipitation potential. 3. Satellite Movement: ○ Satellites closer to the Earth move faster to counteract the stronger gravitational pull. ○ Satellites farther away move more slowly, as the gravitational pull is weaker. 4. Types of Waves: ○ RADAR: Uses radio waves (microwave spectrum). ○ LIDAR: Uses laser light (optical spectrum). 5. Applications of RADAR and LIDAR: ○ RADAR: Used for sea surface height measurement, rainfall detection, and weather radar systems. Its ability to penetrate clouds makes it suitable for large-scale weather monitoring and precipitation detection. ○ LIDAR: Commonly used for aerosol measurement, upper-atmosphere wind measurement, and surface mapping (e.g., forests, ocean winds). Provides high spatial resolution, making it suitable for atmospheric measurements and surface characteristics. 6. Comparison: ○ RADAR is best for large-scale weather monitoring, particularly related to water phenomena such as ocean surfaces and rainfall. ○ LIDAR excels in precise atmospheric measurements, especially for detecting aerosols and upper-atmosphere winds. Lec 1 December (Panel a): Convective activity is concentrated over southern Africa, with the ITCZ shifted southward during the austral summer. August (Panel b): Convective activity is over northern tropical Africa, driven by the northward shift of the ITCZ during the boreal summer. Decreasing Global Temperatures Due to the Himalayas: Gradual cooling occurred due to CO₂ reduction from weathering. The Milankovitch Cycles are long-term variations in Earth's orbital characteristics that affect the amount and distribution of solar energy (insolation) received by the planet, driving natural climate changes over tens of thousands to hundreds of thousands of years. These cycles are named after Serbian scientist Milutin Milankovitch, who studied their role in Earth's ice ages. Lake Kariba is the largest man-made reservoir in the world by volume. It is located on the border between Zambia and Zimbabwe along the Zambezi River. It supplies electricity to both Zambia and Zimbabwe. 6 Turbines: 3 in Zambia (Kariba North Power Station), 3 in Zimbabwe (Kariba South Power Station). Current Operation: At times, only 1 turbine runs in Zambia due to low water levels or maintenance. Crops failed 2023 2023 the lowest this river have ever been And same for 2024 Rain Formation: Warm, moist air near the surface must rise to cool and condense into rain. Tropics: Strong upward motion due to thunderstorms leads to heavy rainfall. Subtropics & Deserts: Air sinks, causing clear skies and making rain formation difficult September: The Sun is directly overhead in West Africa near the equator during the autumnal equinox. Southward Movement: The Sun moves south toward the Tropic of Capricorn, marking seasonal shifts. Rain try to follow the sun End of the Century: The Sahara may become wetter, while Southern Africa could become drier. Rainy Season: Expected to be delayed, impacting agriculture and water availability Rain start in zambia the red pattern (2001–2017), the cloud bands shift downward, meaning: Delayed Rainy Season: The downward shift delays the onset of rain, as the cloud bands are critical for transporting moisture and initiating rainfall. 1.2 cloud band every year less 2.3 cloud bands less in madagascar Madagascar – Drought & Tropical Cyclone northern part of madagascar get cyclone Lecture 2 Monsoon a mixture of heavy and steady rain the Indian Monsoon is a crucial climatic feature that brings much-needed rainfall to the region, especially during the summer months, and is a key factor in the agricultural, economic, and cultural activities of South Asia. Wet belt close to dry bely curve the clouds form The closer a satellite is to Earth, the faster it moves in order to counteract the stronger gravitational pull. The farther away it is, the slower it moves. Type of Waves: RADAR uses radio waves (microwave spectrum). LIDAR uses laser light (optical spectrum). Applications: RADAR is widely used for sea surface height measurement, rainfall detection, and weather radar systems. LIDAR is commonly used for aerosol measurement, atmospheric wind measurement (upper atmosphere), and surface mapping like forests or ocean winds. RADAR is better suited for large-scale weather monitoring, ocean surface measurements, and precipitation because it can penetrate clouds and other atmospheric conditions, giving it an advantage for detecting water-related phenomena. LIDAR, on the other hand, is used for precise atmospheric measurements, including the detection of aerosols, upper-atmosphere winds, and other surface characteristics that require high spatial resolution. There is rain in subtropical it is not completely desert Heavy rainfall in tropics