Multivariate Linear Regression Lecture 5, 2024
Document Details
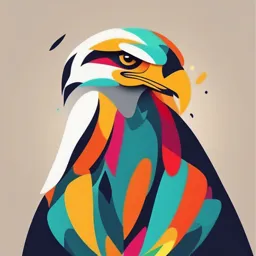
Uploaded by JollyMoldavite4497
Universitat Pompeu Fabra
2024
Tags
Summary
Lecture notes for a multivariate linear regression course, focusing on econometrics concepts and omitted variable bias; helpful for students of econometrics.
Full Transcript
Lecture 5: Multivariate Linear Regression 25117 - Econometrics Universitat Pompeu Fabra October 16th, 2024 What we learned in the last lesson - Hypothesis testing for regression coefficients is analogous to hypothesis testing for the population mean: Use the...
Lecture 5: Multivariate Linear Regression 25117 - Econometrics Universitat Pompeu Fabra October 16th, 2024 What we learned in the last lesson - Hypothesis testing for regression coefficients is analogous to hypothesis testing for the population mean: Use the t-statistic to calculate the p-values and either accept or reject the null hypothesis. Like a confidence interval for the population mean, a 95% confidence interval for a regression coefficient is computed as the estimator ±1.96 standard errors. - When X is binary, the regression model can be used to estimate and test hypotheses about the difference between the population means of the “X = 0” group and the “X = 1” group. - In general, the error ui is heteroskedastic; that is, the variance of u at a given value of Xi , var (ui | Xi = x, depends on x. A special case is when the error is homoskedastic; that is, when var (ui | Xi = x) is constant. Homoskedasticity-only standard errors do not produce valid statistical inferences when the errors are heteroskedastic, but heteroskedasticity-robust standard errors do. Introduction References 2 / 35 What we learned in the last lesson - If the three least squares assumption hold and if the regression errors are homoskedastic, then, as a result of the Gauss–Markov theorem, the OLS estimator is BLUE. - If the three least squares assumptions hold, if the regression errors are homoskedastic, and if the regression errors are normally distributed, then the OLS t-statistic computed using homoskedasticity-only standard errors has a Student t distribution when the null hypothesis is true. The difference between the Student t distribution and the normal distribution is negligible if the sample size is moderate or large. Introduction References 3 / 35 Omitted Variable Bias (OVB) - If β1 is a causal effect, the first assumption of the Gauss-Markov Theorem must hold : E(ui | Xi ) = 0 for all i cov (X ,u) - Remember that β̂1 = β1 + var (X ) and β̂1 will be unbiased only if E( cov (X ,u) var (X ) ) = 0 - Example: - Private university graduates tend to have higher wages than public university graduates. Does that mean that private university education (X1 ) yields higher wages (Y )? - Maybe, but not necessarily — Private university graduates are selected by those institutions - They differ in a number of ways from public university graduates: they have on average better test scores in highschool, they come from wealthier families, etc. - All these relevant but unobserved variables may be omitted, and, if not accounted for, may bias the estimation of β1 - In comparing wages from private and public university graduates, how to identify the causal effect of education (X1 ) and differentiate it from the effect of, say, advantageous social background? - We want to make apple-to-apple comparisons... Introduction References 4 / 35 Omitted Variable Bias (OVB) The bias in the OLS estimator that occurs as a result of an omitted factor, or variable, is called omitted variable bias. For omitted variable bias to occur, the omitted variable Z must satisfy two conditions: 1 Z is a determinant of Y (i.e. Z is part of u); and 2 Z is correlated with the regressor X (i.e., corr (Z , X ) ̸= 0) Omitting Z results in OVB Z X Y Both conditions must hold for the omission of Z to result in omitted variable bias. Introduction References 5 / 35 Omitted Variable Bias (OVB) The bias in the OLS estimator that occurs as a result of an omitted factor, or variable, is called omitted variable bias. For omitted variable bias to occur, the omitted variable Z must satisfy two conditions: 1 Z is a determinant of Y (i.e. Z is part of u); and 2 Z is correlated with the regressor X (i.e., corr (Z , X ) ̸= 0) Omitting Z does not result in OVB Z X Y Both conditions must hold for the omission of Z to result in omitted variable bias. Introduction