West and South Africa is dry compare to them Congo rainfall In the suptroic rain limited like deserts In extra tropic like Europe Rainfall is distributed more evenly throughout the year Sahara desert Annual Precipitation: Less than 100 mm, often as low as 25 mm in central regions. Lecture 3 Weather Prediction (IVP): Focuses on short-term forecasting, starting with a set of initial conditions and predicting future states of the system (e.g., weather for the next few days). Climate Modeling (BVP): Focuses on long-term predictions, using boundary conditions that define the system's state over extended periods (e.g., decades or centuries), such as CO2 concentrations and solar radiation. Weather Models: Focus on short-term forecasting (hours to days) based on initial conditions. They are critical for daily weather predictions and managing immediate weather-related risks. Climate Models: Focus on long-term trends (decades to centuries) driven by changes in boundary conditions (e.g., atmospheric composition). These models are used to predict long-term climate changes, including global warming. Earth’s habitability is due to : It’s distance from the sun Size, which controls its atmospheric composition The scientists stated: There is no doubt that the planet is warming, and human activities are the primary cause. Solar radiation is mostly shortwave and comes from the Sun at a temperature of 6000 K. It includes ➕ visible light (which is visible to the human eye) and infrared radiation (which is not visible to the human eye but can be felt as heat). Shortwave very hot Earth radiation is longwave infrared radiation, emitted by Earth at a temperature of 288 K. This radiation is mostly in the infrared spectrum and is not visible to the human eye. Longwave-> cooler The Earth absorbs shortwave solar radiation (including visible and infrared) and re-emits it as longwave infrared radiation, contributing to the Earth's energy balance. Simplest Mathematical Model for Climate: 1. Energy Balance: The model equates the incoming solar radiation (LHS) to the thermal radiation emitted by Earth (RHS). 2. Solar Radiation (LHS): Represents the solar energy absorbed by Earth, dependent on: ○ Cross-sectional area of Earth. ○ Solar constant (solar energy per unit area). ○ Albedo (fraction of sunlight reflected). 3. Earth's Thermal Radiation (RHS): Represents the energy emitted by Earth, dependent on: ○ Total surface area of Earth. ○ Stefan-Boltzmann constant. ○ Effective temperature of Earth. 4. Effective Temperature Calculation: Balance the incoming solar energy (LHS) with the outgoing thermal radiation (RHS) to calculate Earth's effective temperature Albedo: Measures reflected energy (e.g., ice, clouds, white sand reflect; black sand absorbs). Basic Model Limitation: Predicts -20°C, but Earth's actual average is 15°C due to the atmosphere trapping heat via the greenhouse effect. Need for Atmosphere: To accurately model Earth's temperature Greenhouse Gases and Shortwave Radiation: Most greenhouse gases (like CO₂, CH₄, and H₂O vapor) do not absorb shortwave radiation from the Sun. Instead, this solar radiation passes through the atmosphere and directly heats Earth's surface. Earth’s Emission and Longwave Radiation: The Earth absorbs the Sun’s shortwave radiation, warms up, and re-emits energy as longwave radiation (infrared). Greenhouse gases absorb and trap this longwave radiation, contributing to the greenhouse effect. Ozone Exception: Ozone (O₃), found primarily in the stratosphere, absorbs shortwave ultraviolet (UV) radiation from the Sun, protecting life on Earth by reducing harmful UV exposure. The research over the last century has shown a clear connection between increasing CO₂ concentrations and rising global temperatures. The early work by scientists like Tyndall, Arrhenius, and Callendar provided key insights into how human activities affect the Earth's climate system, reinforcing the importance of addressing CO₂ emissions. Net radiation (R) is the balance between the incoming short-wave radiation and the outgoing long-wave radiation. The net radiation shows a relatively small asymmetry between land and sea areas, but it is more significant in desert regions. The latitudinal variation of short-wave radiation (S ) is much larger than the long-wave radiation (L ), and it dominates the net radiation (R). The zonal asymmetry (difference between land and sea) in net radiation is small. Desert areas: Desert regions, particularly over land, have low net radiation due to high long-wave loss, even though these areas are located at latitudes that would typically receive higher radiation. Lecture 4 At the Equator: Speed: Highest (1,670 km/h). Coriolis Effect: Weakest (minimal deflection). Momentum Deflection: Minimal due to weak Coriolis force despite high speed. At the Poles: Speed: Zero (no lateral movement due to Earth's rotation). Coriolis Effect: Strongest (maximum deflection). Momentum Deflection: Maximum due to strong Coriolis effect despite zero rotational speed. No spin at all The pressure gradient force moves air from high pressure to low pressure. The Coriolis effect deflects the air to the right (or left), and when it balances the pressure gradient force, the air starts moving parallel to the isobars (not from low to high pressure). The Coriolis force deflects moving air to the right in the Northern Hemisphere and to the left in the Southern Hemisphere. It acts perpendicular to the direction of motion (at a 90° angle to the movement). this balance between the pressure gradient force and the Coriolis force holds even stronger due to the greater density of water compared to air. The Geostrophic Approximation is useful for understanding the movement of large-scale wind systems, as it assumes a balance between the Coriolis force and pressure gradients. This equation represents the quasi-geostrophic approximation that simplifies the dynamics of large-scale flows by balancing the Coriolis force with the pressure gradient force and assuming certain conditions. The approach makes it easier to study large-scale weather systems and ocean currents, and the linearization helps find simpler analytical solutions to the equations governing fluid motion. This is the starting point for much of the linearization required to pursue analytical solutions to the equation The Barotropic Rossby Wave equation describes large-scale atmospheric motions. The first numerical weather forecast was based on this equation and run on the ENIAC computer, marking a major step in the development of numerical weather prediction. These equations are critical in understanding atmospheric and oceanic behavior, and they form the foundation for numerical weather prediction (NWP) models that simulate weather patterns and phenomena. Each equation is associated with a physical principle: Navier-Stokes equations: Momentum and fluid flow. Thermodynamic energy equation: Energy conservation. Continuity equation: Mass conservation. Ideal gas law: Thermodynamic state of the fluid. conservation: Water vapor or moisture in the atmosphere. Discretizing the primitive equations on a grid allows for numerical simulations of weather and climate systems. The accuracy of these simulations depends heavily on the grid's resolution and how physical processes are handled in the model. Finer Grids: Finer grids slow down the model due to more grid points, higher computational demand, and smaller time steps. Larger Grids: Larger grids are faster and more efficient, requiring fewer calculations While larger computers, finer grids, and smaller time steps can enhance the detail and precision of weather models, they do not guarantee better forecasts. This is because weather systems are non-linear and highly sensitive to initial conditions Both weather and climate are non-linear dynamical system The Lorenz equations provide a mathematical model for chaotic systems. Their non-linear nature and sensitivity to initial conditions are key features that contribute to the unpredictability of long-term forecasts, as seen in weather systems. The ensemble forecast is used to account for uncertainties in the initial conditions. Instead of relying on a single forecast, multiple model runs are made, each with slightly different initial conditions. This approach provides a range of possible outcomes and gives an idea of the uncertainty associated with the forecast. Lecture 5 Boundary forcing: Solar energy (approx. constant) Atmospheric Composition Greenhouse Gases increasing Aerosol emmissions fluctuating Boundary forcing changes the probabilities of the weather Forcing of monsoons related to aerosols example Aerosols Cooling Reflects sunlight, reducing temperature. Cloud Alters cloud reflectivity and lifespan. Changes Precipitation Can disrupt weather patterns and cause Changes droughts. Acid Rain Damages ecosystems and infrastructure. Health Risks Causes respiratory and cardiovascular diseases. (pollution) Ozone Depletion Contributes to stratospheric ozone loss. Direct Sunlight vs. Curvature Effect: Direct Sunlight (Near the Equator): ○ Locations near the equator receive more heat because sunlight strikes the Earth directly (at a near 90-degree angle). ○ The Sun's energy is concentrated over a smaller surface area, making the equatorial regions warmer. ○ Example: The tropics receive the most heat due to their position. Curvature Effect (Toward the Poles): ○ At higher latitudes (closer to the poles), sunlight hits the Earth at an angle, spreading the energy over a larger surface area. ○ This leads to less heat absorption, making polar regions colder. ○ Example: The Arctic and Antarctic regions receive less energy per unit area due to the slanting rays. Why Some Places Receive More Heat: Due to Earth's curvature, the angle of incoming sunlight varies across different latitudes: 1. Equator (0° latitude): Sunlight is direct and intense. 