References 6 / 35 Omitted Variable Bias (OVB) The bias in the OLS estimator that occurs as a result of an omitted factor, or variable, is called omitted variable bias. For omitted variable bias to occur, the omitted variable Z must satisfy two conditions: 1 Z is a determinant of Y (i.e. Z is part of u); and 2 Z is correlated with the regressor X (i.e., corr (Z , X ) ̸= 0) Omitting Z does not result in OVB Z X Y Both conditions must hold for the omission of Z to result in omitted variable bias. Introduction References 7 / 35 Omitted Variable Bias (OVB) In the California Schools example, 1 Adults’ educational attainment may affect local kids’ test scores; and 2 Adults’ educational attainment is a driving force of local income, and, therefore, likely affects the share of subsidized meals Omitting Adults’ Education Adults’ may result in OVB Education Subsidized Test Meals Scores Again, both conditions must hold for the omission of Z to result in omitted variable bias. Introduction References 8 / 35 Omitted Variable Bias (OVB) There are systematic differences in educational attainment across districts with low and high share of subsidized meals. - Districts more educated adults have higher test scores - Districts less educated adults have more schools with subsidized meals - Among districts with comparable adults’ educational attainment, the effect of subsidized meals is generally smaller Above-median Below-median Difference in % Subsidized meals % Subsidized meals Test Scores 1 0 Y n Y n Y −Y t All Districts 720.2472 250 787.4612 250 67.214 15.0000 By Share w/ College Education: [0;.1339436] 710.3194 108 745.3444 18 35.025 2.9064 [.1350751;.1996677] 724.0533 90 758.4917 36 34.43834 4.2446 [.1997542;.3260502] 731.9475 40 774.4559 84 42.50845 5.2279 [.3309678 ;.7696404] 742.05 12 813.2955 112 71.24553 4.2628 Introduction References 9 / 35 Identifying Causal Effects - Here, we are interested in identifying the causal average treatment effect of the share of subsidized meals on test scores (we are not focused on prediction) - Causality implies understanding if changes in one variable cause changes in another irrespective of all other factors. - To achieve this goal, we want to make ceteris paribus comparisons - It is often useful to think of a causal effect as the one measured in an ideal randomized controlled experiment (RCT). - Randomized: subjects from the population of interest are randomly assigned to a treatment or control group (so there are no confounding factors) - Controlled: having a control group permits measuring the differential effect of the treatment - Experiment: the treatment is assigned as part of the experiment: the subjects have no choice, so there is no “reverse causality” in which subjects choose the treatment they think will work best. - In an ideal RCT, meal subsidies would be randomly allocated to schools, i.e., irrespective of any pre-existing differences across school districts. Introduction References 10 / 35 Identifying Causal Effects Clearly, this is not the case here: treatment and control groups differ in systematic ways. Above-median Below-median Difference in % Subsidized meals % Subsidized meals Test Scores 1 0 Y n Y n Y −Y t All Districts 720.2472 250 787.4612 250 67.214 15.0000 By Share w/ College Education: [0;.1339436] 710.3194 108 745.3444 18 35.025 2.9064 [.1350751;.1996677] 724.0533 90 758.4917 36 34.43834 4.2446 [.1997542;.3260502] 731.9475 40 774.4559 84 42.50845 5.2279 [.3309678 ;.7696404] 742.05 12 813.2955 112 71.24553 4.2628 Introduction References 11 / 35 Another way to see this Introduction References 12 / 35 Another way to see this Introduction References 13 / 35 What to do about it? - Randomization implies that any differences between the treatment and control groups are random — not systematically related to the treatment - From now on, one of the main goals of this course is to explore ways to recover causal treatment effects - Remember, we want to make ceteris paribus, apple-to-apple comparisons — not pear-to-apple, etc. - One way to do it is to control for systematic differences between control and treatment group - In our example, we want to know the average treatment effect of subsidizing school meals on test scores, holding the share of college education, average income, English proficiency, etc. constant (i.e., getting closer to all else equal, or ceteris paribus) - Said differently, we want to know the average impact of