2. Mid-latitudes (30°-60°): Sunlight arrives at an angle, reducing heat intensity. 3. Poles (above 66.5°): Sunlight is very oblique, spreading the energy over a wide area and contributing to colder temperatures. What is Turbulence? 1. It is the irregular or chaotic movement of air, often caused by obstacles such as buildings, trees, or uneven terrain. 2. Unlike steady wind, turbulence creates gusts – short bursts of wind that seem to come and go unexpectedly. Processes vary in space and time: Some weather events (e.g., thunderstorms) happen over a short time and small area, while others (e.g., global climate change) occur over large areas and long periods. Different models for different scales: Because processes occur at different scales, scientists use specific models to study each one separately. Challenge in global climate modeling: Climate models need to consider all these processes together, which makes them complex. Boundary forcing refers to external influences that affect the Earth's climate. These include: 1. Solar Energy: ○ The Sun provides nearly constant energy to the Earth, which drives weather and climate systems. ○ Small variations in solar output can influence climate over long periods. 2. Atmospheric Composition: ○ Changes in the gases and particles in the atmosphere alter how heat is trapped and reflected. ○ Key components include: Greenhouse Gases (e.g., CO₂, CH₄): Increasing levels trap more heat, contributing to global warming. Aerosols (e.g., dust, pollution): These can reflect sunlight back into space, cooling the atmosphere, or absorb heat and warm the air. uman activities (aerosol pollution) contributed significantly to rainfall decline in the Sahel. Regulatory policies (Clean Air Acts) helped restore atmospheric balance and allowed rainfall to recover. Climate models (CMIP6) confirm the role of aerosols in modifying regional climate patterns. Website Summary of El Niño 1. What is El Niño? ○ El Niño is a climatic phenomenon that occurs when the surface waters in the central and eastern Pacific Ocean become warmer than usual, disrupting atmospheric and oceanic patterns. 2. Key Changes During El Niño: ○ Weakened or Reversed Trade Winds: Easterly winds (blowing from the Americas to Asia) weaken or reverse, turning into westerlies, pushing warm water eastward toward the Americas. ○ Reduced Upwelling: The warm water suppresses the rise of cold, nutrient-rich water from deep in the ocean, impacting marine ecosystems and fisheries. ○ Shifts in Atmospheric Circulation: High-pressure systems in the eastern Pacific weaken, leading to changes in atmospheric pressure patterns. 3. Climate and Weather Impacts: ○ Droughts: Southeast Asia, Australia, and parts of Africa experience reduced rainfall and drought conditions. ○ Flooding: South American coasts see heavy rainfall and flooding, affecting agriculture and infrastructure. ○ Storm Patterns: Increased tropical cyclones in the Pacific and reduced hurricane activity in the Atlantic. 4. Marine and Ecological Effects: ○ Decline in fisheries due to lower nutrient availability. ○ Coral bleaching and habitat loss for marine species. 5. Global Influence: ○ Disruptions to global weather patterns, affecting agriculture, water resources, and economies worldwide. ○ Teleconnections: El Niño's effects extend far beyond the Pacific, influencing weather in distant regions. 6. Frequency and Duration: ○ Occurs every 2 to 7 years, typically peaking between November and January, with impacts lasting for months. 7. El Niño vs. La Niña: ○ El Niño brings warming, while La Niña represents a cooling phase with opposite effects, often leading to droughts in the Americas and increased rains in Asia. El Niño is considered one of the most influential climate patterns globally, with wide-ranging environmental, social, and economic consequences. paper 1. The Challenge of Conducting Experiments in Earth Sciences Problem: ○ Large-scale experiments on Earth systems (e.g., climate) are infeasible or ethically problematic. ○ Anthropogenic climate change itself is an uncontrolled long-term experiment. Alternative Approach: ○ Computer simulations are widely used but are expensive, time-consuming, and require expert knowledge with strong assumptions. ○ The rise of big data (satellite, field, and model outputs) allows for new data-driven causal inference methods without physical interventions. 2. Advances in Causal Inference Methods Recent progress in fields like computer science, physics, and statistics has led to methods that infer causal relationships from observational data. Key concept: 1. Reichenbach’s common cause principle: If variables are dependent, they either have a direct causal link or share a common driver. Methods discussed: 1. Granger Causality (GC): Predictive approach for identifying causal relationships over time. 2. Nonlinear Dynamics: Reconstructing system behavior from data patterns. 3. Structural Causal Models (SCMs): Statistical framework for causal analysis. 4. Machine Learning Applications: Uses statistical independence to extract causal dependencies. 3. Importance of Causal Inference vs Traditional Methods Traditional methods like correlation and regression are commonly used but do not reveal true causal relationships. Causal inference provides: ○ A better understanding of system dynamics. ○ The ability to separate direct causes from indirect ones. Example: ○ Deep learning models can predict but not explain causality, whereas causal inference methods provide interpretability. 4. Applications of Causal Inference in Earth Sciences Example 1: Walker Circulation (Climate Science) Discovered relationships between temperature and pressure in the Pacific Ocean. Using causal inference (e.g., PCMCI method) helps avoid incorrect links and better interpret climate data. Example 2: Arctic Teleconnections (Mid-Latitude Extreme Winters) Arctic sea ice extent influences mid-latitude winters. Causal inference confirmed model predictions, showing the importance of Arctic conditions in shaping weather patterns. Example 3: California Current Ecosystem (Ecology) Sardine and anchovy populations are driven by sea surface temperature. Causal state-space models identified ecological relationships that regression analysis could not. 5. Challenges and Future Directions Challenges: ○ Lack of awareness and adoption of causal methods in Earth sciences. ○ Resistance due to traditional reliance on correlation-based approaches. Future Directions: ○ Bridging the gap between method developers and Earth science practitioners. ○ Integrating causal inference with physical climate models. ○ Encouraging open platforms (e.g., CauseMe.net) for benchmarking causal methods. Key Takeaways for Exam Preparation 1. Why causal inference is important: It helps distinguish correlation from causation in complex Earth systems. 2. Main methods used: Granger Causality, Structural Causal Models, Nonlinear Dynamics. 3. Applications: Climate, Arctic ice influence, ecological systems. 4. Limitations of traditional methods: Correlation is not causation; causal inference provides better insights. 1. Conceptual Understanding Questions Q1: What is causal inference, and why is it important in Earth system sciences? Answer: Causal inference is the process of identifying cause-and-effect relationships between variables, rather than just statistical correlations. In Earth system sciences, it is crucial to understand causation because: It helps identify the true drivers of climate variability and environmental changes. It informs climate models and policy decisions to address environmental challenges effectively. It provides insights into interactions between atmospheric, oceanic, and terrestrial systems. Q2: Explain the difference between correlation and causation. Why is correlation alone not sufficient in climate studies? Answer: Correlation: Measures the statistical relationship between two variables but does not imply causation (e.g., ice cream sales and drowning rates both increase in summer, but one does not cause the other). Causation: Establishes a direct cause-and-effect relationship between variables. Correlation alone is insufficient in climate studies because: Climate systems are complex and influenced by multiple interconnected factors. Correlation can be misleading due to hidden confounding variables. Decision-making based on correlation alone can lead to ineffective or harmful policies. Q3: What challenges make it difficult to perform interventional experiments in Earth system sciences? Answer: 1. Ethical concerns: Deliberately altering the Earth's atmosphere or ecosystems is risky. 2. Practical limitations: Large-scale experiments on climate systems are costly and technically challenging. 3. Complexity of Earth systems: Multiple interacting components make it hard to isolate individual effects. 4. Long time scales: Climate processes operate over decades or centuries, making controlled experiments impractical. Q4: How does causal inference complement traditional climate models? Answer: Causal inference helps climate models by providing data-driven insights into cause-effect relationships. It reduces uncertainties in models by identifying key influencing factors. It allows better understanding of feedback mechanisms in the Earth system. 