a change in subsidized meals on test scores within groups where college education, average income, English proficiency, etc. is the same Introduction References 14 / 35 The Multiple Regression Model Yi = β0 + β1 X1i + β2 X2i +... + βk Xki + ui with i = 1,... , n Where - Yi is the ith observation of the dependant variable - X1i , X2i ,..., Xki is the ith observation of the k th regressor - ui is the error term The population regression line is therefore given by: E(Yi | X1i = x1 , X2i = x2 ,..., Xki = xk ) = β0 + β1 x1 + β2 x2 +... + βk xk - βk is the slope of Xki - βk is the expected difference in Yi associated with a unit change in Xki holding all other Xkj {j̸=i} constant - β0 , the intercept, can be thought of as the coefficient on a regressor X0i that is equal to 1 for all observations. β0 is the expected value of Yi when all Xk i are equal to zero. Introduction References 15 / 35 Example for k = 2 Imagine we compare two schools, i and j ( Yi = β0 + β1 X1i + β2 X2i Yj = β0 + β1 X1j + β2 X2j ( Yi = β0 + β1 X1i + β2 X2i ⇒ Yi + ∆Yi = β0 + β1 (X1i + ∆X1i ) + β2 (X2j + ∆X2i ) ⇒ ∆Yi = β1 ∆X1i + β2 ∆X2i When X2 is constant, i.e., X2i = X2j , then ∆X2i = 0 and therefore, ∆Yi = β1 ∆X1i ∆Yi In general, holding all other Xkj {j̸=i} constant, ∆Xki = βk. Introduction References 16 / 35 The OLS Estimator in Multiple Regression As with simple regression, OLS estimators β̂0 , β̂1 , β̂2 ,..., β̂k solve n X min (Yi − b0 − b1 X1i − b2 X2i −... − bk Xki )2 b0 ,b1 ,b2 ,...,bk i=1 The derivative of the sum of squared prediction mistakes with respect to the jth regression coefficient, bj , is n ∂ X = (Yi − b0 − b1 X1i − b2 X2i −... − bk Xki )2 ∂bj i=1 n X ⇔ −2 Xji (Yi − b0 − b1 X1i − b2 X2i −... − bk Xki ) i=1 Combined, these yield the system of k + 1 equations that, when set to 0, constitute the first-order conditions for the OLS estimator, β̂ — the (k + 1) × 1 dimensional vector of the k + 1 estimates of the unknown regression coefficients Introduction References 17 / 35 The OLS Estimator in Multiple Regression The system of equation can be written as (with matricial notation) −2k +1 X ′ (Y − X β̂) = 0k +1 ⇒ X ′ Y − X ′ X β̂ = 0k +1 ⇒ β̂ = (X ′ X )−1 X ′ Y Where Y1 1 X11 X21... Xk1 β̂0 Y2 1 X12 X22... Xk2 β̂1 . ....... ; and β̂(k+1)×1 = . Yn×1 . ; = Xn×(k+1) = ....... . . ....... . Yn 1 X1n X2n... Xkn β̂k Introduction References 18 / 35 Example: The impact of Subsidized Meals on Test Scores Introduction References 19 / 35 Example: The impact of Subsidized Meals on Test Scores Introduction References 20 / 35 Goodness of Fit in Multiple Regression Remember that: Actual = Predicted + Residual (Yi = Ŷi + ûi ) In the previous lectures, we have seen that: - RMSE = std. deviation of ûi without d.f. correction - SER = std. deviation of ûi with d.f. correction - R 2 = share of the variance of Y explained by X without d.f. correction 2 Now, we will introduced the adjusted R 2 , written R 2 ⇒ R = share of the variance of Y explained by X with d.f. correction Introduction References 21 / 35 Goodness of Fit in Multiple Regression With multiple regressors, q P n - RMSE = n1 i=1 ûi2 q Pn - SER = n−(k1 +1) i=1 ûi2 Pn (Ŷi −Y )2 Pn û 2 - R2 = Pni=1 2 =1− Pn i=1 i 2 i=1 (Yi −Y ) i=1 (Yi −Y ) BUT whenever a variable is added to the RHS, the sum of squared residuals is (almost systematically) smaller (the OLS finds the values of the coefficients that minimize the sum of squared residuals) so the SSR ↓⇒ R 2 ↑ Introduction References 22 / 35 Goodness of Fit in Multiple Regression 2 The R , “adjusted” R 2 corrects this by penalizing the inclusion of an additional regressor: Pn 2 2 n−1 i=1 ûi R =1− Pn n − k − 1 i=1 (Ŷi − Y )2 Note that: 2 n−1 - R < R 2 because n−k−1 > 1 but when n → ∞ they become very close. n−1 - Adding one regressor ↓ SSR but also ↑ n−k−1 2 n−1 - R may be negative if the (many) regressors ↓ SSR but ↑↑↑ n−k−1 However, including a variable in a multiple regression should be based on whether including that variable allows you to better identify the causal effect of interest. Introduction References 23 / 35 The OLS Assumptions for Causal Inference in the Multiple Regression Model Yi = β0 + β1 X1i + β2 X2i +... + βk Xki + ui with i = 1,... , n where β1 ,... , βk are causal effects and 1 ui has a conditional mean of 0 given X1i , X2i ,... , Xki ; that is, E(ui | X1i , X2i ,... , Xki ) = 0 2 (X1i , X2i ,... , Xki , Yi ) are i.i.d. draws from their joint distribution. 3 Large outliers are unlikely: (X1i , X2i ,... , Xki , Yi ) have nonzero finite fourth moments 4 There is no perfect multicollinearity. ⇒ If assumptions 1-4 hold, then in large samples the OLS estimators β̂0 , β̂1 ,... , β̂k are jointly normally distributed, and each β̂j ∼ N (βj ; σβ̂2 ). j Introduction References 24 / 35 What is Multicollinearity? Multicollinearity refers to the high correlation between two or more independent variables in a regression model. - Perfect Multicollinearity: A linear relationship exists between independent variables (e.g., X3 = 0.5 × Share w/ College Education). - High Multicollinearity: High correlation but not a perfect linear relationship (e.g., ρ(Share w/ College Education; Median Income) = 0.8755). Introduction References 25 / 35 Example: Perfect Multicolinearity Introduction References 26 / 35 Example: High Multicolinearity Introduction References 27 / 35 Example: High Multicolinearity Introduction References 28 / 35 The dummy variable trap Suppose you have a set of multiple mutually exclusive and exhaustive variables — that is, there are multiple categories and every observation falls in one and only one category (Freshmen, Sophomores, Juniors, Seniors, Other). If you include all these variables and a constant (in general, the intercept), you will have perfect multicollinearity — this is sometimes called the dummy variable trap (as it often occurs with dummy variables). We can then decide to omit one of the groups (or the constant). In this case, the coefficients on the included binary variables represent the incremental effect of being in that category, relative to the base case of the omitted category. Introduction References 29 / 35 Example: Omitted category 1/2 Introduction References 30 / 35 Example: Omitted category 2/2 Introduction References 31 / 35 Control Variables in Multivariate Analysis Definition A control variable W is a regressor included to hold constant factors that, if neglected, could lead the estimated causal effect of interest to suffer from omitted variable bias. This definition means that 1 We need to modify the OLS assumptions such that the OLS estimator of the effect of interest is unbiased, but the OLS coefficients on control variables are, in general, biased and do not have a causal interpretation. 2 A good control variable is one which, when included in the regression, makes the error term uncorrelated with the variable of interest. 3 Holding constant the control variable(s), the variable of interest is “as if” randomly assigned. 4 Among individuals (entities) with the same value of the control variable(s), the variable of interest is uncorrelated with the omitted determinants of Y Introduction References 32 / 35 Conditional Mean Independance - Because a control variable is correlated with an omitted causal factor, the first OLS Assumption, E(ui | X1i , X2i ,... , Xki ) = 0 does not hold anymore - We need a mathematical condition for what makes an effective control variable. This condition is the conditional mean independence - Given the control variable, the mean of ui does not depend on the variable of interest - Question: Conditional on sharing the same exposure to adults’ educational attainment, is the share of subsidized meals as good as random? Introduction References 33 / 35 The OLS Assumptions for Causal Inference in the Multiple Regression Model with Control Variables Yi = β0 + β1 X1i + β2 X2i +... + βk Xki + βk+1 W1i + βk+2 W2i +... + βk +r Wri + ui with i = 1,... , n where β1 ,... , βk are causal effects, Wji are control variables, and 1 ui has a conditional mean that does not depend on the X ’s given the W ’s; that is, E(ui | X1i , X2i ,... , Xki , W1i , W2i ,... , Wri ) = E(ui | W1i , W2i ,... , Wri ) 2 (X1i , X2i ,... , Xki , W1i , W2i ,... , Wri , Yi ) are i.i.d. draws from their joint distribution. 3 Large outliers are unlikely: (X1i , X2i ,... , Xki , W1i , W2i ,... , Wri , Yi ) have nonzero finite fourth moments 4 There is no perfect multicollinearity. ⇒ If assumptions 1-4 hold, then in large samples the OLS estimators of interest, β̂0 , β̂1 ,... , β̂k are jointly normally distributed, and each β̂j ∼ N (βj ; σβ̂2 ) BUT β̂k+1 , β̂k+2 ,... , β̂k+r might be j biased! Introduction References 34 / 35 Material I – Textbooks: - Introduction to Econometrics, 4th Edition, Global Edition, by Stock and Watson – Chapter 6. - Introductory Econometrics, 5th Edition, A Modern Approach, by Jeff. Wooldridge – Chapter 3. Introduction References 35 / 35