2. Methods and Techniques Questions Q5: Describe the main causal inference methods discussed in the paper. Answer: 1. Granger Causality: ○ Checks if past values of one variable improve the prediction of another. ○ Example: If past sea surface temperatures can predict future rainfall. 2. Nonlinear State-Space Models: ○ Captures complex relationships using attractor-based models. ○ Useful for dynamic systems like ocean-atmosphere interactions. 3. Causal Network Learning Algorithms: ○ Builds networks to determine relationships among multiple variables. ○ Helpful in understanding large-scale climate patterns. 4. Structural Causal Models (SCMs): ○ Uses graphs to represent causal relationships mathematically. ○ Helps in scenario analysis and policy evaluation. Q6: Explain the principle of Granger causality and provide an example of its application. Answer: Granger causality states that if the past values of variable XXX help predict the future values of variable YYY, then XXX "Granger-causes" YYY. Example: If past ocean temperatures can be used to improve predictions of El Niño events, then ocean temperatures Granger-cause El Niño. Q7: What is the difference between Granger causality and structural causal models (SCMs)? Answer: Granger causality is a statistical test used to predict one variable from another in a time series context. SCMs go beyond time series and represent causal relationships using equations and directed acyclic graphs (DAGs) to allow for counterfactual reasoning and interventions. 3. Applications and Case Studies Questions Q8: Explain how causal inference methods have been applied to study the Walker circulation. Answer: The study of Walker circulation using causal inference revealed pressure-temperature relationships between the east and west Pacific. Granger causality and advanced causal models helped confirm the role of sea surface temperatures in driving atmospheric circulation patterns related to El Niño-Southern Oscillation (ENSO). Q9: What insights have causal inference techniques provided about Arctic teleconnections and their impact on mid-latitude weather? Answer: It was found that autumn Arctic sea ice extent influences winter weather in the mid-latitudes. The reduced ice cover affects the stratospheric polar vortex, leading to extreme winters in Eurasia and North America. Q10: Discuss the role of causal inference in understanding the California Current ecosystem (sardine and anchovy population dynamics). Answer: Causal models identified sea surface temperature as a common driver for sardine and anchovy populations. Traditional regression methods failed to capture the complex nonlinear interactions, whereas causal inference revealed meaningful relationships. 4. Challenges and Limitations Questions Q11: What are the major challenges in applying causal inference to Earth system sciences? Answer: 1. Data quality issues: Climate data can be noisy and incomplete. 2. Complexity: Earth systems have multiple interacting processes. 3. Computational cost: Advanced methods require significant computing power. 4. Confounding factors: Difficult to isolate true causal relationships. Q12: How does data quality impact causal inference results in climate studies? Answer: Incomplete or biased data can lead to incorrect conclusions about causality. Poor data resolution may miss key causal patterns. Data preprocessing and careful validation are essential to ensure accuracy. 5. General Knowledge and Critical Thinking Questions Q13: What role does machine learning play in causal inference for Earth systems? Answer: Machine learning helps identify patterns and relationships within large climate datasets. It complements causal inference by automating variable selection and improving model accuracy. However, it lacks interpretability compared to traditional causal methods. Q14: How can causal inference methods contribute to climate change mitigation and policy-making? Answer: They provide evidence-based insights for decision-makers. Help in designing targeted interventions (e.g., emission reduction strategies). Enhance climate adaptation planning by understanding long-term trends. Q15: Compare the effectiveness of traditional climate models versus data-driven causal inference methods. Answer: Aspect Traditional Climate Causal Inference Methods Models Basis Physical equations Observational data relationships Complexity High Lower Interpretability Good Better in understanding causality Computational Very high Moderate to high Cost Flexibility Low High